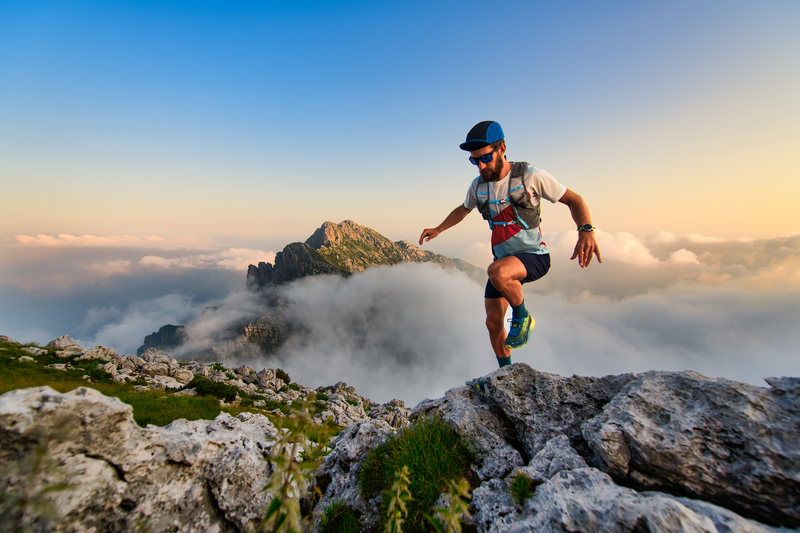
94% of researchers rate our articles as excellent or good
Learn more about the work of our research integrity team to safeguard the quality of each article we publish.
Find out more
BRIEF RESEARCH REPORT article
Front. Aging Neurosci.
Sec. Alzheimer's Disease and Related Dementias
Volume 17 - 2025 | doi: 10.3389/fnagi.2025.1527636
This article is part of the Research Topic Blood, Cerebrospinal Fluid, and Vascular Biomarkers for Dementia View all 15 articles
The final, formatted version of the article will be published soon.
You have multiple emails registered with Frontiers:
Please enter your email address:
If you already have an account, please login
You don't have a Frontiers account ? You can register here
Alzheimer's disease (AD) is a typical neurodegenerative disease that presents challenges due to the lack of biomarkers to identify AD. A growing body of evidence highlights the critical role of circadian rhythms in AD.The differential expressed clock genes (DECG) were identified between AD and ND (non-demented controls). Functional enrichment analysis was executed on the DECG. Candidate diagnostic biomarkers for AD were screened by machine learning. ROC and nomograms were constructed to evaluate candidate biomarkers. In addition, therapeutics targeting predictive biomarkers were screened through the DGIdb website. Finally, the mRNA-miRNA network was constructed.Nine genes were identified through the DECG analysis between AD and ND. Enrichment analysis of 9 genes indicated that the pathways were enriched in Long-term potentiation and Circadian entrainment. Four clock genes (GSTM3, ERC2, PRKCG and HLA-DMA) of AD were screened using Lasso regression, random forest, SVM and GMM. The diagnostic performance of 4 genes was evaluated by ROC curve. Furthermore, the nomogram indicated that ERC2, PRKCG and HLA-DMA are good biomarkers in diagnosing AD. Single-gene GSEA indicated that the main enrichment pathways were oxidative phosphorylation, pathways of neurodegeneration -multiple diseases, etc. The results of immune cell infiltration analysis indicated that there were significant differences in 15 immune cell subsets between AD and ND group. Moreover, 23 drugs targeting HLA-DMA and 8 drugs targeting PRKCG were identified through the DGIdb website.We identified 3 predictive biomarkers for AD associated with clock genes, thus providing promising therapeutic targets for AD.
Keywords: Clock genes, Alzheimer's disease, machine learning, Candidate diagnostic biomarkers, Immune cell infiltration analysis
Received: 14 Nov 2024; Accepted: 03 Mar 2025.
Copyright: © 2025 Li, Li, Su, Zhang, Guo, Ge, Dong and Zhang. This is an open-access article distributed under the terms of the Creative Commons Attribution License (CC BY). The use, distribution or reproduction in other forums is permitted, provided the original author(s) or licensor are credited and that the original publication in this journal is cited, in accordance with accepted academic practice. No use, distribution or reproduction is permitted which does not comply with these terms.
* Correspondence:
Feng Zhang, Third Hospital of Hebei Medical University, Shijiazhuang, China
Disclaimer: All claims expressed in this article are solely those of the authors and do not necessarily represent those of their affiliated organizations, or those of the publisher, the editors and the reviewers. Any product that may be evaluated in this article or claim that may be made by its manufacturer is not guaranteed or endorsed by the publisher.
Research integrity at Frontiers
Learn more about the work of our research integrity team to safeguard the quality of each article we publish.