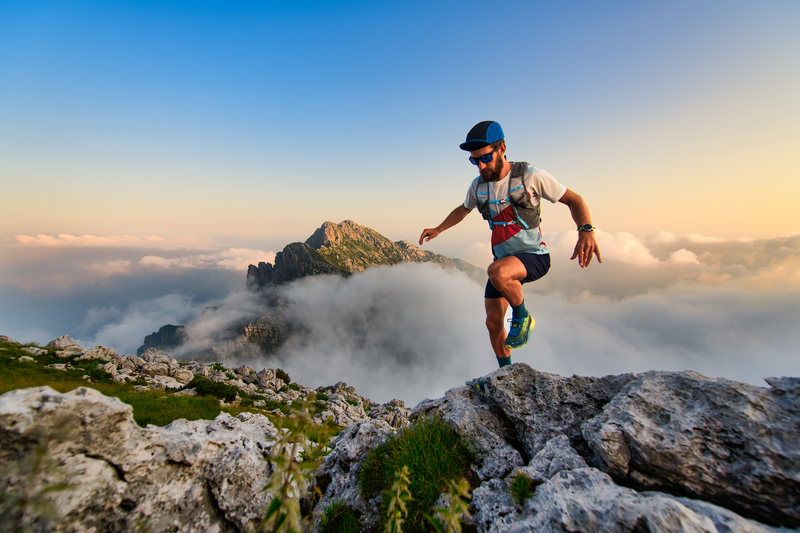
94% of researchers rate our articles as excellent or good
Learn more about the work of our research integrity team to safeguard the quality of each article we publish.
Find out more
SYSTEMATIC REVIEW article
Front. Aging Neurosci. , 15 January 2025
Sec. Alzheimer's Disease and Related Dementias
Volume 16 - 2024 | https://doi.org/10.3389/fnagi.2024.1524622
This article is part of the Research Topic Molecular mechanisms of neurodegeneration View all 5 articles
Mild cognitive impairment (MCI) is characterized by a decline in cognitive functioning without significant interference in daily activities. Its high heterogeneity and elevated conversion rate to dementia pose challenges for accurate diagnosis and monitoring, highlighting the urgent need to identify methodologies focused on the early detection and intervention of MCI. Due to their biological characteristics, microRNAs (miRNAs) are potential candidates as non-invasive molecular markers for the identification and assessment of MCI progression. Therefore, in this study, we conducted a meta-analysis to identify the miRNAs commonly deregulated in MCI, focusing on expression profiles in plasma, serum, and extracellular vesicle samples. Our analysis identified eight upregulated miRNAs, including hsa-miR-149-3p, and four downregulated miRNAs, such as Let-7f-5p. Notably, hsa-miR-149-3p emerged as a central node in interaction networks, suggesting its crucial role in regulating cellular processes relevant to MCI. Additionally, pathway analysis revealed significant enrichment in biological processes associated with transcriptional regulation and neurodegeneration. Our results underscore the potential of circulating miRNAs as non-invasive molecular markers for MCI and open the possibility for new methodologies that enable more accurate diagnosis and monitoring of disease progression. Validating the expression of miRNAs such as hsa-miR-149-3p and Let-7f-5p, along with identifying their functional role in the specific context of MCI, is essential to establish their biological relevance. This work contributes to the understanding of the miRNA profile in mild cognitive impairment using easily accessible samples, which could be useful for the development of various strategies aimed at preventing or delaying MCI in individuals at risk of developing dementia, including Alzheimer’s disease.
Mild Cognitive Impairment (MCI), currently termed as mild neurocognitive disorder by the Diagnostic and Statistical Manual of Mental Disorders-5 (DSM-5), is defined as a decline in cognitive functioning. MCI can be classified based on whether memory is impaired or not, as amnestic, and non-amnestic subtypes, respectively. Moreover, depending on the number of affected domains, MCI can be categorized as single or multiple domains. Other relevant characteristic is that MCI does not interfere in the daily activities (Sachs-Ericsson and Blazer, 2015). Although MCI is not a disease, it has garnered significant interest in recent years because it falls between normal aging and the early stages of dementia (Morris, 2005). It has been reported that the transition from MCI to dementia occurs at an annual rate of 12% in the general population, reaching up to 20% in higher-risk groups (Plassman et al., 2008; Petersen, 2006). This suggests that early identification and timely treatment of MCI may delay the onset of dementia. However, MCI is clinically heterogeneous, which has hindered efforts to identify and monitor it (Sabbagh et al., 2020).
The MCI identification is established considering previous cognitive functioning before memory complain onset, educational level, lifestyle, and ethnic differences (Kouzuki et al., 2022). Currently, the assessment of MCI relies on the sensitivity and specificity of cognitive and functional tests, such as the Mini-Mental Status Examination (MMSE), the Montreal Cognitive Assessment (MoCA), among others (Jia et al., 2021). However, despite their general utility, diagnostic methods for MCI are constantly evolving and not well standardized, which may lead to variations in results and a lack of consistency in the early and accurate diagnosis of MCI (Chen et al., 2021; Lee, 2023; López and Altuna, 2023).
Although molecular markers are not currently included in the diagnostic criteria (Dunne et al., 2021), their potential in identifying and assessing the risk of progression from MCI to dementia has captured the attention of medical research. Indeed, various biomarkers in cerebrospinal fluid (CSF), such as Aβ42 and tau protein, have been explored with the purpose of using them for differential diagnosis between MCI, Alzheimer’s disease (AD), and cognitively normal subjects (Dunne et al., 2021; Papaliagkas et al., 2023). However, assessing biomarkers in CSF involves an invasive procedure, thus there is an urgent need to identify more accessible and less invasive molecular markers present in peripheral fluids that allow for timely diagnosis of MCI (Álvarez-Sánchez et al., 2023; Hansson et al., 2010).
In this context, considerable attention has been directed toward microRNAs (miRNAs) as potential molecular markers for MCI. miRNAs are non-coding RNA molecules that play a crucial role in regulating gene expression (Ranganathan and Sivasankar, 2014; Ratti et al., 2020). These small molecules can repress specific genes by binding complementarily to the 3′ untranslated region (3’UTR) of mRNA, leading to either degradation or inhibition of translation (McNeill and Van Vactor, 2012). Interestingly, miRNAs have also been found to interact with other regions of mRNAs, including the 5’ UTR, the coding sequence, and gene promoters, where they can promote protein translation (Groth et al., 2007; Ørom et al., 2008). This versatility underscores their significance as regulators of cellular activity in both pathological and non-pathological conditions (O’Brien et al., 2018; Ranganathan and Sivasankar, 2014).
Recent studies have indicated that alterations in the expression of specific miRNAs may be linked to the regulation of biological processes involved in neurodegenerative diseases (Li et al., 2023; Mu et al., 2024). Furthermore, various investigations have shown that circulating miRNAs are present in peripheral fluids such as blood, serum, plasma, and saliva, as well as in extracellular vesicles like exosomes. These miRNAs can be easily detected and, due to their high stability, reflect subtle changes that occur during the early stages of neurodegeneration (Kamal and Shahidan, 2020; Kumar and Reddy, 2016).
To date, various studies have evaluated the expression of miRNAs in MCI. However, challenges exist in identifying reliable molecular markers mainly due to the lack of multi-platform standardization, the continuous discovery of new miRNAs, and the small sample size (Condrat et al., 2020). Therefore, integrative approaches such as meta-analysis, which enable the combination of data from multiple studies to identify the most relevant miRNAs, have emerged as a promising option. These methods not only contribute to increasing predictive capacity but also help overcome potential contradictions present in individual research (Roointan et al., 2023; Yuen et al., 2021).
Considering the above, our aim was to identify commonly dysregulated miRNAs in MCI and characterize their biological significance. To achieve this, we conducted a meta-analysis encompassing previously published studies on miRNA expression profiles in samples of plasma, serum, and extracellular vesicles. Additionally, we aimed to characterize the biological significance of these findings through pathway analysis and functional enrichment, as well as the construction of interaction networks between miRNAs and their target genes. This approach significantly contributes to understanding the underlying molecular mechanisms in MCI, as well as to identifying potential molecular markers for this condition.
In this work, the recommendations of the PRISMA statement for the conduct and reporting of meta-analyses were considered (Moher et al., 2009). The databases used to perform the search for original studies were PubMed, Gene Expression Omnibus (GEO), and Sequence Read Archive (SRA). The following search terms were implemented: (“miRNA”) OR (“miRNA*”) OR (“microRNA”) OR (“microRNAs”) AND (“plasma”) OR (“blood”) OR (“serum”) OR (“extracellular vesicles”) AND (“mild cognitive impairment”) OR (“MCI”) OR (“Cognitive Impairment, Mild”) OR (“Impairment, Mild Cognitive”) OR (“Cognitive Impairment*”) AND (“next generation sequencing”) OR (“NGS”) OR (“microArray”) OR (“Array”); these terms were applied in the titles, abstracts, and keywords present in the selected databases.
Original articles meeting the following criteria were included: (a) primary studies. (b) Works evaluating the differential expression profile of miRNAs in samples of plasma, serum, and/or extracellular vesicles from patients with MCI and controls. (c) Studies assessing the relative expression of miRNAs using miRNA microarrays, RNA sequencing, or qRT-PCR. (d) Studies providing a list of differentially expressed miRNAs (DE-miRNAs) or presenting raw data. (e) Studies reporting cut-off criteria for DE-miRNAs. (f) Studies reporting sample size. In contrast, studies were excluded from this analysis if: (a) they were conducted in cell lines and animal models. (b) They evaluated a limited set of pre-selected miRNAs. (c) They did not include MCI cases; they were reviews or other meta-analyses. Two investigators performed the search in an independent manner (YG-G and NV-R) until June 2023.
Lists of DE-miRNAs, along with corresponding Fold-Change (FC) values and p-values, were obtained from publications, supplementary web pages, analysis of raw data or files provided by the authors (Kumar et al., 2017; Sun et al., 2023; Tatara et al., 2023).
For analysis of raw data, the processing of available datasets in the GEO and SRA databases was carried out as follows: studies presenting data from microarray platform (GSE147232, GSE120584) (He et al., 2021; Tao et al., 2020) and PCR panels (GSE90828) (Kayano et al., 2016), were individually processed using the GEO2R tool on the GEO NCBI, which implements the Rstudio packages GEOquery and limma from the Bioconductor project to determine DE-miRNAs (Ritchie et al., 2015). On the other hand, the Galaxy platform was utilized to determine miRNAs from studies presenting raw data available on the SRA platform (ERP133592/E-MTAB-11222) (Xiaohuan, 2022). Briefly, data in its raw form were evaluated using the FASTQ format. The Trim Galore tool was used to remove adapter sequences and non-specific dimers through read trimming and filtering. Read alignment was performed using the MiRDeep2 Mapper and MiRDeep2 Quantifier tools, taking into account human miRNA precursors reported in mirBase. Finally, the DESeq2 tool was used to determine DE-miRNAs (Mohanan et al., 2023).
In all cases, the miRNA expression profile was compared using only samples from patients with MCI and control samples. Independent lists were generated for each study, and significant miRNAs detected were organized into upregulated and downregulated categories. MiRNAs with |log 2 FC| > 0 and p-value <0.05 were considered DE-miRNAs. The miRBase database was used to standardize miRNA names (Griffiths-Jones et al., 2006; Roointan et al., 2023). Non-human miRNA probes were excluded from the meta-analysis.
The meta-analysis was conducted using the Robust Rank Aggregation (RRA) method (Võsa et al., 2014) and the Vote Counting method (Griffith et al., 2006), as described below.
The Robust Rank Aggregation Approach is based on the idea of combining individual rankings from multiple studies in a solid and reliable manner. The main objective is to obtain a global ranking that reflects the hierarchy of the evaluated miRNAs, taking into account the uncertainty and potential sources of variability or bias present in the data (Kolde et al., 2012). Lists of upregulated and downregulated DE-miRNAs were separately ranked based on the relative expression changes reported in each study. The RobustRankAggreg package v1.2.1 in Rstudio was used to integrate the results from the selected studies and determine the DE-miRNAs among them (Võsa et al., 2014). The p-value of each miRNA indicated its ranking in the final list, and miRNAs with a p-value <0.05 were considered significant.
A list was constructed containing the upregulated and downregulated DE-miRNAs from each individual study. The Vote Counting method (VCR) for ranking potential molecular biomarkers was adopted for the meta-analysis (Chan et al., 2008; Griffith et al., 2006). This methodology is based on the number of studies reporting the differential expression of a miRNA, applying a classification based on the total sample size and the Fold Change (FC) (Griffith et al., 2006). MiRNAs with consistent regulation in at least two or more studies were considered commonly dysregulated. All analyses were performed using Rstudio v4.2.3.
The miRNAs resulting from the two meta-analysis methods were termed MCI-miRNA meta-signatures. The online tool DIANA-mirPath v.3 was employed to identify the Kyoto Encyclopedia of Genes and Genomes (KEGG) pathways and gene ontology (GO) terms potentially affected by the dysregulation of these miRNAs (Vlachos et al., 2015). This involved the exploring of various functional categories, including molecular functions, biological processes, and cellular components within the GO. Analyses were conducted separately for miRNAs showing consistent upregulation and those showing consistent downregulation. Statistical significance was assessed using the hypergeometric distribution, with the Benjamini-Hochberg false discovery rate (FDR) of 0.05 applied for multiple testing correction.
The interaction networks for consistently upregulated and downregulated miRNAs were constructed independently. The interaction analysis and network construction between MCI-miRNAs and their target genes were conducted using the miRNet v2.0 platform1. The identification of interactions was based on the miRTarBase v8.0 database, which provides curated information on miRNA-target gene interactions (Chang et al., 2020). For the network of consistently upregulated miRNAs, a filter based on betweenness centrality with a threshold of 10 was applied, allowing the analysis to focus on the most relevant nodes within the network.
To validate the interactions between the MCI-miRNAs and their target genes, an external dataset of differentially downregulated genes in peripheral blood from patients with amnestic MCI was used (Bharthur Sanjay et al., 2022). The MCI-miRNAs consistently upregulated in our study were used to construct the interaction network with these genes. The network was built using the miRNet v2.0 platform (see Footnote 11 ).
A total of 138 studies evaluating miRNA expression in subjects with Mild Cognitive Impairment (MCI) were identified. However, raw data or lists of differentially expressed miRNAs were not available in some cases, and others did not meet the inclusion criteria. Therefore, only seven studies were included in the final analysis. The miRNAs assessed in these studies were detected in different samples, which included plasma (3 studies), serum (2 studies), and exosomes (2 studies). The flow diagram showing the different phases for choosing the included studies is illustrated in Figure 1. Details of the characteristics of the included studies are shown in Table 1.
This meta-analysis included 139 samples from subjects diagnosed with MCI (40 from plasma, 48 from serum, and 51 from exosomes) and 158 samples from subjects with normal cognition (47 from plasma, 46 from serum, and 65 from exosomes). A total of 640 DE-miRNAs (|log 2 FC| > 0 and p-value <0.05) were identified between cases and controls. After to implement the Robust Rank Aggregation (RRA) method no statistically significant results were obtained.
According to the VCR analysis, from 64 miRNAs reported in at least two studies, 12 miRNAs showed a consistent direction of regulation among the analyzed samples. The miRNAs hsa-miR-23a-3p, hsa-miR-5001-5p, hsa-miR-6087, hsa-miR-4488, hsa-miR-6724-5p, hsa-miR-149-3p, hsa-miR-150-3p, and hsa-miR-99b-5p showed consistent upregulation, while hsa-miR-4431, hsa-miR-1185-2-3p, let-7f-5p, and hsa-miR-550a-5p showed consistent downregulation (Figure 2). Furthermore, we also observed that other miRNAs, such as hsa-miR-4745-5p, hsa-miR-4433b-3p, hsa-miR-125b-5p, hsa-miR-191-3p, hsa-miR-762, and hsa-miR-1249-3p, exceeded the identification threshold but showed inconsistent regulation patterns among samples (Figure 2). miRNAs that did not meet the identification threshold or presented inconsistent regulation patterns were excluded from further analysis (Supplementary Table S1).
Figure 2. Heatmap showing the miRNAs reported in two or more studies with statistical significance (p < 0.05). The columns represent the studies included in the meta-analysis, while the rows correspond to the identified miRNAs. The color of the rectangles indicates the expression levels of the miRNAs, with dark blue indicating positive regulation (FC > 1) and white indicating negative regulation (FC < 1). The sample sources used in each study are specified at the top of the figure (Exosome, plasma, serum) (visual representation generated with SRPLOT, https://www.bioinformatics.com.cn/en).
The enrichment analysis of KEGG and GO terms for miRNAs with consistent upregulation and downregulation was performed independently. For miRNAs showing consistent upregulation (hsa-miR-23a-3p, hsa-miR-5001-5p, hsa-miR-6087, hsa-miR-4488, hsa-miR-6724-5p, hsa-miR-149-3p, hsa-miR-150-3p, and hsa-miR-99b-5p, a total of 34), significantly enriched pathways were identified. Among these, the HIF-1 signaling pathway (hsa04066), mTOR signaling pathway (hsa04150), platelet activation (hsa04611), p53 signaling pathway (hsa04115), regulation of actin cytoskeleton (hsa04810), protein processing in endoplasmic reticulum (hsa04141) and neurotrophin signaling pathway (hsa04722) were regulated by four or more miRNAs. We focused on those pathways based on their important role in MCI, according to previous reports previously detected with MCI (Figure 3).
Figure 3. Enrichment analysis of KEGG pathway for consistent dysregulated miRNA. Sankey diagram illustrating the interaction between miRNAs and the identified KEGG pathways. miRNAs consistently upregulated are shown in red, while miRNAs consistently downregulated are depicted in blue. Each ribbon corresponds to a KEGG pathway, and the interactions or involvement of the miRNAs with each pathway are represented by the connections between the ribbons and miRNAs. miRNAs that interact with a greater number of pathways are considered the most influential in the analyzed pathways, highlighting their relevance in the regulation of key biological processes in MCI. The identified pathways include: Prion diseases (hsa05020), Adherens junction (hsa04520), Glioma (hsa05214), p53 signaling pathway (hsa04115), mTOR signaling pathway (hsa04150), Platelet activation (hsa04611), HIF-1 signaling pathway (hsa04066), Focal adhesion (hsa01212), Protein processing in endoplasmic reticulum (hsa04141), Regulation of actin cytoskeleton (hsa04810), Neurotrophin signaling pathway (hsa04722), MAPK signaling pathway (hsa04010), FoxO signaling pathway (hsa04068), Ubiquitin mediated proteolysis (hsa04120), PI3K-Akt signaling pathway (hsa04151), and TGF-beta signaling pathway (hsa04350) (visual representation generated with SRPLOT, https://www.bioinformatics.com.cn/en).
Regarding miRNAs exhibiting consistent downregulation (hsa-miR-4431, hsa-miR-1185-2-3p, let-7f-5p, and hsa-miR-550a-5p), a total of 35 significantly enriched pathways were identified. Some of these pathways were the following: the FoxO signaling pathway (hsa04068) and protein processing in the endoplasmic reticulum (hsa04141) were regulated by three or more miRNAs (Table 2). Additionally, pathways related to the hippo signaling pathway (hsa04390), ubiquitin-mediated proteolysis (hsa04120), PI3K-Akt signaling pathway (hsa04115), MAPK signaling pathway (hsa04010), and TGF-beta signaling pathway (hsa04350) were associated with genes modulated by these miRNAs (Figure 3).
Table 2. Kyoto Encyclopedia of Genes and Genomes (KEGG) pathway enrichment by consistently regulated miRNAs according to the number of miRNAs involved in the enrichment.
The GO terms analyzed in the enrichment of the positively and negatively regulated miRNA groups showed significant results (Supplementary Table S2). It is noteworthy that both upregulated and downregulated miRNAs are involved in a variety of biological processes, cellular components, and molecular functions that may be closely related to MCI.
Regarding biological processes, both miRNA groups showed a strong association with the metabolism of cellular nitrogen compounds and biosynthetic processes, as well as with protein modification and gene expression regulation. The observed differences between upregulated and downregulated miRNAs provide further insights into their potential roles in MCI. Upregulated miRNAs showed a stronger association with the stress response and cell death (Figure 4A). In contrast, downregulated miRNAs were more strongly associated with the signaling of neurotrophin TRK receptors and Toll-like receptor signaling pathways, such as the TRIF-dependent pathway and Toll-like receptor 3 signaling (Figure 4B).
Figure 4. Gene ontology (GO) term analysis associated with biological functions, cellular processes, or cellular components related to miRNAs. (A) GO terms associated with miRNAs that are consistently upregulated. (B) GO terms associated with miRNAs that are consistently downregulated. The size of each circle represents the proportion of genes associated with the corresponding GO term. The X-axis shows the GO terms, while the Y-axis reflects the -log10(p-value), indicating the statistical significance of the association between miRNAs and the GO terms (visual representation generated with SRPLOT, https://www.bioinformatics.com.cn/en).
Regarding molecular functions, both miRNA groups showed a notable association with ion binding and RNA binding. However, upregulated miRNAs were more linked to protein binding and transcription factor activity.
The miRNAs that showed consistent regulation across different samples of subjects with MCI and controls were used to build a network to determine their potential interactions with target genes. miRNA-mRNA interaction networks were constructed independently for miRNAs consistently upregulated and downregulated.
The interaction network for the eight miRNAs consistently upregulated identified a total of 1,496 nodes and 1,716 edges. Specifically, the network comprised 8 miRNA nodes and 1,488 mRNA nodes. Each mRNA node represents a target gene regulated by one or more of the upregulated miRNAs. In this network, the hsa-miR-149-3p represented the most important node with a high degree of connectivity (Degree = 834). On the other hand, it is remarkable that this analysis shows that various of these miRNAs convergent on the same target gene, which are key for connecting this network. For instance, the following genes: NACC1 (nucleus accumbens associated 1), SLC7A5 (solute carrier family 7 member 5), and FOXK1 (forkhead box K1) were identified as the most important nodes in our network based on their high degree and betweenness. Specifically, NACC1 was found to be a common target of hsa-miR-149-3p, hsa-miR-6087, hsa-miR-23a-3p, and hsa-miR-4488. Similarly, SLC7A5 was regulated by hsa-miR-149-3p, hsa-miR-6087, hsa-miR-4488, and hsa-miR-6724-5p, while FOXK1 (hairpin box K1) was the target of hsa-miR-149-3p, hsa-miR-6087, hsa-miR-5001-5p, and hsa-miR-6724-5p (Figure 5A).
Figure 5. Analysis of the mRNA-miRNA interaction network. (A) Network of consistently upregulated miRNAs and (B) Network of consistently downregulated miRNAs. Blue squares represent miRNAs, while fuchsia circles represent mRNAs. The size of the squares and circles reflects the degree of connectivity of each element. The miRNA with the highest degree of connectivity in each network is highlighted in orange.
In contrast, the first-order interaction network of the four consistently downregulated miRNAs revealed a total of 599 nodes and 612 edges. This network consisted of three miRNA nodes and 596 mRNA nodes. Let-7f-5p stood out as the main node in the network (Degree = 397). We identified BACH1 (BTB domain and CNC homolog 1), PMAIP1 (phorbol-12-myristate-13-acetate-induced protein 1), ZNF264 (zinc finger protein 264), and RBM12B (RNA binding motif protein 12B) as mRNA targets commonly regulated by both let-7f-5p and hsa-miR-1185-2-3p. Additionally, MOB4 (MOB family member 4, phocein), WNK1 (WNK lysine deficient protein kinase 1), KLHL15 (kelch like family member 15), and HSPE1-MOB4 (HSPE1-MOB4 readthrough) were identified as mRNA targets shared between hsa-miR-1185-2-3p and hsa-miR-550a-5p. Furthermore, GABPB1 (GA binding protein transcription factor subunit beta 1), SMC1A (structural maintenance of chromosomes 1A), SEMA4C (semaphorin 4C), SLC38A7 (solute carrier family 38 member 7), YOD1 (YOD1 deubiquitinase), KIAA1328 (hinderin), ZNF556 (zinc finger protein 556), and PPP1R15B (protein phosphatase 1 regulatory subunit 15B) were also identified as mRNA targets commonly regulated by let-7f-5p and hsa-miR-550a-5p (Figure 5B).
The MCI-miRNAs with consistent upregulation were used to evaluate their interactions with differentially expressed genes in peripheral blood from patients with amnestic MCI (Bharthur Sanjay et al., 2022). The resulting interaction network included 4 miRNAs and 35 genes, with hsa-miR-23a-3p being the most prominent node, showing a connectivity (Degree = 35) (Supplementary Figure S1).
In this work, we performed an integrative meta-analysis of miRNA expression profiles in plasma, serum, and extracellular vesicles, specifically exosomes, from subjects with MCI and control subjects. We identified eight consistently upregulated miRNAs (hsa-miR-23a-3p, hsa-miR-5001-5p, hsa-miR-6087, hsa-miR-4488, hsa-miR-6724-5p, hsa-miR-149-3p, hsa-miR-150-3p, and hsa-miR-99b-5p) and four consistently downregulated miRNAs (hsa-miR-4431, hsa-miR-1185-2-3p, let-7f-5p, and hsa-miR-550a-5p) by means of VCR method. The interaction network analysis between miRNA-mRNA highlighted the possible key role of hsa-miR-149-3p and Let-7f-5p in MCI.
The hsa-miR-149-3p was found as a central node with a high degree of connectivity in the interaction network of upregulated miRNAs (Figure 4A), suggesting its possible influence in regulating multiple cellular processes through the modulation of a wide range of target genes. Specifically, hsa-miR-149-3p was found to be upregulated in serum samples, indicating its potential as a minimally invasive diagnostic marker (Chen et al., 2008). This miRNA has been implicated in various pathological conditions, including several types of cancer such as glioma (He et al., 2018; Lu et al., 2022; Wang et al., 2023) and central nervous system diseases like amyotrophic lateral sclerosis (ALS) (Katsu et al., 2019). Additionally, it is involved in several critical biological processes such as cell proliferation, apoptosis, and cell cycle regulation (Wang et al., 2023). However, its potential influence on cognitive decline has not yet been established (Xiamin et al., 2020), and the expression of hsa-miR-149-3p in serum samples in MCI has not been validated.
Interestingly, a study reported a positive correlation between serum levels of hsa-miR-149, the precursor of hsa-miR-149-3p, and MMSE scores in Alzheimer’s disease (AD) patients, suggesting that miR-149 might be involved in the progression of this disease (Du et al., 2021). Given that the MMSE is widely used for assessing MCI, it is crucial to investigate whether this association also manifests in specific pathological processes of MCI and determine which of the two strands of the hsa-miR-149 precursor (hsa-miR-149-3p or hsa-miR-149-5p) could be specially involved in these conditions. Contrary to our observations in this meta-analysis, in AD patients this miRNA was observed downregulated. However, in another work, a similar pattern of expression was observed in patients with HIV-associated neurocognitive disorder, where the levels of hsa-miR-149-3p was found increased by comparing this group with HIV patients without this disorder (Asahchop et al., 2016). These findings support the idea that this miRNA might reflect the underlying biological alterations in neurocognitive disorders.
From the interaction network analysis in our study, we also determined that the consistently upregulated miRNAs, including hsa-miR-149-3p, exert significant influence in regulating key genes. We observed that NACC1 and FOXK1 were targets of hsa-miR-149-3p and proved to be important nodes (hubs) within the network. Both NACC1 and FOXK1 have been reported to act as transcriptional regulators (Gao et al., 2020; Schoch et al., 2017). Specifically, NACC1 is expressed in the CNS (Mackler et al., 2000; Xie et al., 2024) and plays a crucial role in maintaining synaptic plasticity (Daniel et al., 2023; Shen et al., 2007). On the other hand, FOXK1, whose DNA methylation has been associated with AD, has been linked to oxidative stress (De Jager et al., 2014; Sanchez-Mut and Gräff, 2015). These associations suggest that both NACC1 and FOXK1 could play significant roles in various biological processes related to MCI. However, further research is needed to confirm their direct involvement and to understand in more detail the specific mechanisms through which these genes and their interactions with hsa-miR-149-3p could influence the progression and pathogenesis of MCI. Additionally, it remains to be determined whether the expression of these genes can be indicative of pathological states related to MCI.
Regarding the consistently downregulated miRNAs, the interaction network identified Let-7f-5p as a central node with a high degree of connectivity (Figure 4B), showing consistent negative regulation in plasma and plasma-derived exosomes from subjects with MCI. It is important to note that the expression of Let-7f-5p has been reported differentially across various sample types in subjects with AD. For instance, Hara et al. (2017) reported upregulation of Let-7f-5p in serum samples from AD patients, whereas Leidinger et al. (2013) reported reduced levels of Let-7f-5p in whole blood. However, a study examining the association between plasma miRNA levels and changes in cognition over time found a negative correlation between Let-7f-5p and MMSE scores, suggesting that Let-7f-5p expression is linked to cognitive decline (Comfort et al., 2022). This indicates potential variation in its expression depending on the disease stage, ranging from pre-symptomatic or MCI stages to dementia or AD development. Nevertheless, further research is needed to confirm this relationship.
The variability in Let-7f-5p expression across different sample types underscores the need to consolidate these findings to establish its utility as a potential marker for MCI. Consolidating data will enable its potential clinical use, offering a non-invasive tool for early detection of MCI and monitoring progression to dementia or AD.
The interaction network analysis in our study revealed that consistently downregulated miRNAs, including Let-7f-5p, exert significant influence in regulating key genes, prominently featuring PMAIP1. This gene, recognized as an essential mediator of p53-dependent apoptosis, plays a crucial role in neurodegenerative disorders, particularly in cellular stress response (Gil-Jaramillo et al., 2023; Roufayel et al., 2022). Furthermore, a recent omics data integration study identified PMAIP1 as a potential risk gene for AD (Wang et al., 2021). These observations and the increased risk of progression to AD due to MCI open new perspectives for evaluating the possible relationship between PMAIP1 and MCI, which could be crucial for better understanding the underlying mechanisms in the transition from MCI to AD.
Additionally, we identified hsa-miR-23a-3p as a central node in the interaction network between upregulated miRNAs and differentially expressed genes in the peripheral blood of patients with amnestic MCI, suggesting its potential as a key regulator in the pathogenesis of MCI. Previously, hsa-miR-23a-3p has been reported as downregulated in plasma extracellular vesicles and serum from patients with AD (Galimberti et al., 2014; Gámez-Valero et al., 2019). However, studies in brain tissue from AD patients have shown upregulation of this miRNA (Lau et al., 2013), highlighting a discrepancy in its expression depending on the type of sample and the biological context in which it is analyzed. According to our results, hsa-miR-23a-3p was consistently reported as upregulated in serum and plasma samples from MCI patients, suggesting that its regulation may depend on factors specific to the disease stage and the particular biological environments of each sample. This discrepancy in the regulation patterns of hsa-miR-23a-3p could indicate a complex biological function across the different stages of the disease. In this context, further studies are imperative to investigate the functional role of hsa-miR-23a-3p in greater depth, in order to elucidate its contribution to the underlying pathological mechanisms in both MCI and AD.
Our KEGG analysis revealed that HIF-1 signaling pathway, p53 signaling pathway, protein processing in the endoplasmic reticulum, and focal adhesion pathways were consistently regulated by both groups of miRNAs (Figure 3). Considering these pathways’ critical roles in neuronal function and early stages of neurodegeneration (Rønn et al., 2000; Sin and Nollen, 2015), these findings suggest that the regulation of these pathways may be linked to pathological mechanisms associated with MCI. Moreover, the involvement of miRNAs in both repression and activation of these pathways highlights the diversity of their regulatory function and suggests a complex interaction of potential molecular mechanisms underlying MCI.
Finally, we found that both groups of miRNAs were associated with biological processes that could influence the regulation of metabolic pathways essential for neuronal function. Their involvement in the modification of cellular proteins and the regulation of gene expression highlights their potential role in modulating key processes for neuronal plasticity and cognition, which are critical factors for maintaining cognitive abilities (Bhat et al., 2022).
Considering that the upregulated miRNAs showed a stronger association with the stress response and cell death, it is possible that they are involved in the adaptive response to neuronal damage, contributing to cellular defense mechanisms and participating in the regulation of neuronal homeostasis in response to stress that may be occurring during MCI (Butterfield, 2023). On the other hand, the consistent downregulation of miRNAs associated with Toll-like receptor signaling pathways suggests an alteration in immune responses and the regulation of brain inflammation. These processes have been identified as key factors in the pathogenesis of MCI, indicating that the regulation of these pathways could have important implications for modulating neuroinflammation (Liang and Wang, 2021; Su et al., 2016).
Together, these results suggest that both upregulated and downregulated miRNAs play complementary roles in the pathophysiology of MCI. Understanding how these miRNAs influence these biological processes could open new avenues for the development of therapeutic strategies aimed at restoring cellular balance and mitigating the effects of neuroinflammation in MCI.
Although the meta-analyses play a crucial role in detecting miRNAs with more reliable differential expression by integrating multiple studies, thereby increasing sample size and overcoming limitations inherent in individual studies (Wang et al., 2015):these analyses may face certain limitations, such as the scarcity of studies evaluating miRNA profiles in subjects with MCI. Additionally, the lack of availability of raw data poses another obstacle to research. For example, while we identified studies in PubMed analyzing miRNA profiles in MCI subjects, many authors do not share raw data in any database. Therefore, it is essential for all studies to provide raw data on expression profiles, which would facilitate access to and reuse of these public datasets for future analyses (Forero et al., 2019).
Other limitation related to our work was the heterogeneity between the included studies, which could affect the robustness of the results obtained using the RRA method. Differences in the platforms and protocols used, along with the scarcity of studies evaluating miRNAs in patients with MCI, complicated the interpretation of the findings. However, the complementary VCR approach provided additional insights, helping to mitigate some of these challenges. Since research on miRNAs in MCI is an emerging field, we believe that addressing these limitations in future studies is essential for identifying the profile and functional role of miRNAs in MCI.
In general, our findings are promising, as they open new perspectives for future research that could lead to the identification of specific molecular markers for MCI in easily accessible biological samples, providing valuable insights into the underlying molecular mechanisms of this condition. Validating the expression of hsa-miR-149-3p, Let-7f-5p, and hsa-miR-23a-3p in plasma and serum samples, as well as elucidating their interactions with target genes, are crucial steps to confirm their clinical relevance in the context of MCI. This knowledge could be key to developing new therapeutic strategies aimed at modulating the expression of specific miRNAs or the activity of their target genes, with the goal of preventing, delaying, or reversing cognitive decline in individuals at risk of developing AD (Abidin et al., 2023; Li et al., 2024).
The identification of specific miRNA profiles for MCI, along with a detailed understanding of the biological mechanisms involved, has crucial implications not only for the diagnosis and treatment of this condition but also for optimizing clinical management and designing more effective and personalized therapeutic strategies. This approach would represent a significant step toward precision medicine in the field of neurodegenerative diseases (Nuzziello et al., 2020). Furthermore, the detection of miRNAs in blood samples, supported by advanced technologies such as next-generation sequencing and PCR, would facilitate their integration into daily clinical practice, improving both diagnostic accuracy and therapeutic outcomes. However, for this approach to be clinically viable, it is essential to validate the findings in a broader biological context, ensuring their applicability across diverse populations and clinical settings, thus consolidating their inclusion in routine clinical practice.
In summary, our results pave the way for promising future research that could bring these advances to fruition, transforming the diagnosis and management of MCI and other neurodegenerative diseases, and providing a solid foundation for future clinical applications.
The datasets presented in this study can be found in online repositories. The names of the repository/repositories and accession number(s) can be found in the article/Supplementary material.
NV-R: Conceptualization, Data curation, Formal analysis, Funding acquisition, Investigation, Methodology, Project administration, Resources, Software, Supervision, Validation, Visualization, Writing – original draft, Writing – review & editing. YG-G: Conceptualization, Data curation, Formal analysis, Investigation, Methodology, Supervision, Writing – review & editing. ÁG: Conceptualization, Formal analysis, Methodology, Writing – review & editing. JG: Conceptualization, Formal analysis, Methodology, Supervision, Writing – review & editing. AA-P: Conceptualization, Formal analysis, Methodology, Supervision, Writing – review & editing.
The author(s) declare that financial support was received for the research, authorship, and/or publication of this article. This research was funded bySistema General de Regalias with BPIN 2020000100357.
The authors declare that the research was conducted in the absence of any commercial or financial relationships that could be construed as a potential conflict of interest.
The authors declare that no Generative AI was used in the creation of this manuscript.
All claims expressed in this article are solely those of the authors and do not necessarily represent those of their affiliated organizations, or those of the publisher, the editors and the reviewers. Any product that may be evaluated in this article, or claim that may be made by its manufacturer, is not guaranteed or endorsed by the publisher.
The Supplementary material for this article can be found online at: https://www.frontiersin.org/articles/10.3389/fnagi.2024.1524622/full#supplementary-material
Abidin, S. Z., Mat Pauzi, N. A., Mansor, N. I., Mohd Isa, N. I., and Hamid, A. A. (2023). A new perspective on Alzheimer’s disease: microRNAs and circular RNAs. Front. Genet. 14:1486. doi: 10.3389/fgene.2023.1231486
Álvarez-Sánchez, L., Peña-Bautista, C., Ferré-González, L., Balaguer, A., Baquero, M., Casanova-Estruch, B., et al. (2023). Assessment of plasma and cerebrospinal fluid biomarkers in different stages of Alzheimer’s disease and frontotemporal dementia. Int. J. Mol. Sci. 24:1226. doi: 10.3390/ijms24021226
Asahchop, E. L., Akinwumi, S. M., Branton, W. G., Fujiwara, E., Gill, M. J., and Power, C. (2016). Plasma microRNA profiling predicts HIV-associated neurocognitive disorder. AIDS 30, 2021–2031. doi: 10.1097/QAD.0000000000001160
Bharthur Sanjay, A., Patania, A., Yan, X., Svaldi, D., Duran, T., Shah, N., et al. (2022). Characterization of gene expression patterns in mild cognitive impairment using a transcriptomics approach and neuroimaging endophenotypes. Alzheimer Dement 18, 2493–2508. doi: 10.1002/alz.12587
Bhat, V. D., Jayaraj, J., and Babu, K. (2022). RNA and neuronal function: the importance of post-transcriptional regulation. Oxf. Open Neurosci. 1:kvac011. doi: 10.1093/oons/kvac011
Butterfield, D. A. (2023). Oxidative stress in brain in amnestic mild cognitive impairment. Antioxidants 12:462. doi: 10.3390/antiox12020462
Chan, S. K., Griffith, O. L., Tai, I. T., and Jones, S. J. M. (2008). Meta-analysis of colorectal cancer gene expression profiling studies identifies consistently reported candidate biomarkers. Cancer Epidemiol Biomarkers Prev 17, 543–552. doi: 10.1158/1055-9965.EPI-07-2615
Chang, L., Zhou, G., Soufan, O., and Xia, J. (2020). miRNet 2.0: network-based visual analytics for miRNA functional analysis and systems biology. Nucleic Acids Res. 48, W244–W251. doi: 10.1093/nar/gkaa467
Chen, X., Ba, Y., Ma, L., Cai, X., Yin, Y., Wang, K., et al. (2008). Characterization of microRNAs in serum: a novel class of biomarkers for diagnosis of cancer and other diseases. Cell Res. 18, 997–1006. doi: 10.1038/cr.2008.282
Chen, Y. X., Liang, N., Li, X. L., Yang, S. H., Wang, Y. P., and Shi, N. N. (2021). Diagnosis and treatment for mild cognitive impairment: a systematic review of clinical practice guidelines and consensus statements. Front. Neurol. 12:719849. doi: 10.3389/fneur.2021.719849
Comfort, N., Wu, H., De Hoff, P., Vuppala, A., Vokonas, P. S., Spiro, A., et al. (2022). Extracellular microRNA and cognitive function in a prospective cohort of older men: the veterans affairs normative aging study. Aging. (Albany, NY). 14, 6859–6886. doi: 10.18632/aging.204268
Condrat, C. E., Thompson, D. C., Barbu, M. G., Bugnar, O. L., Boboc, A., Cretoiu, D., et al. (2020). miRNAs as biomarkers in disease: latest findings regarding their role in diagnosis and prognosis. Cells 9:276. doi: 10.3390/cells9020276
Daniel, J. A., Elizarova, S., Shaib, A. H., Chouaib, A. A., Magnussen, H. M., Wang, J., et al. (2023). An intellectual-disability-associated mutation of the transcriptional regulator NACC1 impairs glutamatergic neurotransmission. Front. Mol. Neurosci. 16:1115880. doi: 10.3389/fnmol.2023.1115880
De Jager, P. L., Srivastava, G., Lunnon, K., Burgess, J., Schalkwyk, L. C., Yu, L., et al. (2014). Alzheimer’s disease: early alterations in brain DNA methylation at ANK1, BIN1, RHBDF2 and other loci. Nat. Neurosci. 17, 1156–1163. doi: 10.1038/nn.3786
Du, W., Lei, C., and Dong, Y. (2021). Microrna-149 is downregulated in Alzheimer’s disease and inhibits β-amyloid accumulation and ameliorates neuronal viability through targeting bace1. Genet. Mol. Biol. 44, e20200064–e20200068. doi: 10.1590/1678-4685-GMB-2020-0064
Dunne, R. A., Aarsland, D., O’Brien, J. T., Ballard, C., Banerjee, S., Fox, N. C., et al. (2021). Mild cognitive impairment: the Manchester consensus. Age Ageing 50, 72–80. doi: 10.1093/ageing/afaa228
Forero, D. A., Lopez-Leon, S., González-Giraldo, Y., and Bagos, P. G. (2019). Ten simple rules for carrying out and writing meta-analyses. PLoS Comput. Biol. 15:e1006922. doi: 10.1371/journal.pcbi.1006922
Galimberti, D., Villa, C., Fenoglio, C., Serpente, M., Ghezzi, L., Cioffi, S. M. G., et al. (2014). Circulating miRNAs as potential biomarkers in Alzheimer’s disease. J. Alzheimers Dis. 42, 1261–1267. doi: 10.3233/JAD-140756
Gámez-Valero, A., Campdelacreu, J., Vilas, D., Ispierto, L., Reñé, R., Álvarez, R., et al. (2019). Exploratory study on microRNA profiles from plasma-derived extracellular vesicles in Alzheimer’s disease and dementia with Lewy bodies. Transl. Neurodegener. 8:31. doi: 10.1186/s40035-019-0169-5
Gao, M., Laschuk Herlinger, A., Wu, R., Wang, T.-L., Shih, I.-M., Kong, B., et al. (2020). NAC1 attenuates BCL6 negative autoregulation and functions as a BCL6 coactivator of FOXQ1 transcription in cancer cells. Aging 12:9275. doi: 10.18632/aging.103203
Gil-Jaramillo, N., Aristizábal-Pachón, A. F., Luque Aleman, M. A., González Gómez, V., Escobar Hurtado, H. D., Girón Pinto, L. C., et al. (2023). Competing endogenous RNAs in human astrocytes: crosstalk and interacting networks in response to lipotoxicity. Front. Neurosci. 17:840. doi: 10.3389/fnins.2023.1195840
Griffith, O. L., Melck, A., Jones, S. J. M., and Wiseman, S. M. (2006). Meta-analysis and meta-review of thyroid cancer gene expression profiling studies identifies important diagnostic biomarkers. J. Clin. Oncol. 24, 5043–5051. doi: 10.1200/JCO.2006.06.7330
Griffiths-Jones, S., Grocock, R. J., van Dongen, S., Bateman, A., and Enright, A. J. (2006). miRBase: microRNA sequences, targets and gene nomenclature. Nucleic Acids Res. 34, D140–D144. doi: 10.1093/nar/gkj112
Groth, A., Corpet, A., Cook, A. J. L., Roche, D., Bartek, J., Lukas, J., et al. (2007). Regulation of replication fork progression through histone supply and demand. Science 318, 1928–1931. doi: 10.1126/science.1148992
Hansson, O., Zetterberg, H., Vanmechelen, E., Vanderstichele, H., Andreasson, U., Londos, E., et al. (2010). Evaluation of plasma Aβ40 and Aβ42 as predictors of conversion to Alzheimer’s disease in patients with mild cognitive impairment. Neurobiol. Aging 31, 357–367. doi: 10.1016/j.neurobiolaging.2008.03.027
Hara, N., Kikuchi, M., Miyashita, A., Hatsuta, H., Saito, Y., Kasuga, K., et al. (2017). Serum microRNA miR-501-3p as a potential biomarker related to the progression of Alzheimer’s disease. Acta Neuropathol. Commun. 5:10. doi: 10.1186/s40478-017-0414-z
He, H., Liu, A., Zhang, W., Yang, H., Zhang, M., Xu, H., et al. (2021). Novel plasma miRNAs as biomarkers and therapeutic targets of Alzheimer’s disease at the prodromal stage. J. Alzheimers Dis. 83, 779–790. doi: 10.3233/JAD-210307
He, Y., Yu, D., Zhu, L., Zhong, S., Zhao, J., and Tang, J. (2018). miR-149 in human cancer: a systemic review. J. Cancer 9, 375–388. doi: 10.7150/jca.21044
Jia, X., Wang, Z., Huang, F., Su, C., Du, W., Jiang, H., et al. (2021). A comparison of the Mini-mental state examination (MMSE) with the Montreal cognitive assessment (MoCA) for mild cognitive impairment screening in Chinese middle-aged and older population: a cross-sectional study. BMC Psychiatry 21:485. doi: 10.1186/s12888-021-03495-6
Kamal, N. N. S. B. N. M., and Shahidan, W. N. S. (2020). Non-exosomal and exosomal circulatory MicroRNAs: which are more valid as biomarkers? Front. Pharmacol. 10:500. doi: 10.3389/fphar.2019.01500
Katsu, M., Hama, Y., Utsumi, J., Takashina, K., Yasumatsu, H., Mori, F., et al. (2019). MicroRNA expression profiles of neuron-derived extracellular vesicles in plasma from patients with amyotrophic lateral sclerosis. Neurosci. Lett. 708:134176. doi: 10.1016/j.neulet.2019.03.048
Kayano, M., Higaki, S., Satoh, J. I., Matsumoto, K., Matsubara, E., Takikawa, O., et al. (2016). Plasma microRNA biomarker detection for mild cognitive impairment using differential correlation analysis. Biomark. Res. 4:22. doi: 10.1186/s40364-016-0076-1
Kolde, R., Laur, S., Adler, P., and Vilo, J. (2012). Robust rank aggregation for gene list integration and meta-analysis. Bioinformatics 28, 573–580. doi: 10.1093/bioinformatics/btr709
Kouzuki, M., Miyamoto, M., Tanaka, N., and Urakami, K. (2022). Validation of a novel computerized cognitive function test for the rapid detection of mild cognitive impairment. BMC Neurol. 22:457. doi: 10.1186/s12883-022-02997-4
Kumar, S., and Reddy, P. H. (2016). Are circulating microRNAs peripheral biomarkers for Alzheimer’s disease? Biochim. Biophys. Acta 1862, 1617–1627. doi: 10.1016/j.bbadis.2016.06.001
Kumar, S., Vijayan, M., and Reddy, P. H. (2017). MicroRNA-455-3p as a potential peripheral biomarker for Alzheimer’s disease. Hum. Mol. Genet. 26, 3808–3822. doi: 10.1093/hmg/ddx267
Lau, P., Bossers, K., Janky, R., Salta, E., Frigerio, C. S., Barbash, S., et al. (2013). Alteration of the microRNA network during the progression of Alzheimer’s disease. EMBO Mol. Med. 5, 1613–1634. doi: 10.1002/emmm.201201974
Lee, J. (2023). Mild cognitive impairment in relation to Alzheimer’s disease: an investigation of principles, classifications, ethics, and problems. Neuroethics 16:16. doi: 10.1007/s12152-023-09522-5
Leidinger, P., Backes, C., Deutscher, S., Schmitt, K., Mueller, S. C., Frese, K., et al. (2013). A blood based 12-miRNA signature of Alzheimer disease patients. Genome Biol. 14:R78. doi: 10.1186/gb-2013-14-7-r78
Li, Y. B., Fu, Q., Guo, M., Du, Y., Chen, Y., and Cheng, Y. (2024). MicroRNAs: pioneering regulators in Alzheimer’s disease pathogenesis, diagnosis, and therapy. Transl. Psychiatry 14:367. doi: 10.1038/s41398-024-03075-8
Li, S., Lei, Z., and Sun, T. (2023). The role of microRNAs in neurodegenerative diseases: a review. Cell Biol. Toxicol. 39, 53–83. doi: 10.1007/s10565-022-09761-x
Liang, Y., and Wang, L. (2021). Inflamma-MicroRNAs in Alzheimer’s disease: from disease pathogenesis to therapeutic potentials. Front. Cellul. Neurosci. 15:785433. doi: 10.3389/fncel.2021.785433
López, C., and Altuna, M. (2023). New community and Sociohealth challenges arising from the early diagnosis of mild cognitive impairment (MCI). J. Pers. Med. 13:1410. doi: 10.3390/jpm13091410
Lu, X., Jing, L., Liu, S., Wang, H., and Chen, B. (2022). MiR-149-3p is a potential prognosis biomarker and correlated with immune infiltrates in uterine Corpus endometrial carcinoma. Int. J. Endocrinol. 2022, 1–15. doi: 10.1155/2022/5006123
Mackler, S. A., Korutla, L., Cha, X.-Y., Koebbe, M. J., Fournier, K. M., Scott Bowers, M., et al. (2000). NAC-1 is a brain POZ/BTB protein that can prevent cocaine-induced sensitization in the rat. J. Neurosci. 20, 6210–6217. doi: 10.1523/JNEUROSCI.20-16-06210.2000
McNeill, E., and Van Vactor, D. (2012). MicroRNAs shape the neuronal landscape. Neuron 75, 363–379. doi: 10.1016/j.neuron.2012.07.005
Mohanan, E. M., Jhala, D., More, C. B., Patel, A. K., and Joshi, C. (2023). Bioinformatics analysis of miRNA and its associated genes to identify potential biomarkers of oral submucous fibrosis and oral malignancy. Cancer Reports 6:e1787. doi: 10.1002/cnr2.1787
Moher, D., Liberati, A., Tetzlaff, J., Altman, D. G., Antes, G., Atkins, D., et al. (2009). Preferred reporting items for systematic reviews and meta-analyses: the PRISMA statement. PLoS Med 6:e1000097. doi: 10.1371/journal.pmed.1000097
Morris, J. C. (2005). Mild cognitive impairment and preclinical Alzheimer’s disease. Geriatrics 9, 9–14.
Mu, C., Gao, M., Xu, W., Sun, X., Chen, T., Xu, H., et al. (2024). Mechanisms of microRNA-132 in central neurodegenerative diseases: a comprehensive review. Biomed. Pharmacother. 170:6029. doi: 10.1016/j.biopha.2023.116029
Nuzziello, N., Ciaccia, L., and Liguori, M. (2020). Precision medicine in neurodegenerative diseases: some promising tips coming from the microRNAs’ world. Cells 9:75. doi: 10.3390/cells9010075
O’Brien, J., Hayder, H., Zayed, Y., and Peng, C. (2018). Overview of microRNA biogenesis, mechanisms of actions, and circulation. Front. Docrinol. 9:402. doi: 10.3389/fendo.2018.00402
Ørom, U. A., Nielsen, F. C., and Lund, A. H. (2008). MicroRNA-10a binds the 5′UTR of ribosomal protein mRNAs and enhances their translation. Mol. Cell 30, 460–471. doi: 10.1016/j.molcel.2008.05.001
Papaliagkas, V., Kalinderi, K., Vareltzis, P., Moraitou, D., Papamitsou, T., and Chatzidimitriou, M. (2023). CSF biomarkers in the early diagnosis of mild cognitive impairment and Alzheimer’s disease. Int. J. Mol. Sci. 24, 24:976. doi: 10.3390/ijms24108976
Petersen, R. C. (2006). Mild cognitive impairment. Lancet 367:1979. doi: 10.1016/S0140-6736(06)68881-8
Plassman, B. L., Langa, K. M., Fisher, G. G., Heeringa, S. G., Weir, D. R., Ofstedal, M. B., et al. (2008). Prevalence of cognitive impairment without dementia in the United States. Ann Intern Med 148, 427–434. doi: 10.7326/0003-4819-148-6-200803180-00005
Ranganathan, K., and Sivasankar, V. (2014). MicroRNAs – biology and clinical applications. J. Oral Maxillofacial Pathol. 18, 229–234. doi: 10.4103/0973-029X.140762
Ratti, M., Lampis, A., Ghidini, M., Salati, M., Mirchev, M. B., Valeri, N., et al. (2020). MicroRNAs (miRNAs) and long non-coding RNAs (lncRNAs) as new tools for cancer therapy: first steps from bench to bedside. Target. Oncol. 15, 261–278. doi: 10.1007/s11523-020-00717-x
Ritchie, M. E., Phipson, B., Wu, D., Hu, Y., Law, C. W., Shi, W., et al. (2015). Limma powers differential expression analyses for RNA-sequencing and microarray studies. Nucleic Acids Res. 43:e47. doi: 10.1093/nar/gkv007
Rønn, L. C., Ralets, I., Hartz, B. P., Bech, M., Berezin, A., Berezin, V., et al. (2000). A simple procedure for quantification of neurite outgrowth based on stereological principles. J Neurosci Methods. 100, 25–32. doi: 10.1016/s0165-0270(00)00228-4
Roointan, A., Gholaminejad, A., Shojaie, B., Hudkins, K. L., and Gheisari, Y. (2023). Candidate MicroRNA biomarkers in lupus nephritis: a meta-analysis of profiling studies in kidney, blood and urine samples. Mol. Diagn. Ther. 27, 141–158. doi: 10.1007/s40291-022-00627-w
Roufayel, R., Younes, K., Al-Sabi, A., and Murshid, N. (2022). BH3-only proteins Noxa and Puma are key regulators of induced apoptosis. Life 12:256. doi: 10.3390/life12020256
Sabbagh, M. N., Boada, M., Borson, S., Chilukuri, M., Doraiswamy, P. M., Dubois, B., et al. (2020). Rationale for early diagnosis of mild cognitive impairment (MCI) supported by emerging digital technologies. J.Prevent. Alzheim. Dis. 7, 158–164. doi: 10.14283/jpad.2020.19
Sachs-Ericsson, N., and Blazer, D. G. (2015). The new DSM-5 diagnosis of mild neurocognitive disorder and its relation to research in mild cognitive impairment. Aging Mental Health 19, 2–12. doi: 10.1080/13607863.2014.920303
Sanchez-Mut, J. V., and Gräff, J. (2015). Epigenetic alterations in Alzheimer’s disease. Front. Behav. Neurosci. 9:347. doi: 10.3389/fnbeh.2015.00347
Schoch, K., Meng, L., Szelinger, S., Bearden, D. R., Stray-Pedersen, A., Busk, O. L., et al. (2017). A recurrent De novo variant in NACC1 causes a syndrome characterized by infantile epilepsy, cataracts, and profound developmental delay. Am. J. Hum. Genet. 100, 343–351. doi: 10.1016/j.ajhg.2016.12.013
Shen, H., Korutla, L., Champtiaux, N., Toda, S., LaLumiere, R., Vallone, J., et al. (2007). NAC1 regulates the recruitment of the proteasome complex into dendritic spines. J. Neurosci. 27, 8903–8913. doi: 10.1523/JNEUROSCI.1571-07.2007
Sin, O., and Nollen, E. A. (2015). Regulation of protein homeostasis in neurodegenerative diseases: the role of coding and non-coding genes. Cell Mol Life Sci. 72, 4027–47. doi: 10.1007/s00018-015-1985-0
Su, W., Aloi, M. S., and Garden, G. A. (2016). MicroRNAs mediating CNS inflammation: small regulators with powerful potential. Brain Behav. Immun 52, 1–8. doi: 10.1016/j.bbi.2015.07.003
Sun, Y., Hefu, Z., Li, B., Lifang, W., Zhijie, S., Zhou, L., et al. (2023). Plasma extracellular vesicle MicroRNA analysis of Alzheimer’s disease reveals dysfunction of a neural correlation network. Research 6:114. doi: 10.34133/research.0114
Tao, Y., Han, Y., Yu, L., Wang, Q., Leng, S. X., and Zhang, H. (2020). The predicted key molecules, functions, and pathways that bridge mild cognitive impairment (MCI) and Alzheimer’s disease (AD). Front. Neurol. 11:233. doi: 10.3389/fneur.2020.00233
Tatara, Y., Yamazaki, H., Katsuoka, F., Chiba, M., Saigusa, D., Kasai, S., et al. (2023). Multiomics and artificial intelligence enabled peripheral blood-based prediction of amnestic mild cognitive impairment. Curr. Res. Transl. Med. 71:103367. doi: 10.1016/j.retram.2022.103367
Vlachos, I. S., Zagganas, K., Paraskevopoulou, M. D., Georgakilas, G., Karagkouni, D., Vergoulis, T., et al. (2015). DIANA-miRPath v3.0: deciphering microRNA function with experimental support. Nucleic Acids Res. 43, W460–W466. doi: 10.1093/nar/gkv403
Võsa, U., Kolde, R., Vilo, J., Metspalu, A., and Annilo, T. (2014). Comprehensive meta-analysis of MicroRNA expression using a robust rank aggregation approach. Methods Mol. Biol. 1182, 361–373. doi: 10.1007/978-1-4939-1062-5_28
Wang, X., Ning, Y., and Guo, X. (2015). Integrative meta-analysis of differentially expressed genes in osteoarthritis using microarray technology. Mol. Med. Rep. 12, 3439–3445. doi: 10.3892/mmr.2015.3790
Wang, Y., Song, Y., Liu, Z., Li, J., Wang, G., Pan, H., et al. (2023). miR-149-3p suppresses the proliferation and metastasis of glioma cells by targeting the CBX2/Wnt/β-catenin pathway. Exp. Ther. Med. 26:562. doi: 10.3892/etm.2023.12261
Wang, Z., Zhang, Q., Lin, J. R., Jabalameli, M. R., Mitra, J., Nguyen, N., et al. (2021). Deep post-GWAS analysis identifies potential risk genes and risk variants for Alzheimer’s disease, providing new insights into its disease mechanisms. Sci. Rep. 11:20511. doi: 10.1038/s41598-021-99352-3
Xiamin, H., Xiang, Z., Zhang, W., Zhijun, Y., Xin, X., Zhang, R., et al. (2020). Protective effect of DLX6-AS1 silencing against cerebral ischemia/reperfusion induced impairments. Aging 12, 23096–23113. doi: 10.18632/aging.104070
Xie, Q., Tong, C., and Xiong, X. (2024). An overview of the co-transcription factor NACC1: beyond its pro-tumor effects. Life Sci. 336:122314. doi: 10.1016/j.lfs.2023.122314
Keywords: biomarkers, early diagnosis, mild cognitive impairment, miRNAs, neurodegeneration
Citation: Vargas-Rondón N, González-Giraldo Y, García Fonseca &Y, Gonzalez J and Aristizabal-Pachon AF (2025) MicroRNAs signatures as potential molecular markers in mild cognitive impairment: a meta-analysis. Front. Aging Neurosci. 16:1524622. doi: 10.3389/fnagi.2024.1524622
Received: 07 November 2024; Accepted: 31 December 2024;
Published: 15 January 2025.
Edited by:
Mounia Chami, Institut National de la Santé et de la Recherche Médicale (INSERM), FranceReviewed by:
Yehong Du, Chongqing Medical University, ChinaCopyright © 2025 Vargas-Rondón, González-Giraldo, García Fonseca, Gonzalez and Aristizabal-Pachon. This is an open-access article distributed under the terms of the Creative Commons Attribution License (CC BY). The use, distribution or reproduction in other forums is permitted, provided the original author(s) and the copyright owner(s) are credited and that the original publication in this journal is cited, in accordance with accepted academic practice. No use, distribution or reproduction is permitted which does not comply with these terms.
*Correspondence: Andrés Felipe Aristizabal-Pachon, YW5kcmVzX2FyaXN0aXphYmFsQGphdmVyaWFuYS5lZHUuY28=
Disclaimer: All claims expressed in this article are solely those of the authors and do not necessarily represent those of their affiliated organizations, or those of the publisher, the editors and the reviewers. Any product that may be evaluated in this article or claim that may be made by its manufacturer is not guaranteed or endorsed by the publisher.
Research integrity at Frontiers
Learn more about the work of our research integrity team to safeguard the quality of each article we publish.