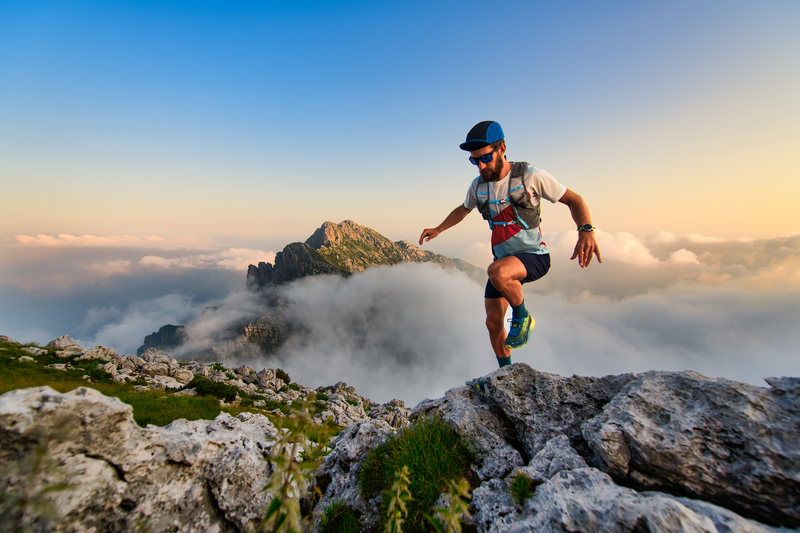
94% of researchers rate our articles as excellent or good
Learn more about the work of our research integrity team to safeguard the quality of each article we publish.
Find out more
ORIGINAL RESEARCH article
Front. Aging Neurosci.
Sec. Alzheimer's Disease and Related Dementias
Volume 17 - 2025 | doi: 10.3389/fnagi.2025.1542514
This article is part of the Research Topic Frontier Research on Artificial Intelligence and Radiomics in Neurodegenerative Diseases View all 7 articles
The final, formatted version of the article will be published soon.
You have multiple emails registered with Frontiers:
Please enter your email address:
If you already have an account, please login
You don't have a Frontiers account ? You can register here
Background: Research in aging, impairment, and Alzheimer's disease (AD) often requires powerful computational models for discriminating between clinical cohorts and identifying early biomarkers and key risk or protective factors. Machine Learning (ML) approaches represent a diverse set of datadriven tools for performing such tasks in big or complex datasets. We present systematic demonstration analyses to compare seven frequently used ML classifier models and two eXplainable Artificial Intelligence (XAI) techniques on multiple performance metrics for a common neurodegenerative disease dataset. The aim is to identify and characterize the best performing ML and XAI algorithms for the present data.We accessed a Canadian Consortium on Neurodegeneration in Aging dataset featuring four well-characterized cohorts: Cognitively Unimpaired (CU), Subjective Cognitive Impairment (SCI), Mild Cognitive Impairment (MCI), and AD (N=255). All participants contributed 102 multi-modal biomarkers and risk factors. Seven ML algorithms were compared along six performance metrics in discriminating between cohorts. Two XAI algorithms were compared using five performance and five similarity metrics.Results: Although all ML models performed relatively well in the extreme-cohort comparison (CU/AD), the Super Learner (SL), Random Forest (RF) and Gradient-Boosted trees (GB) algorithms excelled in the challenging near-cohort comparisons (CU/SCI). For the XAI interpretation comparison, SHapley Additive exPlanations (SHAP) generally outperformed Local Interpretable Model agnostic Explanation (LIME) in key performance properties.The ML results indicate that two tree-based methods (RF and GB) are reliable and effective as initial models for classification tasks involving discrete clinical aging and neurodegeneration data. In the XAI phase, SHAP performed better than LIME due to lower computational time (when applied to RF and GB) and incorporation of feature interactions, leading to more reliable results.
Keywords: Alzheimer's Disease (AD)1, artificial intelligence (AI)2, Canadian Consortium on Neurodegeneration in Aging (CCNA)3, Machine Learning (ML)4, Mild Cognitive Impairment (MCI)5, eXplainable Artificial Intelligence (XAI)6
Received: 10 Dec 2024; Accepted: 11 Feb 2025.
Copyright: © 2025 Fah, Bohn, Greiner and Dixon. This is an open-access article distributed under the terms of the Creative Commons Attribution License (CC BY). The use, distribution or reproduction in other forums is permitted, provided the original author(s) or licensor are credited and that the original publication in this journal is cited, in accordance with accepted academic practice. No use, distribution or reproduction is permitted which does not comply with these terms.
* Correspondence:
Harrison Fah, Neuroscience and Mental Health Institute, University of Alberta, Edmonton, T6G 2E1, Alberta, Canada
Disclaimer: All claims expressed in this article are solely those of the authors and do not necessarily represent those of their affiliated organizations, or those of the publisher, the editors and the reviewers. Any product that may be evaluated in this article or claim that may be made by its manufacturer is not guaranteed or endorsed by the publisher.
Research integrity at Frontiers
Learn more about the work of our research integrity team to safeguard the quality of each article we publish.