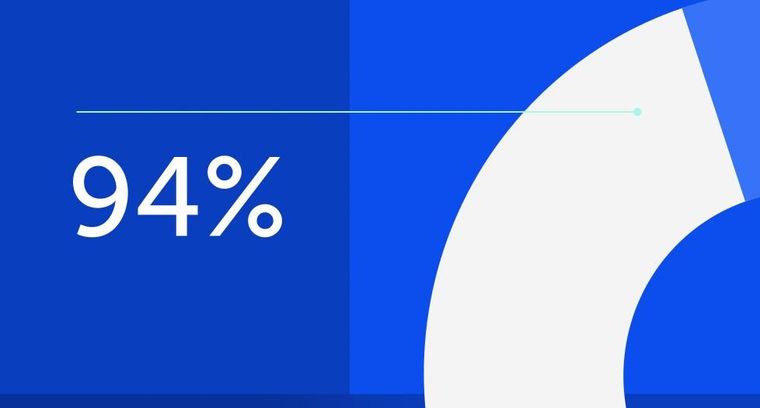
94% of researchers rate our articles as excellent or good
Learn more about the work of our research integrity team to safeguard the quality of each article we publish.
Find out more
ORIGINAL RESEARCH article
Front. Aging Neurosci., 18 March 2025
Sec. Neurocognitive Aging and Behavior
Volume 17 - 2025 | https://doi.org/10.3389/fnagi.2025.1506478
This article is part of the Research TopicEarly Dementia Detection: Memory, Cortical Function, and Biomarkers in the Classification of High-Risk Pre-Dementia Individuals in the Healthy Aging PeopleView all 5 articles
Background: The insula is a critical node of the salience network responsible for initiating network switching, and its dysfunctional connections are linked to the mechanisms of mild cognitive impairment (MCI). This study aimed to explore the changes in functional connectivity (FC) of insular subregions in MCI patients with varying levels of cerebrospinal fluid (CSF) pathological proteins, and to investigate the impact of these proteins on the brain network alterations in MCI.
Methods: Based on CSF Amyloid-beta (Aβ, A) and phosphorylated tau protein (p-tau, T), MCI patients were classified into 54 A−T−, 28 A+T−, and 52 A+T+ groups. Seed-based FC analysis was employed to compare the FC differences of insular subregions across the three groups. Correlation analysis was further conducted to explore the relationship between altered FC and cognitive function. Finally, ROC curve analysis was used to assess the diagnostic value of altered FC of insular subregion in distinguishing between the groups.
Results: In the left ventral anterior insula, left dorsal anterior insula, and bilateral posterior insular subnetworks, both the A+T− and A+T+ groups showed increased FC compared to the A−T− group, with the A+T+ group showing further increased FC compared to the A+T− group. Additionally, FC of the left cerebellar posterior lobe was negatively correlated with RAVLT-learning, and FC of the left middle frontal gyrus was negatively correlated with p-tau levels. Finally, logistic regression analysis demonstrated that multivariable analysis had high sensitivity and specificity in distinguishing between the groups.
Conclusion: This study showed that MCI patients with abnormal CSF pathological protein levels exhibit compensatory increases in FC of insular subregions, which in turn affect cognitive function. Our findings contributed to a better understanding of the pathophysiology and underlying neural mechanisms of MCI.
The core symptom of mild cognitive impairment (MCI) is cognitive decline, which often represents the prodromal stage of Alzheimer’s disease (AD) (Jessen et al., 2014; Xue et al., 2019). The progression rate from MCI to AD varies among studies, with an average annual rate of 10%–15% (Petersen et al., 2001; Guo et al., 2012). Over a span of 6 years, more than 80% of individuals with MCI have been observed to eventually develop AD (Busse et al., 2006). Understanding the pathological mechanisms of MCI, along with early diagnosis and timely intervention, is crucial for potentially reversing its progression (Kim et al., 2021). Studies have shown that various biomarkers can predict the development of MCI and AD, as well as the likelihood of MCI converting to AD years before clinical symptoms appear (Lin et al., 2020; Crystal et al., 2023). Consistent evidence indicates that the hallmark pathologies, Amyloid-beta (Aβ) and tau protein, are central to AD’s core pathology. Multiple studies have focused on the development of Aβ and tau biomarkers to track the progression of AD, revealing that Aβ deposition is one of the earliest events, followed by tau accumulation (Jack et al., 2013; Selkoe and Hardy, 2016; Jack et al., 2018). However, recent evidence suggests tau pathology can emerge independently of Aβ and may even precede Aβ deposition in certain brain regions (Silvestro et al., 2022). These findings highlight the complex interplay between Aβ and tau pathology in the progression of AD, suggesting that their mechanisms may involve multiple interwoven pathological pathways, thereby underscoring the need for further research. Therefore, understanding the relationship between Aβ, tau, and brain function is key to grasping the early functional changes in AD, providing valuable strategies for the early diagnosis and treatment of MCI.
Resting-state functional magnetic resonance imaging (fMRI) has been proven to be an effective method for analyzing complex neural networks by measuring intrinsic brain fluctuations in blood oxygen level-dependent signals (Biswal et al., 1995; Zhang and Raichle, 2010; Xue et al., 2020). The insula is a core region of the salience network (SN), involved in higher-order cognition, autonomic functions, emotional, and sensory processes (Naqvi and Bechara, 2010). The insula consists of several heterogeneous subregions, including the ventral anterior insula (vAI), dorsal anterior insula (dAI), and posterior insula (PI) (Yang et al., 2022; Zhao et al., 2023). The posterior insula is responsible for collecting and integrating various interoceptive signals, which are then relayed to the anterior insula for higher-order representation and perception processing. The anterior insula is associated with arousal, interoceptive awareness, and cognitive-emotional processing, with the dorsal anterior insula being more involved in higher cognitive functions. These distinct functional roles suggest that different insular subregions may contribute uniquely to cognitive, emotional, and sensory processing, making them critical targets for investigating network alterations in MCI and AD.
Previous studies have shown that different insular subregions exhibit distinct resting-state functional connectivity (FC) patterns and are part of different functional networks, which are differentially affected across AD spectrum (Deen et al., 2011; Zhang et al., 2016; Yang et al., 2022). Additionally, changes in the FC of insular subregions have been suggested to contribute to the cognitive, emotional, and sensory symptoms observed in the AD spectrum (Liu et al., 2018; Li et al., 2024; Tian et al., 2024). However, there is still a lack of systematic research on the role of Aβ and tau pathology in the functional organization of insular subregions in MCI patients. In particular, the specific impact of early AD pathological burden on the FC patterns of different insular subregions remains unclear. Therefore, investigating how Aβ and tau burden levels influence changes in insular FC in MCI patients will not only enhance our understanding of the role of pathological burden in neural communication and information transmission during the MCI stage but also provide potential neuroimaging biomarkers for the early identification of AD.
Therefore, the purpose of this study was to investigate the FC abnormalities of insular subregions in MCI patients with different cerebrospinal fluid (CSF) pathological protein levels and their correlation with cognitive function. We hypothesized that the presence of pathological proteins affects the FC of insular subregions and was associated with changes in cognitive function. These alterations were crucial for understanding the pathological mechanisms of MCI and predicting the levels of pathological proteins in MCI.
The data utilized in this study were all sourced from the Alzheimer’s Disease Neuroimaging Initiative (ADNI) database1. The ADNI database provided detailed inclusion and exclusion criteria for MCI. The present study included all baseline MCI patients with resting-state fMRI data from the ADNI-2 and ADNI-3 phases, totaling 241 participants. Due to partial data loss, 225 participants remained. After further excluding 13 participants with excessive head motion (cumulative translation or rotation of > 3.0 mm or 3.0°), a final total of 212 participants were included in the analysis. The specific MCI diagnostic and inclusion criteria can be found in the SI Methods. Following previous studies, a threshold of < 977 pg/ml was applied to identify abnormal CSF Aβ42, and > 24 pg/ml was used to identify abnormal CSF phosphorylated tau protein (p-tau) (Hansson et al., 2018; Vromen et al., 2023). Based on these criteria, the MCI patients were categorized including abnormal Aβ42 and p-tau (A+T+), abnormal Aβ42 and normal p-tau (A+T−), normal Aβ42 and abnormal p-tau (A−T+), and normal Aβ42 and p-tau (A−T−). The A−T+ group was excluded due to their classification outside the AD spectrum according to the A/T/N framework (Yoon et al., 2022). As a result, the present study included 54 A−T− participants, 28 A+T− participants, and 52 A+T+ participants.
Ethical approval for the ADNI study was granted by the institutional review committees of all participating institutions. Written informed consent was obtained from participants or their authorized representatives. Further information was available on the ADNI website2.
To evaluate cognitive function, we conducted comparisons between groups using the composite scores for episodic memory (EM) and executive function (EF). Additional information on EM and EF were provided in SI Methods.
The CSF samples were collected per the Alzheimer’s Association Flow Chart for CSF biomarkers. The INNO-BIAALZBio3 immunoassay kit was used to determine CSF levels of Aβ42, total tau protein (t-tau), and p-tau. In subsequent statistical processing, if the value of Aβ42 exceeds 1,700, it will be treated as 1,700 for statistical purposes.
Detailed scanning information can be obtained from http://adni.loni.usc.edu/wp-content/uploads/2010/05/ADNI2 MRI Training-Manual-FINAL.pdf and http://adni.loni.usc.edu/wp-content/uploads/2017/07/ADNI3-MRI-protocols.pdf.
Preprocessing of resting-state fMRI data was performed using Data Processing and Analysis for Brain Imaging (DPABI3) software and its Data Processing Assistant for Resting-State fMRI (DPARSF) module in MATLAB 2021b4 (Yan et al., 2016). Details regarding image preprocessing were provided in SI Methods.
Seed-based FC analysis was carried out to explore the alternation of insula subdivisions using the DPABI software. According to previous study, six 6 mm spherical region of interest (ROI) were created, including left vAI (MNI space: −33, 13, −7), right vAI (MNI space: 32, 10, −6), left dAI (MNI space: −38, 6, 2), right dAI (MNI space: 35, 7, 3), left PI (MNI space: −38, −6, 5) and right PI (MNI space: 35, −11, 6) (Lu et al., 2020). The mean time series of each ROI was extracted as the reference time course, and voxel-wise Pearson correlation analysis was performed between each ROI and the entire brain within the gray matter mask. Then, a Fisher’s r-to-z transformation was performed to improve the normality. Finally, for each subject, we generated six z-score maps that represented the intrinsic FC patterns of the six insular subregions.
Statistical analyses were conducted using Statistical Package for the Social Sciences (SPSS) software, version 25.0 (IBM, Armonk, New York, NY, United States). ANOVA and chi-square tests were applied to compare demographic characteristics, neurocognitive scales, and CSF pathological protein levels across the three groups: A−T−, A+T−, A+T+. Post hoc comparisons were adjusted using Bonferroni correction, with statistical significance set at p < 0.05.
A one-way ANOVA analysis was performed to assess differences in FC of each insular subregion after controlling for the influence of age, gender, and years of education (GRF corrected, voxel p < 0.005, cluster p < 0.05). Subsequently, post hoc comparisons were conducted using two-sample t-tests, with the resulting mask from the ANOVA analysis with age, gender, and years of education as covariates (GRF corrected, voxel p < 0.005, cluster p < 0.05).
Correlation analyses were carried out in SPSS to explore relationships between altered FC of each insular subregion and cognitive function, as well as CSF pathological proteins, while adjusting for age, sex, and years of education as covariates (p < 0.05).
Univariate and multivariable binary logistic regression were performed using SPSS software to evaluate the diagnosis value of altered FC of each insular subregion in A+T− and A+T+ groups. Altered FCs identified in the univariate analysis were included in the multivariable models through backward elimination, based on the likelihood ratio with a variable selection criterion of p < 0.05. The predictive performance of the univariate and multivariable models was assessed using the receiver operating characteristic (ROC) curve and the area under the ROC curve (AUC), with results presented in terms of accuracy, sensitivity, and specificity.
As shown in Table 1, the A+T+ group was older than both A−T− and A+T− groups. As expected, compared to the A−T− group, the A+T+ group showed lower scores in the immediate recall and learning components of Rey Auditory Verbal Learning Test (RAVLT), and the composite EM score (Bonferroni corrected, p < 0.05).
In the left vAI subnetwork, the ANOVA analysis showed significant alterations in FC across three groups, including the right cerebellum posterior lobe (CPL), and bilateral superior frontal gyrus (SFG). Compared to A-T- group, A+T− group showed increased FC in the right CPL and left SFG while A+T+ showed increased FC in the right CPL and left SFT. Compared to A+T− group, the A+T+ group showed increased FC in the left SFG (GRF corrected, voxel p < 0.005, cluster p < 0.05). These results were obtained while accounting for age, sex, and years of education (Figure 1 and Table 2).
Figure 1. Brain regions exhibiting significant differences in functional connectivity of the left ventral anterior insula. (A) Significant differences in functional connectivity of the left ventral anterior insula among three groups, including A−T−, A+T−, and A+T+ (GRF corrected, voxel p < 0.005, cluster p < 0.05); (B–D) Results of post hoc analysis in voxel-wise analysis (GRF corrected, voxel p < 0.005, cluster p < 0.05). A+T+, abnormal Aβ42 and p-tau; A+T−, abnormal Aβ42 and normal p-tau; A−T−, normal Aβ42 and p-tau.
Table 2. The differences of functional connectivity (FC) of left ventral anterior insula across three groups.
In the left dAI subnetwork, the ANOVA analysis showed significant alternations in FC across three groups, including the right CPL, left SFG and left middle frontal gyrus (MFG). Compared to A−T− group, the A+T− group showed increased FC in the right CPL while A+T+ group showed increased FC in the right CPL, left SFG, and left MFG. Compared to A+T− group, the A+T+ group showed increased FC in the SFG (GRF corrected, voxel p < 0.005, cluster p < 0.05). These results were obtained while accounting for age, sex, and years of education (Figure 2 and Table 3).
Figure 2. Brain regions exhibiting significant differences in functional connectivity of the left dorsal anterior insula. (A) Significant differences in functional connectivity of the left dorsal anterior insula among three groups, including A−T−, A+T−, and A+T+ (GRF corrected, voxel p < 0.005, cluster p < 0.05); (B–D) Results of post hoc analysis in voxel-wise analysis (GRF corrected, voxel p < 0.005, cluster p < 0.05). A+T+, abnormal Aβ42 and p-tau; A+T−, abnormal Aβ42 and normal p-tau; A−T−, normal Aβ42 and p-tau.
Table 3. The difference of functional connectivity (FC) of left dorsal anterior insula across three groups.
In the left PI subnetwork, the ANOVA analysis showed significant alternations in FC across three groups, including bilateral CPL and left MFG. Compared to A−T− group, the A+T− group showed increased right CPL and the A+T+ group showed increased FC in the bilateral CPL and left MFG. Compared to A+T− group, the A+T+ group showed increased FC in the bilateral CPL and left MFG (GRF corrected, voxel p < 0.005, cluster p < 0.05). These results were obtained while accounting for age, sex, and years of education (Figure 3 and Table 4).
Figure 3. Brain regions exhibiting significant differences in functional connectivity of the left posterior insula. (A) Significant differences in functional connectivity of the left posterior insula among three groups, including A−T−, A+T−, and A+T+ (GRF corrected, voxel p < 0.005, cluster p < 0.05); (B–D) Results of post hoc analysis in voxel-wise analysis (GRF corrected, voxel p < 0.005, cluster p < 0.05). A+T+, abnormal Aβ42 and p-tau; A+T−, abnormal Aβ42 and normal p-tau; A−T−, normal Aβ42 and p-tau.
Table 4. The difference of functional connectivity (FC) of left posterior insula across three groups.
In the right PI subnetwork, the ANOVA analysis showed significant alternations in FC across three groups, including bilateral MFG and SFG. Compared to the A−T− group, the A+T− group showed increased FC in the right SFG while the A+T+ group showed increased FC in the bilateral MFG. Compared to the A+T− group, the A+T+ group showed increased FC in the left MFG and left SFG (GRF corrected, voxel p < 0.005, cluster p < 0.05). These results were obtained while accounting for age, sex, and years of education (Figure 4 and Table 5).
Figure 4. Brain regions exhibiting significant differences in functional connectivity of the right posterior insula. (A) Significant differences in functional connectivity of the right posterior insula among three groups, including A−T−, A+T−, and A+T+ (GRF corrected, voxel p <0.005, cluster p < 0.05); (B–D) Results of post hoc analysis in voxel-wise analysis (GRF corrected, voxel p < 0.005, cluster p < 0.05). A+T+, abnormal Aβ42 and p-tau; A+T−, abnormal Aβ42 and normal p-tau; A−T−, normal Aβ42 and p-tau.
Table 5. The difference of functional connectivity (FC) of right posterior insula across three groups.
The value of FC of the left CPL was negatively associated with RAVLT-immediate (r = −0.237, p = 0.044) and p-tau (r = −0.246, p = 0.036) (Figure 5).
Figure 5. Significant associations between altered functional connectivity of each insula subnetwork and cognitive function. (A,B) Age, gender, and years of education were included as covariates of results. CPL.L, left cerebellum posterior lobe; MFG.L, left middle frontal gyrus; A+T+, abnormal Aβ42 and p-tau; A+T−, abnormal Aβ42 and normal p-tau.
The ROC curves of each altered index were presented in Figure 6. Obviously, the best-fitting model was the multivariable models (red line), which combined altered FC of each insular subregion. The AUC for distinguishing A+T− from A−T− using the multivariable model was 0.837, with 79.6% sensitivity, and 75.0% specificity (p < 0.001). In the group of A+T+ and A−T−, the AUC using the multivariable model was 0.857, with 83.3% sensitivity, and 79.8% specificity (p < 0.001). Lastly, the AUC for differentiating A+T+ from A+T− using the multivariable model was 0.882, with 89.3% sensitivity, and 75.0% specificity (p < 0.001).
Figure 6. Diagnosis and differentiation of A+T− and A+T+ based on ROC analysis. (A) ROC curve showing the classification between A+T− and A−T−; (B) ROC curve showing the classification between A+T+ and A−T−; (C) ROC curve showing the classification between A+T+ and A+T−. A+T+, abnormal Aβ42 and p-tau; A+T−, abnormal Aβ42 and normal p-tau; A−T−, normal Aβ42 and p-tau. RAVLT, Rey Auditory Verbal Learning Test; p-tau, phosphorylated tau protein.
This study aimed to investigate the FC abnormalities of insular subregions in MCI patients with different CSF pathological protein levels and their correlation with cognitive function. The findings revealed that as Aβ42 and p-tau abnormalities increased, the FC of insular subregions showed compensatory enhancement, which was associated with cognitive function. Moreover, these abnormalities had significant value in distinguishing between the groups. These discoveries may offer new insights into the pathophysiology of MCI. Clinically, features that provide more accurate and comprehensive information could serve as objective biomarkers, enhancing the ability to diagnose and treat MCI, ultimately improving patient outcomes.
The results of this study indicated that the changes in FC across different insular subregions were similar. As Aβ and tau abnormalities increased, the FC of insular subregions gradually strengthened, which was surprising. According to previous literature, a negative correlation typically existed between tau deposition and FC (Sepulcre et al., 2017; Berron et al., 2020). Previous studies suggested that the loss of connectivity was related to tau-induced progressive structural damage and neuronal death, as tau pathology disrupted axonal stability and impaired axonal transport (Iqbal et al., 2005; Wales and Leung, 2021). Tau-PET studies showed that tau neurofibrillary tangles had adverse effects on brain connectivity in older adults and AD patients, with elevated tau levels linked to weakened intra-network connectivity, a reduction in strongly connected nodes, and decreased global and local network efficiency (Cope et al., 2018; Wisch et al., 2020). However, as research progressed, Quevenco et al. (2020) found that tau pathology was associated with increased activity in more extensive brain regions. They observed that enhanced connectivity between the posterior default mode network (DMN) and areas outside the DMN was positively correlated with overall Aβ levels and local tau accumulation. Moreover, studies proposed that in the preclinical AD process, an initial stage of hyperconnectivity may precede a subsequent stage of diminished connectivity (Schultz et al., 2017). In conclusion, this study revealed a pattern of increased FC in insular subregions as tau pathological burden increased. This phenomenon may involve compensatory neural regulation, early network hyperconnectivity, and potential functional network impairments (Penalba-Sanchez et al., 2022). Future research should further investigate whether these changes represent a transient adaptive adjustment or an early indicator of AD progression, providing new insights for early diagnosis and intervention strategies in the MCI stage.
The results showed a significant increase in the FC between the insula and both the MFG and SFG. The frontal lobe played a critical role in cognitive function, especially in its involvement in the fronto-subcortical circuits (Iaria et al., 2008). Additionally, the MFG and SFG were key regions of the executive control network (ECN) and the DMN, respectively (Liu et al., 2021; Zheng et al., 2024). Notably, the insula is a core region of the salience network (SN) (Xue et al., 2021). The enhanced FC between the insula and these frontal regions also reflected increased FC between the SN and both the ECN and DMN. SN plays a crucial role in responding to biologically and cognitively relevant environmental pressures or events, and it is linked to working memory and higher-order cognitive management (Menon and Uddin, 2010). Within brain networks, the SN is not only an important hub and starting point but also plays a key role in activating brain networks and facilitating the switch between the ECN and DMN during cognitively demanding tasks (Menon and Uddin, 2010; Goulden et al., 2014). In healthy individuals, there was a dynamic balance between the SN and DMN, often showing an inverse relationship (Fox et al., 2005; Sridharan et al., 2008). Although the exact nature of this increased SN connectivity was unclear, one possibility was that diminished inhibitory control may drive the commonly reported lack of task-related DMN suppression associated with Aβ pathology (Sperling et al., 2009; Elman et al., 2016). This disrupted balance may partially explain why hyperconnectivity between the SN and frontal regions was observed in this study. Rather than reflecting a purely compensatory mechanism, this increased FC may indicate reduced network specificity and a breakdown in functional segregation. Thus, the observed hyperconnectivity may represent a compensatory effort by the SN to maintain cognitive functions in the early stages of neurodegeneration, but as pathological burden increases, this mechanism may become inefficient and contribute to cognitive decline. Future studies should investigate whether these FC changes persist or diminish over time and explore their relationship with cognitive performance trajectories.
Additionally, the FC between the insula and the CPL was enhanced and showed a significant association with cognitive function. Specifically, as FC within the cerebellar hemisphere increased, RAVLT-learning scores progressively decreased, indicating that changes in cerebellar FC may be closely linked to declines in learning and memory abilities. This finding was consistent with the study by Aschenbrenner et al., who identified tau pathology as a strong predictor of overall cognitive decline (Aschenbrenner et al., 2018). Other studies similarly showed that patients who were positive for both Aβ and tau experience more severe cognitive decline, aligning with our findings and suggesting that the accumulation of pathological proteins had a significant impact on cognitive function (Kim et al., 2021; Ge et al., 2022). In recent years, researchers have increasingly focused on the role of the cerebellum in cognitive functions. Traditionally viewed as a key regulator of motor coordination, recent studies have demonstrated that the cerebellum also plays a critical role in cognition, emotional regulation, and autonomic nervous system function (Cutando et al., 2022). Numerous prior studies have revealed altered FC in the CPL of MCI/AD patients, underscoring the critical role this region plays in the AD spectrum (Yuan et al., 2023; Xue et al., 2024). These findings highlight the importance of FC between the insula and the CPL, particularly in understanding the pathological mechanisms underlying the AD spectrum. The increased FC between the insula and the CPL may reflect a compensatory response, where heightened connectivity helps maintain cognitive function despite accumulating pathology. This may indicate that the cerebellum and insula work together to compensate for declining cortical efficiency, particularly in memory and executive function tasks. However, as Aβ and tau burden continues to increase, this compensatory mechanism may become insufficient, ultimately leading to network breakdown and severe cognitive impairment. The observed negative correlation between FC of CPL and cognitive performance suggested that, rather than being purely compensatory, hyperconnectivity in these regions may reflect inefficient or maladaptive neural processing. Similar maladaptive hyperconnectivity was reported in other brain networks during the progression from MCI to AD, and excessive connectivity may eventually lead to loss of connectivity as neurodegeneration advances (Xue et al., 2019; Chen et al., 2022; Shu et al., 2022). Thus, investigating the FC between insular subregions and the CPL not only deepens our understanding of the progression of AD but also provides new potential targets for future diagnosis and intervention.
More importantly, the ROC analysis demonstrated the potential of FC-based diagnostic models in early detection and risk stratification of AD pathology in MCI patients. The high AUC values suggested that altered FC in insular subregions could serve as a sensitive neuroimaging biomarker, aiding in the differentiation of MCI subtypes with varying levels of Aβ and tau burden. This is particularly relevant in clinical practice, as early identification of A+T+ individuals is crucial for timely intervention and disease-modifying therapies. Additionally, the multivariable model’s superior performance suggested that combining multiple FC alterations enhances diagnostic accuracy compared to single-region approaches, emphasizing the importance of network-level analyses in AD research. Integrating FC-based diagnostic models with other biomarkers, such as PET imaging and structural MRI, could enhance diagnostic accuracy and reduce reliance on invasive CSF testing. Furthermore, understanding FC alterations may provide insights into disease progression and potential therapeutic targets, paving the way for early interventions that could slow cognitive decline and improve patient outcomes.
Our study had some limitations. Firstly, the study was based on CSF pathological biomarkers, which resulted in a relatively small sample size. However, we applied strict criteria in data processing, which increased the reliability of the results. Future studies should consider validating these findings using a larger sample size. Secondly, CSF pathological proteins in AD lack regional specificity, so we cannot definitively determine whether our findings are driven by localized or whole-brain effects of AD pathology. This limitation may affect the interpretation of how pathological burden influences specific brain regions. In future studies, we plan to incorporate Aβ and tau PET imaging to further investigate the spatial distribution of AD pathology and elucidate its impact on different insular subregions. Thirdly, this study focused only on resting-state fMRI data. In the future, integrating multimodal MRI techniques such as fMRI, DTI, and sMRI may provide deeper insights into the neuroimaging mechanisms of MCI at different pathological protein levels. Additionally, incorporating more dynamic FC analysis methods, such as sliding window correlation analysis, along with task-based fMRI to investigate the dynamic functional changes of insular subregions, may further elucidate their critical role in the progression of MCI.
This study demonstrated that as Aβ42 and p-tau abnormalities increased in MCI patients, cognitive function declines, while compensatory increased in FC of insular subregions occur, indicating enhanced control of the SN over the ECN and DMN. Investigating the changes in FC of insular subregions in MCI patients with different CSF pathological protein levels can provide deeper insights into the neuroimaging mechanisms of MCI.
The original contributions presented in this study are included in this article/supplementary material, further inquiries can be directed to the corresponding authors.
The studies involving humans were approved by Ethical approval for the ADNI study was granted by the institutional review committees of all participating institutions. The studies were conducted in accordance with the local legislation and institutional requirements. The participants provided their written informed consent to participate in this study.
DZ: Data curation, Investigation, Software, Validation, Writing – original draft. CX: Data curation, Software, Writing – original draft, Investigation, Validation, Writing – review and editing. YF: Data curation, Software, Writing – original draft. YR: Data curation, Investigation, Software, Writing – original draft. WQ: Software, Writing – original draft. QY: Software, Writing – original draft. ZL: Investigation, Validation, Writing – original draft, Writing – review and editing. CX: Investigation, Validation, Writing – original draft, Writing – review and editing.
The author(s) declare that no financial support was received for the research and/or publication of this article.
Data collection and sharing for this project was funded by the Alzheimer’s Disease Neuroimaging Initiative (ADNI; National Institutes of Health Grant U01 AG024904) and DOD ADNI (Department of Defense award number W81XWH-12-2-0012). ADNI was funded by the National Institute on Aging, the National Institute of Biomedical Imaging and Bioengineering, and through generous contributions from the following: AbbVie; Alzheimer’s Association; Alzheimer’s Drug Discovery Foundation; Araclon Biotech; BioClinica, Inc.; Biogen; Bristol-Myers Squibb Company; CereSpir, Inc.; Cogstate; Eisai Inc.; Elan Pharmaceuticals, Inc.; Eli Lilly and Company; EuroImmun; F. Hoffmann-La Roche Ltd. and its affiliated company Genentech, Inc.; Fujirebio; GE Healthcare; IXICO Ltd.; Janssen Alzheimer Immunotherapy Research & Development, LLC.; Johnson & Johnson Pharmaceutical Research & Development LLC.; Lumosity; Lundbeck; Merck & Co., Inc.; Meso Scale Diagnostics, LLC.; NeuroRx Research; Neurotrack Technologies; Novartis Pharmaceuticals Corporation; Pfizer Inc.; Piramal Imaging; Servier; Takeda Pharmaceutical Company; and Transition Therapeutics. The Canadian Institutes of Health Research was providing funds to support ADNI clinical sites in Canada. Private sector contributions are facilitated by the Foundation for the National Institutes of Health (www.fnih.org). The grantee organization was the Northern California Institute for Research and Education, and the study was coordinated by the Alzheimer’s Therapeutic Research Institute at the University of Southern California. ADNI data are disseminated by the Laboratory for NeuroImaging at the University of Southern California.
The authors declare that the research was conducted in the absence of any commercial or financial relationships that could be construed as a potential conflict of interest.
The authors declare that no Generative AI was used in the creation of this manuscript.
All claims expressed in this article are solely those of the authors and do not necessarily represent those of their affiliated organizations, or those of the publisher, the editors and the reviewers. Any product that may be evaluated in this article, or claim that may be made by its manufacturer, is not guaranteed or endorsed by the publisher.
Aschenbrenner, A. J., Gordon, B. A., Benzinger, T. L. S., Morris, J. C., and Hassenstab, J. J. (2018). Influence of tau PET, amyloid PET, and hippocampal volume on cognition in Alzheimer disease. Neurology 91, e859–e866. doi: 10.1212/WNL.0000000000006075
Berron, D., van Westen, D., Ossenkoppele, R., Strandberg, O., and Hansson, O. (2020). Medial temporal lobe connectivity and its associations with cognition in early Alzheimer’s disease. Brain 143, 1233–1248. doi: 10.1093/brain/awaa068
Biswal, B., Yetkin, F. Z., Haughton, V. M., and Hyde, J. S. (1995). Functional connectivity in the motor cortex of resting human brain using echo-planar MRI. Magn. Reson. Med. 34, 537–541. doi: 10.1002/mrm.1910340409
Busse, A., Angermeyer, M. C., and Riedel-Heller, S. G. (2006). Progression of mild cognitive impairment to dementia: A challenge to current thinking. Br. J. Psychiatry 189, 399–404. doi: 10.1192/bjp.bp.105.014779
Chen, S., Song, Y., Wu, H., Ge, H., Qi, W., Xi, Y., et al. (2022). Hyperconnectivity associated with anosognosia accelerating clinical progression in amnestic mild cognitive impairment. ACS Chem. Neurosci. 13, 120–133. doi: 10.1021/acschemneuro.1c00595
Cope, T. E., Rittman, T., Borchert, R. J., Jones, P. S., Vatansever, D., Allinson, K., et al. (2018). Tau burden and the functional connectome in Alzheimer’s disease and progressive supranuclear palsy. Brain 141, 550–567. doi: 10.1093/brain/awx347
Crystal, O., Maralani, P. J., Black, S., Fischer, C., Moody, A. R., and Khademi, A. (2023). Detecting conversion from mild cognitive impairment to Alzheimer’s disease using FLAIR MRI biomarkers. Neuroimage Clin. 40:103533. doi: 10.1016/j.nicl.2023.103533
Cutando, L., Puighermanal, E., Castell, L., Tarot, P., Belle, M., Bertaso, F., et al. (2022). Cerebellar dopamine D2 receptors regulate social behaviors. Nat. Neurosci. 25, 900–911. doi: 10.1038/s41593-022-01092-8
Deen, B., Pitskel, N. B., and Pelphrey, K. A. (2011). Three systems of insular functional connectivity identified with cluster analysis. Cereb. Cortex 21, 1498–1506. doi: 10.1093/cercor/bhq186
Elman, J. A., Madison, C. M., Baker, S. L., Vogel, J. W., Marks, S. M., Crowley, S., et al. (2016). Effects of beta-amyloid on resting state functional connectivity within and between networks reflect known patterns of regional vulnerability. Cereb. Cortex 26, 695–707. doi: 10.1093/cercor/bhu259
Fox, M. D., Snyder, A. Z., Vincent, J. L., Corbetta, M., Van Essen, D. C., and Raichle, M. E. (2005). The human brain is intrinsically organized into dynamic, anticorrelated functional networks. Proc. Natl. Acad. Sci. U S A. 102, 9673–9678. doi: 10.1073/pnas.0504136102
Ge, X., Qiao, Y., Choi, J., Raman, R., Ringman, J. M., Shi, Y., et al. (2022). Enhanced association of tau pathology and cognitive impairment in mild cognitive impairment subjects with behavior symptoms. J. Alzheimers Dis. 87, 557–568. doi: 10.3233/JAD-215555
Goulden, N., Khusnulina, A., Davis, N. J., Bracewell, R. M., Bokde, A. L., McNulty, J. P., et al. (2014). The salience network is responsible for switching between the default mode network and the central executive network: Replication from DCM. Neuroimage 99, 180–190. doi: 10.1016/j.neuroimage.2014.05.052
Guo, M., Gao, L., Zhang, G., Li, Y., Xu, S., Wang, Z., et al. (2012). Prevalence of dementia and mild cognitive impairment in the elderly living in nursing and veteran care homes in Xi’an China. J. Neurol. Sci. 312, 39–44. doi: 10.1016/j.jns.2011.08.026
Hansson, O., Seibyl, J., Stomrud, E., Zetterberg, H., Trojanowski, J. Q., Bittner, T., et al. (2018). CSF biomarkers of Alzheimer’s disease concord with amyloid-beta PET and predict clinical progression: A study of fully automated immunoassays in BioFINDER and ADNI cohorts. Alzheimers Dement. 14, 1470–1481. doi: 10.1016/j.jalz.2018.01.010
Iaria, G., Committeri, G., Pastorelli, C., Pizzamiglio, L., Watkins, K. E., and Carota, A. (2008). Neural activity of the anterior insula in emotional processing depends on the individuals’ emotional susceptibility. Hum. Brain Mapp. 29, 363–373. doi: 10.1002/hbm.20393
Iqbal, K., Alonso Adel, C., Chen, S., Chohan, M. O., El-Akkad, E., Gong, C. X., et al. (2005). Tau pathology in Alzheimer disease and other tauopathies. Biochim. Biophys. Acta 1739, 198–210. doi: 10.1016/j.bbadis.2004.09.008
Jack, C. R., Bennett, D. A., Blennow, K., Carrillo, M. C., Dunn, B., Haeberlein, S. B., et al. (2018). NIA-AA research framework: Toward a biological definition of Alzheimer’s disease. Alzheimers Dement. 14, 535–562. doi: 10.1016/j.jalz.2018.02.018
Jack, C. R., Knopman, D. S., Jagust, W. J., Petersen, R. C., Weiner, M. W., Aisen, P. S., et al. (2013). Tracking pathophysiological processes in Alzheimer’s disease: An updated hypothetical model of dynamic biomarkers. Lancet Neurol. 12, 207–216. doi: 10.1016/S1474-4422(12)70291-0
Jessen, F., Wolfsgruber, S., Wiese, B., Bickel, H., Mosch, E., Kaduszkiewicz, H., et al. (2014). AD dementia risk in late MCI, in early MCI, and in subjective memory impairment. Alzheimers Dement. 10, 76–83. doi: 10.1016/j.jalz.2012.09.017
Kim, C. M., Montal, V., Diez, I., Orwig, W., and Alzheimer’s Disease Neuroimaging Initiative (2021). Network interdigitations of Tau and amyloid-beta deposits define cognitive levels in aging. Hum. Brain Mapp. 42, 2990–3004. doi: 10.1002/hbm.25350
Li, H., Fan, X., Li, K., Zhang, C., and Jia, X. (2024). Increased anterior insula connectivity associated with cognitive maintenance in amnestic mild cognitive impairment: A longitudinal study. Brain Imaging Behav. 18, 1001–1009. doi: 10.1007/s11682-024-00899-2
Lin, W., Gao, Q., Yuan, J., Chen, Z., Feng, C., Chen, W., et al. (2020). Predicting Alzheimer’s disease conversion from mild cognitive impairment using an extreme learning machine-based grading method with multimodal data. Front. Aging Neurosci. 12:77. doi: 10.3389/fnagi.2020.00077
Liu, W., Liu, L., Cheng, X., Ge, H., Hu, G., Xue, C., et al. (2021). Functional integrity of executive control network contributed to retained executive abilities in mild cognitive impairment. Front. Aging Neurosci. 13:710172. doi: 10.3389/fnagi.2021.710172
Liu, X., Chen, X., Zheng, W., Xia, M., Han, Y., Song, H., et al. (2018). Altered functional connectivity of insular subregions in Alzheimer’s disease. Front. Aging Neurosci. 10:107. doi: 10.3389/fnagi.2018.00107
Lu, L., Li, F., Chen, H., Wang, P., Zhang, H., Chen, Y. C., et al. (2020). Functional connectivity dysfunction of insular subdivisions in cognitive impairment after acute mild traumatic brain injury. Brain Imaging Behav. 14, 941–948. doi: 10.1007/s11682-020-00288-5
Menon, V., and Uddin, L. Q. (2010). Saliency, switching, attention and control: A network model of insula function. Brain Struct. Funct. 214, 655–667. doi: 10.1007/s00429-010-0262-0
Naqvi, N. H., and Bechara, A. (2010). The insula and drug addiction: An interoceptive view of pleasure, urges, and decision-making. Brain Struct. Funct. 214, 435–450. doi: 10.1007/s00429-010-0268-7
Penalba-Sanchez, L., Oliveira-Silva, P., Sumich, A. L., and Cifre, I. (2022). Increased functional connectivity patterns in mild Alzheimer’s disease: A rsfMRI study. Front. Aging Neurosci. 14:1037347. doi: 10.3389/fnagi.2022.1037347
Petersen, R. C., Doody, R., Kurz, A., Mohs, R. C., Morris, J. C., Rabins, P. V., et al. (2001). Current concepts in mild cognitive impairment. Arch. Neurol. 58, 1985–1992. doi: 10.1001/archneur.58.12.1985
Quevenco, F. C., van Bergen, J. M., Treyer, V., Studer, S. T., Kagerer, S. M., Meyer, R., et al. (2020). Functional brain network connectivity patterns associated with normal cognition at old-age, local beta-amyloid, Tau, and APOE4. Front. Aging Neurosci. 12:46. doi: 10.3389/fnagi.2020.00046
Schultz, A. P., Chhatwal, J. P., Hedden, T., Mormino, E. C., Hanseeuw, B. J., Sepulcre, J., et al. (2017). Phases of hyperconnectivity and hypoconnectivity in the default mode and salience networks track with amyloid and Tau in clinically normal individuals. J. Neurosci. 37, 4323–4331. doi: 10.1523/JNEUROSCI.3263-16.2017
Selkoe, D. J., and Hardy, J. (2016). The amyloid hypothesis of Alzheimer’s disease at 25 years. EMBO Mol. Med. 8, 595–608. doi: 10.15252/emmm.201606210
Sepulcre, J., Sabuncu, M. R., Li, Q., El Fakhri, G., Sperling, R., and Johnson, K. A. (2017). Tau and amyloid beta proteins distinctively associate to functional network changes in the aging brain. Alzheimers Dement. 13, 1261–1269. doi: 10.1016/j.jalz.2017.02.011
Shu, H., Chen, G., Ward, B. D., Chen, G., Wang, Z., Liu, D., et al. (2022). Imminent cognitive decline in normal elderly individuals is associated with hippocampal hyperconnectivity in the variant neural correlates of episodic memory. Eur. Arch. Psychiatry Clin. Neurosci. 272, 783–792. doi: 10.1007/s00406-021-01310-7
Silvestro, S., Valeri, A., and Mazzon, E. (2022). Aducanumab and its effects on Tau pathology: Is this the turning point of amyloid hypothesis? Int. J. Mol. Sci. 23:2011. doi: 10.3390/ijms23042011
Sperling, R. A., Laviolette, P. S., O’Keefe, K., O’Brien, J., Rentz, D. M., Pihlajamaki, M., et al. (2009). Amyloid deposition is associated with impaired default network function in older persons without dementia. Neuron 63, 178–188. doi: 10.1016/j.neuron.2009.07.003
Sridharan, D., Levitin, D. J., and Menon, V. (2008). A critical role for the right fronto-insular cortex in switching between central-executive and default-mode networks. Proc. Natl. Acad. Sci. U S A. 105, 12569–12574. doi: 10.1073/pnas.0800005105
Tian, H., Zheng, W., Wang, J., Liu, S., and Wang, Z. (2024). Altered functional connectivity of insular subregions in subjective cognitive decline. Front. Hum. Neurosci. 18:1404759. doi: 10.3389/fnhum.2024.1404759
Vromen, E. M., de Boer, S. C. M., Teunissen, C. E., Rozemuller, A., Sieben, A., Bjerke, M., et al. (2023). Biomarker A+T-: Is this Alzheimer’s disease or not? A combined CSF and pathology study. Brain 146, 1166–1174. doi: 10.1093/brain/awac158
Wales, R. M., and Leung, H. C. (2021). The effects of amyloid and Tau on functional network connectivity in older populations. Brain Connect. 11, 599–612.
Wisch, J. K., Roe, C. M., Babulal, G. M., Schindler, S. E., Fagan, A. M., Benzinger, T. L., et al. (2020). Resting state functional connectivity signature differentiates cognitively normal from individuals who convert to symptomatic Alzheimer’s disease. J. Alzheimers Dis. 74, 1085–1095. doi: 10.3233/JAD-191039
Xue, C., Sun, H., Hu, G., Qi, W., Yue, Y., Rao, J., et al. (2020). Disrupted patterns of rich-club and diverse-club organizations in subjective cognitive decline and amnestic mild cognitive impairment. Front. Neurosci. 14:575652. doi: 10.3389/fnins.2020.575652
Xue, C., Sun, H., Yue, Y., Wang, S., Qi, W., Hu, G., et al. (2021). Structural and functional disruption of salience network in distinguishing subjective cognitive decline and amnestic mild cognitive impairment. ACS Chem. Neurosci. 12, 1384–1394. doi: 10.1021/acschemneuro.1c00051
Xue, C., Yuan, B., Yue, Y., Xu, J., Wang, S., Wu, M., et al. (2019). Distinct disruptive patterns of default mode subnetwork connectivity across the spectrum of preclinical Alzheimer’s disease. Front. Aging Neurosci. 11:307. doi: 10.3389/fnagi.2019.00307
Xue, C., Zheng, D., Ruan, Y., Guo, W., Hu, J., Alzheimer’s Disease, et al. (2024). Alteration in temporal-cerebellar effective connectivity can effectively distinguish stable and progressive mild cognitive impairment. Front. Aging Neurosci. 16:1442721. doi: 10.3389/fnagi.2024.1442721
Yan, C. G., Wang, X. D., Zuo, X. N., and Zang, Y. F. (2016). DPABI: Data processing & analysis for (resting-state) brain imaging. Neuroinformatics 14, 339–351. doi: 10.1007/s12021-016-9299-4
Yang, W., Wang, S., Luo, J., Yan, C., Tang, F., Du, Y., et al. (2022). Longitudinal resting-state functional connectivity changes in the insular subdivisions of abstinent individuals with opioid use disorder. Psychiatry Res. 317:114808. doi: 10.1016/j.psychres.2022.114808
Yoon, B., Guo, T., Provost, K., Korman, D., Ward, T. J., Landau, S. M., et al. (2022). Abnormal tau in amyloid PET negative individuals. Neurobiol. Aging 109, 125–134. doi: 10.1016/j.neurobiolaging.2021.09.019
Yuan, Q., Xue, C., Liang, X., Qi, W., Chen, S., Song, Y., et al. (2023). Functional changes in the salience network of patients with amnesic mild cognitive impairment before and after repetitive transcranial magnetic stimulation. Brain Behav. 13:e3169. doi: 10.1002/brb3.3169
Zhang, D., and Raichle, M. E. (2010). Disease and the brain’s dark energy. Nat. Rev. Neurol. 6, 15–28. doi: 10.1038/nrneurol.2009.198
Zhang, Y., Xie, B., Chen, H., Li, M., Guo, X., and Chen, H. (2016). Disrupted resting-state insular subregions functional connectivity in post-traumatic stress disorder. Medicine 95:e4083. doi: 10.1097/MD.0000000000004083
Zhao, H., Turel, O., Bechara, A., and He, Q. (2023). How distinct functional insular subdivisions mediate interacting neurocognitive systems. Cereb. Cortex 33, 1739–1751. doi: 10.1093/cercor/bhac169
Keywords: mild cognitive impairment, Amyloid-beta, tau protein, insular subdivisions, functional connectivity, functional magnetic resonance imaging
Citation: Zheng D, Xue C, Feng Y, Ruan Y, Qi W, Yuan Q, Li Z and Xiao C (2025) The abnormal accumulation of pathological proteins and compensatory functional connectivity enhancement of insula subdivisions in mild cognitive impairment. Front. Aging Neurosci. 17:1506478. doi: 10.3389/fnagi.2025.1506478
Received: 05 October 2024; Accepted: 05 March 2025;
Published: 18 March 2025.
Edited by:
Oded Meiron, Bar-Ilan University, IsraelReviewed by:
Cristiano Capurso, University of Foggia, ItalyCopyright © 2025 Zheng, Xue, Feng, Ruan, Qi, Yuan, Li and Xiao. This is an open-access article distributed under the terms of the Creative Commons Attribution License (CC BY). The use, distribution or reproduction in other forums is permitted, provided the original author(s) and the copyright owner(s) are credited and that the original publication in this journal is cited, in accordance with accepted academic practice. No use, distribution or reproduction is permitted which does not comply with these terms.
*Correspondence: Zonghong Li, MTM5NTE3MzQ5ODlAMTYzLmNvbQ==; Chaoyong Xiao, eGNoYW95b25nQG5qbXUuZWR1LmNu
†These authors have contributed equally to this work and share first authorship
‡Data used in preparation of this article were obtained from the Alzheimer’s Disease Neuroimaging Initiative (ADNI) database (adni.loni.usc.edu). As such, the investigators within the ADNI contributed to the design and implementation of ADNI and/or provided data but did not participate in analysis or writing of this report. A complete listing of ADNI investigators can be found at: http://adni.loni.usc.edu/wp-content/uploads/how_to_apply/ADNI_Acknowledgement_List.pdf
Disclaimer: All claims expressed in this article are solely those of the authors and do not necessarily represent those of their affiliated organizations, or those of the publisher, the editors and the reviewers. Any product that may be evaluated in this article or claim that may be made by its manufacturer is not guaranteed or endorsed by the publisher.
Research integrity at Frontiers
Learn more about the work of our research integrity team to safeguard the quality of each article we publish.