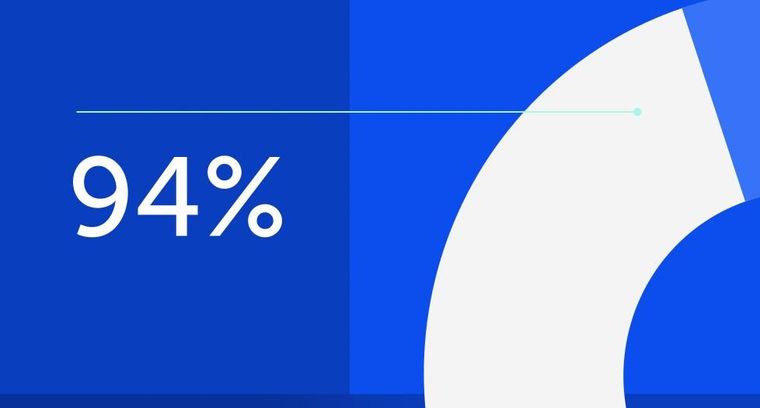
94% of researchers rate our articles as excellent or good
Learn more about the work of our research integrity team to safeguard the quality of each article we publish.
Find out more
SYSTEMATIC REVIEW article
Front. Aging Neurosci., 10 April 2025
Sec. Alzheimer's Disease and Related Dementias
Volume 17 - 2025 | https://doi.org/10.3389/fnagi.2025.1547727
Introduction: Frontotemporal dementia (FTD) is a neurodegenerative disorder characterized by progressive degeneration of the frontal and temporal lobes, leading to significant changes in personality, behavior, and language abilities. Early and accurate differential diagnosis between FTD, its subtypes, and other dementias, such as Alzheimer's disease (AD), is crucial for appropriate treatment planning and patient care. Machine learning (ML) techniques have shown promise in enhancing diagnostic accuracy by identifying complex patterns in clinical and neuroimaging data that are not easily discernible through conventional analysis.
Methods: This systematic review, following PRISMA guidelines and registered in PROSPERO, aimed to assess the strengths and limitations of current ML models used in differentiating FTD from other neurological disorders. A comprehensive literature search from 2013 to 2024 identified 25 eligible studies involving 6,544 patients with dementia, including 2,984 with FTD, 3,437 with AD, 103 mild cognitive impairment (MCI) and 20 Parkinson's disease dementia or probable dementia with Lewy bodies (PDD/DLBPD).
Results: The review found that Support Vector Machines (SVMs) were the most frequently used ML technique, often applied to neuroimaging and electrophysiological data. Deep learning methods, particularly convolutional neural networks (CNNs), have also been increasingly adopted, demonstrating high accuracy in distinguishing FTD from other dementias. The integration of multimodal data, including neuroimaging, EEG signals, and neuropsychological assessments, has been suggested to enhance diagnostic accuracy.
Discussion: ML techniques showed strong potential for improving FTD diagnosis, but challenges like small sample sizes, class imbalance, and lack of standardization limit generalizability. Future research should prioritize the development of standardized protocols, larger datasets, and explainable AI techniques to facilitate the integration of ML-based tools into real-world clinical practice.
Systematic review registration: https://www.crd.york.ac.uk/PROSPERO/view/CRD42024520902.
Neurodegenerative dementias are an increasingly common cause of mortality and disability worldwide, especially among the elderly. Alzheimer's disease (AD) is the most common cause of dementia (Feigin et al., 2019). However, recent epidemiological studies and the refinement of clinical criteria have revealed that frontotemporal dementia (FTD) is also a widespread form (Nuytemans et al., 2024).
While the exact etiopathogenesis of this complex and multifaceted disorder is unknown, FTD has been linked to various genetic mutations. Nearly 40% of all FTD cases are familial, meaning they occur in families with a history of the disorder (Seelaar et al., 2010). Several genes have been implicated in FTD, including MAPT, GRN, and C9orf72. Mutations in these genes can lead to the abnormal accumulation of tau or TDP-43 proteins in neurons, which is thought to contribute to the disease process (Giunta et al., 2021; Kertesz and Munoz, 2004).
FTD differs from other types of dementia, such as vascular dementia and Lewy body dementia (DLB), in its clinical presentation and underlying pathology. FTD is characterized by the progressive degeneration of the frontal and temporal lobes, leading to profound changes in personality, behavior, language abilities, along with occasional physical symptoms, including tremor, rigidity, akinesia, etc. (Neary et al., 1998). In contrast, Vascular dementia arises from cerebrovascular damage, progressing stepwise with abrupt onset after strokes (Gorelick et al., 2016), while DLB features visual hallucinations, Parkinsonism (e.g., tremors, rigidity), and fluctuating cognition (Walker et al., 2015).
FTD also differs from AD, which is commonly mistaken for it. While AD is typically associated with prominent memory loss, FTD primarily manifests through significant changes in social and personal behavior, neglect of personal care, impaired judgment, and aphasia (Ratnavalli et al., 2002). In addition to these distinct symptom profiles, FTD and AD present different patterns of brain atrophy. AD is primarily characterized by medial temporal lobe atrophy, particularly affecting the hippocampus and entorhinal cortex, regions critical for memory processing (Gold et al., 2012). Conversely, FTD predominantly involves the frontal and anterior temporal lobes, with a more pronounced atrophy pattern in the orbitofrontal cortex, anterior cingulate, and insula, depending on the specific clinical variant (Rohrer, 2012; Yu et al., 2021). These distinct symptom profiles are crucial for accurate diagnosis and subject specific management of each dementia type.
Furthermore, FTD is frequently underdiagnosed due to clinical overlap with various psychiatric disorders. The early symptoms of FTD, such as personality changes, impulsivity, and apathy, often mimic conditions like bipolar disorder, schizophrenia, or major depressive disorder, leading to misdiagnosis and delays in appropriate treatment (Antonioni et al., 2023; Chaudhary and Duggal, 2014).
FTD encompasses several subtypes, each affecting different aspects of behavior or language abilities. These include behavioral variant FTD (bvFTD), semantic variant primary progressive aphasia (svPPA), and nonfluent/agrammatic variant PPA (nfvPPA) (Gorno-Tempini et al., 2011). The updated clinical diagnostic criteria for the bvFTD by Rascovsky and colleagues (Rascovsky et al., 2011) highlight behavioral and cognitive symptoms to better distinguish bvFTD from AD and other dementias. bvFTD is characterized by prominent changes in behavior, personality, and social conduct. Individuals with bvFTD may exhibit disinhibition, impulsivity, apathy, and loss of empathy (Williams et al., 2005). These changes can have a profound impact on personal and social relationships, often leading to challenges in maintaining employment and engaging in daily activities. Behavioral disturbances can be distressing for both the affected individuals and their caregivers, necessitating a multidisciplinary approach to care. svPPA, on the other hand, primarily affects language skills. Individuals with svPPA experience difficulties in understanding and using words, as well as a decline in semantic memory, which is the ability to recognize and understand the meaning of words and objects. This subtype often leads to profound communication challenges, affecting not only verbal expression but also written language and comprehension (Josephy-Hernandez et al., 2023). As a result, individuals with svPPA may struggle to convey their thoughts and feelings, impacting their ability to maintain social connections and participate in activities that require effective communication (Hodges and Patterson, 2007). In nfvPPA, patients have difficulty producing speech but can still understand language. NfvPPA can lead to frustration and social withdrawal as communication becomes increasingly challenging. The impact of nfvPPA extends beyond verbal communication, affecting daily activities that require coordination and motor skills (Gorno-Tempini et al., 2004).
Currently, there is no cure for FTD. In terms of treatment, pharmacological interventions for FTD remain limited, with symptomatic management focusing on the alleviation of behavioral and cognitive symptoms. For example, selective serotonin reuptake inhibitors (SSRIs) can help manage behavioral symptoms, while speech and language therapy can support those with language difficulties (Gorno-Tempini et al., 2011). Non-pharmacological approaches, including behavioral interventions, cognitive rehabilitation, and caregiver support, play a crucial role in enhancing quality of life for patients with FTD and their families. Advances in diagnostic criteria, genetic discoveries, and neuroimaging modalities have enhanced our understanding of FTD heterogeneity and facilitated early diagnosis and disease monitoring. Despite therapeutic challenges, ongoing research efforts hold promise for the development of targeted treatments to mitigate the impact of FTD on affected individuals and their families, and, ultimately, a cure for this disease.
Machine learning, a subset of Artificial Intelligence (AI), has made notable progress in recent years, enhancing clinical applications in the diagnosis, prognosis, and treatment of neurodegenerative disorders, including AD and behavioral variant such as bvFTD. Through the application of advanced mathematical models, ML enables algorithms to learn from training data and identify patterns in new datasets. In the investigation of AD and bvFTD, ML has been used extensively to extract relevant information from complex neuroimaging data, resulting in accurate and reliable diagnostic models. This progress has led to the development of robust diagnostic tools for these conditions (Habes et al., 2020). As a consequence, ML has gained significant attention in the medical field as a tool for improving diagnostic accuracy, personalizing treatment plans, and optimizing patient outcomes. Moreover, ML's ability to iteratively refine its performance and uncover hidden patterns within data highlights its potential to transform healthcare and advance precision medicine (Rajkomar et al., 2019).
Various neuroimaging techniques, when combined with ML approaches, have proven effective in the diagnosis of neurodegenerative diseases. Structural magnetic resonance imaging (MRI), which is able to capture morphometric brain features, combined with traditional ML algorithms such as logistic regression classifier, has demonstrated high accuracy in distinguishing bvFTD and AD, from healthy controls (HCs) (Bachli et al., 2020). In addition to MRI, electroencephalography (EEG) has shown promise as a complementary tool for differential diagnosis, particularly in revealing unique patterns of neural activity (Miltiadous et al., 2021). Researchers are also leveraging ML methods for pattern analysis to enhance the classification and diagnosis of FTD from multimodal data and feature extraction techniques (Ducharme, 2023). Although ML-based approaches using MRI have achieved high accuracy in distinguishing dementia patients from controls, a key limitation is the generalizability of these models across diverse populations and clinical settings (Rathore et al., 2017). Moreover, the complexity of image-derived features often impedes the seamless translation of these findings into routine clinical practice, highlighting the need for streamlined, interpretable solutions that bridge research advancements with real-world diagnostic workflows.
In particular, deep learning (DL), a subset of ML, offers solutions to some of the limitations associated with preprocessing raw data, allowing for the exploration of sample complexity to a greater extent. Recent research indicates that deep network architectures, comparable to traditional ML models, can effectively address the differential diagnosis of neurodegenerative diseases (Spasov et al., 2019; Basaia et al., 2019; Hu et al., 2021). DL models have shown good performance at mining MRI features by utilizing the extensive depth, width, and inter-layer connections of neural networks. This capability allows them to extract hierarchical features that represent different levels of abstraction in a data-driven manner. As a result, these models significantly improve the accuracy and robustness of diagnostic applications. However, one of the major concerns with AI tools used to assist clinicians in diagnosis, evaluation, and treatment planning is the lack of interpretability of current models. DL neural networks are often perceived as opaque, with their intricate data processing making it nearly impossible to figure out how they arrive at predictions, such as class probabilities. In response, the field of explainable AI (XAI) (Gunning et al., 2019; Guidotti et al., 2018) has introduced pioneering methods like Local Interpretable Model-agnostic Explanations (LIME) (Ribeiro et al., 2016) and SHapley Additive exPlanations (SHAP) (Lundberg and Lee, 2017), which offer clear, localized insights into the decision-making process of black-box models by attributing specific contributions of features to individual predictions, thereby enhancing transparency and enabling a deeper understanding of AI behavior.
The objective of this study is to systematically assess the strengths and limitations of current AI models used in the differential diagnosis of FTD. Notably, while AD has been extensively studied in the context of ML applications (Kishore and Goel, 2024; Shukla et al., 2023; Moorthy et al., 2023), FTD remains underexplored. Specifically, FTD presents unique diagnostic challenges due to its overlap with other neurological disorders and its variable clinical manifestations across subtypes. Through a comprehensive analysis, we sought to assess their performance relative to existing literature and identify opportunities for further refinement. By providing an overview of the current state of AI applications in this field, this work seeks to inform both the clinical and research communities, while highlighting research gaps and potential directions for future advancements.
The literature search followed the Preferred Reporting Items for Systematic Reviews and Meta-Analyses (PRISMA) guidelines (Page et al., 2021). The systematic review is registered in the PROSPERO database with the identifier CRD42024520902.
To ensure the selection of relevant and high-quality studies for this systematic review, both inclusion and exclusion criteria were established. Studies were eligible for inclusion if they met the following criteria: (1) studies applying ML techniques specifically for the differential diagnosis between FTD and other neurological disorders, without predefining which disorders were considered; (2) studies employing supervised, unsupervised, or semi-supervised learning methods; (3) studies using clinical data (e.g., neuroimaging, neuropsychological, or biomarker data) for differential diagnosis; (4) studies involving human participants; (5) original research articles published in peer-reviewed journals; (6) papers written in English; (7) studies published from 2013 to 2024. Review articles, meta-analyses, systematic reviews, conference abstracts, editorials, and case reports were excluded.
The literature search was conducted across the PubMed, Web of Science, and Embase databases, covering studies from 2013 to 2024. The selected range was chosen to capture the most recent advancements in the field while ensuring the inclusion of studies that reflect contemporary methodologies, technologies, and evolving theoretical frameworks. Studies published prior to 2013 may not fully represent the current state of research or the significant developments in the area under review. By focusing on the past decade, we aim to incorporate the latest findings that are most relevant to current practices and emerging trends. Given the highly specialized nature of the topic and the inclusion of databases that do not use MeSH indexing, MeSH terms were not utilized. Instead, a keyword-based search approach was adopted, targeting the title, abstract, and, where available, keyword fields. The search terms used were: (“frontotemporal dementia” OR “frontotemporal degeneration” OR “frontotemporal neurodegeneration” in Title) AND (“machine learning” OR “deep learning” OR “neural networks” OR “classifier” in Title, Abstract, Keywords) OR (“differential diagnosis” in Title) AND (“frontotemporal dementia” OR “frontotemporal degeneration” OR “frontotemporal neurodegeneration” in Title) AND (“machine learning” OR “deep learning” OR “neural networks” OR “classifier” in Title, Abstract, Keywords).
The study selection process was carried out in two phases. First, two independent reviewers screened the titles and abstracts of the records obtained from the search, applying the predefined inclusion and exclusion criteria, as reported in the previous section. In the second phase, the full texts of potentially eligible studies were independently reviewed by the same two reviewers for final inclusion. Figure 1 provides a PRISMA flow diagram that outlines the entire study selection process, including the number of studies screened, assessed for eligibility, and included, as well as reasons for exclusions at each stage. Data extraction from the included studies was performed using a customized spreadsheet that captured various study characteristics. Additionally, the two reviewers independently extracted information relevant to assessing the risk of bias in each study. Any differences that arose during the screening phase and data extraction between the two reviewers were resolved through discussion until a consensus was reached.
The following data were extracted from each study: 1) study details, including the first author and year of publication; 2) population characteristics, such as sample size, sub-groups, and age; 3) machine learning methods used; 4) key results and findings relevant to the review's focus; and 5) the outcomes of the risk of bias assessment.
The risk of bias for each study included in this systematic review was assessed using the National Institutes of Health (NIH) quality assessment tool for observational cohort and cross-sectional studies. Studies were rated as “Good,” “Fair,” or “Poor” based on their compliance with various criteria, such as study selection, comparability, exposure, and outcomes. These criteria included the clarity of the research question, the definition of the study population, the selection and measurement of exposure and outcomes, the consideration of potential confounders, the statistical analysis methods, and the reporting of participant recruitment and retention rates. Any differences in scoring between the reviewers were resolved through discussion until agreement was reached.
In this review, the performance of ML models for the differential diagnosis of FTD is mainly assessed using accuracy, which was the common evaluation measure among all the included studies. Additionally, the area under the receiver operating characteristic curve (AUC-ROC) is employed as a general measure of a model's ability to discriminate between FTD and non-FTD cases.
The implemented search strategy identified 68 articles excluding duplicates, non-articles and non-English records. After reviewing the titles and abstracts, 25 studies met the eligibility criteria and were selected for full-text screening (Figure 1). All 25 studies were subsequently included in the review. Table 1 provides detailed information on the articles, including demographic data of participants, the aim of the study, information on the ML model, and findings.
The included studies were evaluated using the 14-item NIH quality assessment tool for observational cohort and cross-sectional studies. The evaluation revealed a strong methodological framework, with all studies clearly articulating their objectives. Each study effectively assessed varying levels of exposure in relation to the outcomes and consistently applied well-defined, validated, and reliable exposure and outcome measures across participants. The overall quality of the studies was predominantly rated as “Good,” indicating that almost all demonstrated good methodological quality (Table 2). Only one study received a “Fair” rating due to the lack of demographic data of the participants involved, which impacts the study's transparency and limits its generalizability.
The review includes a total of 6,544 patients with dementia (mean age: 67.94 ± 5.24 years), involving 2,984 FTD (mean age: 66.18 ± 4.17 years), 3,437 AD (mean age: 69.5 ± 5.81 years), 103 mild cognitive impairment (MCI; mean age: 71.2 ± 7.4 years), 20 Parkinson's disease dementia or probable dementia with Lewy bodies (PDD/DLBPD; mean age: 74.8 ± 8.5 years). Mean age was calculated from the included studies reporting this information (24 out of 25). Most of the papers that met our inclusion criteria primarily focused on bvFTD, likely due to its higher prevalence in both clinical practice and research. To assess the severity status of participants with dementia across the reviewed articles, the most commonly used scales were the Mini-Mental State Examination (MMSE) and the Clinical Dementia Rating (CDR). These scales were mainly employed to evaluate the cognitive impairment in FTD, bvFTD, and AD. In four studies, other scales like the Montreal Cognitive Assessment (MoCA) and the Addenbrooke's Cognitive Examination (ACE) were also used.
To assess the patterns associated with FTD, AD, and other dementias, various neuroimaging, electrophysiological, cognitive, and behavioral data were analyzed across the included studies.
SVMs, a widely used set of supervised ML algorithms, have emerged as the most frequently employed ML techniques in the reviewed studies (Miltiadous et al., 2021; Garcia-Gutierrez et al., 2022; Garćıa-Gutierrez et al., 2022; Pérez-Millan et al., 2023, 2024; Garn et al., 2017; Wang et al., 2024; Birba et al., 2022; Maito et al., 2023; Möller et al., 2016; Lage et al., 2021; Raamana et al., 2014; Rostamikia et al., 2024; Ajra et al., 2023; Ma et al., 2020). SVMs are favored for their robustness in handling high-dimensional data and their effectiveness in both binary and multi-class classification tasks. They have been applied to a diverse array of data types, including neuroimaging data such as structural MRI features (Pérez-Millan et al., 2023, 2024; Möller et al., 2016) and FDG-PET imaging (Garćıa-Gutierrez et al., 2022). Additionally, SVMs have been applied to EEG data (Garn et al., 2017; Wang et al., 2024; Rostamikia et al., 2024) and to cognitive and behavioral assessments (Garcia-Gutierrez et al., 2022; Maito et al., 2023).
In two studies, SVMs were combined with feature selection or dimensionality reduction techniques to enhance performance. Particularly, Principal Component Analysis (PCA) was employed to reduce feature dimensionality before classification (Pérez-Millan et al., 2023; Garn et al., 2017). These combinations aimed to improve the classifier's efficiency and accuracy by focusing on the most informative features.
Other ML algorithms were used across the reviewed literature. k-Nearest Neighbors (KNN), a straightforward non-parametric ML technique, was employed in seven studies (Miltiadous et al., 2021; Garćıa-Gutierrez et al., 2022; Lage et al., 2021; Rostamikia et al., 2024; Ajra et al., 2023; Lal et al., 2024; D́ıaz-Álvarez et al., 2022), often applied to cognitive, behavioral, and EEG data, sometimes in combination with feature selection methods. Several studies (Miltiadous et al., 2021; Garcia-Gutierrez et al., 2022; Garćıa-Gutierrez et al., 2022; Maito et al., 2023; Rostamikia et al., 2024; Lal et al., 2024) utilized Random Forests, employing ensemble learning to improve classification performance and robustness to overfitting. Other ensemble methods were used across studies: XGBoost (Birba et al., 2022; Lal et al., 2024; Sadeghi et al., 2024), AdaBoost (Garcia-Gutierrez et al., 2022; Garćıa-Gutierrez et al., 2022), Gradient Boosting (Garcia-Gutierrez et al., 2022; Garćıa-Gutierrez et al., 2022) and Extra Trees (Lal et al., 2024). Naive Bayes classifiers, a family of probabilistic algorithms, were applied in various studies (Miltiadous et al., 2021; Garcia-Gutierrez et al., 2022; Garćıa-Gutierrez et al., 2022; Rostamikia et al., 2024; D́ıaz-Álvarez et al., 2022; Wang et al., 2016). They proved effective in modeling of feature distributions, particularly with neuropsychological and imaging data. Elastic net regression was employed in one study (Bouts et al., 2018), offering a balance between feature selection and model complexity control. Linear discriminant analysis (LDA), a classification technique that maximizes class separability, was used in Kim et al. (2019) after applying a Laplace Beltrami operator and PCA, for noise removal and feature dimension reduction, respectively.
Deep learning models, including Convolutional Neural Networks (CNNs), were employed in six studies Hu et al. (2021); Ajra et al. (2023); Ma et al. (2020, 2024); Nguyen et al. (2023); Rogeau et al. (2024). These approaches use deep neural architectures to automatically identify patterns in raw data without requiring manual feature selection. For example, DL-based methods were applied to volumetric data derived from structural MRI (Hu et al., 2021; Ma et al., 2020, 2024; Nguyen et al., 2023). In Ma et al. (2020), the authors implemented a framework using Generative Adversarial Networks (GANs), a DL model designed to generate new data from an existing dataset, for data augmentation, combined with deep neural networks (DNNs) for classification. A 3D CNN was applied to FDG-PET scans (Rogeau et al., 2024) and shallow CNNs classified EEG-based spectral-temporal features and functional connectivity patterns, demonstrating versatility across data modalities (Ajra et al., 2023).
The performance of the ML models was primarily evaluated using metrics such as accuracy, sensitivity, specificity, and AUC-ROC. Accuracy measures the overall correctness of the model's predictions by calculating the proportion of correctly classified cases out of the total cases, and was reported in most studies. Sensitivity and specificity assess the model's ability to correctly identify true positives and true negatives, respectively. The AUC is a widely used metric for evaluating the discriminative ability of classifiers, with values closer to one indicating better performance. Some studies (Garcia-Gutierrez et al., 2022; Garćıa-Gutierrez et al., 2022; Maito et al., 2023; D́ıaz-Álvarez et al., 2022; Ajra et al., 2023; Lal et al., 2024; Pérez-Millan et al., 2024; Sadeghi et al., 2024) also employed metrics like F1-score, which balances sensitivity and precision by computing the harmonic mean of the two. The F1-score is especially valuable in datasets with class imbalance, ensuring that both false positives and false negatives are taken into account when evaluating model performance. K-fold, leave-one-out and nested cross-validation techniques were commonly used to assess model robustness and generalizability. These methods helped prevent overfitting and provided a more reliable estimate of model performance.
Table 3, Figure 2 show the frequency of the ML approaches used across the included studies.
Many reviewed papers employed multiple ML methods simultaneously within their analyses, allowing for direct comparisons. In this section, we summarized the best-performing ML models for each included study, highlighting the most effective techniques contributing to improved diagnostic accuracy. The reviewed studies are grouped into sections based on the best-performing ML method employed.
SVM classifiers have been extensively employed to enhance the differential diagnosis between FTD, AD, and HC. Overall, the accuracies reported for SVM-based methods ranged from approximately 60%–100%, with most studies achieving accuracies between 82% and 94.5%. This variation reflects differences in sample sizes, data modalities, and feature selection techniques, while demonstrating the strong performance of SVM classifiers in the differential diagnosis of neurodegenerative diseases. The included studies are organized into subsections, according to the type of data used for ML.
Structural MRI data analyzed using SVMs in Möller et al. (2016) resulted in accuracies of 85% for FTD vs. HC, and 82% for FTD vs. AD, demonstrating high predictive power across independent datasets. In Raamana et al. (2014), non-linear SVMs with Gaussian kernel were employed for direct three-way classification among AD, bvFTD, and normal controls. Using ventricular displacement features, the model achieved a weighted AUC of 0.765, with pairwise AUCs of 0.938 for bvFTD vs. HC, and 0.653 for bvFTD vs. AD, underscoring the diagnostic potential of ventricular morphology while also highlighting its limitations in distinguishing between dementia subtypes. In Pérez-Millan et al. (2023) the authors applied SVM classifiers to both cross-sectional and longitudinal MRI data, obtaining improved classification accuracy for FTD vs. HC in longitudinal analyses (87.8%), although distinguishing between AD and FTD remained challenging in both analyses with accuracies around 60%.
The potential of EEG features combined with SVMs was demonstrated in Garn et al. (2017), where classifiers achieved 100% accuracy in pairwise comparisons among groups of 20 AD, 20 PDD or dementia with Lewy bodies (DLB), and 21 FTD. The study underscores the efficacy of non-invasive EEG markers, although the authors highlighted the need for further studies using larger numbers of patients. In Wang et al. (2024), the integration of aperiodic EEG components of power spectral density with SVM classification significantly enhanced the differentiation between FTD and AD. The authors separated the raw power into periodic and aperiodic components, with the periodic components represented by periodic power and the aperiodic components represented by offsets and exponents. The study showed that using the combination of aperiodic parameters and the theta periodic power as features resulted in the best classification performance (mean AUC = 0.73 ± 0.12). In Rostamikia et al. (2024), the authors used SVM classifiers on EEG features, obtaining 93.5% accuracy for diagnosing dementia (FTD and AD vs. HC) and 87.8% for differentiating FTD from AD, underscoring the versatility of SVMs across different data types.
In Garcia-Gutierrez et al. (2022), SVM models utilizing neuropsychological assessments, adjusted by demographic factors, as features, achieved accuracies of 91.6% for FTD from HC, and 84.5% for differentiating AD from FTD. Furthermore, in Garćıa-Gutierrez et al. (2022), the authors showed that combining PET imaging and cognitive data using a multimodal ML approach including SVM binary classifiers, obtained an accuracy of 92.6% in distinguishing between FTD, AD and HC. Additionally, in Pérez-Millan et al. (2024), the integration of MRI-derived structural data, cerebrospinal fluid biomarkers, and age into SVM classifiers improved the classification accuracy between AD and FTD to 88.5%, providing a probabilistic assessment of diagnostic confidence. The accuracy of classification between FTD and HCs was 86.5%.
Deep learning methods, particularly CNNs, have also been effective in this domain. In Nguyen et al. (2023), a Deep Grading method incorporating U-Nets and a multi-layer perceptron (MLP) achieved an overall accuracy of 86.0% (87.9% on an external dataset) when distinguishing FTD, AD, and HC using MRI-based structural data. The accuracy for binary classification between FTD and AD was 94.6% (86.1% for the external validation dataset). Ajra et al. (2023) utilized a shallow CNN with four estimation methods of EEG-derived functional connectivity, achieving an accuracy of 94.54% in distinguishing from FTD, AD and HC when using the Amplitude Envelope Correlation (AEC) without any thresholding method. Similarly, in Ma et al. (2020), the authors developed a multi-scale, multi-type deep neural network (MMDNN) augmented with GANs, which achieved an accuracy of 88.28% in classifying FTD, AD, and HCs based on structural MRI features.
Hu et al. (2021) demonstrated the efficacy of deep learning applied to raw MRI data without any preprocessing or manual intervention by medical experts, achieving classification accuracies of 93.45% for distinguishing FTD from HCs, and 93.05% for differentiating FTD from AD. These findings underscore the potential of CNNs to autonomously capture intricate patterns within imaging data, highlighting their capability to obviate the need for extensive preprocessing and manual feature extraction in neuroimaging analyses. In Rogeau et al. (2024), a 3D CNN model using FDG-PET scans outperformed clinicians' interpretation in distinguishing between FTD, AD, and HCs, achieving an overall accuracy of 89.8%. In a complementary analysis with FTD and AD data only, model accuracy was 87.2%. Ma et al. (2024) introduced an explainable DNN that classified FTD subtypes with an overall balanced accuracy of 83.6%, providing insights into the structural markers specific to each subtype. To enhance model transparency and interpretability, they utilized an XAI technique called “Integrated Gradient”, which provides importance scores to each MRI input feature, highlighting their individual contributions to the model's predictions.
These DL studies reported accuracies ranging from 86.0% to 94.6%, indicating the potential of neural networks in employing complex neuroimaging and electrophysiological data for accurate diagnosis.
Random Forests algorithms also demonstrated strong performance. In Maito et al. (2023), a Random Forests model achieved an accuracy of 93.2% and an AUC of 0.965 in differentiating FTD from AD using routine clinical and cognitive assessments in Latin American populations. Miltiadous et al. (2021) reported that Random Forests reached an accuracy of 97.7% for FTD vs. AD classification based on EEG features using a 10-fold cross-validation method.
Gradient boosting methods, such as XGBoost used in Sadeghi et al. (2024), combined fMRI time-course data with clinical and demographic variables (except for age) to achieve a balanced accuracy of 91.1%, significantly improving the classification of AD, FTD, mild cognitive impairment (MCI), and HCs compared to imaging data alone. A classifier based on an XGBoost was also used by Birba et al., who built a classifier algorithm using interoceptive EEG features, structural MRI measures, and functional connectivity markers to distinguish bvFTD from AD. The classifier achieved an accuracy of 82% and an AUC of 0.81, demonstrating the potential of integrating electrophysiological and neuroimaging biomarkers for reliable diagnosis Birba et al. (2022).
Naive Bayes classifiers were notably effective in certain studies. In Wang et al. (2016), the authors found that neuropsychological features analyzed with a Naive Bayes classifier achieved an accuracy of 62.39% in distinguishing AD from FTD, outperforming MRI-based measures (accuracy = 51.38). D́ıaz-Álvarez et al. (2022) used a BayesNet Naive classifier combined with genetic algorithm-based feature selection, achieving a high accuracy of 98.8% for FTD vs. AD using FDG-PET imaging.
KNN algorithm showed high efficacy in Lal et al. (2024), where it achieved an accuracy of 91% for FTD vs. AD, indicating its potential for EEG-based diagnostics. These results were achieved using SVD entropy for EEG features extraction, an XAI feature importance array, and 90% overlap for sliding windowing, In Lage et al. (2021), KNN was used on eye-tracking data achieving an accuracy of 92.46% for bvFTD vs. AD, outperforming SVM. KNN was also evaluated in D́ıaz-Álvarez et al. (2022), although it was outperformed by the BayesNet Naive classifier.
Other ML techniques were also employed. In Kim et al. (2019), LDA, a classical linear learning method, within a hierarchical classification framework achieved an accuracy of 90.8% in distinguishing FTD from AD, 86.9% between bvFTD and PPA, and 92.1% for nfvPPA vs svPPA. In Bouts et al. (2018) elastic net regression was used in a multiparametric model, achieving an accuracy of 77.7% (AUC = 0.81) for FTD vs. AD differentiation by integrating structural, diffusion tensor, and resting-state functional MRI measures.
A comparative analysis of ML techniques reveals distinct strengths and limitations in their application to the differential diagnosis of FTD (Table 4). Different models vary in terms of accuracy, interpretability, computational demands, and suitability for clinical implementation. DL models achieve high accuracy, particularly with neuroimaging data, but require large datasets and computational resources. In contrast, traditional ML methods such as SVMs, RF, and gradient boosting demonstrate strong performance with structured data like cognitive assessments and multimodal data. These models often require feature engineering but provide robust classification capabilities with more manageable computational demands. These variations highlight the importance of dataset composition, preprocessing, and feature selection in optimizing model performance.
This systematic review underscores the potential of ML techniques in improving the differential diagnosis of FTD, a critical challenge in clinical neurology.
Traditional diagnostic methods often rely on clinical assessments and neuropsychological tests, which may not capture subtle early-stage differences between these conditions (Bron et al., 2015; Vieira et al., 2017; Korolev et al., 2016). Early and accurate diagnosis and differentiation between FTD and other types of dementia, such as AD and PDD, are therefore essential for appropriate treatment planning and patient care. Indeed, different dementia types have distinct pathophysiological mechanisms and may respond differently to treatments.
By leveraging diverse data modalities such as neuroimaging and neuropsychological assessments, ML models offer a powerful tool for identifying subtle, disease-specific patterns that traditional methods may overlook. As it emerges from the revised papers, the performance of the ML algorithms often depends on the employed model and the data analyzed. Therefore the choice of the classification methodology can play a critical role in enhancing diagnostic performance across various types of dementia.
The ML techniques applied across the included studies are characterized by a predominance of SVMs and an increasing adoption of DL methods. SVMs have demonstrated consistent effectiveness in differentiating between FTD, AD, and HCs. The performance variation found across studies likely derives from heterogeneity in data sources, sample sizes, and features used.
While SVMs have remained a dominant choice due to their consistent effectiveness, recent years have seen a growing interest in DL models. These models, particularly those leveraging raw neuroimaging data, excel in identifying intricate patterns. This places them as competitive for multi-class tasks essential for real-world scenarios where clinicians need to differentiate among multiple neurodegenerative diseases. Notably, although several studies used DL approaches for multi-class classification, the highest accuracies are found in binary tasks (Nguyen et al., 2023; Hu et al., 2021).
Random Forests and Naive Bayes classifiers have demonstrated strong performance in binary classifications, with reported accuracies as high as 98.8% for FTD vs. AD, especially with neuroimaging data (D́ıaz-Álvarez et al., 2022; Miltiadous et al., 2021). However, these methods often lack the scalability and adaptability of DL models for complex datasets and were frequently outperformed by both DL techniques and SVMs. KNN algorithms also performed well, but one study reported that their efficacy is less robust than other approaches Garcia-Gutierrez et al. (2022); D́ıaz-Álvarez et al. (2022); Miltiadous et al. (2021); Rostamikia et al. (2024).
Regarding the features used by the ML studies analyzed in the review, integrating multimodal data, such as demographic, clinical, cognitive, structural and functional neuroimaging, and cerebrospinal fluid biomarker features, has been shown to improve diagnostic accuracy (Pérez-Millan et al., 2024; Bouts et al., 2018; Maito et al., 2023; Birba et al., 2022; Garćıa-Gutierrez et al., 2022; Sadeghi et al., 2024).
The application of ML approaches in the differential diagnosis of FTD from other types of dementia has shown great potential, particularly in the context of early diagnosis. Recent ML research in diagnosis has shifted from classifying a specific brain disease against controls to focusing on differential diagnosis. While earlier studies primarily relied on neuroimaging as a data source, current efforts emphasize the need of integrating multimodal data.
Although the reviewed studies demonstrate significant progress, several limitations exist. As reported in Table 1, many studies have small sample sizes, which may limit the generalizability of the models (Miltiadous et al., 2021; Garn et al., 2017; Wang et al., 2024; Birba et al., 2022; Lage et al., 2021; Raamana et al., 2014; Rostamikia et al., 2024; Ajra et al., 2023; Lal et al., 2024; Bouts et al., 2018). Class imbalance (Rahman and Davis, 2013) is another common issue found across the included articles. AD is consistently overrepresented compared to FTD and its subtypes, which can bias models toward better performance in AD classification. Recent research used GANs to address class imbalance in AD diagnosis. GANs have reconstructed missing PET images, improving classification performance on imbalanced datasets (Hu et al., 2021), and GAN-based oversampling methods have significantly enhanced brain disease diagnosis accuracy (Rezaei et al., 2020). Unsupervised GAN approaches detect AD at various stages by reconstructing adjacent brain MRI slices, achieving high diagnostic accuracy (Han et al., 2022). Additionally, GANs can generate synthetic brain MRI and PET images for different AD stages, addressing limited data in developing robust automated diagnosis models (Islam and Zhang, 2020). An interesting finding of our review is the prevalence of bvFTD in the examined sample. Further exploring different variants could provide more comprehensive insights into the differential diagnosis of FTD subtypes. Furthermore, the integration of longitudinal data could also improve the understanding of disease progression and enhance predictive modeling. Moreover, ethnic diversity represents a significant concern, as most studies rely on datasets from North America and Europe, with only a few incorporating data from Asia, Latin America, or other regions. This bias limits the generalizability of findings, potentially reducing diagnostic accuracy for diverse populations. Data source variability represents another challenge, particularly in MRI and PET-based studies, where different imaging protocols across centers can introduce inconsistencies. Although some papers implement harmonization techniques, many do not explicitly address these discrepancies, potentially impacting model performance.
In the context of clinical implementation, ML models must balance accuracy, interpretability, and computational efficiency. SVMs, RF, and LDA are the most clinically feasible due to their moderate computational requirements and interpretability, making them suitable for decision-support systems. Gradient boosting techniques, while effective, require careful tuning to prevent overfitting. Deep learning models, despite their accuracy, are challenging due to their black-box nature, high data demands, and computational cost, limiting their immediate integration into clinical practice. In this regard, the development of XAI techniques such as SHAP and LIME will be crucial for clinical adoption, as clinicians require transparency in decision-making processes. In our review, only two studies employed XAI techniques (Lal et al., 2024; Ma et al., 2024). XAI techniques can help elucidate how models make decisions, highlighting the most influential features and enabling clinicians to validate model outputs (Tjoa and Guan, 2020; Chaddad et al., 2023). XAI models can be broadly categorized into two approaches: model-agnostic (Jahan et al., 2023; Guan et al., 2022; Yousefzadeh et al., 2024) and model-specific (Umeda-Kameyama et al., 2021; Jahan et al., 2023). Model-agnostic methods, such as SHAP and LIME, provide general insights into model predictions by attributing outcomes to input feature contributions. Although SHAP quantifies feature importance, it remains in many respects a “black-box” method that often fails to highlight the interactions between features that drive model decisions (Al Olaimat et al., 2023; Brusini et al., 2024). In brain MRI analysis, for instance, SHAP identifies key features but does not always provide a clear, intuitive sense of how these features merge to shape predictions (Jahan et al., 2023). While model-agnostic techniques offer flexibility across diverse model types, model-specific methods focus on providing transparency and interpretability directly linked to individual AI models, particularly in DL and complex ML algorithms. Techniques like Layer-wise Relevance Propagation (LRP) (Bach et al., 2015) analyze contributions of individual neurons to final decisions, and Grad-CAM (Gradient-weighted Class Activation Mapping) (Selvaraju et al., 2017), visualizes areas of input that are important for predictions in CNN. These methods enhance the trust and effectiveness of AI systems by making their operations transparent and justifiable.
Additionally, a critical next step in advancing ML-based FTD diagnosis is real-world validation through prospective clinical trials. While many studies demonstrate high diagnostic accuracy using retrospective datasets, the true clinical applicability of these models remains uncertain without validation in real-world settings. Future studies should focus on integrating ML models into clinical workflows and testing their performance in prospective patient cohorts to ensure their robustness and clinical applicability. Moreover, prospective validation will enable clinicians to evaluate the practical challenges of implementing ML models in routine practice. Developing standardized protocols for real-world evaluation will be essential in bridging the gap between ML advancements and their clinical use for FTD diagnosis.
In summary, exploiting various data modalities and advanced algorithms, ML models can enhance diagnostic accuracy, leading to timely interventions and better patient outcomes. Addressing current limitations through standardization, larger datasets, and XAI will be essential for translating these advances into clinical practice.
This systematic review highlights significant advancements in applying ML techniques to differentiate FTD from other neurodegenerative conditions. To our knowledge, this is the first review to systematically evaluate machine learning algorithms specifically tailored to distinguish between FTD subtypes and to differentiate FTD from other neurodegenerative conditions, addressing a gap in the literature. The use of SVMs and deep learning algorithms, particularly CNNs, consistently achieved high diagnostic accuracy, showing particular promise in leveraging neuroimaging data for distinguishing FTD from AD and HCs. The integration of multimodal data, such as structural and functional neuroimaging, and neuropsychological assessments, has improved diagnostic performance by capturing complementary features across different domains of brain function. Despite these advancements, challenges such as small sample sizes, class imbalance, and lack of standardization limit the generalizability of current models. Future studies should prioritize creating and analyzing large, diverse, multi-center datasets to reduce bias and enhance generalizability. Standardized data collection protocols should focus on harmonizing imaging sequences, EEG preprocessing pipelines, and neuropsychological test administration to ensure consistency. Incorporating longitudinal data could provide insights into disease progression, enabling the development of models that predict both diagnosis and prognosis.
The development of XAI techniques could introduce transparency in ML models, increasing their interpretability and trustworthiness for clinical decision-making. To facilitate the transition from research to clinical practice, interdisciplinary collaboration between AI researchers, neurologists, and imaging experts is essential. Establishing standardized protocols and ensuring regulatory compliance will be crucial to successfully integrating ML-based diagnostics into routine healthcare. Additionally, ML-based medical tools must undergo rigorous regulatory approval processes, such as those required by the U.S. Food and Drug Administration (FDA) and European Conformity (CE) marking, to ensure safety, efficacy, and reliability in real-world clinical settings. Ethical considerations, including patient privacy, data security, and bias mitigation, should also be addressed to promote responsible AI application in neurology. Overall, ML approaches have shown promise in improving early and accurate diagnosis of FTD, potentially leading to timely interventions and better patient outcomes. Nevertheless, their success hinges on overcoming current challenges. Collaborative, interdisciplinary efforts combining methodological innovation with robust clinical validation will be essential for translating these advancements into the real-world applications.
The original contributions presented in the study are included in the article, further inquiries can be directed to the corresponding author.
SD: Conceptualization, Investigation, Methodology, Writing – original draft. AI: Data curation, Investigation, Methodology, Writing – original draft. GV: Investigation, Methodology, Writing – review & editing. AC: Methodology, Writing – review & editing. AQ: Funding acquisition, Writing – review & editing. LB: Investigation, Methodology, Supervision, Writing – review & editing.
The author(s) declare that financial support was received for the research and/or publication of this article. This research was funded by the Italian Ministry of Health through Current Research Funds 2024.
The authors declare that the research was conducted in the absence of any commercial or financial relationships that could be construed as a potential conflict of interest.
The author(s) declare that no Gen AI was used in the creation of this manuscript.
All claims expressed in this article are solely those of the authors and do not necessarily represent those of their affiliated organizations, or those of the publisher, the editors and the reviewers. Any product that may be evaluated in this article, or claim that may be made by its manufacturer, is not guaranteed or endorsed by the publisher.
Ajra, Z., Xu, B., Dray, G., Montmain, J., and Perrey, S. (2023). Using shallow neural networks with functional connectivity from EEG signals for early diagnosis of Alzheimer's and frontotemporal dementia. Front. Neurol. 14:1270405. doi: 10.3389/fneur.2023.1270405
Al Olaimat, M., Martinez, J., Saeed, F., Bozdag, S., and Initiative, A. D. N. (2023). PPAD: a deep learning architecture to predict progression of Alzheimer's disease. Bioinformatics 39, i149–i157. doi: 10.1093/bioinformatics/btad249
Antonioni, A., Raho, E. M., Lopriore, P., Pace, A. P., Latino, R. R., Assogna, M., et al. (2023). Frontotemporal dementia, where do we stand? A narrative review. Int. J. Mol. Sci. 24:11732. doi: 10.3390/ijms241411732
Bach, S., Binder, A., Montavon, G., Klauschen, F., Müller, K.-R., and Samek, W. (2015). On pixel-wise explanations for non-linear classifier decisions by layer-wise relevance propagation. PLoS ONE 10:e0130140. doi: 10.1371/journal.pone.0130140
Bachli, M. B., Sede no, L., Ochab, J. K., Piguet, O., Kumfor, F., Reyes, P., et al. (2020). Evaluating the reliability of neurocognitive biomarkers of neurodegenerative diseases across countries: a machine learning approach. Neuroimage 208:116456. doi: 10.1016/j.neuroimage.2019.116456
Basaia, S., Agosta, F., Wagner, L., Canu, E., Magnani, G., Santangelo, R., et al. (2019). Automated classification of Alzheimer's disease and mild cognitive impairment using a single MRI and deep neural networks. NeuroImage: Clinical 21:101645. doi: 10.1016/j.nicl.2018.101645
Birba, A., Santamaría-García, H., Prado, P., Cruzat, J., Ballesteros, A. S., Legaz, A., et al. (2022). Allostatic-interoceptive overload in frontotemporal dementia. Biol. Psychiatry 92, 54–67. doi: 10.1016/j.biopsych.2022.02.955
Bouts, M. J., Möller, C., Hafkemeijer, A., van Swieten, J. C., Dopper, E., van der Flier, W. M., et al. (2018). Single subject classification of Alzheimer's disease and behavioral variant frontotemporal dementia using anatomical, diffusion tensor, and resting-state functional magnetic resonance imaging. J. Alzheimer's Dis. 62, 1827–1839. doi: 10.3233/JAD-170893
Bron, E. E., Smits, M., Van Der Flier, W. M., Vrenken, H., Barkhof, F., Scheltens, P., et al. (2015). Standardized evaluation of algorithms for computer-aided diagnosis of dementia based on structural MRI: the caddementia challenge. Neuroimage 111, 562–579. doi: 10.1016/j.neuroimage.2015.01.048
Brusini, L., Cruciani, F., Dall'Aglio, G., Zajac, T., Galazzo, I. B., Zucchelli, M., et al. (2024). XAI-based assessment of the AMURA model for detecting amyloid-β and tau microstructural signatures in Alzheimer's disease. IEEE J. Transl. Eng. Health Med. 12, 569–579. doi: 10.1109/JTEHM.2024.3430035
Chaddad, A., Peng, J., Xu, J., and Bouridane, A. (2023). Survey of explainable AI techniques in healthcare. Sensors 23:634. doi: 10.3390/s23020634
Díaz-Álvarez, J., Matias-Guiu, J. A., Cabrera-Martín, M. N., Pytel, V., Segovia-Ríos, I., García-Gutiérrez, F., et al. (2022). Genetic algorithms for optimized diagnosis of Alzheimer's disease and frontotemporal dementia using fluorodeoxyglucose positron emission tomography imaging. Front. Aging Neurosci. 13:708932. doi: 10.3389/fnagi.2021.708932
Ducharme, S. (2023). Brain MRI research in neurodegenerative dementia: time to deliver on promises. Brain 146, 4403–4404. doi: 10.1093/brain/awad320
Feigin, V. L., Nichols, E., Alam, T., Bannick, M. S., Beghi, E., Blake, N., et al. (2019). Global, regional, and national burden of neurological disorders, 1990-2016: a systematic analysis for the global burden of disease study 2016. Lancet Neurol. 18, 459–480. doi: 10.1016/S1474-4422(18)30499-X
Garcia-Gutierrez, F., Delgado-Alvarez, A., Delgado-Alonso, C., Díaz-Álvarez, J., Pytel, V., Valles-Salgado, M., et al. (2022). Diagnosis of Alzheimer's disease and behavioural variant frontotemporal dementia with machine learning-aided neuropsychological assessment using feature engineering and genetic algorithms. Int. J. Geriatr. Psychiatry 37:2. doi: 10.1002/gps.5667
García-Gutierrez, F., Díaz-Álvarez, J., Matias-Guiu, J. A., Pytel, V., Matías-Guiu, J., Cabrera-Martín, M. N., et al. (2022). GA-MADRID: design and validation of a machine learning tool for the diagnosis of Alzheimer's disease and frontotemporal dementia using genetic algorithms. Med. Biol. Eng. Comput. 60, 2737–2756. doi: 10.1007/s11517-022-02630-z
Garn, H., Coronel, C., Waser, M., Caravias, G., and Ransmayr, G. (2017). Differential diagnosis between patients with probable Alzheimer's disease, Parkinson's disease dementia, or dementia with lewy bodies and frontotemporal dementia, behavioral variant, using quantitative electroencephalographic features. J. Neural Transm. 124, 569–581. doi: 10.1007/s00702-017-1699-6
Giunta, M., Solje, E., Gardoni, F., Borroni, B., and Benussi, A. (2021). Experimental disease-modifying agents for frontotemporal lobar degeneration. J. Exp. Pharmacol. 13, 359–376. doi: 10.2147/JEP.S262352
Gold, B. T., Johnson, N. F., Powell, D. K., and Smith, C. D. (2012). White matter integrity and vulnerability to Alzheimer's disease: preliminary findings and future directions. Biochim. Biophys. Acta. 1822, 416–422. doi: 10.1016/j.bbadis.2011.07.009
Gorelick, P. B., Counts, S. E., and Nyenhuis, D. (2016). Vascular cognitive impairment and dementia. Biochimica et Biophysica Acta 1862, 860–868. doi: 10.1016/j.bbadis.2015.12.015
Gorno-Tempini, M. L., Dronkers, N. F., Rankin, K. P., Ogar, J. M., Phengrasamy, L., Rosen, H. J., et al. (2004). Cognition and anatomy in three variants of primary progressive aphasia. Ann. Neurol. 55, 335–346. doi: 10.1002/ana.10825
Gorno-Tempini, M. L., Hillis, A. E., Weintraub, S., Kertesz, A., Mendez, M., Cappa, S. F., et al. (2011). Classification of primary progressive aphasia and its variants. Neurology 76, 1006–1014. doi: 10.1212/WNL.0b013e31821103e6
Guan, H., Wang, C., Cheng, J., Jing, J., and Liu, T. (2022). A parallel attention-augmented bilinear network for early magnetic resonance imaging-based diagnosis of Alzheimer's disease. Hum. Brain Mapp. 43, 760–772. doi: 10.1002/hbm.25685
Guidotti, R., Monreale, A., Ruggieri, S., Turini, F., Giannotti, F., and Pedreschi, D. (2018). A survey of methods for explaining black box models. ACM comp. Surv. 51, 1–42. doi: 10.1145/3236009
Gunning, D., Stefik, M., Choi, J., Miller, T., Stumpf, S., and Yang, G.-Z. (2019). XAI–explainable artificial intelligence. Sci. Robot. 4:eaay7120. doi: 10.1126/scirobotics.aay7120
Habes, M., Grothe, M. J., Tunc, B., McMillan, C., Wolk, D. A., and Davatzikos, C. (2020). Disentangling heterogeneity in Alzheimer's disease and related dementias using data-driven methods. Biol. Psychiatry 88, 70–82. doi: 10.1016/j.biopsych.2020.01.016
Han, K., He, M., Yang, F., and Zhang, Y. (2022). Multi-task multi-level feature adversarial network for joint Alzheimer's disease diagnosis and atrophy localization using sMRI. Phys. Med. Biol. 67:085002. doi: 10.1088/1361-6560/ac5ed5
Hodges, J. R., and Patterson, K. (2007). Semantic dementia: a unique clinicopathological syndrome. Lancet Neurol. 6, 1004–1014. doi: 10.1016/S1474-4422(07)70266-1
Hu, J., Qing, Z., Liu, R., Zhang, X., Lv, P., Wang, M., et al. (2021). Deep learning-based classification and voxel-based visualization of frontotemporal dementia and Alzheimer's disease. Front. Neurosci. 14:626154. doi: 10.3389/fnins.2020.626154
Islam, J., and Zhang, Y. (2020). Gan-based synthetic brain pet image generation. Brain Inform. 7:3. doi: 10.1186/s40708-020-00104-2
Jahan, S., Abu Taher, K., Kaiser, M. S., Mahmud, M., Rahman, M. S., Hosen, A. S., et al. (2023). Explainable AI-based Alzheimer's prediction and management using multimodal data. PLoS ONE 18:e0294253. doi: 10.1371/journal.pone.0294253
Josephy-Hernandez, S., Rezaii, N., Jones, A., Loyer, E., Hochberg, D., Quimby, M., et al. (2023). Automated analysis of written language in the three variants of primary progressive aphasia. Brain Commun. 5:fcad202. doi: 10.1093/braincomms/fcad202
Kertesz, A., and Munoz, D. (2004). Relationship between frontotemporal dementia and corticobasal degeneration/progressive supranuclear palsy. Dement. Geriatr. Cogn. Disord. 17, 282–286. doi: 10.1159/000077155
Kim, J. P., Kim, J., Park, Y. H., Park, S. B., San Lee, J., Yoo, S., et al. (2019). Machine learning based hierarchical classification of frontotemporal dementia and Alzheimer's disease. NeuroImage: Clini. 23:101811. doi: 10.1016/j.nicl.2019.101811
Kishore, N., and Goel, N. (2024). A review of machine learning techniques for diagnosing Alzheimer's disease using imaging modalities. Neural Comp. Appl. 36, 21957–21984. doi: 10.1007/s00521-024-10399-5
Korolev, I. O., Symonds, L. L., Bozoki, A. C., and Initiative, A. D. N. (2016). Predicting progression from mild cognitive impairment to Alzheimer's dementia using clinical, MRI, and plasma biomarkers via probabilistic pattern classification. PLoS ONE 11:e0138866. doi: 10.1371/journal.pone.0138866
Lage, C., López-García, S., Bejanin, A., Kazimierczak, M., Aracil-Bola nos, I., Calvo-Córdoba, A., et al. (2021). Distinctive oculomotor behaviors in Alzheimer's disease and frontotemporal dementia. Front. Aging Neurosci. 12:603790. doi: 10.3389/fnagi.2020.603790
Lal, U., Chikkankod, A. V., and Longo, L. (2024). A comparative study on feature extraction techniques for the discrimination of frontotemporal dementia and Alzheimer's disease with electroencephalography in resting-state adults. Brain Sci. 14:335. doi: 10.3390/brainsci14040335
Lundberg, S. M., and Lee, S.-I. (2017). A unified approach to interpreting model predictions. arXiv [preprint] arXiv:1705.07874. doi: 10.48550/arXiv.1705.07874
Ma, D., Lu, D., Popuri, K., Wang, L., Beg, M. F., and Initiative, A. D. N. (2020). Differential diagnosis of frontotemporal dementia, Alzheimer's disease, and normal aging using a multi-scale multi-type feature generative adversarial deep neural network on structural magnetic resonance images. Front. Neurosci. 14:853. doi: 10.3389/fnins.2020.00853
Ma, D., Stocks, J., Rosen, H., Kantarci, K., Lockhart, S. N., Bateman, J. R., et al. (2024). Differential diagnosis of frontotemporal dementia subtypes with explainable deep learning on structural MRI. Front. Neurosci. 18:1331677. doi: 10.3389/fnins.2024.1331677
Maito, M. A., Santamaría-García, H., Moguilner, S., Possin, K. L., Godoy, M. E., Avila-Funes, J. A., et al. (2023). Classification of Alzheimer's disease and frontotemporal dementia using routine clinical and cognitive measures across multicentric underrepresented samples: a cross sectional observational study. Lancet Regional Health-Am. 17:100387. doi: 10.1016/j.lana.2022.100387
Miltiadous, A., Tzimourta, K. D., Giannakeas, N., Tsipouras, M. G., Afrantou, T., Ioannidis, P., et al. (2021). Alzheimer's disease and frontotemporal dementia: a robust classification method of EEG signals and a comparison of validation methods. Diagnostics 11:1437. doi: 10.3390/diagnostics11081437
Möller, C., Pijnenburg, Y. A., van der Flier, W. M., Versteeg, A., Tijms, B., de Munck, J. C., et al. (2016). Alzheimer disease and behavioral variant frontotemporal dementia: automatic classification based on cortical atrophy for single-subject diagnosis. Radiology 279, 838–848. doi: 10.1148/radiol.2015150220
Moorthy, D. K., Nagaraj, P., and Subhashini, S. (2023). “A review on Alzheimer's disease detection using machine learning,” in 2023 Second International Conference on Augmented Intelligence and Sustainable Systems (ICAISS) (Trichy: IEEE), 573–581.
Neary, D., Snowden, J. S., Gustafson, L., Passant, U., Stuss, D., Black, S., et al. (1998). Frontotemporal lobar degeneration: a consensus on clinical diagnostic criteria. Neurology 51, 1546–1554. doi: 10.1212/WNL.51.6.1546
Nguyen, H.-D., Clément, M., Planche, V., Mansencal, B., and Coupé, P. (2023). Deep grading for MRI-based differential diagnosis of Alzheimer's disease and frontotemporal dementia. Artif. Intell. Med. 144:102636. doi: 10.1016/j.artmed.2023.102636
Nuytemans, K., Franzen, S., Broce, I. J., Caramelli, P., Ellajosyula, R., Finger, E., et al. (2024). Gaps in biomedical research in frontotemporal dementia: a call for diversity and disparities focused research. Alzheimer's Dement. 20, 9014–9036. doi: 10.1002/alz.14312
Page, M. J., McKenzie, J. E., Bossuyt, P. M., Boutron, I., Hoffmann, T. C., Mulrow, C. D., et al. (2021). The prisma 2020 statement: an updated guideline for reporting systematic reviews. BMJ 372:n71. doi: 10.31222/osf.io/v7gm2
Pérez-Millan, A., Contador, J., Juncà-Parella, J., Bosch, B., Borrell, L., Tort-Merino, A., et al. (2023). Classifying Alzheimer's disease and frontotemporal dementia using machine learning with cross-sectional and longitudinal magnetic resonance imaging data. Hum. Brain Mapp. 44, 2234–2244. doi: 10.1002/hbm.26205
Pérez-Millan, A., Thirion, B., Falgàs, N., Borrego-Écija, S., Bosch, B., Juncà-Parella, J., et al. (2024). Beyond group classification: probabilistic differential diagnosis of frontotemporal dementia and Alzheimer's disease with MRI and CSF biomarkers. Neurobiol. Aging 144, 1–11. doi: 10.1016/j.neurobiolaging.2024.08.008
Raamana, P. R., Rosen, H., Miller, B., Weiner, M. W., Wang, L., and Beg, M. F. (2014). Three-class differential diagnosis among alzheimer disease, frontotemporal dementia, and controls. Front. Neurol. 5:71. doi: 10.3389/fneur.2014.00071
Rahman, M. M., and Davis, D. N. (2013). Addressing the class imbalance problem in medical datasets. Int. J. Mach. Learn. Comp. 3:224. doi: 10.7763/IJMLC.2013.V3.307
Rajkomar, A., Dean, J., and Kohane, I. (2019). Machine learning in medicine. N. Engl. J. Med. 380, 1347–1358. doi: 10.1056/NEJMra1814259
Rascovsky, K., Hodges, J. R., Knopman, D., Mendez, M. F., Kramer, J. H., Neuhaus, J., et al. (2011). Sensitivity of revised diagnostic criteria for the behavioural variant of frontotemporal dementia. Brain 134, 2456–2477. doi: 10.1093/brain/awr179
Rathore, S., Habes, M., Iftikhar, M. A., Shacklett, A., and Davatzikos, C. (2017). A review on neuroimaging-based classification studies and associated feature extraction methods for Alzheimer's disease and its prodromal stages. Neuroimage 155, 530–548. doi: 10.1016/j.neuroimage.2017.03.057
Ratnavalli, E., Brayne, C., Dawson, K., and Hodges, J. (2002). The prevalence of frontotemporal dementia. Neurology 58, 1615–1621. doi: 10.1212/WNL.58.11.1615
Rezaei, M., Zereshki, E., Shahsavari, S., Salehi, M. G., and Sharini, H. (2020). Prediction of Alzheimer's disease using machine learning classifiers. Disease Diagn. 9, 116–120. doi: 10.34172/iejm.2020.21
Ribeiro, M. T., Singh, S., and Guestrin, C. (2016). ““Why should i trust you?” Explaining the predictions of any classifier,” in Proceedings of the 22nd ACM SIGKDD International Conference on Knowledge Discovery and Data Mining (New York, NY: Association for Computing Machinery), 1135–1144.
Rogeau, A., Hives, F., Bordier, C., Lahousse, H., Roca, V., Lebouvier, T., et al. (2024). A 3D convolutional neural network to classify subjects as Alzheimer's disease, frontotemporal dementia or healthy controls using brain 18F-FDG pet. Neuroimage 288:120530. doi: 10.1016/j.neuroimage.2024.120530
Rohrer, J. D. (2012). Structural brain imaging in frontotemporal dementia. Biochimica et Biophysica Acta 1822, 325–332. doi: 10.1016/j.bbadis.2011.07.014
Rostamikia, M., Sarbaz, Y., and Makouei, S. (2024). EEG-based classification of Alzheimer's disease and frontotemporal dementia: a comprehensive analysis of discriminative features. Cogn. Neurodyn. 18, 3447–3462. doi: 10.1007/s11571-024-10152-7
Sadeghi, M. A., Stevens, D., Kundu, S., Sanghera, R., Dagher, R., Yedavalli, V., et al. (2024). Detecting Alzheimer's disease stages and frontotemporal dementia in time courses of resting-state fMRI data using a machine learning approach. J. Imag. Inform. Med. 37, 2768–2783. doi: 10.1007/s10278-024-01101-1
Seelaar, H., Rohrer, J. D., Pijnenburg, Y. A., Fox, N. C., and Van Swieten, J. C. (2010). Clinical, genetic and pathological heterogeneity of frontotemporal dementia: a review. J. Neurol. Neurosurg. Psychiat. 82, 476–86. doi: 10.1136/jnnp.2010.212225
Selvaraju, R. R., Cogswell, M., Das, A., Vedantam, R., Parikh, D., and Batra, D. (2017). “Grad-CAM: visual explanations from deep networks via gradient-based localization,” in Proceedings of the IEEE International Conference on Computer Vision (Venice: IEEE), 618–626.
Shukla, A., Tiwari, R., and Tiwari, S. (2023). Review on Alzheimer disease detection methods: Automatic pipelines and machine learning techniques. Science 5:13. doi: 10.3390/sci5010013
Spasov, S., Passamonti, L., Duggento, A., Lio, P., Toschi, N., Initiative, A. D. N., et al. (2019). A parameter-efficient deep learning approach to predict conversion from mild cognitive impairment to Alzheimer's disease. Neuroimage 189, 276–287. doi: 10.1016/j.neuroimage.2019.01.031
Tjoa, E., and Guan, C. (2020). A survey on explainable artificial intelligence (XAI): toward medical XAI. IEEE Trans. Neural Netw. Learn. Syst. 32, 4793–4813. doi: 10.1109/TNNLS.2020.3027314
Umeda-Kameyama, Y., Kameyama, M., Tanaka, T., Son, B.-K., Kojima, T., Fukasawa, M., et al. (2021). Screening of Alzheimer's disease by facial complexion using artificial intelligence. Aging 13:1765. doi: 10.18632/aging.202545
Vieira, S., Pinaya, W. H., and Mechelli, A. (2017). Using deep learning to investigate the neuroimaging correlates of psychiatric and neurological disorders: methods and applications. Neurosci. Biobehav. Rev. 74, 58–75. doi: 10.1016/j.neubiorev.2017.01.002
Walker, Z., Possin, K. L., Boeve, B. F., and Aarsland, D. (2015). Lewy body dementias. Lancet 386, 1683–1697. doi: 10.1016/S0140-6736(15)00462-6
Wang, J., Redmond, S. J., Bertoux, M., Hodges, J. R., and Hornberger, M. (2016). A comparison of magnetic resonance imaging and neuropsychological examination in the diagnostic distinction of Alzheimer's disease and behavioral variant frontotemporal dementia. Front. Aging Neurosci. 8:119. doi: 10.3389/fnagi.2016.00119
Wang, Z., Liu, A., Yu, J., Wang, P., Bi, Y., Xue, S., et al. (2024). The effect of aperiodic components in distinguishing Alzheimer's disease from frontotemporal dementia. Geroscience 46, 751–768. doi: 10.1007/s11357-023-01041-8
Williams, G. B., Nestor, P. J., and Hodges, J. R. (2005). Neural correlates of semantic and behavioural deficits in frontotemporal dementia. Neuroimage 24, 1042–1051. doi: 10.1016/j.neuroimage.2004.10.023
Yousefzadeh, N., Tran, C., Ramirez-Zamora, A., Chen, J., Fang, R., and Thai, M. T. (2024). Neuron-level explainable ai for Alzheimer's disease assessment from fundus images. Sci. Rep. 14:7710. doi: 10.1038/s41598-024-58121-8
Keywords: frontotemporal dementia, machine learning, differential diagnosis, Alzheimer's disease, neuroimaging, deep learning, Support Vector Machines, convolutional neural networks
Citation: Dattola S, Ielo A, Varone G, Cacciola A, Quartarone A and Bonanno L (2025) Frontotemporal dementia: a systematic review of artificial intelligence approaches in differential diagnosis. Front. Aging Neurosci. 17:1547727. doi: 10.3389/fnagi.2025.1547727
Received: 18 December 2024; Accepted: 31 March 2025;
Published: 10 April 2025.
Edited by:
Thomas Van Groen, University of Alabama at Birmingham, United StatesReviewed by:
Agnès Pérez-Millan, Universitat de Barcelona, SpainCopyright © 2025 Dattola, Ielo, Varone, Cacciola, Quartarone and Bonanno. This is an open-access article distributed under the terms of the Creative Commons Attribution License (CC BY). The use, distribution or reproduction in other forums is permitted, provided the original author(s) and the copyright owner(s) are credited and that the original publication in this journal is cited, in accordance with accepted academic practice. No use, distribution or reproduction is permitted which does not comply with these terms.
*Correspondence: Augusto Ielo, YXVndXN0by5pZWxvQGlyY2NzbWUuaXQ=
Disclaimer: All claims expressed in this article are solely those of the authors and do not necessarily represent those of their affiliated organizations, or those of the publisher, the editors and the reviewers. Any product that may be evaluated in this article or claim that may be made by its manufacturer is not guaranteed or endorsed by the publisher.
Research integrity at Frontiers
Learn more about the work of our research integrity team to safeguard the quality of each article we publish.