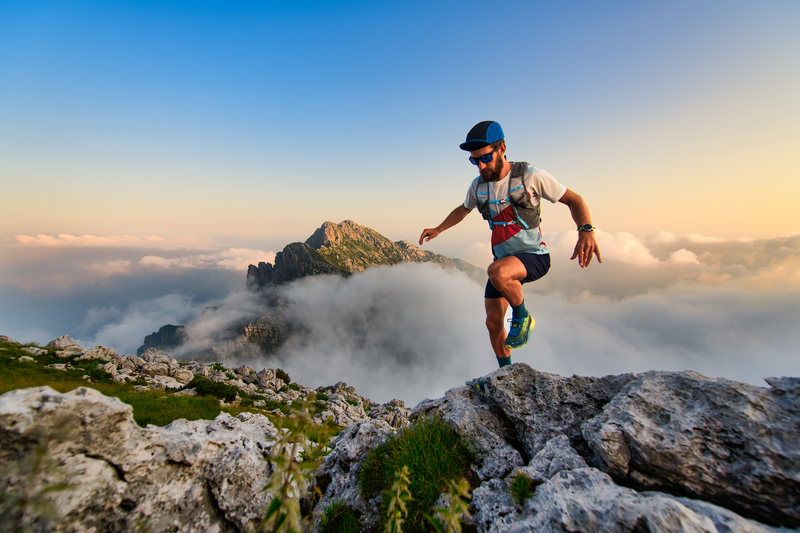
95% of researchers rate our articles as excellent or good
Learn more about the work of our research integrity team to safeguard the quality of each article we publish.
Find out more
SYSTEMATIC REVIEW article
Front. Aging Neurosci.
Sec. Alzheimer's Disease and Related Dementias
Volume 17 - 2025 | doi: 10.3389/fnagi.2025.1547727
The final, formatted version of the article will be published soon.
You have multiple emails registered with Frontiers:
Please enter your email address:
If you already have an account, please login
You don't have a Frontiers account ? You can register here
Frontotemporal dementia (FTD) is a neurodegenerative disorder characterized by progressive degeneration of the frontal and temporal lobes, leading to significant changes in personality, behavior, and language abilities. Early and accurate differential diagnosis between FTD, its subtypes, and other dementias, such as Alzheimer's disease (AD), is crucial for appropriate treatment planning and patient care. Machine learning (ML) techniques have shown promise in enhancing diagnostic accuracy by identifying complex patterns in clinical and neuroimaging data that are not easily discernible through conventional analysis.This systematic review, following PRISMA guidelines and registered in PROSPERO, aimed to assess the strengths and limitations of current ML models used in differentiating FTD from other dementias neurological disorders. A comprehensive literature search from 2013 to 2024 identified 25 eligible studies involving 6471 6544 patients with dementia, including 3024 2984 with FTD, and 3324 3437 with AD, 103 mild cognitive impairment (MCI) and 20 Parkinson's disease dementia or probable dementia with Lewy bodies (PDD/DLBPD).The review found that Support Vector Machines (SVMs) were the most frequently used ML technique, often applied to neuroimaging and electrophysiological data. Deep learning methods, particularly convolutional neural networks (CNNs), have also been increasingly adopted, demonstrating high accuracy in distinguishing FTD from other dementias. The integration of multimodal data, including neuroimaging, EEG signals, and neuropsychological assessments, has been suggested to enhance diagnostic accuracy. However, limitations such as small sample sizes, class imbalance, and lack of standardization across studies were noted.1 Dattola et al.Although the findings highlight the promising role of ML techniques in advancing the differential diagnosis of FTD. further research with larger, standardized datasets is needed to improve generalizability and clinical applicability.ML techniques showed strong potential for improving FTD diagnosis, but challenges like small sample sizes, class imbalance, and lack of standardization limit generalizability. Future research should prioritize the development of standardized protocols, larger datasets, and explainable AI techniques to facilitate the integration of ML-based tools into real-world clinical practice.
Keywords: Frontotemporal Dementia, machine learning, differential diagnosis, Alzheimer's disease, Neuroimaging, deep learning, Support Vector Machines, Convolutional Neural Networks
Received: 18 Dec 2024; Accepted: 31 Mar 2025.
Copyright: © 2025 Dattola, Ielo, Varone, Cacciola, Quartarone and Bonanno. This is an open-access article distributed under the terms of the Creative Commons Attribution License (CC BY). The use, distribution or reproduction in other forums is permitted, provided the original author(s) or licensor are credited and that the original publication in this journal is cited, in accordance with accepted academic practice. No use, distribution or reproduction is permitted which does not comply with these terms.
* Correspondence:
Augusto Ielo, IRCCS Centro Neurolesi Bonino-Pulejo, Messina, Italy
Disclaimer: All claims expressed in this article are solely those of the authors and do not necessarily represent those of their affiliated organizations, or those of the publisher, the editors and the reviewers. Any product that may be evaluated in this article or claim that may be made by its manufacturer is not guaranteed or endorsed by the publisher.
Research integrity at Frontiers
Learn more about the work of our research integrity team to safeguard the quality of each article we publish.