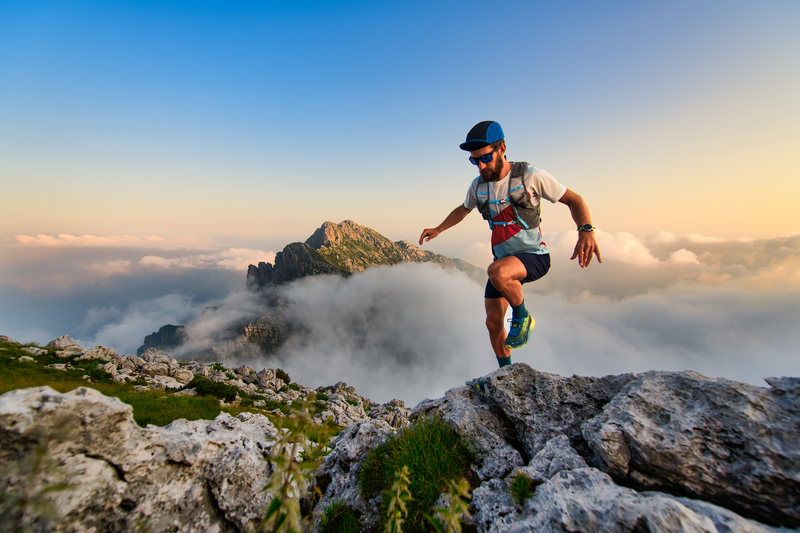
94% of researchers rate our articles as excellent or good
Learn more about the work of our research integrity team to safeguard the quality of each article we publish.
Find out more
ORIGINAL RESEARCH article
Front. Aging Neurosci.
Sec. Parkinson’s Disease and Aging-related Movement Disorders
Volume 17 - 2025 | doi: 10.3389/fnagi.2025.1549589
This article is part of the Research Topic Complex network dynamics of cognitive processing in health and disease: Current knowledge and future research View all articles
The final, formatted version of the article will be published soon.
You have multiple emails registered with Frontiers:
Please enter your email address:
If you already have an account, please login
You don't have a Frontiers account ? You can register here
Background: There is a huge heterogeneity of magnetic resonance imaging findings in Parkinson's disease (PD)with impulse control disorders (ICDs) studies. Here, we hypothesized that brain regions identified by structural and functional imaging studies of PD with ICDs could be reconciled in a common network.Methods: In this study, an initial systematic literature review was conducted to collect and evaluate whole-brain functional and structural magnetic resonance imaging studies related to PD with ICDs. We subsequently utilized the Human Connectome Project (HCP) dataset (n = 1093) and a novel functional connectivity network mapping (FCNM) technique to identify a common brain network affected in PD with ICDs.Results: A total of 19 studies with 25 contrasts, incorporating 345 individuals with PD and ICDs, and 787 individuals with PD without ICDs were included in the analysis. By using the HCP dataset and a novel FCNM technique, we ultimately identified that the aberrant neural networks predominantly involve the default mode network (middle and inferior temporal gyrus, anterior cingulate cortex, angular gyrus) and subcortical network (caudate nucleus).Conclusions: This study suggests that the heterogeneous neuroimaging findings in PD with ICDs can be attributed to shared abnormalities in the default mode and subcortical networks. These dysfunctions are associated with impaired self-regulation, decision-making, and heightened impulsivity in PD with ICDs. Our findings integrate diverse neuroimaging results from previous studies, providing a clearer understanding of the neurobiological mechanisms underlying PD with ICDs at a network level.
Keywords: Parkinson's disease, Impulse Control Disorders, Network localization, voxel-based morphometry, functional connectivity network mapping
Received: 21 Dec 2024; Accepted: 17 Mar 2025.
Copyright: © 2025 Hucheng, Gu, Sun, Zhang, Pan and Dai. This is an open-access article distributed under the terms of the Creative Commons Attribution License (CC BY). The use, distribution or reproduction in other forums is permitted, provided the original author(s) or licensor are credited and that the original publication in this journal is cited, in accordance with accepted academic practice. No use, distribution or reproduction is permitted which does not comply with these terms.
* Correspondence:
PingLei Pan, Nanjing Medical University, Nanjing, China
Disclaimer: All claims expressed in this article are solely those of the authors and do not necessarily represent those of their affiliated organizations, or those of the publisher, the editors and the reviewers. Any product that may be evaluated in this article or claim that may be made by its manufacturer is not guaranteed or endorsed by the publisher.
Research integrity at Frontiers
Learn more about the work of our research integrity team to safeguard the quality of each article we publish.