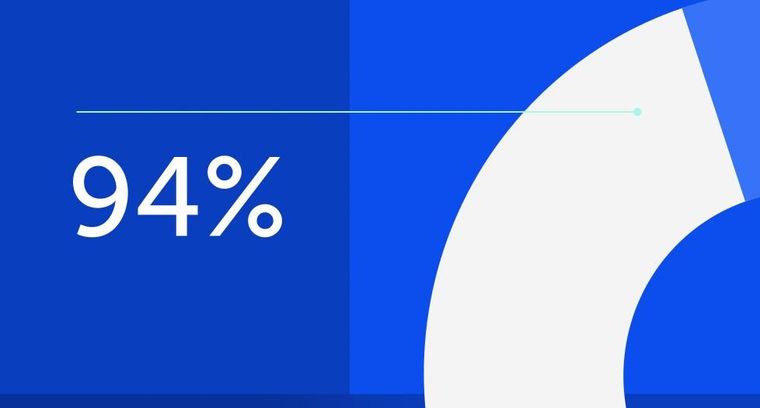
94% of researchers rate our articles as excellent or good
Learn more about the work of our research integrity team to safeguard the quality of each article we publish.
Find out more
SYSTEMATIC REVIEW article
Front. Aging Neurosci., 09 April 2025
Sec. Neurocognitive Aging and Behavior
Volume 17 - 2025 | https://doi.org/10.3389/fnagi.2025.1492800
This article is part of the Research TopicMild cognitive impairment and cognitive agingView all 5 articles
Objective: Mild cognitive impairment (MCI), a common state of cognitive impairment without significant impairment in daily functioning among older adults, is mainly identified using various neuropsychological tests, clinical interviews, and collateral history with some subjective interferences. This systematic review aimed to investigate the functional near-infrared spectroscopy (fNIRS) features of older adults with MCI compared with those with normal cognitive function to assist in the diagnosis of MCI.
Methods: A literature search was conducted in electronic databases, including PubMed, Web of Science, Embase, and Cochrane Library, up to June 15, 2024. The data on article information (first author and year of publication), participant characteristics, task paradigms, regions of interest (ROIs), fNIRS device attributes, and results related to cerebral oxygenation and hemodynamics were extracted.
Results: Finally, 34 relevant studies were identified, involving 1033 patients with MCI and 1107 age-, sex-, and education-matched controls with normal cognitive function. We found that the studies frequently used working memory–related task paradigms and resting-state measurements. Also, the prefrontal cortex was a primary ROI, and the changes in oxygenated hemoglobin concentration were the most basic research attributes used to derive measures such as functional connectivity (FC), FC variability, slope, and other parameters. However, ROI activation levels differed inconsistently between patients with MCI and individuals with normal cognition across studies. In general, the activation levels in the ROI of MCI patients may be higher than, lower than, or comparable to those in the normal control group.
Conclusion: Research on fNIRS in elderly patients with MCI aims to provide an objective marker for MCI diagnosis. The current findings are mixed. However, these differences can be partly explained with the theoretical support from the interaction of cognitive load theory and scaffolding theory of aging and cognition, taking into account factors such as unspecified MCI subtypes, task difficulty, task design, monitoring duration, and population characteristics. Therefore, future studies should consider definite MCI subtypes, strict and well-designed paradigms, long-term monitoring, and large sample sizes to obtain the most consistent results, thereby providing objective references for the clinical diagnosis of MCI in elderly patients.
The healthy aging process usually causes cognitive changes in healthy older adults; also, the cognitive decline in patients with mild cognitive impairment (MCI) is more severe than expected for their age (Tuokko and Mcdowell, 2006). Generally, MCI is defined as an intermediate state of cognitive impairment between healthy aging and dementia (Albert et al., 2011). Epidemiological studies show that MCI is highly prevalent among individuals aged more than 65 years, affecting about 10%–20% of this population (Di Carlo et al., 2007). Additionally, according to Mayo MCI criteria: (i) memory complaint, preferably corroborated by an informant, (ii) objective memory impairment for age, (iii) relatively preserved general cognition for age, (iv) essentially intact activities of daily living, and (v) not demented. about 12% of patients with MCI progress to Alzheimer’s disease (AD) annually (Petersen, 2004). And Petersen (2004) proposed the amnestic MCI criterion: (i) Memory complaint usually corroborated by an informant, (ii) Objective memory impairment for age, (iii) Essentially preserved general cognitive function largely intact functional activities, (iv) Not demented. In Diagnostic and Statistical Manual of Mental Disorders, Fifth Edition, memory impairment was replaced by multiple cognitive impairment categories (complex attention, executive function, learning and memory, language, perception-action or social cognition). Early identification and intervention for elderly individuals in the prodromal stage of AD are crucial prophylactic–therapeutic measures. At present, the diagnosis of MCI primarily relies on neuropsychological tests, clinical interviews, and collateral history. But because of wide variation in neuropsychological tests reported specificity and sensitivity (De Roeck et al., 2019). And these tests are highly influenced by motivation and attention (Brown, 2015). We are exploring methods to monitor brain function to assist in the diagnosis of MCI.
Brain function tests can be generally classified into two categories: electrophysiological examinations (electroencephalogram and magnetoencephalogram) and blood oxygen examinations [positron emission computed tomography, functional magnetic resonance imaging, and functional near-infrared spectroscopy (fNIRS)]. Among these, fNIRS is a noninvasive neuroimaging technology with a unique anti-interference capability, allowing it to assess brain function levels even during speech or movement by measuring changes in the concentrations of local oxygenated and deoxygenated hemoglobin (HbO2 and HbR) (Scholkmann et al., 2014). The local oxygen metabolism rate and cerebral blood flow rate increase when the cerebral cortex is active to provide the energy required for neuronal activity (Scholkmann et al., 2014). This is defined as functional brain congestion and neurovascular coupling (Nippert et al., 2018). Finally, in the active brain regions, HbO2 levels ultimately increase, whereas HbR levels decrease because of the increase in the concentration of local oxygenated blood. In addition, fNIRS has the following advantages: portable, low cost, convenient operation and low demand for the subjects. Thus, fNIRS is suitable for assessing the brain function in elderly patients with MCI.
Compared to healthy younger populations, current fNIRS studies in cognitively healthy older adults reveal the following characteristics: (1) Compensatory overactivation in the prefrontal cortex (Heinzel et al., 2013); (2) Reduced resting-state functional connectivity (Yeung and Chan, 2020); (3) Delayed hemodynamic responses (Schroeter et al., 2002). These changes reflect adaptive neurovascular remodeling during aging. In contrast, individuals with dementia exhibit generally suppressed hemodynamic responses on fNIRS, including more pronounced reductions in resting-state connectivity and hypoactivation (Butters et al., 2023). However, findings in MCI patients remain inconsistent, potentially demonstrating both hypoactivation and compensatory hyperactivation patterns (Butters et al., 2023). In this systematic review, we discussed the fNIRS features of older adults with MCI compared with those with normal cognitive function, aiming to provide a new marker for assessing cognitive function in these patients.
The guidelines from the Preferred Reporting Items for Systematic Reviews and Meta-analysis statements were referenced in this study (Page et al., 2021).
The literature search was conducted in electronic databases, including PubMed, Web of Science, Embase, and Cochrane Library, up to June 15, 2024. The following two groups of keywords were used: (1) mild cognitive impairment, mild cognitive dysfunction, mild cognitive disorder, MCI, and (2) fNIRS, functional near-infrared spectroscopy, near-infrared spectroscopy, and NIRS. The terms related to keywords and medical subject headings were used in this search. Also, relevant professional journals and reference lists of publications were searched when necessary. The search algorithm for each database is provided in the Supplementary material. Only articles with full-text availability in English were included.
The PICO framework was used to formulate the inclusion criteria, which were as follows: (1) Population (P): older adult participants with MCI (aged more than 60 years; considered a pre-dementia or pre-AD stage; objective evidence of cognitive impairment came from standardized neuropsychological tests; no significant impairment in daily life function; cognitive impairment does not only occur in delirium situations and cannot be better explained by another mental illness; no other neurological disorders; amnestic MCI and non-amnestic MCI included according to Petersen’s criteria.); (2) Intervention (I): various task paradigms combined with fNIRS; (3) Comparison (C): age-, sex-, and education-matched healthy population with normal cognitive function; and (4) Outcome (O): studies used fNIRS to quantify changes in hemoglobin concentration in brain regions of interest (ROIs).
Duplicate studies were removed using Endnote X9 (Clarivate Analytics, MA, USA), and unrecognized repetitions were manually screened (DX and QXF). Then, the titles, abstracts, and full texts of the remaining studies were screened by two independent reviewers (GL and LYJ) to determine eligibility. The exclusion criteria were as follows: cognitive impairment consistent with dementia; MCI due to other causes, such as trauma and stroke; and no monitoring of the cerebral hemodynamic response during tasks. Conference proceedings, review articles, and case reports were also excluded. Any disagreements on eligibility were resolved through discussion with a third reviewer (YNW).
Three reviewers (YB, DX, and QXF) completed the data extraction task independently using a preliminarily developed extraction sheet. The following data were retrieved from included studies: (1) article information: first author and year of publication; (2) participant characteristics: sample size, age, sex, educational level, and cognition level; (3) task paradigm; (4) ROI; (5) fNIRS device attributes: manufacturer, distance from the transmitter to the detector, wavelength, and sampling frequency; (6) results related to cerebral oxygenation and hemodynamics. For incomplete or unclear data, the corresponding author of the study was contacted by email. The two researchers (GL and LYJ) compared the extracted data for accuracy, and any discrepancies were resolved through discussion with a third reviewer (YNW).
The quality of the included studies was assessed independently by two reviewers (LYJ and GL) using the Newcastle–Ottawa Scale (NOS) (Stang, 2010). The NOS consists of three domains: selection of study subjects, comparability, and outcome measurement, with eight items. The evaluation was based on a scoring system, with a maximum of nine points. The higher scores suggested a higher quality of the study. The disagreements about the quality were also resolved through discussion with the third independent reviewer (YNW). The results of this assessment are provided in the Table 1.
Due to the heterogeneity of the clinical populations, methods used, and data presented, meta-analysis was not possible. The results were synthesized into a brief narrative review, focusing on the differences in various fNIRS findings between elderly patients with MCI and those with normal cognitive function.
We identified 445 records: PubMed (111), Embase (180), Web of Science (71), and Cochrane Library (83). A total of 261 duplicates were excluded, and 184 records were evaluated by titles and abstracts. Then, 13 studies were excluded, including 58 non-relevant studies, 40 trial registry records, 20 reviews, and 19 conference abstracts. Further, we reviewed the full texts of the remaining 47 studies. We found three studies on MCI caused by other diseases (tumor and chronic obstructive pulmonary disease) and five focusing on MCI in young adults, early AD, and subjective memory impairment, which were unsuitable for inclusion in the study. In addition, four non-control studies and one study without specific data were excluded. No additional articles were included using other methods or by scanning reference lists of selected articles. Finally, 34 studies investigated the fNIRS differences between older adults and patients with MCI were included. A flow chart presenting the selection of included records is displayed in Figure 1.
All included studies were non-randomized controlled trials involving 1033 elderly patients with MCI (recorded male/female: 450/511; amnestic MCI 288 in studies documenting subtypes of MCI) and 1107 age-, sex-, and education-matched normal cognitive old individuals. The sample size of MCI varied from 8 to 98 cases, and the average age of the study population ranged from 61.58 to 78.1 years. The cognitive function scales used in the studies included Mini-Mental State Examination (average score 21.4–28.9), Montreal Cognitive Assessment (average score 17.3–27.0), and Clinical Dementia Rating (average score 0.3–0.5). A few studies used the Auditory Verbal Learning Test and Hasegama’s Dementia Scale-Revised (HDS-R). The scores of all research participants met the MCI criteria. Further details on article information, fNIRS device attributes, quality assessment, and qualitative synthesis are shown in Table 2.
Task paradigms are standard experimental procedures used for the classical experimental tasks in the study of cognitive psychology. Seven of the included studies adopted the N-back task (Nguyen et al., 2019; Baik et al., 2022; Talamonti et al., 2022; Yeung et al., 2016a; Yang et al., 2019; Yoo and Hong, 2019; Vermeij et al., 2017), a typical working memory task (WMT) paradigm. Other memory function–related task paradigms included Delayed Match to Sample Task, Digit Span Test, Visuospatial Working Memory Task, and Modified Serial Number Task (Khan et al., 2022; Kim et al., 2021; Li R. et al., 2018; Li et al., 2020; Liu et al., 2023; Nakamura et al., 2021; Ung et al., 2020; Yu et al., 2020). Moreover, tasks assessing thinking flexibility and planning, such as Shiritori and category fluency tasks and verbal fluency tasks(VFT), were also included (Kato et al., 2017; Katzorke et al., 2018; Baik et al., 2022; Takahashi et al., 2022; Yang et al., 2019; Yang et al., 2020; Yap et al., 2017; Yoon et al., 2019; Yeung et al., 2016b). Resting state, defined as subjects being awake, with eyes closed and relaxed, was used in 10 studies (Bu et al., 2019; Canario et al., 2022; Ghafoor et al., 2019; Ho et al., 2022; Li X. et al., 2018; Niu et al., 2019; Baik et al., 2022; Yang and Hong, 2021; Zeller et al., 2019; Zhang et al., 2022). The Stroop task, an attention-related task, was also chosen (Baik et al., 2022; Yang et al., 2019; Yoon et al., 2019; Zhang et al., 2023). Furthermore, three studies involved dual-task paradigms: finger tapping with category VFT, talking with the 2-back task, and balance control with sequential subtraction (Takahashi et al., 2022; Talamonti et al., 2022; Xu et al., 2024). Some studies also incorporated graded task difficulty and a combination of multiple tasks (Yeung et al., 2016a; Yang et al., 2019; Vermeij et al., 2017; Ung et al., 2020).
The frontal skull’s thinness and lack of hair interference facilitate fNIRS detection. Besides, the frontal cortex’s involvement in movement, cognition, and behavior regulation makes it a key area of focus. Therefore, the cerebral blood flow and cerebral oxygenation signals in the prefrontal cortex (PFC) were detected in all included studies. Furthermore, the detectors used in some studies covered multiple brain regions simultaneously to detect connections between brain regions, including functional connectivity (FC) and effective connectivity (EC).
This systematic review revealed that fNIRS devices from different manufacturers were used in various studies. The number of channels varied from 6 to 204, and the maximum sampling rate was 138 Hz (3.91–125 Hz). Furthermore, the near-infrared light wavelengths detected in the studies varied from 600 to 900 nm.
Functional near-infrared spectroscopy mainly reflects the changes in hemoglobin concentration in the ROI. Therefore, the changes in the concentration of oxygenated hemoglobin (△oxyHb) were the most commonly studied parameters in almost all included studies. Also, some studies analyzed the FC of ROIs (Bu et al., 2019; Canario et al., 2022; Ghafoor et al., 2019; Li et al., 2020; Nguyen et al., 2019; Yang and Hong, 2021; Yu et al., 2020; Zhang et al., 2022). The slope of △oxyHb was also a significant parameter (Ung et al., 2020; Li R. et al., 2018). Niu et al. (2019) selected dynamic FC variability as the research parameter, which was defined as the area under the curve of the power spectrum across the low-frequency (<0.1-Hz) band. Moreover, one study analyzed the multiscale entropy (MSE) of △oxyHb (Li X. et al., 2018). Lateralization, the asymmetric activation of brain regions, was also observed in some studies (Haberstumpf et al., 2022; Yeung et al., 2016b).
The change in oxyHb concentration during the task can reflect the activation level of ROIs. In this review, 10 studies suggested that the cortical △oxyHb of patients with MCI was reduced compared with that of the controls during the task (Haberstumpf et al., 2022; Kato et al., 2017; Khan et al., 2022; Liu et al., 2023; Baik et al., 2022; Yang et al., 2019; Yang et al., 2020; Yeung et al., 2016a; Yoo and Hong, 2019; Zhang et al., 2023), it was the most significant in the PFC. The PFC activation of patients with MCI was normal under a low WMT load, which showed decreased activation with an increase in the WMT load (Yeung et al., 2016a). Patients with MCI having lower cognitive scores were more likely to show reduced PFC activation (Kato et al., 2017). Baik et al. (2022) found that △oxyHb in the PFC was reduced in the semantic VFT but not in the N-back task or resting state. In addition, a study compared the bilateral PFC activation of patients with MCI and found that the activation reduction of the left PFC was more significant (Yang et al., 2019). Moreover, the EC and FC in patients with MCI was also reduced (Bu et al., 2019; Ghafoor et al., 2019; Yang and Hong, 2021; Zhang et al., 2022). The slope of △oxyHb decreased in patients with MCI, indicating reduced activation of the PFC (Li R. et al., 2018).
However, some other studies reported opposite results, demonstrating higher PFC activation in patients with MCI than in controls (Kim et al., 2021; Li et al., 2020; Talamonti et al., 2022; Ung et al., 2020; Vermeij et al., 2017; Xu et al., 2024; Yu et al., 2020). The slope of FPC △oxyHb in patients with MCI increased more significantly under a higher WMT load than a lower WMT load (Ung et al., 2020). However, a higher WMT load did not cause an increase in FPC △oxyHb compared with a lower WMT load (Vermeij et al., 2017). Talamonti et al. (2022) also suggested that △oxyHb increased during single-task walking but not during dual-task walking.
Furthermore, nine studies did not obtain a difference in △oxyHb and FC between patients with MCI and healthy controls (Canario et al., 2022; Ho et al., 2022; Li R. et al., 2018; Nguyen et al., 2019; Takahashi et al., 2022; Yap et al., 2017; Yeung et al., 2016b; Yoon et al., 2019; Zeller et al., 2019). Some studies selected other research fNIRS parameters. Li X. et al. (2018) found no difference in the MSE of △oxyHb between patients with MCI and healthy controls. Niu et al. (2019) suggested that the static FC did not change, but dynamic FC variability increased in MCI. Besides, the △oxyHb of the left PFC in patients with MCI did not increase in the same manner as in a healthy population, which was defined as frontal lateralization (Haberstumpf et al., 2022; Yeung et al., 2016b).
This systematic review explored the differences in fNIRS between elderly patients with MCI and healthy controls, revealing mixed results. Some studies suggested that cortical activation was reduced in MCI, while others reported opposite results. Additionally, some studies reached neutral conclusions.
The aforementioned results were mixed, which might have been caused by the heterogeneity of the MCI group and the lack of standardization in methods (Stern et al., 2012; Butters et al., 2023). MCI is difficult to diagnose and classify into subtypes (Díaz-Mardomingo et al., 2017), leading to inevitable heterogeneity. The difference in task types, ROIs, and monitoring time make it difficult to standardize methods. According to cognitive load theory and scaffolding theory of aging and cognition, the PFC can perform compensatory activation to offset cognitive deterioration in MCI (Park and Bischof, 2013; Merlo et al., 2019). This theory might partially explain the differences in ROI activation under the aforementioned heterogeneous conditions. This compensation did not occur at low WMT load or during the resting state, which is why no differences in ROI activation were noted between patients with MCI and healthy population at that time (Baik et al., 2022; Ung et al., 2020; Yeung et al., 2016a). When the WMT load appropriately increases, compensation activates in patients with MCI but not in healthy subjects (Ung et al., 2020). However, such compensation is limited. Therefore, as the WMT load further increases, patients with MCI show reduced activation of ROIs (Baik et al., 2022; Yeung et al., 2016a). Besides, we speculate that this limitation might be common in aging elderly subjects during excessive WMT load (Ren et al., 2012; Yap et al., 2017; Heinzel et al., 2014). At this point, the activation inertness of ROIs between the MCI group and the control group might become similar (Talamonti et al., 2022; Vermeij et al., 2017). The FC between PFC and other brain regions was reduced in MCI (Farràs-Permanyer et al., 2015; Cohen et al., 2014). However, the FC within the PFC was activated through a compensatory mechanism (Qi et al., 2010; Liang et al., 2014). In the included studies, we found that FC was mostly reduced in the resting state (Bu et al., 2019; Ghafoor et al., 2019; Yang and Hong, 2021; Zhang et al., 2022) and increased during tasks (Li et al., 2020; Yu et al., 2020). We speculated that this might be related to more compensatory function assistance between brain regions in task states. Moreover, the neural compensatory mechanisms may involve upregulation in excitatory synapses, synaptic and metabolic activity, enhancement of neurotrophic milieu, and changes in glial cell reactivity and inflammation (Merlo et al., 2019). This enhanced synaptic activity thus manifests in the form of hyperactivation in the affected region (Merlo et al., 2019). However, such neural compensation may not be timely. Patients with MCI may require increased oxygen consumption due to functional impairments. This leads to a greater reduction in oxyHb shortly after the cognitive task onset, followed by the delayed oxyHb influx. This might explain the decrease in △oxyHb in some studies, which might be due to the short fNIRS monitoring time. In addition, the cognitive task selectively activated the left PFC, leading to the difference in △oxyHb in bilateral PFC (Arai et al., 2006).
Although these differences may be partly attributed to the lens of cognitive load theory and the scaffolding theory of aging and cognition, we cannot ignore the limitations of current fNIRS studies in MCI. Since most current studies do not specify MCI subtypes based on Petersen and/or DSM criteria, we are unable to provide a more detailed interpretation of the results according to subtype classifications. Furthermore, these limitations encompass the lack of standardization in task difficulty, task types, and monitoring duration for different patients with MCI.
Progressive studies on fNIRS in elderly patients with MCI have been conducted using various task paradigms, evaluation parameters, and ROIs to provide an objective marker for MCI diagnosis. However, the current research findings are inconsistent. To obtain consistent, accurate, and reliable evidence for the application of fNIRS in MCI, future studies should clearly characterize the type of MCI the participants have according to Petersen and/or DSM criteria. And representative ROIs and suitable task paradigms for various patients with MCI need be aimed. Additionally, the duration of fNIRS monitoring should be further explored. Larger sample sizes and more appropriate research indicators are also essential. Prospective studies should be encouraged to evaluate the potential prognostic role of fNIRS. Furthermore, comparing NIRS findings with objective measurements (e.g., AD biomarkers) would allow for the estimation of sensitivity and specificity of the method for dementia diagnosis.
The original contributions presented in this study are included in this article/Supplementary material, further inquiries can be directed to the corresponding author.
BY: Data curation, Writing – original draft, Investigation. XD: Data curation, Investigation, Writing – review and editing. XQ: Data curation, Investigation, Writing – review and editing. YL: Methodology, Software, Visualization, Writing – original draft. LG: Methodology, Software, Validation, Writing – original draft. NY: Conceptualization, Writing – review and editing.
The author(s) declare that financial support was received for the research and/or publication of this article. This work was supported by the Youth Talent Fund 2023(2023QN19) of Sichuan Academy of Medical Sciences, Sichuan Provincial People’s Hospital.
The authors declare that the research was conducted in the absence of any commercial or financial relationships that could be construed as a potential conflict of interest.
The reviewer JL declared a shared affiliation with the authors BY, XD, XQ, YL, NY to the handling editor at the time of review.
All claims expressed in this article are solely those of the authors and do not necessarily represent those of their affiliated organizations, or those of the publisher, the editors and the reviewers. Any product that may be evaluated in this article, or claim that may be made by its manufacturer, is not guaranteed or endorsed by the publisher.
The Supplementary Material for this article can be found online at: https://www.frontiersin.org/articles/10.3389/fnagi.2025.1492800/full#supplementary-material
Ahn, H. J., Chin, J., Park, A., Lee, B. H., Suh, M. K., Seo, S. W., et al. (2010). Seoul neuropsychological screening battery-dementia version (SNSB-D): A useful tool for assessing and monitoring cognitive impairments in dementia patients. J. Korean Med. Sci. 25, 1071–1076. doi: 10.3346/jkms.2010.25.7.1071
Albert, M. S., DeKosky, S. T., Dickson, D., Dubois, B., Feldman, H. H., Fox, N. C., et al. (2011). The diagnosis of mild cognitive impairment due to Alzheimer’s disease: Recommendations from the National Institute on Aging-Alzheimer’s Association workgroups on diagnostic guidelines for Alzheimer’s disease. Alzheimers Dement. 7, 270–279. doi: 10.1016/j.jalz.2011.03.008
American Psychiatric Association [APA], (2013). “Neurocognitive disorders,” in Diagnostic and Statistical Manual of Mental Disorders, 5th Edn, (Arlington, VA: American Psychiatric Association), 591–643.
Arai, H., Takano, M., Miyakawa, K., Ota, T., Takahashi, T., Asaka, H., et al. (2006). A quantitative near-infrared spectroscopy study: A decrease in cerebral hemoglobin oxygenation in Alzheimer’s disease and mild cognitive impairment. Brain Cogn. 61, 189–194. doi: 10.1016/j.bandc.2005.12.012
Baik, J. S., Ko, M. H., Ko, S. H., Min, J. H., Choi, J. K., Baek, J. Y., et al. (2022). Assessment of functional near-infrared spectroscopy by comparing prefrontal cortex activity: A cognitive impairment screening tool. Alzheimer Dis. Associat. Disord. 36, 266–268. doi: 10.1097/WAD.0000000000000475
Brown, J. (2015). The use and misuse of short cognitive tests in the diagnosis of dementia. J. Neurol. Neurosurg. Psychiatry 86, 680–685. doi: 10.1136/jnnp-2014-309086
Bu, L., Huo, C., Qin, Y., Xu, G., Wang, Y., and Li, Z. (2019). Effective connectivity in subjects with mild cognitive impairment as assessed using functional near-infrared spectroscopy. Am. J. Phys. Med. Rehabil. 98, 438–445. doi: 10.1097/PHM.0000000000001118
Butters, E., Srinivasan, S., O’Brien, J. T., Su, L., and Bale, G. (2023). A promising tool to explore functional impairment in neurodegeneration: A systematic review of near-infrared spectroscopy in dementia. Ageing Res. Rev. 90:101992. doi: 10.1016/j.arr.2023.101992
Canario, E., Chen, D., Han, Y., Niu, H., and Biswal, B. (2022). Global network analysis of Alzheimer’s disease with minimum spanning trees. J. Alzheimers Dis. 89, 571–581. doi: 10.3233/JAD-215573
Cohen, M. S., Rissman, J., Suthana, N. A., Castel, A. D., and Knowlton, B. J. (2014). Value-based modulation of memory encoding involves strategic engagement of fronto-temporal semantic processing regions. Cognit. Affect. Behav. Neurosci. 14, 578–592. doi: 10.3758/s13415-014-0275-x
Cummings, J. L., Dubois, B., Molinuevo, J. L., and Scheltens, P. (2013). International work group criteria for the diagnosis of Alzheimer disease. Med. Clin. 97, 363–368. doi: 10.1016/j.mcna.2013.01.001
De Roeck, E. E., De Deyn, P. P., Dierckx, E., and Engelborghs, S. (2019). Brief cognitive screening instruments for early detection of Alzheimer’s disease: A systematic review. Alzheimers Res. Ther. 11:21. doi: 10.1186/s13195-019-0474-3
Di Carlo, A., Lamassa, M., Baldereschi, M., Inzitari, M., Scafato, E., Farchi, G., et al. (2007). CIND and MCI in the Italian elderly: Frequency, vascular risk factors, progression to dementia. Neurology 68, 1909–1916. doi: 10.1212/01.wnl.0000263132.99055.0d
Díaz-Mardomingo, M. D. C., García-Herranz, S., Rodríguez-Fernández, R., Venero, C., and Peraita, H. (2017). Problems in classifying mild cognitive impairment (MCI): One or multiple syndromes? Brain Sci. 7:111. doi: 10.3390/brainsci7090111
Farràs-Permanyer, L., Guàrdia-Olmos, J., and Peró-Cebollero, M. (2015). Mild cognitive impairment and fMRI studies of brain functional connectivity: The state of the art. Front. Psychol. 6:1095. doi: 10.3389/fpsyg.2015.01095
Ghafoor, U., Lee, J. H., Hong, K. S., Park, S. S., Kim, J., and Yoo, H. R. (2019). Effects of acupuncture therapy on MCI patients using functional near-infrared spectroscopy. Front. Aging Neurosci. 11:237. doi: 10.3389/fnagi.2019.00237
Haberstumpf, S., Seidel, A., Lauer, M., Polak, T., Deckert, J., and Herrmann, M. J. (2022). Reduced parietal activation in participants with mild cognitive impairments during visual-spatial processing measured with functional near-infrared spectroscopy. J. Psychiatr. Res. 146, 31–42. doi: 10.1016/j.jpsychires.2021.12.021
Heinzel, S., Lorenz, R. C., Brockhaus, W. R., Wüstenberg, T., Kathmann, N., and Heinz, A. (2014). Working memory load-dependent brain response predicts behavioral training gains in older adults. J. Neurosci. 34, 1224–1233. doi: 10.1523/JNEUROSCI.2463-13.2014
Heinzel, S., Metzger, F. G., Ehlis, A. C., Korell, R., Alboji, A., Haeussinger, F. B., et al. (2013). Aging-related cortical reorganization of verbal fluency processing: A functional near-infrared spectroscopy study. Neurobiol. Aging 34, 439–450. doi: 10.1016/j.neurobiolaging.2012.05.021
Ho, T. K. K., Kim, M., Jeon, Y., Kim, B. C., Kim, J. G., Lee, K. H., et al. (2022). Deep learning-based multilevel classification of Alzheimer’s disease using non-invasive functional near-infrared spectroscopy. Front. Aging Neurosci. 14:810125. doi: 10.3389/fnagi.2022.810125
Jack, C. R. Jr., Albert, M. S., Knopman, D. S., McKhann, G. M., Sperling, R. A., Carrillo, M. C., et al. (2011). Introduction to the recommendations from the national institute on aging-Alzheimer’s association workgroups on diagnostic guidelines for Alzheimer’s disease. Alzheimers Dement. 7, 257–262.
Kato, Y., Shoji, Y., Morita, K., Inoue, M., Ishii, Y., Sato, M., et al. (2017). Evaluation of changes in oxyhemoglobin during Shiritori task in elderly subjects including those with Alzheimer’s disease. Psychogeriatrics 17, 238–246. doi: 10.1111/psyg.12226
Katzorke, A., Zeller, J. B. M., Müller, L. D., Lauer, M., Polak, T., Deckert, J., et al. (2018). Decreased hemodynamic response in inferior frontotemporal regions in elderly with mild cognitive impairment. Psychiatry Res. Neuroimaging 274, 11–18. doi: 10.1016/j.pscychresns.2018.02.003
Khan, M. N. A., Ghafoor, U., Yoo, H. R., and Hong, K. S. (2022). Acupuncture enhances brain function in patients with mild cognitive impairment: Evidence from a functional-near infrared spectroscopy study. Neural Regen. Res. 17, 1850–1856. doi: 10.4103/1673-5374.332150
Kim, E., Yu, J. W., Kim, B., Lim, S. H., Lee, S. H., Kim, K., et al. (2021). Refined prefrontal working memory network as a neuromarker for Alzheimer’s disease. Biomed. Opt. Express 12, 7199–7222. doi: 10.1364/BOE.438926
Li, R., Rui, G., Chen, W., Li, S., Schulz, P. E., and Zhang, Y. (2018). Early detection of Alzheimer’s disease using non-invasive near-infrared spectroscopy. Front. Aging Neurosci. 10:366. doi: 10.3389/fnagi.2018.00366
Li, R., Rui, G., Zhao, C., Wang, C., Fang, F., and Zhang, Y. (2020). Functional network alterations in patients with amnestic mild cognitive impairment characterized using functional near-infrared spectroscopy. IEEE Trans. Neural Syst. Rehabil. Eng. 28, 123–132. doi: 10.1109/TNSRE.2019.2956464
Li, X., Zhu, Z., Zhao, W., Sun, Y., Wen, D., Xie, Y., et al. (2018). Decreased resting-state brain signal complexity in patients with mild cognitive impairment and Alzheimer’s disease: A multiscale entropy analysis. Biomed. Opt. Express 9, 1916–1929. doi: 10.1364/BOE.9.001916
Liang, P., Li, Z., Deshpande, G., Wang, Z., Hu, X., and Li, K. (2014). Altered causal connectivity of resting state brain networks in amnesic MCI. PLoS One 9:e88476. doi: 10.1371/journal.pone.0088476
Liu, Y., Zeng, Z., Huang, S., Shang, P., Lv, Z., Wang, Y., et al. (2023). Brain activation during working memory task in amnestic mild cognitive impairment patients and its association with memory and attention. J. Alzheimers Dis. 91, 863–875. doi: 10.3233/JAD-220815
McKhann, G., Drachman, D., Folstein, M., Katzman, R., Price, D., and Stadlan, E. M. (1984). Clinical diagnosis of Alzheimer’s disease: Report of the NINCDSADRDA work group* under the auspices of department of health and human services task force on Alzheimer’s disease. Neurology 34, 939–939. doi: 10.1212/wnl.34.7.939
Merlo, S., Spampinato, S. F., and Sortino, M. A. (2019). Early compensatory responses against neuronal injury: A new therapeutic window of opportunity for Alzheimer’s Disease? CNS Neurosci. Therapeut. 25, 5–13. doi: 10.1111/cns.13050
Nakamura, S., Yomota, S., Ito, H., Akinaga, N., Hori, A., Chinomi, K., et al. (2021). A novel cognitive function scale using functional near-infrared spectroscopy for evaluating cognitive dysfunction. J. Alzheimers Dis. 81, 1579–1588. doi: 10.3233/JAD-210072
Nguyen, T., Kim, M., Gwak, J., Lee, J. J., Choi, K. Y., Lee, K. H., et al. (2019). Investigation of brain functional connectivity in patients with mild cognitive impairment: A functional near-infrared spectroscopy (fNIRS) study. J. Biophotonics 12:e201800298. doi: 10.1002/jbio.201800298
Nippert, A. R., Biesecker, K. R., and Newman, E. A. (2018). Mechanisms mediating functional hyperemia in the brain. Neuroscientist 24, 73–83. doi: 10.1177/1073858417703033
Niu, H., Zhu, Z., Wang, M., Li, X., Yuan, Z., Sun, Y., et al. (2019). Abnormal dynamic functional connectivity and brain states in Alzheimer’s diseases: Functional near-infrared spectroscopy study. Neurophotonics 6:025010. doi: 10.1117/1.NPh.6.2.025010
Page, M. J., McKenzie, J. E., Bossuyt, P. M., Boutron, I., Hoffmann, T. C., Mulrow, C. D., et al. (2021). The PRISMA 2020 statement: An updated guideline for reporting systematic reviews. BMJ 372:n71. doi: 10.1136/bmj.n71
Park, D. C., and Bischof, G. N. (2013). The aging mind: Neuroplasticity in response to cognitive training. Dial. Clin. Neurosci. 15, 109–119. doi: 10.31887/DCNS.2013.15.1/dpark
Petersen, R. C. (2004). Mild cognitive impairment as a diagnostic entity. J. Internal Med. 256, 183–194. doi: 10.1111/j.1365-2796.2004.01388.x
Portet, F., Ousset, P. J., Visser, P. J., Frisoni, G. B., Nobili, F., Scheltens, P., et al. (2006). Mild cognitive impairment (MCI) in medical practice: A critical review of the concept and new diagnostic procedure. Report of the MCI Working Group of the European Consortium on Alzheimer’s Disease. J. Neurol. Neurosurg. Psychiatry 77, 714–718. doi: 10.1136/jnnp.2005.085332
Qi, Z., Wu, X., Wang, Z., Zhang, N., Dong, H., Yao, L., et al. (2010). Impairment and compensation coexist in amnestic MCI default mode network. NeuroImage 50, 48–55. doi: 10.1016/j.neuroimage.2009.12.025
Ren, T., Ho, R. C., and Mak, A. (2012). Dysfunctional cortico-basal ganglia-thalamic circuit and altered hippocampal-amygdala activity on cognitive set-shifting in non-neuropsychiatric systemic lupus erythematosus. Arthritis Rheum. 64, 4048–4059. doi: 10.1002/art.34660
Scholkmann, F., Kleiser, S., Metz, A. J., Zimmermann, R., Mata Pavia, J., Wolf, U., et al. (2014). A review on continuous wave functional near-infrared spectroscopy and imaging instrumentation and methodology. NeuroImage 85(Pt 1), 6–27. doi: 10.1016/j.neuroimage.2013.05.004
Schroeter, M. L., Zysset, S., Kupka, T., Kruggel, F., and Yves von Cramon, D. (2002). Near-infrared spectroscopy can detect brain activity during a color-word matching Stroop task in an event-related design. Hum. Brain Mapp. 17, 61–71. doi: 10.1002/hbm.10052
Stang, A. (2010). Critical evaluation of the Newcastle-Ottawa scale for the assessment of the quality of nonrandomized studies in meta-analyses. Eur. J. Epidemiol. 25, 603–605. doi: 10.1007/s10654-010-9491-z
Stern, Y., Rakitin, B. C., Habeck, C., Gazes, Y., Steffener, J., Kumar, A., et al. (2012). Task difficulty modulates young-old differences in network expression. Brain Res. 1435, 130–145. doi: 10.1016/j.brainres.2011.11.061
Takahashi, S., Tomita, Y., Tanaka, S., Sakurai, N., and Kodama, N. (2022). Prefrontal cerebral oxygenated hemoglobin concentration during the category fluency and finger-tapping tasks in adults with and without mild cognitive impairment: A near-infrared spectroscopy study. Brain Sci. 12:1636. doi: 10.3390/brainsci12121636
Talamonti, D., Gagnon, C., Vincent, T., Nigam, A., Lesage, F., and Bherer, L. (2022). Exploring cognitive and brain oxygenation changes over a 1-year period in physically active individuals with mild cognitive impairment: A longitudinal fNIRS pilot study. BMC Geriatr. 22:648. doi: 10.1186/s12877-022-03306-x
Tuokko, H. A., and Mcdowell, I. (2006). An overview of mild cognitive impairment. N. Engl. J. Med. 356, 1175–1175. doi: 10.1056/NEJMcibr067658
Ung, W. C., Yap, K. H., Ebenezer, E. G. M., Chin, P. S., Nordin, N., Chan, S. C., et al. (2020). Assessing neural compensation with visuospatial working memory load using near-infrared imaging. IEEE Trans. Neural Syst. Rehabil. Eng. 28, 13–22. doi: 10.1109/TNSRE.2019.2956459
Vermeij, A., Kessels, R. P. C., Heskamp, L., Simons, E. M. F., Dautzenberg, P. L. J., and Claassen, J. A. H. R. (2017). Prefrontal activation may predict working-memory training gain in healthy aging and mild cognitive impairment. Brain Imaging Behav. 11, 141–154. doi: 10.1007/s11682-016-9508-7
Writing Group for Chinese Guidelines of Dementia and Cognitive Impairment Diagnoses and Treatments and Committee of Cognitive Disorders of Neurologist Branch of Chinese Medical Doctor Association (2018). 2018 guidelines for diagnoses and treatments of dementias and cognitive impairments in China (5): The diagnosis and treatment of mild cognitive impairment. Nat. Med. J. China 98, 1294–1301
Xu, G., Zhou, M., Chen, Y., Song, Q., Sun, W., and Wang, J. (2024). Brain activation during standing balance control in dual-task paradigm and its correlation among older adults with mild cognitive impairment: A fNIRS study. BMC Geriatr. 24:144. doi: 10.1186/s12877-024-04772-1
Yang, D., and Hong, K. S. (2021). Quantitative assessment of resting-state for mild cognitive impairment detection: A functional near-infrared spectroscopy and deep learning approach. J. Alzheimers Dis. 80, 647–663. doi: 10.3233/JAD-201163
Yang, D., Hong, K. S., Yoo, S. H., and Kim, C. S. (2019). Evaluation of neural degeneration biomarkers in the prefrontal cortex for early identification of patients with mild cognitive impairment: An fNIRS study. Front. Hum. Neurosci. 13:317. doi: 10.3389/fnhum.2019.00317
Yang, D., Huang, R., Yoo, S. H., Shin, M. J., Yoon, J. A., Shin, Y. I., et al. (2020). Detection of mild cognitive impairment using convolutional neural network: Temporal-feature maps of functional near-infrared spectroscopy. Front. Aging Neurosci. 12:141. doi: 10.3389/fnagi.2020.00141
Yap, K. H., Ung, W. C., Ebenezer, E. G. M., Nordin, N., Chin, P. S., Sugathan, S., et al. (2017). Visualizing hyperactivation in neurodegeneration based on prefrontal oxygenation: A comparative study of mild Alzheimer’s disease, mild cognitive impairment, and healthy controls. Front. Aging Neurosci. 9:287. doi: 10.3389/fnagi.2017.00287
Yeung, M. K., and Chan, A. S. (2020). Functional near-infrared spectroscopy reveals decreased resting oxygenation levels and task-related oxygenation changes in mild cognitive impairment and dementia: A systematic review. J. Psychiatr. Res. 124, 58–76. doi: 10.1016/j.jpsychires.2020.02.017
Yeung, M. K., Sze, S. L., Woo, J., Kwok, T., Shum, D. H., and Yu, R. (2016b). Altered frontal lateralization underlies the category fluency deficits in older adults with mild cognitive impairment: A near-infrared spectroscopy study. Front. Aging Neurosci. 8:59. doi: 10.3389/fnagi.2016.00059
Yeung, M. K., Sze, S. L., Woo, J., Kwok, T., Shum, D. H., Yu, R., et al. (2016a). Reduced frontal activations at high working memory load in mild cognitive impairment: Near-infrared spectroscopy. Dement. Geriatr. Cognit. Disord. 42, 278–296. doi: 10.1159/000450993
Yoo, S. H., and Hong, K. S. (2019). “Hemodynamics analysis of patients with mild cognitive impairment during working memory tasks,” in Proceedings of the Annual International Conference of the IEEE Engineering in Medicine and Biology Society. IEEE Engineering in Medicine and Biology Society, (Berlin: IEEE), 4470–4473. doi: 10.1109/EMBC.2019.8856956
Yoon, J. A., Kong, I. J., Choi, J., Baek, J. Y., Kim, E. J., Shin, Y. I., et al. (2019). Neural compensatory response during complex cognitive function tasks in mild cognitive impairment: A near-infrared spectroscopy study. Neural Plastic. 2019:7845104. doi: 10.1155/2019/7845104
Yu, J. W., Lim, S. H., Kim, B., Kim, E., Kim, K., Kyu Park, S., et al. (2020). Prefrontal functional connectivity analysis of cognitive decline for early diagnosis of mild cognitive impairment: A functional near-infrared spectroscopy study. Biomed. Opt. Express 11, 1725–1741. doi: 10.1364/BOE.382197
Zeller, J. B. M., Katzorke, A., Müller, L. D., Breunig, J., Haeussinger, F. B., Deckert, J., et al. (2019). Reduced spontaneous low frequency oscillations as measured with functional near-infrared spectroscopy in mild cognitive impairment. Brain Imaging Behav. 13, 283–292. doi: 10.1007/s11682-018-9827-y
Zhang, C., Yang, H., Fan, C. C., Chen, S., Fan, C., and Hou, Z. G. (2023). Comparing multi-dimensional fNIRS features using bayesian optimization-based neural networks for mild cognitive impairment (MCI) detection. IEEE Trans. Neural Syst. Rehabil. Eng. 31, 1019–1029. doi: 10.1109/TNSRE.2023.3236007
Keywords: cerebral hemodynamics, cerebral oxygenation, functional near-infrared spectroscopy, mild cognitive impairment, systematic review
Citation: Yang B, Deng X, Qu X, Li Y, Guo L and Yu N (2025) Identification of functional near-infrared spectroscopy for older adults with mild cognitive impairment: a systematic review. Front. Aging Neurosci. 17:1492800. doi: 10.3389/fnagi.2025.1492800
Received: 08 September 2024; Accepted: 13 March 2025;
Published: 09 April 2025.
Edited by:
Ion Beratis, American College of Greece, GreeceReviewed by:
Jing Lu, University of Electronic Science and Technology of China, ChinaCopyright © 2025 Yang, Deng, Qu, Li, Guo and Yu. This is an open-access article distributed under the terms of the Creative Commons Attribution License (CC BY). The use, distribution or reproduction in other forums is permitted, provided the original author(s) and the copyright owner(s) are credited and that the original publication in this journal is cited, in accordance with accepted academic practice. No use, distribution or reproduction is permitted which does not comply with these terms.
*Correspondence: Nengwei Yu, MTg5ODE4Mzg2NTJAMTI2LmNvbQ==
Disclaimer: All claims expressed in this article are solely those of the authors and do not necessarily represent those of their affiliated organizations, or those of the publisher, the editors and the reviewers. Any product that may be evaluated in this article or claim that may be made by its manufacturer is not guaranteed or endorsed by the publisher.
Research integrity at Frontiers
Learn more about the work of our research integrity team to safeguard the quality of each article we publish.