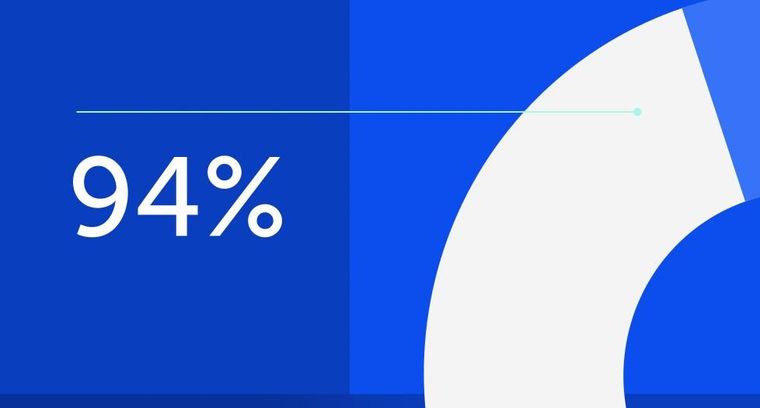
94% of researchers rate our articles as excellent or good
Learn more about the work of our research integrity team to safeguard the quality of each article we publish.
Find out more
ORIGINAL RESEARCH article
Front. Aging Neurosci., 07 February 2025
Sec. Parkinson’s Disease and Aging-related Movement Disorders
Volume 17 - 2025 | https://doi.org/10.3389/fnagi.2025.1529197
This article is part of the Research TopicAdvancing personalized diagnosis and treatment in Parkinson's Disease: Integrating biomarkers, neuroimaging, and artificial intelligenceView all 8 articles
Background: This study aimed to explore the association between a new inflammatory marker, systemic immune-inflammation index (SII), and the risk of Parkinson’s disease (PD) in adult population.
Methods: A cross-sectional design was used, participants were recruited from the National Health and Nutrition Examination Survey (NHANES) from 2005 to 2020. Three logistic regression models were used to explore the association between SII and the risk of PD, and subgroup analysis and sensitivity analysis were used. In addition, the restricted cubic spline (RCS) was used to explore the dose-response relationship between SII and PD. Receiver operating characteristic (ROC) curves was used to explore the diagnostic value of SII for PD.
Results: A total of 54,027 adults (mean age 35 years) were included in this study. The results of logistic regression showed that after adjusted for all covariates, compared with the Q1 group (lowest quartile in SII), the risk of PD in the Q3 group (OR = 1.82, 95%CI = 1.20–2.82, p < 0.001) and the Q4 group increased (OR = 2.49, 95%CI = 1.69–3.77, p < 0.001), with p-trend < 0.001. After excluding individuals with any missing values, sensitivity analysis also found a positive association between SII and PD. Subgroup analysis showed that this association was more significant in women, younger than 60 years old, non-smokers, alcohol drinkers, non-obese, and without a history of stroke, diabetes, or coronary heart disease. In addition, there was a positive dose-response relationship between SII and PD, and SII had an acceptable diagnostic value for PD (AUC = 0.72).
Conclusion: SII is positively correlated with the prevalence of PD in the adult population, and SII can help differentiate between PD and non-PD cases.
Parkinson’s disease (PD) is a rapidly growing neurodegenerative disease (Dorsey et al., 2018). According to the latest international diagnostic criteria, PD is diagnosed by the presence of bradykinesia together with rigidity or tremor, along with supporting features (Bloem et al., 2021; Kobylecki, 2020). PD is considered the second most common neurodegenerative disease after Alzheimer’s disease (Ascherio and Schwarzschild, 2016). In developed countries, the median age-standardized annual incidence is 14 per 100,000 people in the total population and 160 per 100,000 people in people aged 65 years or older (Ascherio and Schwarzschild, 2016). It is generally believed that the age of onset of PD is mainly 60 years old (de Lau and Breteler, 2006), but several studies found that early-onset PD can appear before the age of 40, indicating that PD can occur at different ages (Gershanik, 2003; Riboldi et al., 2022; Schrag and Schott, 2006).
Among the exogenous factors known to influence the risk of Parkinson’s disease, such as exposure to pesticides (Brouwer et al., 2017), consumption of dairy products (Hughes et al., 2017), a history of melanoma (Gao et al., 2009), and traumatic brain injury (Lee et al., 2012), while a decreased risk is associated with smoking (Rose et al., 2024), caffeine consumption (Ross et al., 2000), elevated serum urate concentrations (Shen et al., 2013), physical activity (Fang et al., 2018), and use of ibuprofen (Gao et al., 2011) and other common medications (Ascherio and Schwarzschild, 2016), although these studies didn’t consider genetic factors (Trinh and Farrer, 2013), which is also associated with risk of PD. However, the pathogenesis of PD remains unclear. A large number of experimental and postmortem studies (Gate, 2022) have shown that inflammation plays an important role in the pathogenesis of PD. A study by Chen et al. (Chen et al., 2007) found that men with high plasma interleukin-6 concentrations had an increased risk of PD. Some case-control studies have found that IL-6, TNF-a, IL-1β, ST NFR 1, CRP, CCL 2, CX 3 CL 1, and CX CL 12 are elevated in the PD group (Qin et al., 2016; Qiu et al., 2019; Qu et al., 2023).
Systemic immune-inflammation index (SII) is a new type of systemic inflammation evaluation index that can objectively reflect the balance between host inflammatory and immune response status (Hu et al., 2014; Xu et al., 2024). An elevated SII usually suggests an elevated inflammatory status and weak immune response in patients (Hu et al., 2014). The calculation formula is the product of the platelet to neutrophil to lymphocyte ratio (Hu et al., 2014). SII has been found to be closely associated with the occurrence and prognosis of diseases in the elderly. A study by Tian et al. (2022) suggested that SII was associated with the prognosis of elderly patients with digestive system tumors. In terms of neurological diseases, Xu et al. (2024) conducted a study on 102 traumatic brain injuries (TBI) and found that the SII index increased in the early stages of TBI and was an independent risk factor for predicting poor prognosis in patients. Bao et al. (2023) found that SII was associated with cognitive impairment after acute ischemic cerebral infarction. Algul and Kaplan (2024) found that SII was associated with the severity of dementia in patients with Alzheimer’s disease. It is worth noting that SII was found to be negatively correlated with the motor performance of PD patients (Li et al., 2021). However, no study has yet explored the relationship between SII and the risk of PD in a large population sample.
To fill the gap of current studies, our study aims to explore the association between SII and the risk of PD in adults using data from a nationally representative cross-sectional survey, and further explore the diagnostic value of SII for the risk of PD. We hypothesize that individuals with higher SII are more likely to have a risk of PD and that SII can distinguish PD from non-PD in the population.
NHANES was constructed by the Centers for Disease Control and Prevention of the United States. The survey year is from 1999 to the present. The survey cycle is every 2 years. The sample size of each year is about 5000. The samples come from all over the country. NHANES includes demographic data, questionnaire data, dietary data, examination data and laboratory data. This study was approved by the National Cancer Institute and reviewed by the Health Statistics Research Ethics Review Committee. All participants signed informed consent. The research design and related data of NHANES can be downloaded at https://www.cdc.gov/nchs/nhanes/. The inclusion criteria of this study are as follows: (1) adults over 20 years old; (2) with prescription drug use data in the past 30 days; (3) with complete laboratory test data: platelet, neutrophil and lymphocyte data. After excluding all samples that did not meet the inclusion criteria, a total of 54,027 people were included in this study. The specific data flow chart is shown in Figure 1.
Figure 1. Flowchart of the participant selection from NHANES 2005–2020. NHANES, National Health and Nutrition Examination Survey; SII, systemic immune-inflammation index.
PD was defined based on the information provided by the subjects on prescription drug use in the past 30 days. Subjects were defined as having PD if they reported taking medication used to treat PD, including carbidopa, levodopa, methyldopa, benztropine, ropinirole, entacapone, and amantadine (Liu et al., 2023). With this definition, NHANES 2005-2020 reported 211 (1.06%) cases of PD in participants aged 45 and older, and 131 (1.27%) in participants aged 60 or older, whereas previous studies reported figures ranging from 0.47 to 0.77% of people with PD aged 45 and older in North America in 2012 (Willis et al., 2022), and 1% of people aged 65 or older with PD in industrialized countries in 2006 (de Lau and Breteler, 2006).
Based on previous study (Hu et al., 2014), we calculated SII using the following formula:
Platelet, neutrophil, and lymphocyte counts were derived from the CBC laboratory data of NHANES. The method used to derive CBC parameters was based on the Beckman Coulter counting and quantification method, combined with an automatic dilution and mixing device for sample processing, and a single-beam photometer for hemoglobin determination. WBC differentials used VCS technology (Volume, Conductivity, Scatter). For detailed detection procedures, please refer to the NHANES Laboratory/Medical Technician Procedure Manual (LPM) (CDC, 2020).
We included the following covariates: age (<60 years, ≥60 years), gender (male, female), race (Mexican American, other Hispanic, non-Hispanic white, non-Hispanic black, other race-including multi-racial), education (less than high school, high school or equivalent, college or above), marital status (married, widowed, divorced, separated, never married, living with partner), alcohol use (never, past drinker, current drinker), smoking (yes, no), BMI (<18.5, 18.5–24.9, 25.0–29.9, ≥ 30.0), sleep disorders (yes, no), hypertension (yes, no), diabetes (yes, no), coronary heart disease (yes, no), stroke (yes, no). Previous disease diagnosis information was based on self-reported information.
Since the NHANES database uses a complex, multi-stage sampling method for investigation, this study used 2-year MEC exam weights (WTMEC2YR)1 to perform weighted analysis of relevant indicators. First, we calculated the SII quartiles (first quartile = 296.47, median = 429.00, third quartile = 620.00) based on the SII levels of all participants and divided them into four groups according to these quartiles (see the distribution of SII levels in Supplementary Figure S1). Next, descriptive statistics were performed on the overall population and each group of participants. Continuous variables were described by mean and standard deviation, and categorical variables were described by frequency and percentage. The chi-square test was used to compare categorical variables between groups, and analysis of variance was used to compare continuous variables. Second, we used three logistic regression models to explore the relationship between SII levels and the risk of PD in the population. Model 1 (Crude model) did not adjust for covariates. Model 2 adjusted for age, gender, race, education level, marital status. Model 3 further adjusted for alcohol use, smoking-cigarette use, BMI, sleep disorders, hypertension, diabetes, coronary heart disease, and stroke based on Model 2. Next, we used subgroup analysis to explore the relationship between SII levels and the risk of PD in different populations. One model was fitted per covariate and adjusted for the other covariates, then we fit another adjusted model for each covariate with an interaction term between the covariate and the SII levels to study interaction effect. In addition, we excluded all individuals with missing values and performed sensitivity analysis to verify the robustness of our results. Finally, we used the restricted cubic spline (RCS) after adjusted for age, gender, race, education level, marital status, alcohol use, smoking-cigarette use, BMI, sleep disorders, hypertension, diabetes, coronary heart disease, and stroke to explore the dose-response relationship between SII levels and the risk of PD, we fit three models with three, four, five knots and the model with the lowest AIC was selected in our study (Frank and Harrell, 2015). Further, we used the Receiver operating characteristic (ROC) curves to detect the diagnostic value of SII on the risk of PD. All statistical analyses were performed using R (version 4.4.1). R package “rms” (version 6.9-0) was used to conduct RCS analysis. We used a two-sided test, p < 0.05 was considered statistically significant. For all models, the associations were reported with adjusted odds ratios (ORs), 95% confidence intervals (CIs), and p-values.
A total of 54,027 people were included in this study, of which 49.5% were male, 50.5% were female, 78.7% were under 60 years old, 21.3% were 60 years old and above, the average age was 35 years old (SD, 24.1; Range, 20–85), 37.6% were non-Hispanic white, and 23.4% were non-Hispanic black. The number of patients diagnosed with PD was 260 (0.5%). We found that there were significant differences in the prevalence of PD among different SII level groups (p < 0.001). The distribution of SII was shown in Supplementary Figure S1. In addition, we found statistically significant group differences in age, race, marital status, BMI, alcohol consumption, sleep disorders, hypertension, diabetes, coronary heart disease, and stroke (all p < 0.001) (Table 1).
When SII was used as a numerical variable, without adjusting for any covariates, the higher the level of SII, the higher the risk of PD (OR = 1.02, 95%CI = 1.05–1.21, p = 0.003). After adjusting for age, gender, race, education level, and marital status, SII was positively correlated with the risk of PD (OR = 1.07, 95%CI = 1.02–1.14, p = 0.002). After further adjusting for alcohol use, smoking-cigarette use, BMI, sleep disorders, hypertension, diabetes, coronary heart disease and stroke, the relationship between SII and PD still held (OR = 1.07, 95%CI = 1.02–1.14, p = 0.006). When SII was treated as a categorical variable, in the three logistic regression models, compared with the SII level of first quartile (Q1), in model 1, the risk of PD in the Q2 group was significantly increased (OR = 1.66, 95%CI = 1.08–2.60, p = 0.024), which is also significant in Q3 (OR = 2.16, 95%CI = 1.43–3.33, p < 0.001) and Q4 group (OR = 3.33, 95% CI = 2.27–5.03, p < 0.001); In model 2, the risk of PD increased in group Q3 (OR = 1.67, 95%CI = 1.10–2.58, p = 0.018) and Q4 (OR = 2.29, 95%CI = 1.55–3.47, p < 0.001). In model 3, the risk of PD in group Q3 (OR = 1.82, 95%CI = 1.20–2.82, p < 0.001) and group Q4 (OR = 2.49, 95%CI = 1.69–3.77, p < 0.001) was increased, and the three models showed significant trends of increasing risk of PD with increasing SII levels (all p-trend < 0.001) (Table 2). Detailed summaries of multivariate logistic regressions were shown in Supplementary Table S2.
The results of subgroup analysis showed that, compared with Q1, the association between high level SII (Q4) and increased risk of PD was more likely to be found in female (OR = 3.40, 95%CI = 1.91–6.50, p < 0.001), younger than 60 years old (OR = 3.67, 95%CI = 1.99–7.31, p < 0.001), non-Hispanic white (OR = 3.04, 95%CI = 1.71–5.90, p < 0.001), high school education or above (OR = 5.98, 95%CI = 2.89–14.5, p < 0.001), not currently smoking (OR = 3.94, 95%CI = 2.20–7.70, p < 0.001), alcohol drinker (OR = 3.20, 95%CI = 1.86–5.87, p < 0.001), non-obese (OR = 3.38, 95%CI = 1.97–6.12, p < 0.001), without a history of stroke (OR = 2.56, 95%CI = 1.70–3.96, p < 0.001), without a history of diabetes (OR = 3.31, 95% CI = 2.08–5.51, p < 0.001), and without a history of coronary heart disease (OR = 2.63 95%CI = 1.75–4.07, p < 0.001) (Table 3). We conducted a sensitivity analysis for missing values. After excluding subjects with missing values in any covariate, compared with the low-level SII group, the high-level SII group still had a higher risk of PD (Table 4).
Table 3. Subgroup analysis of associations between SII levels and the risks of Parkinson’s diseasesa.
Table 4. Associations between SII levels and the risks of Parkinson’s diseases among participants without missing valuesa.
Four knots were selected in our study, details of model fit were shown in Supplementary Table S3. The RCS curve results showed that after adjusting for age, gender, race, education level, marital status, alcohol drinking, smoking-cigarette use, BMI, sleep disorders, hypertension, diabetes, coronary heart disease and stroke, the higher the level of SII, the higher the risk of PD, a non-linear relationship was found (p overall < 0.001, p for non-linearity < 0.001), and SII > 426.13 indicated a steep increased risk of PD (Figure 2).
Figure 2. Dose-response relationship between systemic immune-inflammation index and Parkinson’s disease.
The ROC curve results showed that the level of SII can distinguish PD from non-PD in the population (AUC = 0.72, 95% CI: 0.69–0.75) (Figure 3). In addition, the accuracy of the model was 0.76, the sensitivity was 0.79, the specificity was 0.71, and Youden index was 0.50.
Figure 3. The diagnostic value of systemic immune-inflammation index for Parkinson’s disease risk. AUC, Area Under the Curve.
Our study is the first to examine the relationship between SII levels and risk of PD in a large, nationally representative cross-sectional adult population sample. We found that the higher the SII level, the higher the risk of adult PD, and this trend is very significant, especially among women, younger than 60 years old, non-Hispanic white, high school education or above, not currently smoking, alcohol drinker, non-obese, without a history of stroke, diabetes, or coronary heart disease. In addition, we found a positive dose-response relationship between SII levels and the risk of PD, and SII levels can distinguish PD from non-PD in adult population.
The relationship between SII and the risk of PD has not been studied, but a large number of previous studies have shown that peripheral blood inflammatory markers play an important role in the occurrence and development of PD. The results of a case-control study on early PD by Kim et al. (2018) showed that the levels of IL-1β, IL-2 and IL-6 in the PD group were significantly higher than those in the control group, and IL-10 was associated with patients’ non-motor symptoms. Studies have shown that chronic proinflammatory states already exist in the prodromal stage of PD, such as iRBD (Lindestam Arlehamn et al., 2020; Stokholm et al., 2017; Sulzer et al., 2017). A study by Chen et al. (2007) showed that men with high plasma IL-6 levels have an increased risk of PD, but the sample size was small and there was a lack of discussion of other inflammatory biomarkers. A meta-analysis showed that PD patients had higher levels of IL-6, tumor necrosis factor-α, IL-1β, IL-2, IL-10, C-reactive protein and RANTES in peripheral blood, which further strengthened the clinical evidence that PD patients have peripheral inflammatory responses (Qin et al., 2016). Moreover, a case-control study by Pedersen et al. (2023) found nine CSF inflammatory markers associated with PD (increased levels of CD5, CDCP1, IL-18R1, and IL-6 and decreased levels of ADA, CCL23, CD8A, FGF-19, and MCP-2).
On the basis of the above studies, our study further explored the relationship between SII, a new systemic inflammatory response index based on peripheral blood inflammatory cells, and the risk of PD. The calculation of SII is based on the counts of platelets, neutrophils and lymphocytes, that is, the product of platelets and the ratio of neutrophils and lymphocytes (Hu et al., 2014). These peripheral blood inflammatory markers are associated with the risk of PD. Platelets originate from megakaryocytes in the bone marrow and are defined as very small anucleated cell fragments with a diameter of 2-4 μm, which are involved in thrombosis (Beura et al., 2022). Platelet dysfunction is believed to be related to endothelial cell damage, and platelets can express neuron-specific molecules and receptors; it also expresses several PD-specific biomarkers, such as α-synuclein, parkin, PTEN-induced kinase 1, tyrosine hydroxylase, and dopamine transporter (Beura et al., 2022). Therefore, platelets are often used to build peripheral models of PD, and antiplatelet drugs are considered to have potential therapeutic value for PD.
Neutrophils are immune cells with unique biological characteristics and strong antimicrobial properties (Burn et al., 2021). These cells phagocytose and subsequently kill prokaryotes and eukaryotes very effectively. The neutrophil-to-lymphocyte ratio (NLR) is a complete blood count (CBC)-based biomarker that reflects the balance between immunity and systemic inflammation (Hosseini et al., 2023). Studies have found that NLR in the plasma of PD patients is significantly higher than that of HC (Li et al., 2024; Munoz-Delgado et al., 2021). The results also highlighted the correlation between plasma NLR and the total UPDRS score and UPDRS I-III score in PD patients (Li et al., 2024), indicating that NLR is associated with the severity of symptoms in PD patients. Neutrophils are able to penetrate the epithelial and vascular wall cell layers and promote the body’s inflammatory response by regulating chemokines (Ley et al., 2018). An animal experiment by Jensen et al. (2021) found that T cell infiltration was detected in the hippocampus, neocortex, striatal perivascular area, and parenchyma of PD mice, and lower lymphocyte counts were associated with a greater risk of PD. In addition, the study by Dommershuijsen et al. (2022) found that the higher the lymphocyte count, the lower the likelihood of developing PD. Some studies have shown that NLR is higher in PD patients (Munoz-Delgado et al., 2021).
In addition, this study also found that the relationship between SII and the risk of PD has certain heterogeneity in different subgroups of people, which may be related to the differences in the risk of PD in different groups. Gender is considered an important risk factor for PD, with men having a higher incidence of PD than women, and a large number of studies have shown that there are also differences in symptoms, development, and response to treatment between male and female PD patients (Collaborators, 2019; Hirsch et al., 2016). Although sex differences in the association of SII with PD have not been studied, one longitudinal study showed (Cheng et al., 2024) that the impact of SII on mortality in male and female hypertensive patients was significantly different, with women with higher SII levels having a higher risk of death. Similar to the findings of this study, SII is more significantly associated with poor prognosis in women. Wei et al. (2024) found that in an age-stratified analysis, increased SII was a better predictor of the risk of metabolic syndrome in young people than in older people. This shows that SII can better reflect the systemic inflammatory status of young and middle-aged people. Inflammatory factors in young people are precisely and strictly regulated and are at lower levels (Singh and Newman, 2011). Elderly people are often in a state of chronic inflammation (De Maeyer et al., 2020; Sarkar and Fisher, 2006). Therefore, fluctuations in SII levels in young people are more sensitive to disease. Similarly, previous studies have shown (Demir and Demir, 2023) that compared with non-smokers, smokers have higher SII levels and are in a chronic inflammatory state for a long time. Therefore, the link between changes in SII levels and PD risk is more obvious among non-smokers. In terms of alcohol use, people who drink a small or moderate amount of beer have a lower risk of PD, while people who drink a higher amount of wine have an increased risk of PD (Imhof et al., 2001). It was found that moderate drinking can reduce the body’s inflammation level (Zhang et al., 2014). Our results suggest that among people who drink alcohol, the link between SII and PD risk is more significant. Further research is needed to identify this mechanism.
Our study found that the relationship between SII and the occurrence of PD in non-obese people is more significant, which may be related to the fact that the disease onset of non-obese people is more sensitive to fluctuations in SII levels. Zhou et al. (2024) found that obese people have higher SII levels, suggesting that inflammation may play an important role in the occurrence and development of obesity, and obesity may also lead to a sustained inflammatory response in the body. Nie et al. (2023) found that elevated SII has a stable relationship with an increased risk of diabetes, indicating that patients with diabetes have higher levels of inflammation. A prospective cohort study involving 13,929 adults by Xu et al. (2021) found that high SII was associated with increased risk of total stroke and ischemic stroke. A large number of previous studies have found that SII has a certain predictive effect on the occurrence of coronary heart disease (Yang et al., 2020) and is associated with the severity of coronary heart disease (Candemir et al., 2021). Our results concluded that SII levels are only associated with the risk of PD in people without coronary heart disease, stroke, or diabetes. On the one hand, the link between SII and PD risk is more significant in people with lower inflammation levels. This may be related to the fact that changes in the body’s SII levels are more sensitive to indicating the occurrence of PD in a low-inflammatory state. The specific mechanism needs to be further studied. On the other hand, pharmacological interventions and primary prevention measures targeting these diseases may attenuate the association between SII and PD. Insulin resistance is closely related to chronic inflammation, and the use of antidiabetic drugs may improve insulin resistance and reduce chronic inflammation (Shoelson et al., 2006). Medications such as statins and aspirin help reduce inflammation in people with coronary artery disease (Cheng et al., 2022; Shoelson et al., 2006).
Another important finding is that our results suggested a clear dose-response relationship between SII and the increased risk of PD, and high levels of SII can differentiate the occurrence of PD in the population with acceptable performance. Although the diagnostic value of SII for the risk of PD in the population has not been explored, previous studies have explored the predictive value of SII for other neurological diseases. Cheng et al. (2023) found that SII and SIRI can better predict the occurrence of stroke in 5907 asthma patients, and SIRI has a better predictive value for stroke prevalence than SII. Algul et al. found that SII, as a new inflammatory marker, is related to the severity of dementia in AD patients (Algul and Kaplan, 2024). In the study of the relationship between other inflammatory markers and PD, the neutrophil-to-lymphocyte ratio (NLR), lymphocyte-to-monocyte ratio (LMR) and neutrophil-to-high-density lipoprotein ratio (NHR) are considered to be able to predict the occurrence of PD, among which NLR has a relatively better predictive value (Li et al., 2024). The findings of this study are an important supplement to the association of PD by inflammatory markers. Whether the diagnostic value of SII for PD is different from that of other inflammatory markers needs further exploration.
Although this study has some important findings related to PD, we have several limitations. First, we used a cross-sectional design and cannot directly infer causality. Thus, the association between SII and PD needs to be further explored in longitudinal studies. Second, the assessment of PD is based on self-reported prescription medication data, and some subjects may have recall bias. Consequently, patients with non-PD parkinsonism may have been inadvertently included. Finally, participants of our study are from American population, and the results cannot be applied to the world population. This finding needs to be verified in populations from different countries.
In summary, our study is the first to find an association between high levels of SII and the risk of PD in a nationally representative cross-sectional sample, and further explore the heterogeneity of this association in different populations. In addition, we also found a positive dose-response relationship between SII and PD, and SII level has a good diagnostic value for the risk of PD in the population. Since SII is a new, valuable, and easy-to-measure inflammatory marker, this study provides important insights into the exploration of risk factors for PD and its prevention.
The datasets presented in this study can be found in online repositories. The names of the repository/repositories and accession number(s) can be found below: The related data of NHANES can be downloaded at https://www.cdc.gov/nchs/nhanes/.
The studies involving humans were approved by the National Cancer Institute and the Health Statistics Research Ethics Review Committee. The studies were conducted in accordance with the local legislation and institutional requirements. The participants provided their written informed consent to participate in this study.
JZ: Conceptualization, Data curation, Formal analysis, Investigation, Methodology, Project administration, Supervision, Validation, Visualization, Writing – original draft, Writing – review & editing. ZW: Conceptualization, Methodology, Writing – original draft, Writing – review & editing. FC: Conceptualization, Methodology, Validation, Writing – review & editing. XY: Conceptualization, Formal analysis, Methodology, Supervision, Writing – review & editing. ZS: Conceptualization, Methodology, Supervision, Writing – original draft.
The author(s) declare that no financial support was received for the research, authorship, and/or publication of this article.
We appreciate all participants from the study of NHANES, who provided data for our work. Further, we thank every staff from NCHS for their invaluable efforts and contributions.
The authors declare that the research was conducted in the absence of any commercial or financial relationships that could be construed as a potential conflict of interest.
The authors declare that no Generative AI was used in the creation of this manuscript.
All claims expressed in this article are solely those of the authors and do not necessarily represent those of their affiliated organizations, or those of the publisher, the editors and the reviewers. Any product that may be evaluated in this article, or claim that may be made by its manufacturer, is not guaranteed or endorsed by the publisher.
The Supplementary Material for this article can be found online at: https://www.frontiersin.org/articles/10.3389/fnagi.2025.1529197/full#supplementary-material
Algul, F., and Kaplan, Y. (2024). Increased systemic immune-inflammation index as a novel indicator of Alzheimer’s disease severity. J. Geriatr. Psychiatry Neurol. doi: 10.1177/08919887241280880 Online ahead of print.
Ascherio, A., and Schwarzschild, M. (2016). The epidemiology of Parkinson’s disease: Risk factors and prevention. Lancet Neurol. 15, 1257–1272. doi: 10.1016/S1474-4422(16)30230-7
Bao, Y., Wang, L., Du, C., Ji, Y., Dai, Y., and Jiang, W. (2023). Association between systemic immune inflammation index and cognitive impairment after acute ischemic stroke. Brain Sci. 13:464. doi: 10.3390/brainsci13030464
Beura, S., Panigrahi, A., Yadav, P., and Singh, S. (2022). Role of platelet in parkinson’s disease: Insights into pathophysiology & theranostic solutions. Ageing Res. Rev. 80:101681. doi: 10.1016/j.arr.2022.101681
Bloem, B., Okun, M., and Klein, C. (2021). Parkinson’s disease. Lancet 397, 2284–2303. doi: 10.1016/S0140-6736(21)00218-X
Brouwer, M., Huss, A., van der Mark, M., Nijssen, P., Mulleners, W., Sas, A., et al. (2017). Environmental exposure to pesticides and the risk of Parkinson’s disease in the Netherlands. Environ. Int. 107, 100–110. doi: 10.1016/j.envint.2017.07.001
Burn, G., Foti, A., Marsman, G., Patel, D., and Zychlinsky, A. (2021). The neutrophil. Immunity 54, 1377–1391. doi: 10.1016/j.immuni.2021.06.006
Candemir, M., Kiziltunc, E., Nurkoc, S., and Sahinarslan, A. (2021). Relationship between systemic immune-inflammation index (Sii) and the severity of stable coronary artery disease. Angiology 72, 575–581. doi: 10.1177/0003319720987743
CDC, (2020). Statistics NCFH. Publication Using Serum, Plasma, and Urine Specimens. Available online at: https://www.cdc.gov/nchs/nhanes/biospecimens/publications_usingspu.htm (accessed November 1, 2024).
Chen, H., O’Reilly, E., Schwarzschild, M., and Ascherio, A. (2007). Peripheral inflammatory biomarkers and risk of Parkinson’s disease. Am. J. Epidemiol. 167, 90–95. doi: 10.1093/aje/kwm260
Cheng, T., Yu, D., Tang, Q., Qiu, X., Li, G., Zhou, L., et al. (2024). Gender differences in the relationship between the systemic immune-inflammation index and all-cause and cardiovascular mortality among adults with hypertension: Evidence from Nhanes 1999-2018. Front. Endocrinol (Lausanne). 15:1436999. doi: 10.3389/fendo.2024.1436999
Cheng, W., Bu, X., Xu, C., Wen, G., Kong, F., Pan, H., et al. (2023). Higher systemic immune-inflammation index and systemic inflammation response index levels are associated with stroke prevalence in the asthmatic population: A cross-sectional analysis of the Nhanes 1999-2018. Front. Immunol. 14:1191130. doi: 10.3389/fimmu.2023.1191130
Cheng, W., Wang, L., and Chen, S. (2022). Differences in lipid profiles and atherogenic indices between hypertensive and normotensive populations: A cross-sectional study of 11 Chinese cities. Front. Cardiovasc. Med. 9:887067. doi: 10.3389/fcvm.2022.887067
Collaborators, G. (2019). Global, regional, and national burden of neurological disorders, 1990-2016: A systematic analysis for the global burden of disease study 2016. Lancet Neurol. 18, 459–480. doi: 10.1016/S1474-4422(18)30499-X
de Lau, L., and Breteler, M. (2006). Epidemiology of Parkinson’s disease. Lancet Neurol. 5, 525–535. doi: 10.1016/S1474-4422(06)70471-9
De Maeyer, R., van de Merwe, R., Louie, R., Bracken, O., Devine, O., Goldstein, D., et al. (2020). Blocking elevated P38 Mapk restores efferocytosis and inflammatory resolution in the elderly. Nat. Immunol. 21, 615–625. doi: 10.1038/s41590-020-0646-0
Demir, C., and Demir, M. (2023). The impact of smoking on inflammation indices: A cross-sectional study. Med. Sci. Int. Med. J. 12, 347–351. doi: 10.5455/medscience.2023.02.028
Dommershuijsen, L., Ruiter, R., Erler, N., Rizopoulos, D., Ikram, M., and Ikram, M. (2022). Peripheral immune cell numbers and C-Reactive protein in Parkinson’s disease: Results from a population-based study. J. Parkinsons Dis. 12, 667–678. doi: 10.3233/JPD-212914
Dorsey, E., Sherer, T., Okun, M., and Bloem, B. (2018). The emerging evidence of the Parkinson pandemic. J. Parkinsons Dis. 8, S3–S8. doi: 10.3233/jpd-181474
Fang, X., Han, D., Cheng, Q., Zhang, P., Zhao, C., Min, J., et al. (2018). Association of levels of physical activity with risk of Parkinson disease: A systematic review and meta-analysis. JAMA Netw. Open 1:e182421.
Frank, E., and Harrell, J. (2015). Regression Modeling Strategies with Applications to Linear Models, Logistic and Ordinal Regression, and Survival Analysis. Berlin: Springer.
Gao, X., Chen, H., Schwarzschild, M., and Ascherio, A. (2011). Use of Ibuprofen and risk of Parkinson disease. Neurology 76, 863–869.
Gao, X., Simon, K., Han, J., Schwarzschild, M., and Ascherio, A. (2009). Family history of melanoma and Parkinson disease risk. Neurology 73, 1286–1291.
Gate, D. (2022). New perspectives on immune involvement in Parkinson’s disease pathogenesis. J. Parkinsons Dis. 12, S5–S11. doi: 10.3233/jpd-223240
Hirsch, L., Jette, N., Frolkis, A., Steeves, T., and Pringsheim, T. (2016). The incidence of Parkinson’s disease: A systematic review and meta-analysis. Neuroepidemiology 46, 292–300. doi: 10.1159/000445751
Hosseini, S., Shafiabadi, N., Khanzadeh, M., Ghaedi, A., Ghorbanzadeh, R., Azarhomayoun, A., et al. (2023). Neutrophil to lymphocyte ratio in Parkinson’s disease: A systematic review and meta-analysis. BMC Neurol. 23:333. doi: 10.1186/s12883-023-03380-7
Hu, B., Yang, X., Xu, Y., Sun, Y., Sun, C., Guo, W., et al. (2014). Systemic immune-inflammation index predicts prognosis of patients after curative resection for hepatocellular carcinoma. Clin. Cancer Res. 20, 6212–6222. doi: 10.1158/1078-0432.CCR-14-0442
Hughes, K., Gao, X., Kim, I., Wang, M., Weisskopf, M., Schwarzschild, M., et al. (2017). Intake of dairy foods and risk of Parkinson disease. Neurology 89, 46–52.
Imhof, A., Froehlich, M., Brenner, H., Boeing, H., Pepys, M., and Koenig, W. (2001). Effect of alcohol consumption on systemic markers of inflammation. Lancet 357, 763–767. doi: 10.1016/S0140-6736(00)04170-2
Jensen, M., Jacobs, B., Dobson, R., Bandres-Ciga, S., Blauwendraat, C., Schrag, A., et al. (2021). Lower lymphocyte count is associated with increased risk of Parkinson’s disease. Ann. Neurol. 89, 803–812. doi: 10.1002/ana.26034
Kim, R., Kim, H., Kim, A., Jang, M., Kim, A., Kim, Y., et al. (2018). Peripheral blood inflammatory markers in early Parkinson’s disease. J. Clin. Neurosci. 58, 30–33. doi: 10.1016/j.jocn.2018.10.079
Kobylecki, C. (2020). Update on the diagnosis and management of Parkinson’s disease. Clin. Med (Lond). 20, 393–398. doi: 10.7861/clinmed.2020-0220
Lee, P., Bordelon, Y., Bronstein, J., and Ritz, B. (2012). Traumatic brain injury, paraquat exposure, and their relationship to Parkinson disease. Neurology 79, 2061–2066.
Ley, K., Hoffman, H., Kubes, P., Cassatella, M., Zychlinsky, A., Hedrick, C., et al. (2018). Neutrophils: New insights and open questions. Sci. Immunol. 3:eaat4579. doi: 10.1126/sciimmunol.aat4579
Li, F., Weng, G., Zhou, H., Zhang, W., Deng, B., Luo, Y., et al. (2024). The neutrophil-to-lymphocyte ratio, lymphocyte-to-monocyte ratio, and neutrophil-to-high-density-lipoprotein ratio are correlated with the severity of Parkinson’s disease. Front. Neurol. 15:1322228. doi: 10.3389/fneur.2024.1322228
Li, S., Zhang, Q., Gao, Y., Nie, K., Liang, Y., Zhang, Y., et al. (2021). Serum folate, vitamin B12 levels, and systemic immune-inflammation index correlate with motor performance in Parkinson’s disease: A cross-sectional study. Front. Neurol. 12:665075. doi: 10.3389/fneur.2021.665075
Lindestam Arlehamn, C., Dhanwani, R., Pham, J., Kuan, R., Frazier, A., Rezende Dutra, J., et al. (2020). Alpha-Synuclein-specific T cell reactivity is associated with preclinical and early Parkinson’s disease. Nat. Commun. 11:1875. doi: 10.1038/s41467-020-15626-w
Liu, L., Shen, Q., Bao, Y., Xu, F., Zhang, D., Huang, H., et al. (2023). Association between dietary intake and risk of Parkinson’s disease: Cross-sectional analysis of survey data from Nhanes 2007-2016. Front. Nutr. 10:1278128. doi: 10.3389/fnut.2023.1278128
Munoz-Delgado, L., Macias-Garcia, D., Jesus, S., Martin-Rodriguez, J., Labrador-Espinosa, M., Jimenez-Jaraba, M., et al. (2021). Peripheral immune profile and neutrophil-to-lymphocyte ratio in Parkinson’s disease. Mov. Disord. 36, 2426–2430. doi: 10.1002/mds.28685
Nie, Y., Zhou, H., Wang, J., and Kan, H. (2023). Association between systemic immune-inflammation index and diabetes: A population-based study from the Nhanes. Front. Endocrinol (Lausanne). 14:1245199. doi: 10.3389/fendo.2023.1245199
Pedersen, C., Ushakova, A., Skogseth, R., Alves, G., Tysnes, O., Aarsland, D., et al. (2023). Inflammatory biomarkers in newly diagnosed patients with Parkinson disease and related neurodegenerative disorders. Neurol. Neuroimmunol. Neuroinflamm 10:e200132. doi: 10.1212/NXI.0000000000200132
Qin, X., Zhang, S., Cao, C., Loh, Y., and Cheng, Y. (2016). Aberrations in peripheral inflammatory cytokine levels in Parkinson disease: A systematic review and meta-analysis. JAMA Neurol. 73, 1316–1324. doi: 10.1001/jamaneurol.2016.2742
Qiu, X., Xiao, Y., Wu, J., Gan, L., Huang, Y., and Wang, J. C. - (2019). Reactive protein and risk of Parkinson’s disease: A systematic review and meta-analysis. Front. Neurol. 10:384. doi: 10.3389/fneur.2019.00384
Qu, Y., Li, J., Qin, Q., Wang, D., Zhao, J., An, K., et al. (2023). A systematic review and meta-analysis of inflammatory biomarkers in Parkinson’s disease. NPJ Parkinsons Dis. 9:18. doi: 10.1038/s41531-023-00449-5
Riboldi, G., Frattini, E., Monfrini, E., Frucht, S., and Di Fonzo, A. A. (2022). Practical approach to early-onset Parkinsonism. J. Parkinsons Dis. 12, 1–26. doi: 10.3233/JPD-212815
Rose, K., Schwarzschild, M., and Gomperts, S. (2024). Clearing the smoke: What protects smokers from Parkinson’s disease? Mov. Disord. 39, 267–272. doi: 10.1002/mds.29707
Ross, G., Abbott, R., Petrovitch, H., Morens, D., Grandinetti, A., Tung, K., et al. (2000). Association of coffee and caffeine intake with the risk of Parkinson disease. JAMA 283, 2674–2679.
Sarkar, D., and Fisher, P. (2006). Molecular mechanisms of aging-associated inflammation. Cancer Lett. 236, 13–23. doi: 10.1016/j.canlet.2005.04.009
Schrag, A., and Schott, J. (2006). Epidemiological, clinical, and genetic characteristics of early-onset Parkinsonism. Lancet Neurol. 5, 355–363. doi: 10.1016/S1474-4422(06)70411-2
Shen, C., Guo, Y., Luo, W., Lin, C., and Ding, M. (2013). Serum urate and the risk of Parkinson’s disease: Results from a meta-analysis. Can. J. Neurol. Sci. 40, 73–79.
Shoelson, S., Lee, J., and Goldfine, A. (2006). Inflammation and insulin resistance. J. Clin. Invest. 116, 1793–1801. doi: 10.1172/JCI29069
Singh, T., and Newman, A. (2011). Inflammatory markers in population studies of aging. Ageing Res. Rev. 10, 319–329. doi: 10.1016/j.arr.2010.11.002
Stokholm, M., Iranzo, A., Ostergaard, K., Serradell, M., Otto, M., Svendsen, K., et al. (2017). Assessment of neuroinflammation in patients with idiopathic rapid-eye-movement sleep behaviour disorder: A case-control study. Lancet Neurol. 16, 789–796. doi: 10.1016/S1474-4422(17)30173-4
Sulzer, D., Alcalay, R., Garretti, F., Cote, L., Kanter, E., Agin-Liebes, J., et al. (2017). T cells from patients with Parkinson’s disease recognize alpha-synuclein peptides. Nature 546, 656–661. doi: 10.1038/nature22815
Tian, B., Yang, Y., Yang, C., Yan, L., Ding, Z., Liu, H., et al. (2022). Systemic immune-inflammation index predicts prognosis of cancer immuno therapy: Systemic review and meta-analysis. Immunotherapy 14, 1481–1496. doi: 10.2217/imt-2022-0133
Trinh, J., and Farrer, M. (2013). Advances in the genetics of Parkinson disease. Nat. Rev. Neurology 9, 445–454.
Wei, H., Xu, D., Chen, J., Yu, H., Zhang, X., Liu, Z., et al. (2024). Age difference in the connection between systemic inflammatory response and metabolic syndrome. J. Clin. Endocrinol. Metab. doi: 10.1210/clinem/dgae669 Online ahead of print.
Willis, A., Roberts, E., Beck, J., Fiske, B., Ross, W., Savica, R., et al. (2022). Incidence of Parkinson disease in North America. npj Parkinson’s Dis. 8:170. doi: 10.1038/s41531-022-00410-y
Xu, H., Wu, W., Zhu, Q., Wang, J., Ding, P., Zhuang, Z., et al. (2024). Systemic immune-inflammation index predicts the prognosis of traumatic brain injury. World Neurosurg. 183, e22–e27. doi: 10.1016/j.wneu.2023.10.081
Xu, M., Chen, R., Liu, L., Liu, X., Hou, J., Liao, J., et al. (2021). Systemic immune-inflammation index and incident cardiovascular diseases among middle-aged and elderly Chinese adults: The Dongfeng-Tongji cohort study. Atherosclerosis 323, 20–29. doi: 10.1016/j.atherosclerosis.2021.02.012
Yang, Y., Wu, C., Hsu, P., Chen, S., Huang, S., Chan, W., et al. (2020). Systemic immune-inflammation index (Sii) predicted clinical outcome in patients with coronary artery disease. Eur. J. Clin. Invest. 50:e13230. doi: 10.1111/eci.13230
Zhang, D., Jiang, H., and Xie, J. (2014). Alcohol intake and risk of Parkinson’s disease: A meta-analysis of observational studies. Mov. Disord. 29, 819–822. doi: 10.1002/mds.25863
Keywords: systemic immune-inflammation index, Parkinson’s disease, inflammation, cross-sectional, risk factor
Citation: Zhao J, Wu Z, Cai F, Yu X and Song Z (2025) Higher systemic immune-inflammation index is associated with increased risk of Parkinson’s disease in adults: a nationwide population-based study. Front. Aging Neurosci. 17:1529197. doi: 10.3389/fnagi.2025.1529197
Received: 22 November 2024; Accepted: 20 January 2025;
Published: 07 February 2025.
Edited by:
Elisa Tatti, City College of New York (CUNY), United StatesReviewed by:
François-Xavier Lejeune, INSERM U1127 Institut du Cerveau et de la Moelle épinière (ICM), FranceCopyright © 2025 Zhao, Wu, Cai, Yu and Song. This is an open-access article distributed under the terms of the Creative Commons Attribution License (CC BY). The use, distribution or reproduction in other forums is permitted, provided the original author(s) and the copyright owner(s) are credited and that the original publication in this journal is cited, in accordance with accepted academic practice. No use, distribution or reproduction is permitted which does not comply with these terms.
*Correspondence: Zhenyu Song, c29uZ3poeTE5QGdtYWlsLmNvbQ==
Disclaimer: All claims expressed in this article are solely those of the authors and do not necessarily represent those of their affiliated organizations, or those of the publisher, the editors and the reviewers. Any product that may be evaluated in this article or claim that may be made by its manufacturer is not guaranteed or endorsed by the publisher.
Research integrity at Frontiers
Learn more about the work of our research integrity team to safeguard the quality of each article we publish.