- Division of Geriatric Endocrinology, The First Affiliated Hospital of Nanjing Medical University, Nanjing, China
Background: Cognitive frailty (CF), characterized by the coexistence of physical frailty and cognitive impairment, is linked to increased morbidity and mortality in older adults. While CF has been linked to multiple physiological and lifestyle factors, the underlying biological mechanisms remain poorly understood. This study investigated the risk factors for CF and explored the relationship between mitochondrial function and CF in hospitalized patients.
Methods: A total of 279 hospitalized individuals were recruited from December 2020 to August 2022, conducted comprehensive clinical assessments, and collected peripheral blood samples. CF was evaluated using the Physical Frailty Phenotype and Montreal Cognitive Assessment scales. Nutritional status was assessed with the Mini Nutritional Assessment, and depression was measured using the Geriatric Depression Scale. DNA was obtained from the peripheral blood and interrogated for mitochondrial DNA copy number (mtDNAcn). Peripheral blood mononuclear cells isolated from peripheral blood were examined for respiratory function and reactive oxygen species (ROS) levels. Additionally, plasma samples were analyzed for inflammatory markers and Carnitine Palmitoyltransferase II (CPT2).
Results: Among the participants, 90 were classified as CF and 46 as non-CF. Logistic regression analysis revealed that increased age (OR 1.156, 95% CI 1.064–1.255), lower educational attainment (OR 0.115, 95% CI 0.024–0.550), malnutrition (OR 0.713, 95% CI 0.522–0.973), and higher depression scores (OR 1.345, 95% CI 1.065–1.699) were significantly associated with CF. The independent t tests and Mann–Whitney U tests showed the CF group exhibited impaired mitochondrial function, characterized by reduced mtDNAcn and respiratory activity, coupled with elevated ROS, interleukin-6, and CPT2 levels compared with the non-CF group. After adjusted for age, sex, and BMI, compared with non-CF group, the OR values for the CF group of mtDNAcn and ROS were 0.234 (95% CI = 0.065–0.849) (p = 0.027) and 1.203 (95% CI = 1.075–1.347) (p = 0.001), respectively. The Sensitive analysis showed that the area under curve values for mtDNAcn and ROS were 0.653 and 0.925.
Conclusion: Age, lower educational attainment, malnutrition, and depression are significant risk factors for CF. Moreover, mitochondrial dysfunction, characterized by decreased mtDNAcn, impaired respiratory function and increased ROS levels appears to be a critical phenotype of CF.
1 Introduction
Since the early 21st century, the world has transitioned into an aging society, prioritizing healthy aging (Clegg et al., 2013; United Nations Department of Economic and Social Affairs, Population Division, 2024). And preserving cognitive function plays a crucial role in maintain health throughout the aging process (Davies et al., 2018). Generally, cognitive impairment is commonly recognized as a hallmark symptom in individuals with dementia, such as Alzheimer’s disease and vascular dementia. However, recent advancements in geriatric medicine have increasingly recognized cognitive frailty (CF), as a hidden yet pervasive syndrome, posing a significant threat to the cognitive health of many older adults. CF, introduced as a new concept in 2013, is characterized by the simultaneous presence of both physical frailty and mild cognitive impairment (MCI) (Panza et al., 2018; Kelaiditi et al., 2013; Sugimoto et al., 2022). While both CF and dementia in older adults involve cognitive impairment, they exhibit significant differences in clinical characteristics and pathological features. CF is defined as stable MCI that does not progress to dementia, whereas typical dementia is marked by a progressive decline in cognitive functions (Nader et al., 2023). Additionally, CF encompasses physical frailty, while dementia-related cognitive impairments tend to advance rapidly and are irreversible, with imaging studies revealing distinct changes such as brain atrophy and amyloid deposits (Kocagoncu et al., 2022).
Previous studies have shown that CF significantly increases risks for older adults, including falls, depression, malnutrition, disability, and higher hospitalization rates, positioning it as a predictor of adverse outcomes in this demographic (Guo X. et al., 2023; Zou et al., 2023; Tang et al., 2023; Qiu et al., 2023). Individuals with CF have a higher mortality rate compared with healthy older adults, those with MCI, and frail subjects, which places a substantial burden on society (Rivan et al., 2021; Vargas-Torres-Young et al., 2022). However, due to its potential reversibility, identifying risk factors and biomarkers for CF, coupled with targeted interventions, can facilitate healthier aging.
CF is reversible and may be mitigated through several interventions (Ibrahim et al., 2024; Hou et al., 2024; Tam et al., 2022; Li et al., 2022; Murukesu et al., 2020). Numbers of studies demonstrated that physical activity can ameliorate CF. A 24-month randomized controlled trial study reported that an organized moderate-intensity exercise can reduce CF (Liu et al., 2018). And another randomized controlled trial revealed that practicing mindfulness-based Tai Chi Chuan can boost both cognitive and physical functions in older adults (Jiayuan et al., 2022). Gutiérrez-Reguero et al. (2024) found that physical exercise can markedly enhance cognitive function, while dietary intervention alone cannot significantly improve cognitive frailty. Recently, Ibrahim et al. explored a multidimensional intervention for the reversal of CF, which consisted of vascular management, diet, exercise, cognitive and psychosocial stimulation (Ibrahim et al., 2024). Therefore, CF is not permanent and can potentially be alleviated through appropriate interventions, contributing to improved quality of life and independent living capabilities for older adults. And this feature of reversibility emphasizes the necessity for early detection and diagnosis of CF.
While CF has been linked to multiple physiological and lifestyle factors, the underlying biological mechanisms remain poorly understood. Mitochondria, known as the “energy factories” of the cells, are essential for supplying energy to neurons and regulating critical neuronal processes such as survival, regeneration, and the plasticity of axons and dendrites (Rangaraju et al., 2019). Impaired mitochondrial function in nerve cells is associated with cognitive decline, highlighting the close connection between mitochondrial health and cognitive abilities (Devine and Kittler, 2018; Apaijai et al., 2020). Restoration of mitochondrial function is critical for the management of cognitive dysfunction (Su et al., 2019). Previous studies reported that there was a negative correlation between mitochondrial DNA (mtDNA) levels in human peripheral blood mononuclear cells (PBMCs) and frailty, as well as cognitive decline (Ashar et al., 2015; Zhang et al., 2023a; Filograna et al., 2021; Tian et al., 2024). However, the relationship between mitochondrial function and CF remains unclear (Holland et al., 2024; Nader et al., 2023). Therefore, we hypothesize that mitochondrial dysfunction might be associated with CF and be a feature of CF in older adults.
This study aims to address this gap by investigating the risk factors associated with CF and exploring the relationship between mitochondrial function in peripheral blood and CF in hospitalized patients, offering insights into potential biomarkers and intervention strategies.
2 Methods
2.1 Participants, data collection and assessment scales
This study analyzed clinical data from 279 patients admitted to Jiangsu Province Hospital between October 2020 and August 2022 (Figure 1). Inclusion criteria were: (1) aged 50 years or older, (2) able to undergo all required examinations, and (3) stable underlying disease controlled by medication (for example, hypertension, coronary heart disease, diabetes, thyroid disease, etc.). Exclusion criteria included: (1) under 50 years of age, (2) long-term bed rest or significant impairment in activities of daily living, (3) severe cardiopulmonary dysfunction, (4) diagnosis of mitochondrial diseases (e.g., mitochondrial myopathy), (5) history of autoimmune disease or use of systemic steroids or immunosuppressive agents within the past 3 months, (6) recent (within 3 months) infection, surgery, chemotherapy, or radiation therapy for carcinomas, (7) recent (within 3 months) major physical trauma or diagnosed with depression, which means psychological impact of distressing events, major including accidents and natural disasters within last 3 months; and (8) inability to cooperate with examinations. The Ethical Committee of Jiangsu Province Hospital approved the study (2019-NT-48, 2024-SR-087), and all participants provided signed informed consent.
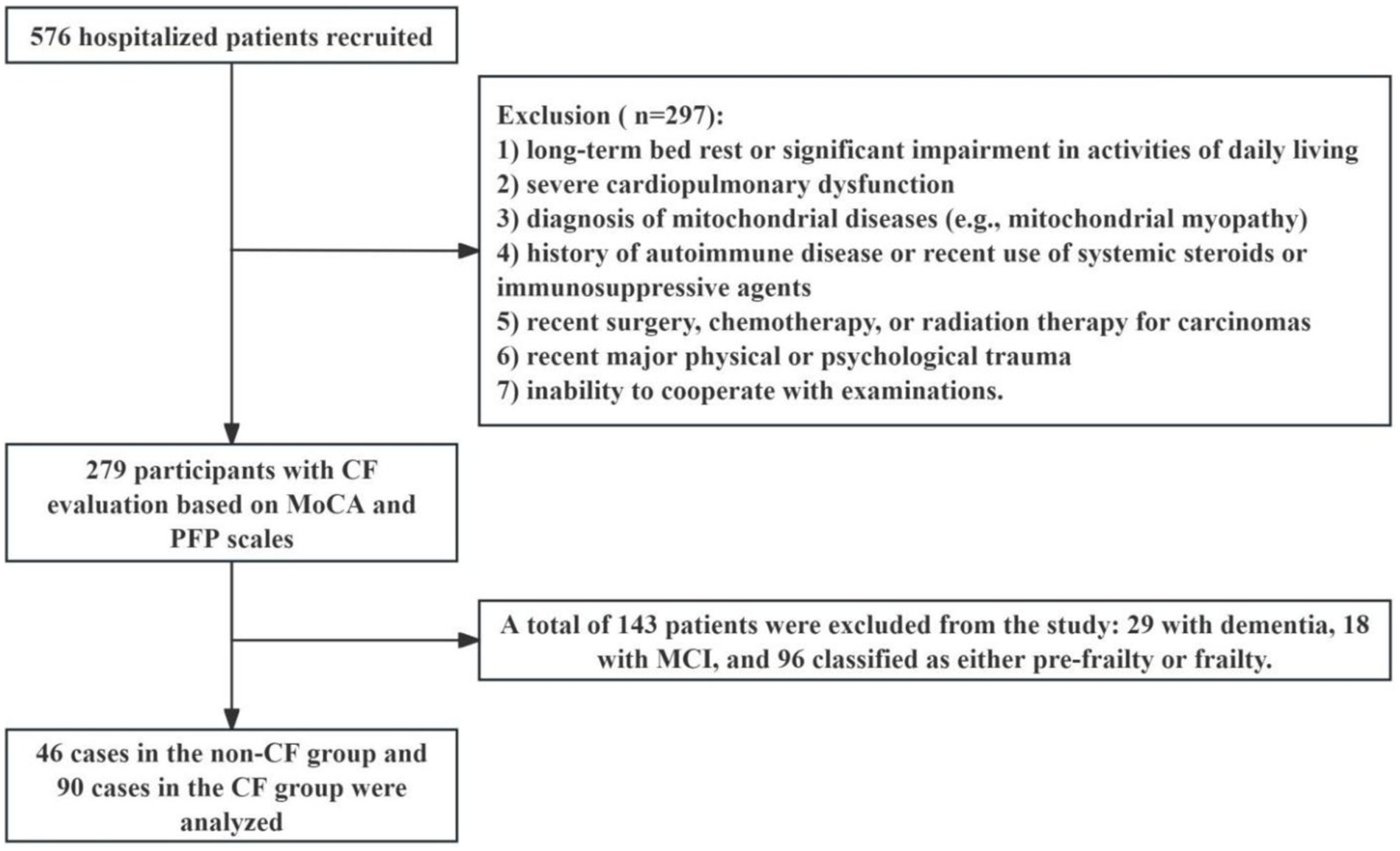
Figure 1. Flow chart of participants selection. CF: cognitive frailty, MCI, mild cognitive impairment; MoCA, the Montreal cognitive assessment; PFP, the physical frailty phenotype.
Patients underwent assessments across various health dimensions: (1) CF status measured with the Montreal Cognitive Assessment (MoCA) and the Physical Frailty Phenotype (PFP). Frailty status was assessed based on PFP including five components (Fried et al., 2001): PFP score < 1 = non-frail, 1 ≤ PFP score < 3 = pre-frail, 3 ≤ PFP score ≤ 5 = frail; Cognitive function was evaluated based on MoCA (Nasreddine et al., 2005): 26 ≤ MoCA score = normal cognitive function, 15 ≤ MoCA score < 26 = mild cognitive impairment (MCI), MoCA score ≤ 15 = dementia. Subjects were classified into the non-CF group if they had a PFP score = 0 and a MoCA score ≥ 26. In contrast, subjects with a PFP score of ≥1 and a MoCA score between 16 and 25 were classified into the CF group; (2) Mental Health, measured with the Self-Rating Anxiety Scale (SAS) and the Geriatric Depression Scale (GDS). SAS: total score ranges from 20 to 80, and higher scores correspond to a more pronounced tendency toward anxiety. GDS: total score ranges from 0 to 30, and higher scores indicate greater severity of depressive symptoms. (3) Physical Function, assessed with the Timed Up and Go test (TUG), the Short Physical Performance Battery (SPPB), and the strength, assistance walking, rise from a chair, climb stairs, and falls combined with calf circumference (SARC-Calf); (4) Nutritional Status, determined via the Mini Nutritional Assessment (MNA); (5) Fall Risk, evaluated using the Morse Fall Scale (MFS); (6) Comorbidity, assessed using the Cumulative Illness Rating Scale - Comorbidity Index (CIRS-CI).
2.2 Body composition
Dual-energy X-ray absorptiometry (DXA) (HOLOGIC, US) scans provided detailed assessments of body composition, including total fat mass, android and gynoid fat mass, and appendicular skeletal muscle mass. These measurements facilitated the calculation of the Fat Mass Index (FMI, defined as total fat mass divided by height squared), the Android-to-Gynoid (A/G) ratio, and the Appendicular Skeletal Muscle Mass Index (ASMI, calculated as appendicular skeletal muscle mass divided by height squared).
2.3 Total DNA extracted from peripheral blood
A total of 200 μL of fresh human venous blood was collected from participants using Vacutainer tubes containing sodium heparin as an anticoagulant. Total DNA was extracted from this peripheral blood, yielding a final volume of 75 μL, utilizing the FastPure® Blood DNA Isolation Mini Kit V2 (Vazyme, China), according to the manufacturer’s instructions.
2.4 Quantitative real-time polymerase chain reactions (qRT-PCR)
Mitochondrial DNA copy number (mtDNAcn) was assessed using Quantitative Real-Time Polymerase Chain Reactions (qRT-PCR) Instrument (Thermo Fisher, US). The reactions were conducted in a 10 μL volume, consisting of 2 μL of mtDNA template and 5 μL of ChamQ Universal SYBR qPCR Master Mix (Vazyme, China). qRT-PCR data were analyzed using the 2 − ΔΔCT method. The forward primer sequence for β-actin: ATTGGCAATGAGCGGTTCCGC, reverse primer: CTCCTGCTTGCTGATCCACATC; Forward primer sequence for MT-ND-1: CACTCACATCACAGCGCTAA; reverse primer: GGATTATGGATGCGGTTGCT.
2.5 Isolation of peripheral blood mononuclear cells (PBMCs)
All blood samples were obtained after overnight fasting. Two milliliters of freshly collected whole blood, anticoagulated with heparin, were obtained from participants for the isolation of PBMCs (T cells, B cells, dendritic cells, monocyte, phagocyte, natural killer cells, and a few other cell types) and plasma via Ficoll gradient centrifugation (TBD, China), in accordance with the manufacturer’s guidelines. The isolated PBMCs were subsequently used to evaluate oxygen consumption rate (OCR) and reactive oxygen species (ROS), while the plasma was stored at −80°C Refrigerator (Sanyo, Japan) for later analysis.
2.6 Oxygen consumption rate measurements (OCR) in mitochondria of PBMCs
OCR measurements in PBMCs were conducted using a Seahorse XFe24 extracellular flux analyzer (Agilent, US), following the manufacturer’s instructions. Briefly, 2 × 105 PBMCs were seeded onto Cell-Tak-coated XF24 V7 PS cell culture microplates in XF assay medium and incubated at 37°C for 1 h in a non-CO2 incubator. The XF assay medium was comprised of XF DMEM medium supplemented with 1 mM pyruvate, 1 mM glutamine, and 1 mM glucose. Subsequently, OCR was monitored at baseline and during sequential injections of oligomycin (1.5 μM), Carbonyl cyanide-4 (trifluoromethoxy) phenylhydrazone (FCCP, 0.5 μM), and a mix of rotenone and antimycin A (Rot/AA, 0.5 μM for each), using the XFe24 system. Data were collected and analyzed with Wave software and Microsoft Excel. The following mitochondrial respiration parameters were calculated: Basal Respiration = (baseline OCR) - (OCR after rotenone/antimycin A treatment), ATP-Linked Respiration = (Basal Respiration) - (OCR after Oligomycin treatment), Proton leak = (OCR after Oligomycin treatment) - (OCR after rotenone/antimycin A treatment), Maximal Respiration = (OCR after FCCP treatment) - (OCR after rotenone/antimycin A treatment), Spare Respiratory Capacity = (Maximal Respiration) - (Basal Respiration).
2.7 Reactive oxygen species (ROS) detection in PBMCs and flow Cytometric analysis
ROS levels in PBMCs were assessed using a Reactive Oxygen Species Assay Kit (Beyotime, China), according to the manufacturer’s instructions. Notably, 2′,7′-Dichlorofluorescin diacetate (DCFH-DA) is a non-fluorescent probe that can be oxidized by reactive oxygen species (ROS), resulting in the formation of the highly fluorescent dichlorofluorescein (DCF). Freshly isolated PBMCs were incubated with 10 μmol/L DCFH-DA probes at 37°C for 20 min, with mixing every 3 min. PBMCs were then washed three times with phosphate-buffered saline (PBS). Sample analysis was performed using flow cytometer (BD, USA).
2.8 Enzyme-linked immunosorbent assay (ELISA)
Concentrations of inflammatory markers (IL-1β, IL-6, TNF-α) (Multi Sciences, China) and metabolites (CPT2, L-Carnitine) (Maisha, China) in plasma were measured using ELISA kits, following the manufacturers’ protocols (Niu et al., 2024; Xuekelati et al., 2024). Optical density was measured at wavelengths of 570 nm and 630 nm using a microplate reader.
2.9 Statistical analysis
Data were analyzed using IBM SPSS Statistics Version 25. Normality was assessed using measures of Skewness and Kurtosis, the Shapiro–Wilk test, histograms, and Q-Q plots. Continuous variables were expressed as mean ± standard deviation (SD). We first examined differences in participants’ characteristics by CF status. We used independent t tests or Mann–Whitney U tests for used independent t tests for continuous variables, and chi-square for categorical variables, as appropriate. To investigate the risk factors of CF, we used the binary logistic regression models, including the univariate and multivariate analyses, which adjusted for age, sex, BMI, education level, (MNA) scores, and (GDS) scores. We then examined the association between the level of mtDNAcn, mitochondrial respiration, ROS, CPT2, L-Carnitine, IL-1β, IL-6, or TNF-α and CF using the independent t tests or Mann–Whitney U tests, as appropriate. To adjust for age, sex, and BMI, we also used logistic regression analysis to investigate the relationship between mtDNAcn or ROS and CF. Furthermore, to evaluate whether mtDNAcn or ROS may be a predictor of CF, we used the sensitivity analysis to analyze the sensitivity of data. Differences in mitochondrial function between groups were illustrated through columnar scatter plots generated using GraphPad Prism software, and ROS levels were analyzed with FlowJo software. The sensitivity analysis was conducted by IBM SPSS Statistics Version 25 and GraphPad Prism software. Statistical significance was defined at p < 0.05.
3 Results
3.1 Demographics and group distribution
This study involved 279 participants, with a mean age of 73.63 (± 10.19 years) and a Body Mass Index (BMI) of 23.95 (± 3.21 kg/m2), including 185 males (66.31%) and 94 females (33.69%). Participants were categorized based on the PFP and the MoCA scales. The non-CF group (n = 46) exhibited no signs of frailty and maintained normal cognitive function, while the CF group (n = 90) showed indicators of pre-frailty or frailty combined with MCI. An additional 143 participants were identified as having conditions such as frailty, MCI, or dementia.
Comorbidities included hypertension (47.83% in the non-CF group, 22 cases; 32.72% in the CF group, 53 cases), diabetes (39.13% in the non-CF group, 18 cases; 25.17% in the CF group, 37 cases), coronary heart disease (10.87% in the non-CF group, 5 cases; 35.94% in the CF group, 23 cases), malnutrition (4.35% in the non-CF group, 2 cases; 44.83% in the CF group, 26 cases), and dyslipidemia (43.48% in the non-CF group, 20 cases; 31.25% in the CF group, 35 cases). The hospital length of stay was 8.98 ± 1.20 days for the non-CF group and 12.90 ± 0.94 days for the CF group. There were notable differences in malnutrition and the hospital length of stay between the two groups (Table 1). Additionally, in the assessment of CF using the MoCA and PFP scales in 279 participants, 143 individuals were excluded, including 29 diagnosed with dementia, 18 with MCI without frailty, and 96 classified as pre-frail or frail without MCI.
3.2 Clinical factors and group comparisons
Baseline characteristics are summarized in Table 1. The CF group was significantly older and had higher scores on the MFS, TUG, GDS, SAS, and CIRS-CI. Conversely, the CF group exhibited significantly lower educational attainment, BMI, ASMI, A/G ratios, SPPB scores, handgrip strength, 4-meter gait speed, and MNA scores, and various biochemical markers, including insulin, triglycerides, albumin, retinol-binding protein, prealbumin, hemoglobin, and free triiodothyronine. No significant differences were found in gender distribution, waist-to-hip ratio (WHR), smoking or drinking history, FMI, Glycosylated Hemoglobin, total cholesterol, glucose, low-density lipoprotein, high-density lipoprotein, total protein, transferrin, free thyroxine, thyroid-stimulating hormone, and vitamin D between the groups.
The binary logistic regression model included sex, age, BMI, education, MNA scores, and GDS scores (Figure 2). Results showed that older age was associated with increased of CF risk (OR 1.156, 95% CI 1.064–1.255), lower educational levels linked to higher CF risk (OR 0.115, 95% CI 0.024–0.550), declining MNA scores indicated greater CF risk (OR 0.713, 95% CI 0.522–0.973), and higher GDS scores correlated with increased CF risk (OR 1.345, 95% CI 1.065–1.699).
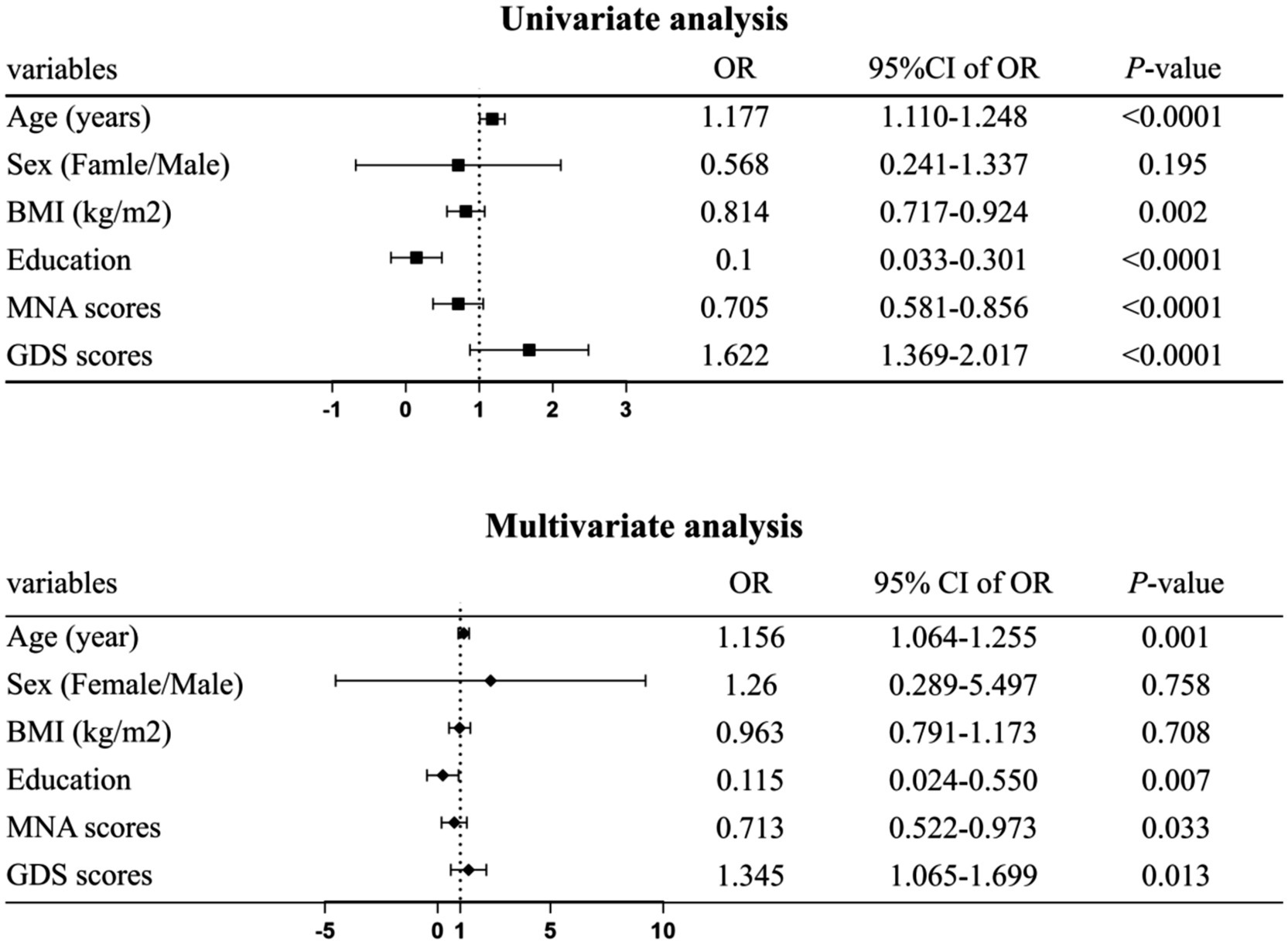
Figure 2. Forest plot factors significantly associated with cognitive frailty (CF). Data were analyzed using binary logistic regression. Adjusted for age, sex, body mass index (BMI), education level, mini nutritional assessment (MNA) scores, and geriatric depression scale (GDS) scores. Statistical significance was defined at p < 0.05.
3.3 Correlation between mitochondrial function and CF
Our results indicated that the CF group had lower mtDNAcn expression levels compared with the non-CF group (Figure 3A). Additionally, key mitochondrial respiratory function parameters—such as basal respiration, ATP-linked respiration, maximal respiration, and spare respiratory capacity—were significantly reduced in the CF group, while proton leak did not show significant differences between the groups (Figure 3B). ROS levels were higher in the CF group relative to the non-CF group (Figure 3C). Notably, although plasma levels of Carnitine Palmitoyltransferase II (CPT2) were elevated in the CF group, circulating levels of L-carnitine did not differ statistically (Figure 3D).
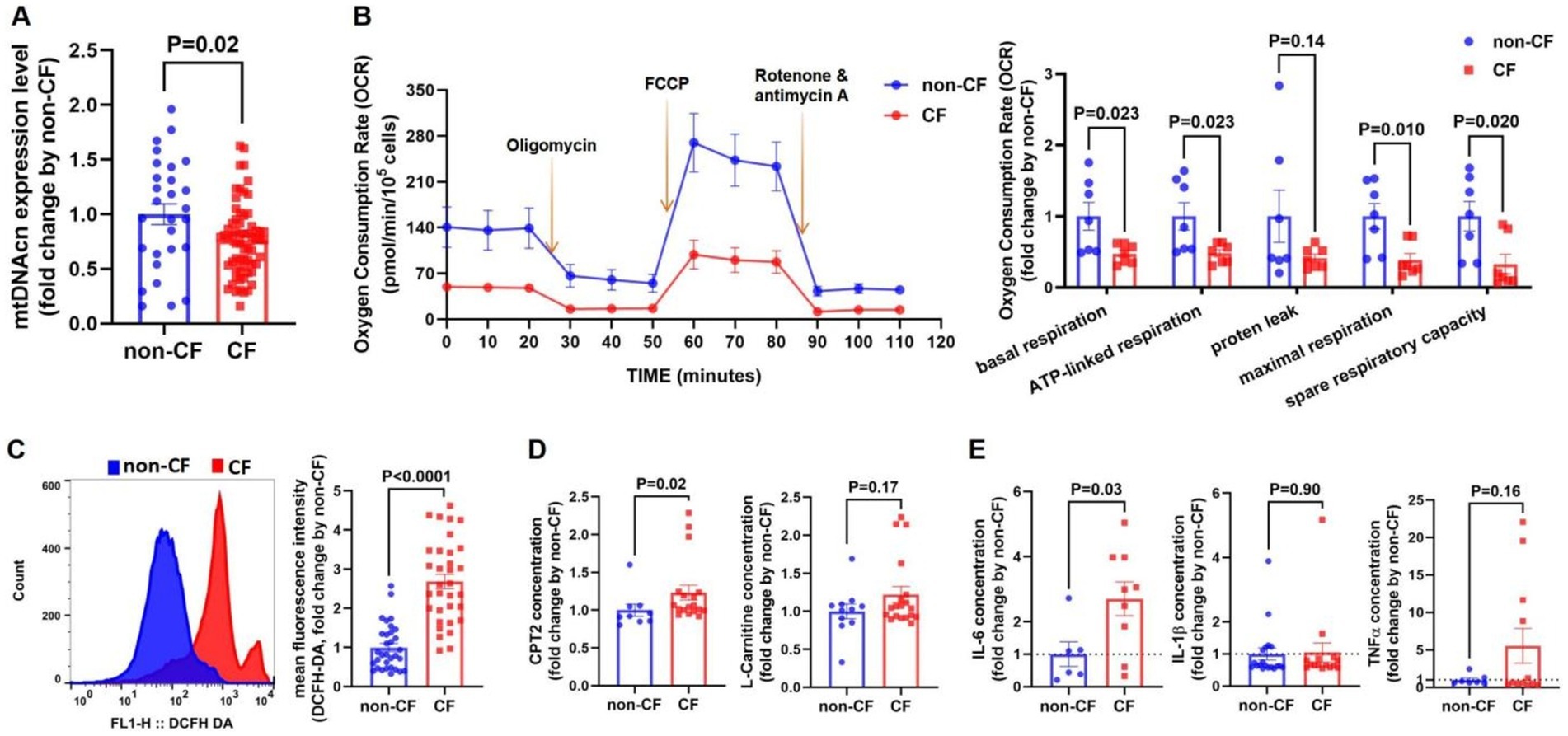
Figure 3. Mitochondrial dysfunction and inflammatory cytokines levels are increased in the cognitive frailty (CF) group. (A) qPCR was used to validate mitochondrial DNA copy number (mtDNAcn) levels in peripheral blood n (non-CF) = 28, n (CF) = 64. (B) Peripheral blood mononuclear cells (PBMCs) PBMCs underwent a mitochondrial stress test to measure oxygen consumption rate (OCR). Arrows indicate the addition of oligomycin, carbonyl cyanide-4 (trifluoromethoxy) phenylhydrazone (FCCP), and rotenone/antimycin A (left). Parameters analyzed included basal espiration, ATP-linked respiration, proten leak, maximal respiration, and spare respiratory capacity (right). n (non-CF) = 7, n (CF) = 7. (C) Flow cytometry analysis of DCFH-DA expression in PBMCs (left). Quantitative fluorescence analysis of DCFH-DA (right). n (non-CF) = 32, n (CF) = 33. (D) Secretion levels carnitine palmitoyltransferase 2 (CPT2) [n (non-CF) = 9, n (CF) = 18], and L-carnitine [n (non-CF) = 11, n (CF) = 19]. (E) Cytokines levels of interleukin-6 (IL-6) [n (non-CF) = 6, n (CF) = 9], interleukin-1 beta (IL-1β) [n (non-CF) = 7, n (CF) = 12] and tumor necrosis factor-α (TNF-α) [n (non-CF) = 19, n (CF) = 15] in plasma determined by ELISA. Notes: Differences in mitochondrial function between the two groups were illustrated using columnar scatter plots generated with GraphPad Prism software, while ROS levels were analyzed with FlowJo. Statistical comparisons were evaluated using Mann–Whitney U tests or Student’s t-tests, as appropriate. Data are presented as means ± standard error of the mean (SEM). Statistical significance was defined at p < 0.05.
After adjustment for age, sex, and BMI, compared with non-CF group, the OR values for the CF group of mtDNAcn and ROS were 0.234 (95% CI = 0.065–0.849) (p = 0.027) and 1.203 (95% CI = 1.075–1.347) (p = 0.001), respectively. Similar results were obtained in both the unadjusted and the age-sex-adjusted model (Table 2).
3.4 Correlation between inflammatory cytokines levels and CF
In addition, plasma IL-6 levels were elevated in the CF group compared with the non-CF group, while no significant differences were found for IL-1β or TNF-α levels between the groups (Figure 3E).
3.5 The diagnostic value of mtDNAcn and ROS for CF
The CF group was designated as the positive cohort, while the non-CF group served as the negative cohort for plotting the ROC curve. The analysis revealed that the area under curve (AUC) values for mtDNAcn and ROS in predicting CF were 0.653 and 0.925, respectively, as shown in Table 3 and Figure 4.
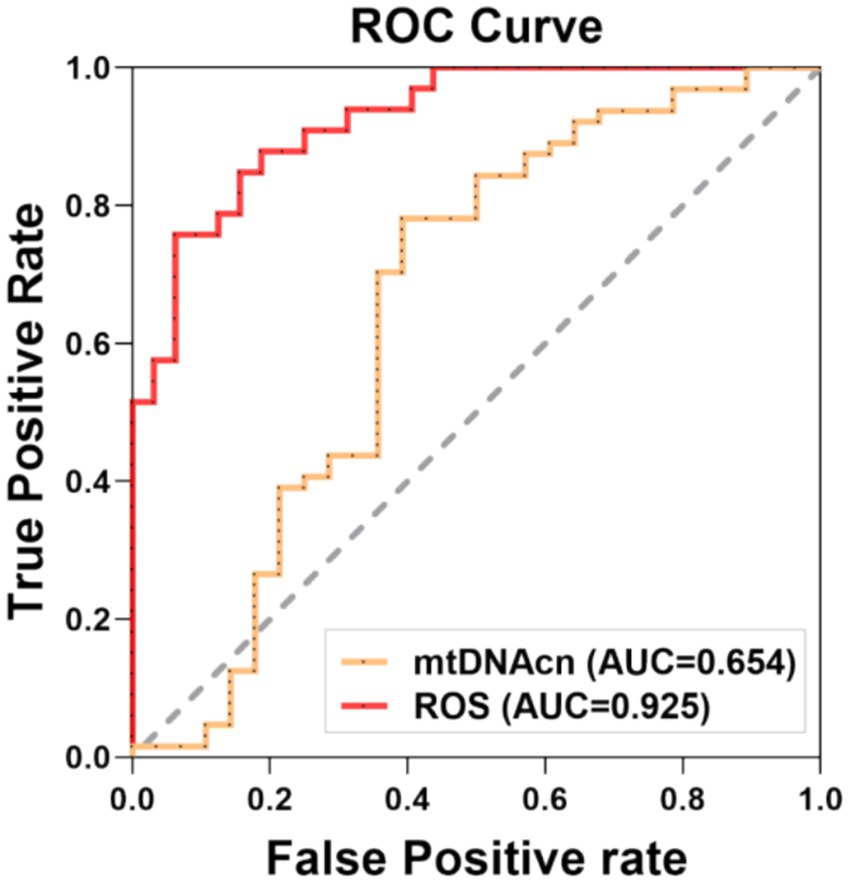
Figure 4. Receiver operating characteristic (ROC) curve for the prediction model. The sensitivity analysis was conducted by ROC curve, which was assessed the capacity of mtDNAcn and ROS to predictive CF, respectively. Statistical significance was defined at p < 0.05. AUC: area under the curve, mtDNAcn: mitochondrial DNA copy number, ROS: reactive oxygen species.
4 Discussion
This study identified a 32.26% prevalence of CF among inpatients. Our results showed that the CF group had a longer hospital length of stay compared with the non-CF group. The independent t tests, Mann–Whitney U tests and binary logistic regression suggested that notable risk factors of CF included advancing age, lower educational attainment, malnutrition, and depressive states. The study included 9 females (19%) in the non-CF group and 27 females (30%) in the CF group. While women were generally more frail, they appeared less susceptible to mortality than men (Arosio et al., 2023; Bai et al., 2023). To assess potential gender differences between the two groups, we performed a chi-square test (χ2 = 1.703, p = 0.192) and a multivariate logistic regression analysis (95% CI: 0.289–5.497, p = 0.758), both of which indicated no significant effect of gender on CF. We found that lower levels of insulin, triglycerides, albumin, retinol-binding protein, prealbumin, hemoglobin, and free triiodothyronine in the CF group. Sugimoto et al. also reported the risk factors of CF included sex, age, education level, socioeconomic status, malnutrition, and depressive states (Sugimoto et al., 2022). Moreover, multidomain lifestyle interventions for the older people, which incorporating exercise, nutritional support, and cognitive training, have proven effective in enhancing both physical frailty and cognitive function (Murukesu et al., 2020; Zhang et al., 2023b). Therefore, early detection, diagnosis, and intervention are essential for managing the onset and progression of CF.
Previous studies have utilized human peripheral blood samples as biomarkers for diagnosing various conditions, including heart failure, cognitive impairments, frailty, and dementia (Alexovič et al., 2022; Mahapatra et al., 2023; Lee et al., 2022). Furthermore, human PBMCs are widely recognized as critical targets for assessing mitochondrial function (Silaidos et al., 2018). CF, characterized as a geriatric syndrome affecting both physical and cognitive functions, is linked not only to mitochondrial dysfunction in specific tissues but also to systemic aging of tissues and organs (Robinson et al., 2022; Ruan et al., 2017; Zhang X. M. et al., 2022; Arosio et al., 2023; Ma and Chan, 2020). Consequently, to analyze the correlation between CF and mitochondrial function, we chose to assess mitochondrial function in peripheral blood cells rather than focusing on specific tissues such as skeletal muscle.
MtDNAcn quantifies mitochondrial content, influenced by various factors. Previous studies have linked elevated mtDNAcn levels in whole blood to an increased risk of glioma carcinogenesis, while lower mtDNAcn has been associated with greater susceptibility to aging and age-related diseases, such as coronary heart disease, cognitive impairments, and frailty (Shen et al., 2016; Wachsmuth et al., 2016; Mengel-From et al., 2014; Ashar et al., 2015; Klein et al., 2021; Yang et al., 2021). A review on aging and mtDNA suggested that most studies reported a decrease in mtDNAcn with aging (Filograna et al., 2021). Tian et al. demonstrated that higher mtDNAcn in human blood is associated with lower cognitive decline and frailty risk (Tian et al., 2024). However, a study reported that mtDNAcn increased with aging in liver samples (Wachsmuth et al., 2016). And another study showed no correlation between age and mitochondrial health index in PBMC (Karan et al., 2020). The variability in the relationship between age and mtDNAcn may be attributed to the heterogeneous composition of leukocytes and platelet abundance, which fluctuate with time of day, aging, and disease (Picard, 2021). In this study, the results demonstrated that the mtDNAcn in the CF group was lower than that in the non-CF group. After adjusted for age, sex, and BMI, our results still suggested that reduced mtDNAcn remained positively correlated with CF. Moreover, the Sensitivity analysis suggested that mtDNAcn could be a key parameter for evaluating CF. Therefore, low mtDNAcn level in blood is associated with CF and may serve as a characteristic feature of CF.
Mitochondrial respiration is essential for maintaining normal cellular function. Mitochondrial health is particularly crucial in age-related disorders, including cardiovascular and neurodegenerative diseases, which often stem from imbalances in energy supply and demand (Amorim et al., 2022). The brain, being a high-energy-demand organ, is especially vulnerable to deficits in oxygen and energy. Mitochondrial dysfunction can trigger pathological responses in neural tissues, leading to cognitive decline (Yin et al., 2016; Cunnane et al., 2020). Moreover, Mahapatra et al., 2023 confirmed a positive association between mitochondrial respiratory capacity in human PBMCs and both cognitive ability and hippocampal volume. Additionally, aging-related impairments in object recognition and spatial memory have been linked to reduced synaptic mitochondrial ATP production in the hippocampus (Olesen et al., 2020). Ultimately, a decrease in neural energy availability may contribute to cognitive decline through impaired neural structure and function (Cheng et al., 2022; Rangaraju et al., 2019). Our study indicated that the CF group exhibited lower basal respiration, ATP-linked respiration, maximal respiration, and spare respiratory capacity compared with the non-CF group. These findings suggest that impaired mitochondrial respiration is associated with CF.
Mitochondria are the primary intracellular source of ROS; and previous reviews have suggested a potential link between CF and increased oxidative stress due to mitochondrial dysfunction (Bertero and Maack, 2018; Zhang B. et al., 2022). While ROS generated by the mitochondrial respiratory chain are critical signaling molecules in healthy cells, their excessive production during oxidative stress can lead to mtDNA damage, mitochondrial dysfunction, and cell death (Zarse and Ristow, 2021). In general, age-related mitochondrial abnormalities, such as accumulation of mtDNA mutations, diminished respiratory chain activity, and increased ROS generation, are implicated in the aging process. Some reviews proposed that the balance between ROS generation and clearance were disturbed in aging and neurodegenerative diseases (Guo Y. et al., 2023; Ionescu-Tucker and Cotman, 2021). And in aging animal models, ROS production in synaptic mitochondria disrupts essential neuronal proteins, contributing to cognitive decline (Kron et al., 2020; Olesen et al., 2020). Our results showed that ROS levels in PBMCs were significantly higher in the CF group compared with that in the non-CF group. After adjusted for age and sex, we observed that ROS levels in the CF group were significantly higher than that in the non-CF group. Furthermore, our results demonstrated that ROS levels might be an important parameter to assess CF. Therefore, high levels of ROS is correlated with CF, and may serve as a critical feature of CF.
Cellular stress can alter membrane permeability, and mitochondrial damage and its byproducts may exacerbate inflammation (Picca et al., 2021; Vringer and Tait, 2023). Recent studies suggested that mitochondria modulate inflammation through signaling pathways involving mtDNA and ROS, which trigger the release of TNF-α and IL-1β, disrupting the balance between pro-inflammatory and anti-inflammatory factors (Victorelli et al., 2023; Jiménez-Loygorri and Boya, 2024; Marchi et al., 2023). Previous research had highlighted the role of IL-6 and other pro-inflammatory markers in aging and cognitive decline, supporting their potential as disease predictors (Weaver et al., 2002; Leonardo and Fregni, 2023; Lyra et al., 2021; Parks et al., 2020). Given these reports, we assessed inflammatory cytokines levels in the bloodstream and the results revealed that elevated plasma IL-6 levels were associated with CF.
This study has several limitations. Firstly, while CF should be defined as excluding dementia, our use of the PFP and MoCA scales as diagnostic tools, may have inadvertently included participants with early-stage dementia. Future follow-up studies are planned to more rigorously exclude cases of rapidly progressing dementia (Nasreddine et al., 2005; Malek-Ahmadi and Nikkhahmanesh, 2024). Additionally, there was a significant age difference between the two groups in our study. Although we re-analyzed the relationship between mitochondrial function and CF, it would be more rigorous to compare subjects within the same age range in both the non-CF and CF group. Next, other techniques for broader range of ROS assays is necessary to better understand the relationship between ROS and CF, such as spin trap compounds coupled with electron paramagnetic resonance spectroscopy, luminescence, mass spectrometry, and electrochemistry (Qu et al., 2020; Kornienko et al., 2018). Moreover, there was insufficient evidence to support the direct mechanistic roles of mitochondrial dysfunction, inflammation and CF in our study. Experimental studies should investigate mitochondria, metabolic and inflammatory alteration in the brain, such as metabolites levels in cerebrospinal fluid, PET brain glucose uptake, and magnetic resonance imaging. Additionally, the cross-sectional nature of this study limits our capacity to capture the temporal dynamics between mitochondrial function and CF progression. To address this, we will implement longitudinal follow-up assessments to better understand these relationships over time.
In summary, our findings highlight significant correlations between mitochondrial dysfunction and CF, suggesting that mitochondrial dysfunction in peripheral blood may serve as a potential phenotype of CF. These results underscore the need for further mechanistic studies to explore this relationship more comprehensively.
Data availability statement
The raw data supporting the conclusions of this article will be made available by the authors, without undue reservation.
Ethics statement
The studies involving humans were approved by the Ethical Committee of Jiangsu Province Hospital. The studies were conducted in accordance with the local legislation and institutional requirements. The participants provided their written informed consent to participate in this study.
Author contributions
LQ: Formal analysis, Investigation, Methodology, Writing – original draft, Writing – review & editing. TH: Formal analysis, Investigation, Methodology, Writing – original draft, Writing – review & editing. DZ: Formal analysis, Investigation, Methodology, Writing – original draft. LW: Data curation, Writing – original draft. GL: Data curation, Writing – original draft. QZ: Data curation, Writing – original draft. QT: Data curation, Writing – original draft. GD: Conceptualization, Project administration, Supervision, Writing – review & editing. JL: Conceptualization, Funding acquisition, Project administration, Supervision, Visualization, Writing – review & editing.
Funding
The author(s) declare that financial support was received for the research, authorship, and/or publication of this article. This study was supported by the National Natural Science Foundation of China (Nos. 81871106 and 82071584).
Acknowledgments
We extend our gratitude to the National Natural Science Foundation of China for their financial support under Grant Nos. 81871106 and 82071584. We also express our deep appreciation to all participants in this study.
Conflict of interest
The authors declare that the research was conducted in the absence of any commercial or financial relationships that could be construed as a potential conflict of interest.
Generative AI statement
The authors declare that no Generative AI was used in the creation of this manuscript.
Publisher’s note
All claims expressed in this article are solely those of the authors and do not necessarily represent those of their affiliated organizations, or those of the publisher, the editors and the reviewers. Any product that may be evaluated in this article, or claim that may be made by its manufacturer, is not guaranteed or endorsed by the publisher.
Abbreviations
A/G, Android-to-Gynoid; ALB, Albumin; ASMI, Appendicular Skeletal Muscle Mass Index; ATP, Adenosine Triphosphate; AUC, Area Under Curve; BMI, Body Mass Index; CF, Cognitive Frailty; CHD, Coronary Atherosclerotic Heart Disease; CI, Confidence Intervals; CIRS-CI, Cumulative Illness Rating Scale - Comorbidity Index; CPT2, Carnitine Palmitoyltransferase II; DCFH-DA, 2′,7′-Dichlorofluorescin diacetate; DMEM, Dulbecco's Modified Eagle Medium; DXA, Dual-energy X-ray absorptiometry; ELISA, Enzyme-Linked Immunosorbent Assay; FCCP, Carbonyl cyanide-4 (trifluoromethoxy) phenylhydrazone; FMI, Fat Mass Index; FT3, Free Triiodothyronine; FT4, Free Thyroxine; GDS, Geriatric Depression Scale; GLU, Glucose; HbA1c, Glycosylated Hemoglobin; HDL, High-density Lipoprotein; HGB, Hemoglobin; IL-1β, Interleukin-1beta; IL-6, Interleukin-6; INS, Insulin; LDL, Low-density lipoprotein; LOS, Length of Stay; MCI, Mild Cognitive Impairment; MFS, Morse Fall Scale; μM, Micromole; mM, Millimole; MNA, Mini Nutritional Assessment; MoCA, Montreal Cognitive Assessment; mtDNA, Mitochondrial DNA; mtDNAcn, Mitochondrial DNA Copy Number; OCR, Oxygen Consumption Rate; OR, Odds Ratio; PA, Prealbumin; PBMCs, Peripheral Blood Mononuclear Cells; PBS, Phosphate-Buffered Saline; PFP, Physical Frailty Phenotype; Q-Q, Quantile-Quantile; qRT-PCR, Quantitative Real-Time Polymerase Chain Reactions; RBP, Retinal Binding Protein; ROC, Receiver Operating Characteristic; ROS, Reactive Oxygen Species; CIRS-CI, Cumulative Illness Rating Scale - Comorbidity Index; SARC-calf, strength, assistance walking, rise from a chair, climb stairs, and falls combined with calf circumference; SAS, Self-Rating Anxiety Scale; SD, Standard Deviation; SEM, Standard Error of the Mean; SPPB, Short Physical Performance Battery; TC, Total Cholesterol; TG, Triglyceride; TNF-α, Tumor Necrosis Factor-alpha; TP, Total Protein; TRF, Transferrin; TSH, Thyrotrophin; TUG, Timed Up and Go test; VIT-D, 25-Hydroxyvitamin D; WHR, Waist-to-Hip Ratio
References
Alexovič, M., Lindner, J. R., Bober, P., Longuespée, R., Sabo, J., and Davalieva, K. (2022). Human peripheral blood mononuclear cells: a review of recent proteomic applications. Proteomics 22:e2200026. doi: 10.1002/pmic.202200026
Amorim, J. A., Coppotelli, G., Rolo, A. P., Palmeira, C. M., Ross, J. M., and Sinclair, D. A. (2022). Mitochondrial and metabolic dysfunction in ageing and age-related diseases. Nat. Rev. Endocrinol. 18, 243–258. doi: 10.1038/s41574-021-00626-7
Apaijai, N., Sriwichaiin, S., Phrommintikul, A., Jaiwongkam, T., Kerdphoo, S., Chansirikarnjana, S., et al. (2020). Cognitive impairment is associated with mitochondrial dysfunction in peripheral blood mononuclear cells of elderly population. Sci. Rep. 10:21400. doi: 10.1038/s41598-020-78551-4
Arosio, B., Calvani, R., Ferri, E., Coelho-Junior, H. J., Carandina, A., Campanelli, F., et al. (2023). Sarcopenia and cognitive decline in older adults: targeting the muscle-brain Axis. Nutrients 15:1853. doi: 10.3390/nu15081853
Ashar, F. N., Moes, A., Moore, A. Z., Grove, M. L., Chaves, P. H. M., Coresh, J., et al. (2015). Association of mitochondrial DNA levels with frailty and all-cause mortality. J. Mol. Med. 93, 177–186. doi: 10.1007/s00109-014-1233-3
Bertero, E., and Maack, C. (2018). Calcium signaling and reactive oxygen species in mitochondria. Circ. Res. 122, 1460–1478. doi: 10.1161/circresaha.118.310082
Bai, R., Liu, Y., Zhang, L., Dong, W., Bai, Z., Zhou, M., et al. (2023). Projections of future life expectancy in China up to 2035: a modelling study. Lancet Public Health, 8, e915–e922. doi: 10.1016/S2468-2667(22)00338-3
Cheng, X. T., Huang, N., and Sheng, Z. H. (2022). Programming axonal mitochondrial maintenance and bioenergetics in neurodegeneration and regeneration. Neuron 110, 1899–1923. doi: 10.1016/j.neuron.2022.03.015
Clegg, A., Young, J., Iliffe, S., Rikkert, M. O., and Rockwood, K. (2013). Frailty in elderly people. Lancet 381, 752–762. doi: 10.1016/s0140-6736(12)62167-9
Cunnane, S. C., Trushina, E., Morland, C., Prigione, A., Casadesus, G., Andrews, Z. B., et al. (2020). Brain energy rescue: an emerging therapeutic concept for neurodegenerative disorders of ageing. Nat. Rev. Drug Discov. 19, 609–633. doi: 10.1038/s41573-020-0072-x
Davies, G., Lam, M., Harris, S. E., Trampush, J. W., Luciano, M., Hill, W. D., et al. (2018). Study of 300, 486 individuals identifies 148 independent genetic loci influencing general cognitive function. Nat. Commun. 9:2098. doi: 10.1038/s41467-018-04362-x
Devine, M. J., and Kittler, J. T. (2018). Mitochondria at the neuronal presynapse in health and disease. Nat. Rev. Neurosci. 19, 63–80. doi: 10.1038/nrn.2017.170
Filograna, R., Mennuni, M., Alsina, D., and Larsson, N. G. (2021). Mitochondrial DNA copy number in human disease: the more the better? FEBS Lett. 595, 976–1002. doi: 10.1002/1873-3468.14021
Fried, L. P., Tangen, C. M., Walston, J., Newman, A. B., Hirsch, C., Gottdiener, J., et al. (2001). Frailty in older adults: evidence for a phenotype. J. Gerontol. A Biol. Sci. Med. Sci. 56, M146–M157. doi: 10.1093/gerona/56.3.m146
Guo, Y., Guan, T., Shafiq, K., Yu, Q., Jiao, X., Na, D., et al. (2023). Mitochondrial dysfunction in aging. Ageing Res. Rev. 88:101955. doi: 10.1016/j.arr.2023.101955
Guo, X., Pei, J., Ma, Y., Cui, Y., Guo, J., Wei, Y., et al. (2023). Cognitive frailty as a predictor of future falls in older adults: a systematic review and Meta-analysis. J. Am. Med. Dir. Assoc. 24, 38–47. doi: 10.1016/j.jamda.2022.10.011
Gutiérrez-Reguero, H., Buendía-Romero, Á., Franco-López, F., Martínez-Cava, A., Hernández-Belmonte, A., Courel-Ibáñez, J., et al. (2024). Effects of multicomponent training and HMB supplementation on disability, cognitive and physical function in institutionalized older adults aged over 70 years: a cluster-randomized controlled trial. J. Nutr. Health Aging 28:100208. doi: 10.1016/j.jnha.2024.100208
Holland, C., Dravecz, N., Owens, L., Benedetto, A., Dias, I., Gow, A., et al. (2024). Understanding exogenous factors and biological mechanisms for cognitive frailty: a multidisciplinary scoping review. Ageing Res. Rev. 101:102461. doi: 10.1016/j.arr.2024.102461
Hou, D., Sun, Y., Liu, Z., Sun, H., Li, Y., and Wang, R. (2024). A longitudinal study of factors associated with cognitive frailty in middle-aged and elderly population based on the health ecology model. J. Affect. Disord. 352, 410–418. doi: 10.1016/j.jad.2024.02.014
Ibrahim, A. M., Singh, D. K. A., Ludin, A. F. M., Subramaniam, P., Ai-Vyrn, C., Ibrahim, N., et al. (2024). Multidomain intervention for the reversal of cognitive frailty using a personalized approach (AGELESS trial): recruitment and baseline characteristics of participants. J. Prev. Alzheimers Dis. 11, 1291–1306. doi: 10.14283/jpad.2024.111
Ionescu-Tucker, A., and Cotman, C. W. (2021). Emerging roles of oxidative stress in brain aging and Alzheimer's disease. Neurobiol. Aging 107, 86–95. doi: 10.1016/j.neurobiolaging.2021.07.014
Jiayuan, Z., Xiang-Zi, J., Li-Na, M., Jin-Wei, Y., and Xue, Y. (2022). Effects of mindfulness-based tai chi Chuan on physical performance and cognitive function among cognitive frailty older adults: a six-month follow-up of a randomized controlled trial. J. Prev. Alzheimers Dis. 9, 1–9. doi: 10.14283/jpad.2021.40
Jiménez-Loygorri, J. I., and Boya, P. (2024). Aging STINGs: mitophagy at the crossroads of neuroinflammation. Autophagy 20, 1684–1686. doi: 10.1080/15548627.2024.2322421
Karan, K. R., Trumpff, C., McGill, M. A., Thomas, J. E., Sturm, G., Lauriola, V., et al. (2020). Mitochondrial respiratory capacity modulates LPS-induced inflammatory signatures in human blood. Brain Behav. Immun. Health 5:100080. doi: 10.1016/j.bbih.2020.100080
Kelaiditi, E., Cesari, M., Canevelli, M., van Kan, G. A., Ousset, P. J., Gillette-Guyonnet, S., et al. (2013). Cognitive frailty: rational and definition from an (I.A.N.A./I.A.G.G.) international consensus group. J. Nutr. Health Aging 17, 726–734. doi: 10.1007/s12603-013-0367-2
Klein, H. U., Trumpff, C., Yang, H. S., Lee, A. J., Picard, M., Bennett, D. A., et al. (2021). Characterization of mitochondrial DNA quantity and quality in the human aged and Alzheimer's disease brain. Mol. Neurodegener. 16:75. doi: 10.1186/s13024-021-00495-8
Kocagoncu, E., Nesbitt, D., Emery, T., Hughes, L. E., Henson, R. N., and Rowe, J. B. (2022). Neurophysiological and brain structural markers of cognitive frailty differ from Alzheimer's disease. J. Neurosci. 42, 1362–1373. doi: 10.1523/jneurosci.0697-21.2021
Kornienko, N., Zhang, J. Z., Sokol, K. P., Lamaison, S., Fantuzzi, A., van Grondelle, R., et al. (2018). Oxygenic Photoreactivity in photosystem II studied by rotating ring disk electrochemistry. J. Am. Chem. Soc. 140, 17923–17931. doi: 10.1021/jacs.8b08784
Kron, N. S., Schmale, M. C., and Fieber, L. A. (2020). Changes in metabolism and Proteostasis drive aging phenotype in Aplysia californica sensory neurons. Front. Aging Neurosci. 12:573764. doi: 10.3389/fnagi.2020.573764
Lee, C. S., Chang, C. H., Chen, C. Y., Shih, C. Y., Peng, J. K., Huang, H. L., et al. (2022). Upregulation of cluster of differentiation 36 mRNA expression in peripheral blood mononuclear cells correlates with frailty severity in older adults. J. Cachexia. Sarcopenia Muscle 13, 1948–1955. doi: 10.1002/jcsm.13003
Leonardo, S., and Fregni, F. (2023). Association of inflammation and cognition in the elderly: a systematic review and meta-analysis. Front. Aging Neurosci. 15:1069439. doi: 10.3389/fnagi.2023.1069439
Li, X., Zhang, Y., Tian, Y., Cheng, Q., Gao, Y., and Gao, M. (2022). Exercise interventions for older people with cognitive frailty-a scoping review. BMC Geriatr. 22:721. doi: 10.1186/s12877-022-03370-3
Liu, Z., Hsu, F. C., Trombetti, A., King, A. C., Liu, C. K., Manini, T. M., et al. (2018). Effect of 24-month physical activity on cognitive frailty and the role of inflammation: the LIFE randomized clinical trial. BMC Med. 16:185. doi: 10.1186/s12916-018-1174-8
Lyra, E. S. N. M., Gonçalves, R. A., Pascoal, T. A., Lima-Filho, R. A. S., Resende, E. P. F., Vieira, E. L. M., et al. (2021). Pro-inflammatory interleukin-6 signaling links cognitive impairments and peripheral metabolic alterations in Alzheimer's disease. Transl. Psychiatry 11:251. doi: 10.1038/s41398-021-01349-z
Ma, L., and Chan, P. (2020). Understanding the physiological links between physical frailty and cognitive decline. Aging Dis. 11, 405–418. doi: 10.14336/ad.2019.0521
Mahapatra, G., Gao, Z., Bateman, J. R. 3rd, Lockhart, S. N., Bergstrom, J., DeWitt, A. R., et al. (2023). Blood-based bioenergetic profiling reveals differences in mitochondrial function associated with cognitive performance and Alzheimer's disease. Alzheimers Dement. 19, 1466–1478. doi: 10.1002/alz.12731
Malek-Ahmadi, M., and Nikkhahmanesh, N. (2024). Meta-analysis of Montreal cognitive assessment diagnostic accuracy in amnestic mild cognitive impairment. Front. Psychol. 15:1369766. doi: 10.3389/fpsyg.2024.1369766
Marchi, S., Guilbaud, E., Tait, S. W. G., Yamazaki, T., and Galluzzi, L. (2023). Mitochondrial control of inflammation. Nat. Rev. Immunol. 23, 159–173. doi: 10.1038/s41577-022-00760-x
Mengel-From, J., Thinggaard, M., Dalgård, C., Kyvik, K. O., Christensen, K., and Christiansen, L. (2014). Mitochondrial DNA copy number in peripheral blood cells declines with age and is associated with general health among elderly. Hum. Genet. 133, 1149–1159. doi: 10.1007/s00439-014-1458-9
Murukesu, R. R., Singh, D. K. A., Shahar, S., and Subramaniam, P. (2020). A multi-domain intervention protocol for the potential reversal of cognitive frailty: "WE-RISE" randomized controlled trial. Front. Public Health 8:471. doi: 10.3389/fpubh.2020.00471
Nader, M. M., Cosarderelioglu, C., Miao, E., Whitson, H., Xue, Q. L., Grodstein, F., et al. (2023). Navigating and diagnosing cognitive frailty in research and clinical domains. Nat. Aging 3, 1325–1333. doi: 10.1038/s43587-023-00504-z
Nasreddine, Z. S., Phillips, N. A., Bédirian, V., Charbonneau, S., Whitehead, V., Collin, I., et al. (2005). The Montreal cognitive assessment, MoCA: a brief screening tool for mild cognitive impairment. J. Am. Geriatr. Soc. 53, 695–699. doi: 10.1111/j.1532-5415.2005.53221.x
Niu, J., Li, Y., Zhou, Q., Liu, X., Yu, P., Gao, F., et al. (2024). The association between physical activity and delayed neurocognitive recovery in elderly patients: a mediation analysis of pro-inflammatory cytokines. Aging Clin. Exp. Res. 36:192. doi: 10.1007/s40520-024-02846-z
Olesen, M. A., Torres, A. K., Jara, C., Murphy, M. P., and Tapia-Rojas, C. (2020). Premature synaptic mitochondrial dysfunction in the hippocampus during aging contributes to memory loss. Redox Biol. 34:101558. doi: 10.1016/j.redox.2020.101558
Panza, F., Lozupone, M., Solfrizzi, V., Sardone, R., Dibello, V., Di Lena, L., et al. (2018). Different cognitive frailty models and health-and cognitive-related outcomes in older age: From epidemiology to prevention. J. Alzheimers Dis. 62, 993–1012. doi: 10.3233/jad-170963
Parks, E. E., Logan, S., Yeganeh, A., Farley, J. A., Owen, D. B., and Sonntag, W. E. (2020). Interleukin 6 reduces allopregnanolone synthesis in the brain and contributes to age-related cognitive decline in mice. J. Lipid Res. 61, 1308–1319. doi: 10.1194/jlr.RA119000479
Picard, M. (2021). Blood mitochondrial DNA copy number: what are we counting? Mitochondrion 60, 1–11. doi: 10.1016/j.mito.2021.06.010
Picca, A., Calvani, R., Coelho-Junior, H. J., and Marzetti, E. (2021). Cell death and inflammation: the role of mitochondria in health and disease. Cells 10:537. doi: 10.3390/cells10030537
United Nations Department of Economic and Social Affairs, Population Division. (2024). World Population Prospects 2024: Summary of Results (UN DESA/POP/2024/TR/NO. 9). Available at: https://www.un.org/development/desa/pd/sites/www.un.org.development.desa.pd/files/files/documents/2024/Jul/wpp2024summaryofresultsfinal_web.pdf (accessed Oct 1, 2024).
Qiu, Y., Li, G., Zheng, L., Liu, W., Li, X., Wang, X., et al. (2023). Relationship between cognitive frailty and mortality in older adults: a systematic review and Meta-analysis. J. Am. Med. Dir. Assoc. 24, 1637–1638. doi: 10.1016/j.jamda.2023.08.001
Qu, F., Wang, P., Zhang, K., Shi, Y., Li, Y., Li, C., et al. (2020). Manipulation of Mitophagy by "all-in-one" nanosensitizer augments sonodynamic glioma therapy. Autophagy 16, 1413–1435. doi: 10.1080/15548627.2019.1687210
Rangaraju, V., Lewis, T. L. Jr., Hirabayashi, Y., Bergami, M., Motori, E., Cartoni, R., et al. (2019). Pleiotropic mitochondria: the influence of mitochondria on neuronal development and disease. J. Neurosci. 39, 8200–8208. doi: 10.1523/jneurosci.1157-19.2019
Rivan, N. F. M., Singh, D. K. A., Shahar, S., Wen, G. J., Rajab, N. F., Din, N. C., et al. (2021). Cognitive frailty is a robust predictor of falls, injuries, and disability among community-dwelling older adults. BMC Geriatr. 21:593. doi: 10.1186/s12877-021-02525-y
Robinson, T. L., Gogniat, M. A., and Miller, L. S. (2022). Frailty and cognitive function in older adults: a systematic review and Meta-analysis of cross-sectional studies. Neuropsychol. Rev. 32, 274–293. doi: 10.1007/s11065-021-09497-1
Ruan, Q., D'Onofrio, G., Sancarlo, D., Greco, A., Lozupone, M., Seripa, D., et al. (2017). Emerging biomarkers and screening for cognitive frailty. Aging Clin. Exp. Res. 29, 1075–1086. doi: 10.1007/s40520-017-0741-8
Shen, J., Song, R., Lu, Z., and Zhao, H. (2016). Mitochondrial DNA copy number in whole blood and glioma risk: a case control study. Mol. Carcinog. 55, 2089–2094. doi: 10.1002/mc.22453
Silaidos, C., Pilatus, U., Grewal, R., Matura, S., Lienerth, B., Pantel, J., et al. (2018). Sex-associated differences in mitochondrial function in human peripheral blood mononuclear cells (PBMCs) and brain. Biol. Sex Differ. 9:34. doi: 10.1186/s13293-018-0193-7
Su, X., Wu, Z., Mai, F., Fan, Z., Du, S., Qian, H., et al. (2019). 'Governor vessel-unblocking and mind-regulating' acupuncture therapy ameliorates cognitive dysfunction in a rat model of middle cerebral artery occlusion. Int. J. Mol. Med. 43, 221–232. doi: 10.3892/ijmm.2018.3981
Sugimoto, T., Arai, H., and Sakurai, T. (2022). An update on cognitive frailty: its definition, impact, associated factors and underlying mechanisms, and interventions. Geriatr Gerontol Int 22, 99–109. doi: 10.1111/ggi.14322
Tam, A. C. Y., Chan, A. W. Y., Cheung, D. S. K., Ho, L. Y. W., Tang, A. S. K., Christensen, M., et al. (2022). The effects of interventions to enhance cognitive and physical functions in older people with cognitive frailty: a systematic review and meta-analysis. Eur. Rev. Aging Phys. Act. 19:19. doi: 10.1186/s11556-022-00299-9
Tang, K. F., Teh, P. L., and Lee, S. W. H. (2023). Cognitive frailty and functional disability among community-dwelling older adults: a systematic review. Innov. Aging 7:igad005. doi: 10.1093/geroni/igad005
Tian, Q., Zweibaum, D. A., Qian, Y., Oppong, R. F., Pilling, L. C., Casanova, F., et al. (2024). Mitochondrial DNA copy number associated dementia risk by somatic mutations and frailty. Geroscience. doi: 10.1007/s11357-024-01355-1
Vargas-Torres-Young, D. A., Salazar-Talla, L., Cuba-Ruiz, S., Urrunaga-Pastor, D., Runzer-Colmenares, F. M., and Parodi, J. F. (2022). Cognitive frailty as a predictor of mortality in older adults: a longitudinal study in Peru. Front. Med. 9:910005. doi: 10.3389/fmed.2022.910005
Victorelli, S., Salmonowicz, H., Chapman, J., Martini, H., Vizioli, M. G., Riley, J. S., et al. (2023). Apoptotic stress causes mtDNA release during senescence and drives the SASP. Nature 622, 627–636. doi: 10.1038/s41586-023-06621-4
Vringer, E., and Tait, S. W. G. (2023). Mitochondria and cell death-associated inflammation. Cell Death Differ. 30, 304–312. doi: 10.1038/s41418-022-01094-w
Wachsmuth, M., Hübner, A., Li, M., Madea, B., and Stoneking, M. (2016). Age-related and Heteroplasmy-related variation in human mtDNA copy number. PLoS Genet. 12:e1005939. doi: 10.1371/journal.pgen.1005939
Weaver, J. D., Huang, M. H., Albert, M., Harris, T., Rowe, J. W., and Seeman, T. E. (2002). Interleukin-6 and risk of cognitive decline: MacArthur studies of successful aging. Neurology 59, 371–378. doi: 10.1212/wnl.59.3.371
Xuekelati, S., Maimaitiwusiman, Z., Bai, X., Xiang, H., Li, Y., and Wang, H. (2024). Sarcopenia is associated with hypomethylation of TWEAK and increased plasma levels of TWEAK and its downstream inflammatory factor TNF-α in older adults: a case-control study. Exp. Gerontol. 188:112390. doi: 10.1016/j.exger.2024.112390
Yang, S. Y., Castellani, C. A., Longchamps, R. J., Pillalamarri, V. K., O'Rourke, B., Guallar, E., et al. (2021). Blood-derived mitochondrial DNA copy number is associated with gene expression across multiple tissues and is predictive for incident neurodegenerative disease. Genome Res. 31, 349–358. doi: 10.1101/gr.269381.120
Yin, F., Sancheti, H., Patil, I., and Cadenas, E. (2016). Energy metabolism and inflammation in brain aging and Alzheimer's disease. Free Radic. Biol. Med. 100, 108–122. doi: 10.1016/j.freeradbiomed.2016.04.200
Zarse, K., and Ristow, M. (2021). Mitochondrial ROS signals prevent excessive immune response. Nat. Metab. 3, 588–589. doi: 10.1038/s42255-021-00387-7
Zhang, Y., Liu, X., Wiggins, K. L., Kurniansyah, N., Guo, X., Rodrigue, A. L., et al. (2023a). Association of Mitochondrial DNA copy number with brain MRI markers and cognitive function: a meta-analysis of community-based cohorts. Neurology 100, e1930–e1943. doi: 10.1212/wnl.0000000000207157
Zhang, B., Pan, C., Feng, C., Yan, C., Yu, Y., Chen, Z., et al. (2022). Role of mitochondrial reactive oxygen species in homeostasis regulation. Redox Rep. 27, 45–52. doi: 10.1080/13510002.2022.2046423
Zhang, X. M., Wu, X. J., Cao, J., Jiao, J., and Chen, W. (2022). Association between cognitive frailty and adverse outcomes among older adults: a Meta-analysis. J. Nutr. Health Aging 26, 817–825. doi: 10.1007/s12603-022-1833-5
Zhang, Y., Zhou, J. J., Zhang, X. M., Liu, J. T., Li, M. R., Liang, J. Y., et al. (2023b). Management of cognitive frailty: a network meta-analysis of randomized controlled trials. Int. J. Geriatr. Psychiatry 38:e5994. doi: 10.1002/gps.5994
Keywords: aging, cognitive frailty, mitochondrial function, peripheral blood cells, risk factors
Citation: Qin L, Huang T, Zhang D, Wei L, Li G, Zhu Q, Tong Q, Ding G and Liu J (2024) The mitochondrial function of peripheral blood cells in cognitive frailty patients. Front. Aging Neurosci. 16:1503246. doi: 10.3389/fnagi.2024.1503246
Edited by:
Takao Yamasaki, Minkodo Minohara Hospital, JapanReviewed by:
María J. Morán, Research Institute Hospital 12 de Octubre, SpainGargi Mahapatra, University of California San Diego, United States
Copyright © 2024 Qin, Huang, Zhang, Wei, Li, Zhu, Tong, Ding and Liu. This is an open-access article distributed under the terms of the Creative Commons Attribution License (CC BY). The use, distribution or reproduction in other forums is permitted, provided the original author(s) and the copyright owner(s) are credited and that the original publication in this journal is cited, in accordance with accepted academic practice. No use, distribution or reproduction is permitted which does not comply with these terms.
*Correspondence: Juan Liu, bGlvdWphbmVAbmptdS5lZHUuY24=; Guoxian Ding, ZGluZ2d4QG5qbXUuZWR1LmNu
†These authors have contributed equally to this work
‡ORCID: Li Qin, orcid.org/0009-0002-9892-1002
Tingting Huang, orcid.org/0009-0006-8649-8277