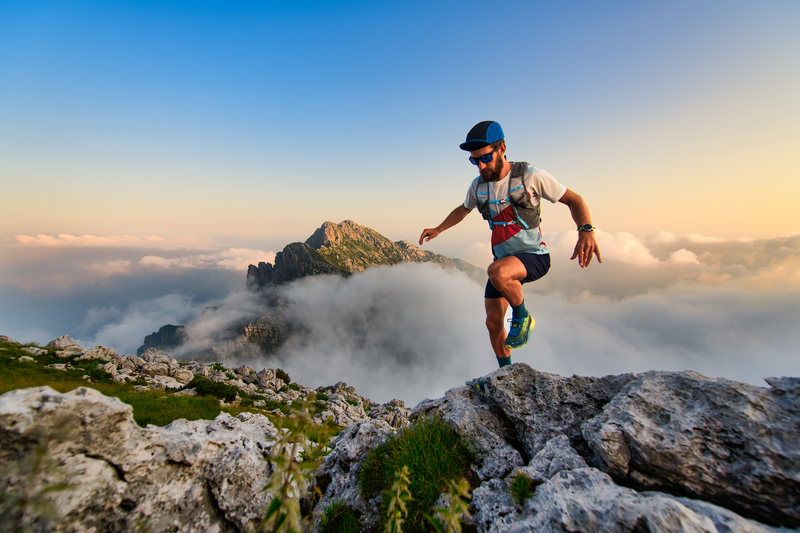
94% of researchers rate our articles as excellent or good
Learn more about the work of our research integrity team to safeguard the quality of each article we publish.
Find out more
ORIGINAL RESEARCH article
Front. Aging Neurosci.
Sec. Neurocognitive Aging and Behavior
Volume 17 - 2025 | doi: 10.3389/fnagi.2025.1498478
The final, formatted version of the article will be published soon.
You have multiple emails registered with Frontiers:
Please enter your email address:
If you already have an account, please login
You don't have a Frontiers account ? You can register here
Introduction: This study systematically explores the overall impact and interactions of body composition and nutritional inflammatory indices on early-onset mild cognitive impairment (EOMCI) in type 2 diabetes mellitus (T2DM). Methods: A cross-sectional study included 816 T2DM patients. Body composition indices included body mass index (BMI), waist circumference (WC), a body shape index (ABSI), body roundness index (BRI), visceral fat area (VFA), body fat percentage (BF%), and skeletal muscle mass index (SMMI). Nutritional inflammatory indices included the geriatric nutritional risk index (GNRI), prognostic nutritional index (PNI), C-reactive protein-albumin-lymphocyte index (CALLY), and fibrinogen-to-albumin ratio (FAR). K-means clustering and quantile g-computation (QGC) assessed the combined impact, with interactions evaluated by simple slope analysis. Results: K-means clustering revealed two distinct patterns: Low-pattern and High-pattern. The Low-pattern group exhibited significantly lower body composition indices (BMI 24.6 vs. 27.7 kg/m²; WC 88 vs. 99 cm; ABSI 0.081 vs. 0.084; BRI 3.89 vs. 5.02; VFA 91 vs. 112; BF% 29% vs. 31%; SMMI 9.38 vs. 10.48 kg/m²; all P<0.001) and poorer nutritional status with higher inflammation (GNRI 97.9 vs. 104.6; PNI 47.9 vs. 53.1; CALLY index 4 vs. 5; FAR 0.082 vs. 0.072; all P<0.05). This group had a higher prevalence of EOMCI (32% vs. 23%, P=0.006). After adjusting for confounders, the Low-pattern group had a 1.45-fold increased risk of EOMCI (OR 1.45, 95% CI 1.01-2.08). QGC analysis demonstrated that the combined overall effect of body composition and nutritional inflammatory indices was negatively associated with EOMCI risk. A one-quintile increase in all indices was linked to a significant 31.3% reduction in EOMCI risk (95% CI -44.4%, -15.0%). Interaction analysis revealed that abdominal obesity (ABSI > 0.08), combined with malnutrition (low GNRI), significantly increased EOMCI risk (Pinteraction = 0.018). Similarly, low muscle mass (SMMI < 11.33 kg/m²), when combined with malnutrition and high inflammation (low CALLY index), further exacerbated EOMCI risk (Pinteraction = 0.028).Discussion: The findings suggest that in T2DM patients, the interactions and overall effects of underweight, reduced muscle mass, abdominal obesity, malnutrition, and elevated inflammation are significantly associated with an increased risk of EOMCI. Integrated management of these factors is essential to mitigate EOMCI risk.
Keywords: mild cognitive impairment1, Type 2 diabetes mellitus2, sarcopenia3, malnutrition4, inflammation5, interaction6
Received: 19 Sep 2024; Accepted: 14 Mar 2025.
Copyright: © 2025 Lin, Zhai, Wu, An, Chen, Qiu, Li and Lin. This is an open-access article distributed under the terms of the Creative Commons Attribution License (CC BY). The use, distribution or reproduction in other forums is permitted, provided the original author(s) or licensor are credited and that the original publication in this journal is cited, in accordance with accepted academic practice. No use, distribution or reproduction is permitted which does not comply with these terms.
* Correspondence:
Jing-Na Lin, Tianjin Union Medical Centre, Nankai University, Tianjin, China
Disclaimer: All claims expressed in this article are solely those of the authors and do not necessarily represent those of their affiliated organizations, or those of the publisher, the editors and the reviewers. Any product that may be evaluated in this article or claim that may be made by its manufacturer is not guaranteed or endorsed by the publisher.
Research integrity at Frontiers
Learn more about the work of our research integrity team to safeguard the quality of each article we publish.