- Department of Health Statistics, School of Public Health, Shanxi Medical University, Taiyuan, Shanxi, China
Objectives: Few studies have examined the underlying mechanisms of education, diets, and cognitive function in older adults. This study analyses the relationship between cognitive trajectories, education, and different dietary patterns in older adults from a network perspective, and further explores their longitudinal associations and mediation effects.
Methods: Data on cognitive trajectories were derived from the Chinese Longitudinal Healthy Longevity Survey (CLHLS) between 2008 and 2018. Group-Based Trajectory Model (GBTM) was used to identify potential heterogeneity in the longitudinal changes in cognitive function. Multinomial logistic regression and network analysis were then applied to examine the relationships between different cognitive trajectories and years of education, food variety (FV), and plant-based dietary patterns. Cross-lagged panel model was used to examine the longitudinal associations between education, FV, and plant-based diet patterns. Furthermore, we constructed a mediation model based on categorical variables for cognitive trajectories to investigate the mediating effect of FV and plant-based diet index on education and cognitive.
Results: A total of 2,115 older adults were included in this study, revealing three distinct cognitive function trajectories. After controlling for potential confounders, education and dietary-related variables were associated with a cognitive stable decline trajectory (OR: 0.857/0.929/1.027) and a cognitive rapid decline trajectory (OR: 0.859/0.914, 95% CI: 0.775–0.882) compared to the cognitive stable trajectory. In the education, diet, and cognition network model, overall Plant-Based Diet Index (PDI) [expected influence (EI) = 1.82] and years of education (EI = 0.54) were the most central domains. There were longitudinal associations between education, FV, and plant-based dietary patterns, which were significant only in the slow decline group. FV acting as a mediator between education and cognitive trajectories.
Conclusion: Years of education are longitudinally associated with the diet of older adults in the slow cognitive decline group. Food diversity partially mediates the relationship between years of education and cognitive trajectories. Interventions targeting education and dietary behaviors may help alleviate cognitive decline in older adults.
1 Introduction
With the aging of society—globally—the world faces a formidable challenge. The latest census data in China reveals that the population of adults 65 years old and above has soared to 190 million, constituting 13.5% of the overall population, signifying China's formal entry into the advanced stage of population aging. It follows that, the incidence, prevalence, and mortality rates of age-related diseases also increases, especially neurodegenerative diseases such as dementia, which severely affects the health and wellbeing of older adults (Ministry of Civil Affairs, People's Republic of China, 2022). Dementia is a chronic and progressive neurological disorder marked by a progressive decline in cognitive abilities, with particularly pronounced impairments in memory, language, judgment, attention, and executive functioning. The number of persons who are 60 years of age and older with dementia in China has reached 15.07 million, accounting for 25.5% of the 49 global patient count (Rujing et al., 2021). Each year, Alzheimer's disease, the most common cause of dementia, imposes an economic burden of 167.7 billion 50 US dollars (Jia et al., 2020), exerting tremendous pressure on families and society. The future social challenges will likely increase in level and cost (Boss et al., 2015). Therefore, adopting proactive and effective strategies to prevent dementia or to identify and intervene at an early stage to delay its progression is crucial for improving the quality of life for older adults in their later years and for reducing the socio-economic burden.
Cognitive decline is a precursor to dementia, and intervention during this stage provides an opportunity to prevent dementia (Petersen et al., 1999). Therefore, timely detection of cognitive decline in older adults, identification of modifiable risk factors is crucial for preventing the onset and progression of dementia. However, cognitive decline is a dynamic process that can worsen or reverse over time (Malek-Ahmadi, 2016). Relevant research indicated that the trajectory of cognitive decline varies around the population mean trajectory, highlighting the intra-individual and inter-individual heterogeneity in cognitive function changes (Li et al., 2017; Min, 2018). Ignoring the differences in cognitive trajectories and treating all study subjects uniformly could potentially affect the effectiveness of interventions. By discussing risk factors in a stratified manner based on these trajectories, we can gain a comprehensive understanding of cognitive changes in different population groups, thereby providing targeted interventions.
Increasing evidence suggests that education and diet play crucial roles in the development of cognitive impairment. Higher education levels are associated with slower cognitive decline (Marioni et al., 2012). Education is one of the three proxies for cognitive reserve (i.e., education, occupation, leisure activities) (Nucci et al., 2012) and the only factor linked to the volume of both gray and white matter in the brain (Foubert-Samier et al., 2012), further highlighting the extensive impact of education on brain function. Healthy dietary patterns and adequate nutrient intake are associated with healthy cognition (Berendsen et al., 2017; Tangney et al., 2014; Wengreen et al., 2013; Haring et al., 2016; Smyth et al., 2015; Chen et al., 2017; Neelakantan et al., 2018). Some interventions focusing on specific dietary patterns, nutrients, and nutritional supplements for the older people have shown success, indicating that nutrition is a modifiable factor for the older people (Jennings et al., 2019; Iuliano et al., 2021; Lin et al., 2021; Nakazaki et al., 2021).
Furthermore, multiple studies have shown that individuals with higher levels of education are more likely to maintain healthier dietary patterns (Seow et al., 1998; Loughrey et al., 2017; Thorpe et al., 2019). As mentioned earlier, healthy diets and adequate nutrient intake are linked to cognitive health. Therefore, dietary patterns and nutrient intake are likely mediators in the relationship between education and cognitive function.
However, there is limited evidence on the potential mediating role of diet in the relationship between education and cognitive function. To address this knowledge gap, we assess nutritional intake and dietary patterns in older adults using food variety (FV) and plant-based dietary patterns, and evaluate educational levels using years of education. Based on multi-wave follow-up data from the Chinese Longitudinal Healthy Longevity Survey (CLHLS) from 2008 to 2018, this study investigates the longitudinal association and mediating effect between education, diet, and cognitive function changes, stratified by cognitive trajectories. The aim is to better tailor interventions to specific populations to achieve more effective outcomes.
2 Methods
2.1 Data source
The data for this study were sourced from the CLHLS, which commenced in 1998 with follow-up surveys conducted every 3–4 years. The CLHLS survey spans 23 provinces in China and includes face-to-face household interviews with people aged 65 and older. All participants provided informed consent, with family members consenting for those unable to sign themselves (Zhang and Liu, 2007). Detailed information on the survey's sampling procedure and data quality is available in previous studies (Zeng et al., 2017). The survey has received approval from the Biomedical Ethics Committee of Peking University (IRB00001052-13074). Our team was granted data usage rights from the Peking University Open Research Data Platform.
2.2 Participants
This study used data from four waves of the CLHLS conducted between 2008 and 2018. Participants were older adults who completed cognitive function assessments in all four surveys. Samples missing dietary information or covariates at baseline and follow-ups were excluded, resulting in 2,115 participants. The selection process is shown in Supplementary Figure S1.
2.3 Food variety measurement
Food variety (FV) is a key factor in dietary quality and nutritional adequacy (Kennedy, 2009), ensuring a rich supply of all food groups as well as a wide range of macro and micronutrients (Kennedy et al., 2011). In this study, the Food Variety Score (FVS) was used to assess the food variety consumed by older adults from 2008 to 2018. The FVS was calculated based on the frequency of consumption of 13 specific food types included in the CLHLS questionnaire. These food types are: fresh fruits, fresh vegetables, meat, fish, eggs, legumes, preserved vegetables, sugar, garlic, dairy products, nuts, mushrooms, and tea. For fruit and vegetable consumption, respondents selected from four frequency options: daily, often, occasionally, or almost never. A score of 1 was assigned for “daily” or “often” consumption, while a score of 0 was assigned for “occasionally” or “rarely.” For the remaining 11 food items, consumption frequency was categorized into five options: daily, weekly, monthly, occasionally, and almost never. Again, a score of 1 was assigned for “daily” or “weekly” consumption, and a score of 0 was assigned for “monthly,” “occasionally,” or “almost never.” Each participant's final FVS was obtained by summing the scores across all food categories, yielding a range from 0 to 13. A higher score indicates a more diverse diet (Zhang et al., 2022).
2.4 Calculation of plant-based diet indices
Instead of focusing on individual foods or nutrients, overall dietary patterns consider the combined effects of multiple foods and nutrients. Given that plant-based diets align well with Asian dietary habits, this study used Plant-Based Diet Indices to assess older adults' dietary patterns. The dietary information for calculating the plant-based diet indices was primarily obtained through a simplified Food Frequency Questionnaire (FFQ), and these data were used to compute the plant-based diet scores. Initially, based on the nature of the foods, 16 common foods from the Chinese daily diet were grouped into three categories [in contrast to food diversity, the plant-based dietary pattern also considers the intake of grains (divided into whole grains and refined grains) and edible oil]: (1) healthful plant-based foods (whole grains, fresh fruits, fresh vegetables, legumes, garlic, vegetable oils, nuts, and tea), (2) unhealthful plant-based foods [refined grains, preserved vegetables, and sugar (white granulated sugar or candies)], and (3) animal-based foods (animal fat, eggs, fish and aquatic products, meat, and milk and dairy products). The questionnaire offered five response options for legumes, garlic, nuts, tea, salted preserved vegetables, sugar, eggs, fish, meat, and milk: “almost every day,” “≥1 time per week,” “≥1 time per month,” “occasionally,” and “rarely or never.” For whole grains, refined grains, vegetable oils, and animal fats, participants could respond with either “yes” or “no.” For fruits and fresh vegetables, the available choices were “almost every day,” “quite often,” “occasionally,” or “rarely or never.”
Using self-reported dietary frequencies, we calculated three plant-based diet indices: the overall Plant-Based Diet Index (PDI), the healthful Plant-Based Diet Index (hPDI), and the unhealthful Plant-Based Diet Index (uPDI). The consumption of each food was taken into account when calculating these indices. In line with previous studies (Satija et al., 2016, 2019, 2017), each food was assigned a score ranging from 1 to 5, with the specific focus of each index varying slightly. For the PDI, positive scores were assigned to plant-based food groups (with 1 indicating the least frequent consumption and 5 the most frequent), while animal-based food groups received reverse scores (fivee for the least frequent and one for the most frequent consumption). For the hPDI, healthful plant-based foods were scored positively, whereas unhealthful plant-based foods and animal-based foods were given reverse scores. In the case of the uPDI, positive scores were assigned to unhealthful plant-based foods, while both healthful plant-based foods and animal-based foods received reverse scores. The PDI, hPDI, and uPDI scores were calculated by summing the scores for each of the 16 food groups, with a theoretical range from 16 to 80.
2.5 Cognitive and education assessment
The CLHLS measured global cognitive function with the Chinese version of Mini-Mental State Examination (MMSE). The scale comprises 24 items, yielding a maximum score of 30 points, where higher scores indicate better cognitive function. It focuses on five primary domains: general ability, responsiveness, attention and calculation ability, recollection, language comprehension, and self-coordination. The validity and reliability of the Chinese MMSE have been verified (Chan et al., 2002; Lök et al., 2019).
The assessment of education level in the elderly population was primarily based on self-reported years of schooling.
2.6 Covariates
This study included demographic characteristics (gender, age, region, marital status, living conditions), socioeconomic status (Household income, wealth status, and health insurance coverage), and lifestyle factors (smoking, drinking, Exercise and BMI.) as covariates (Supplementary material).
2.7 Statistical analysis
Categorical variables were analyzed using frequencies (%) and continuous variables using means (SD). To study the cognitive trajectories of older adults, we employed a Group-Based Trajectory Model (GBTM) (Nagin, 1999). Additionally, a Mixed Graph Model (MGM) (Haslbeck and Waldorp, 2015) was utilized to construct a network model to explore the interconnections between education, dietary patterns, and cognitive trajectories. The Expected Influence (EI) metric was used to assess the core domains within the education, diet, and cognition network, with higher EI values indicating a stronger influence of a node on other nodes within the network. Detailed descriptions of the GBTM and network analysis methods are provided in the Supplementary material. To enhance the reliability of the network results, we conducted two sensitivity network analyses while controlling for potential confounding factors.
To examine the longitudinal associations between baseline education and follow-up FV, PDI, hPDI, and uPDI, we employed a cross-lagged Structural Equation Model (SEM) (Hamaker et al., 2015). This model simultaneously estimates multiple regression equations and assesses the directionality of associations while adjusting for potential confounders.
Finally, we analyzed the mediating mechanisms between education, diet, and cognitive trajectories using mediation analysis methods suited for categorical dependent variables. We adjusted the model linking the outcome variable (Y), mediator (M), and independent variable (X) from linear regression to cumulative logistic regression to accommodate the multicategorical nature of our outcome data. The mediating effect was tested using the product of coefficients method. Detailed descriptions of the methodology can be found in the referenced literature (Iacobucci, 2012).
All statistical analyses were performed using R 4.4.0 (R Core Team, Vienna, Austria). Two-sided p-values < 0.05 were considered statistically significant.
3 Results
3.1 Basic characteristics of different cognitive trajectories
Based on the selection criteria in Supplementary Table S2 and the cognitive trajectory curves in Supplementary Figure S3, we ultimately identified three cognitive trajectories: “High Stability” with 1,102 individuals (52.1%), “Slow Decline” with 710 individuals (33.3%), and “Rapid Decline” with 303 individuals (14.3%). Table 1 displayed the basic characteristics of the population in each cognitive trajectory group. In comparison to the other two cognitive trajectories, older adults in the “Rapid Decline” group were likely to be older, female, unmarried, not wealthy, never smokers, never drinkers, never exercisers, underweight, have lower years of education, have more children, lower PDI, hPDI, FV, and higher uPDI. Details on the whole sample can be found in Supplementary Table S1 and Supplementary Figure S2.
In the multinomial logistic regression analysis with the “High Stability” group as the reference, age (OR: 1.063/1.174), gender (OR: 0.532/0.506), years of education (OR: 0.857/0.829), and FV (OR: 0.929/0.914) were common determinants for both the “Slow Decline” and “Rapid Decline” groups. uPDI (OR: 1.501) and formerly drinking (OR: 1.027) were determinants for the “Slow Decline” group. See Figure 1 for details.
3.2 Network analysis results
Figure 2 illustrated the network structure and centrality of education, diet, and cognitive function in older adults (primary network model). PDI (EI = 1.85) and education (EI = 0.54) emerged as the most central domains within the education, diet, and cognition model. Positive correlations were observed between cognition and education (edge weight > 0), as well as between both education and cognition with FV (edge weight > 0). In the sensitivity network analyses presented in Supplementary Figures S4, S5, even after adjusting for covariates affecting the relationships among education, diet, and cognition, the central domains remained consistent with those found in the primary network analysis. The stability and accuracy of the primary network analysis are supported by Supplementary Figure S6, which shows a cs-coefficient for EI of 0.75, indicating stable and accurate centrality values. Supplementary Figure S7 demonstrates the reliability and stability of the edges through the bootstrap range of the estimated edge weights' 95% confidence intervals. Supplementary Figures S8, S9 highlight significant differences in EI and edge weights within the network model.
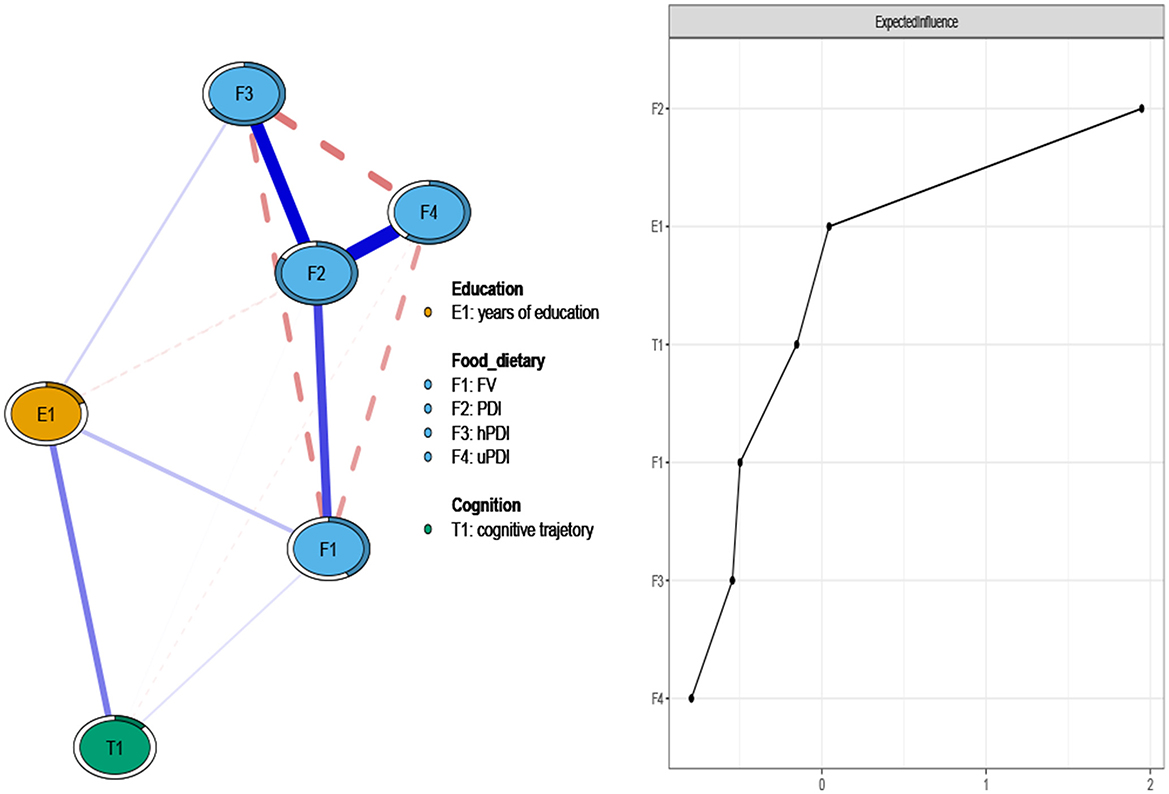
Figure 2. Network analysis of education, diets, and cognition in older adults (primary network analysis). The cognitive trajectory groups in our study are defined as follows: high stability group = 3, slow decline group = 2, and rapid decline group = 1. By default, higher values indicate more stable cognitive function.
3.3 Association between cognitive trajectories and education years and diet
Supplementary Table S3 presents the unadjusted and adjusted estimates of the association between years of education, diet-related scores, and cognitive function from the multinomial logistic regression analysis. In Model 1, while without adjusting for any covariates, individuals in the “Slow Decline” group had a reduced chance of cognitive decline if they had higher FV scores and more years of education, as well as lower uPDI scores, compared to those in the “High Stability” group. Similarly, individuals in the “Rapid Decline Class” who had higher FV scores, PDI scores, hPDI scores, and more years of education had a reduced chance of cognitive decline compared to those in the “High Stability” group. In Model 2 and 3, after controlling for age, gender, region, marital, econ state, living conditions, children number (Model 2), Smoke, Drink, Exercise and BMI (Model 3), the associations between FV, years of education, and cognitive trajectories remained unchanged for both the “Slow Decline” and the “Rapid Decline” compared to the “High Stability”.
3.4 Longitudinal association between education, diet and cognition
Figure 3 illustrated the path relationship between years of education and FV among the older adults. It is evident that baseline years of education were associated with baseline FV (r1 = 2.08, P < 0.001), baseline years of education were associated with follow-up years of education (β1 = 0.84, P < 0.001), and baseline FV was associated with follow-up FV (β2 = 0.19, P < 0.001), indicating a certain level of stability in education years and FV over 10 years. After adjusting for gender, age, marital, econ state, smoking, drinking, and exercise, the path coefficient from baseline FV to follow-up years of education was not statistically significant (ρ1 = 0.003, P > 0.05), while the path coefficient from baseline years of education to follow-up FV was statistically significant (ρ2 = 0.009, P < 0.001). These results suggest a unidirectional causal relationship between years of education and FV.
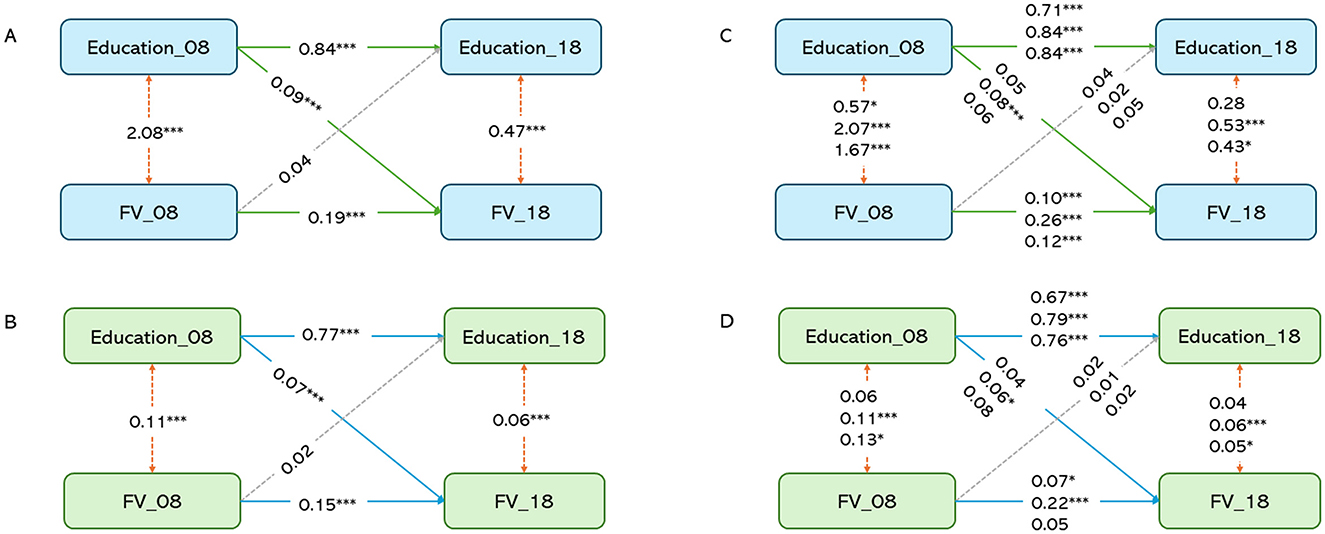
Figure 3. Cross lagged model diagram between education and FV. (A) Unadjusted and stratified original model; (B) covariate-adjusted model; (C) coarse model stratified according to trajectory group; (D) covariate-adjusted and trajectory group stratified model. *P < 0.05, **P < 0.01, ***P < 0.001.
Further stratification based on 10-year cognitive trajectories revealed that after adjusting for covariates, the association between baseline years of education and follow-up FV was significant only in the “Stable Decline”. This finding indicates that the influence of education on FV is primarily observed among older people experiencing a slow decline cognitive trajectory.
Based on the above analytical steps, we further examined the longitudinal associations between years of education and three dietary patterns (PDI, hPDI, and uPDI) as well as cognitive. Similar to the findings for FV, years of education showed significant temporal associations with PDI, hPDI, and cognition in both the overall unstratified model and the stratified slow decline cognitive trajectory. However, the association with uPDI was not significant, indicating no temporal relationship between baseline years of education and follow-up uPDI. Detailed results can be found in Supplementary Figures S10–S13.
3.5 Mediating effect
Based on the above research results, to verify the mediating effect of diet on the relationship between years of education and changes in cognitive function in the older adults, we further conducted a mediation analysis with baseline years of education as the independent variable, follow-up FV, PDI, hPDI, and uPDI as mediating variables, and cognitive trajectories as the dependent variable. The results, shown in Table 2 and Figure 4, indicated that FV was the only significant mediating factor before and after controlling for covariates. The results demonstrated that both the direct effect of years of education and the indirect effect of FV on cognitive function were significant (95% CI did not include 0). The mediation effect explained 3.5% [0.021/(0.021 + 0.582)] of the total variance. These findings suggest that FV mediates the impact of years of education on changes in cognitive function.
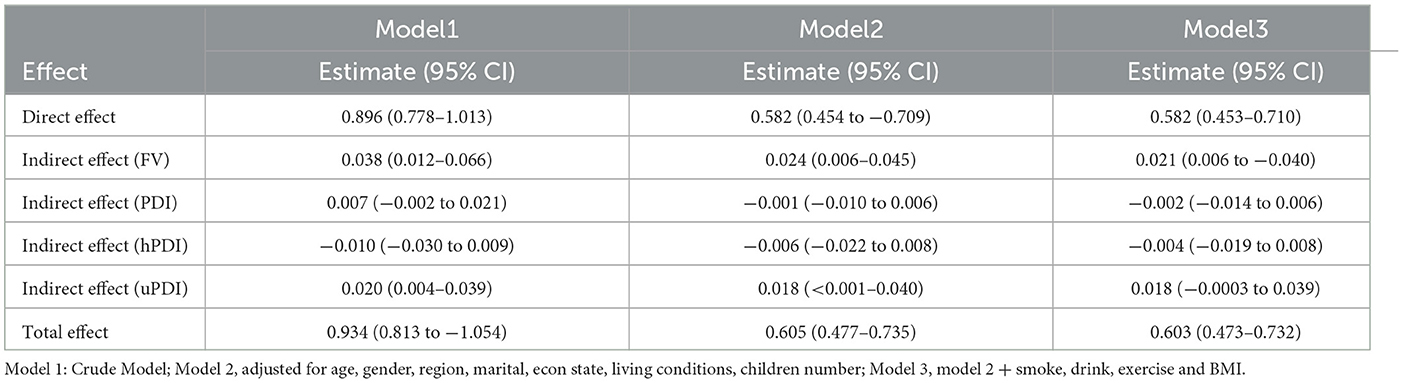
Table 2. Mediating effects of FV and plant-based dietary patterns on the relationship between education and cognitive function changes.
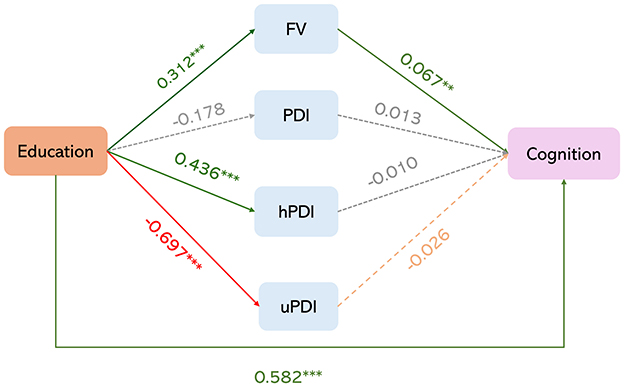
Figure 4. Mediation analysis of education and cognitive function changes mediated by FV and plant-based dietary patterns (adjusted covariates). *P < 0.05, **P < 0.01, ***P < 0.001.
4 Discussion
This longitudinal study adds to the limited research on the changes in cognitive function over time in older adults and helps us understand the factors influencing different cognitive trajectories in individuals aged 65 and above. Additionally, this study further elucidates the mediating role of diet between educational attainment and cognitive function. The results indicated that there were three distinct trajectories of cognitive function over a 10-year period in older adults: high stability, slow decline, and rapid decline. Among these, the high stability group comprises the largest proportion of participants. These findings are consistent with the results of Tampubolon (2015), Min (2018), and Tu et al. (2020), suggesting that cognitive function remains stable for the majority of participants during aging.
Additionally, the analysis of related factors in this study indicated that characteristics such as education, FV, age, gender, drinking habits, and uPDI were associated with cognitive decline. These findings are consistent with the results of Tu et al. (2020) and Wu et al. (2019), which also validated the associations between age, gender, educational attainment, diet quality, and cognitive function in older adults. It demonstrates that higher educational attainment, being male, and better diet quality can reduce the risk of cognitive decline.
Moreover, our study revealed an interesting phenomenon: overall, baseline education level was significantly associated with FV, PDI, hPDI, and cognitive function at the 2018 follow-up, which is consistent with most previous research conclusions. Higher levels of education predict that individuals are more likely to maintain healthier dietary behaviors (Kant, 2004; Harrington et al., 2014; Mishra et al., 2006; Tabung et al., 2016) or improve their dietary behaviors over time (Harrington et al., 2014; Arabshahi et al., 2011); higher education levels tend to be associated with slower cognitive decline (Marioni et al., 2012). However, stratified analyses revealed that these significant associations did not apply to all older adults. For instance, the longitudinal associations between education and FV, PDI, and hPDI were observed only in the group with slow cognitive decline, while the longitudinal association between education and cognitive function was significant only in the stable group. Considering the differences in mean years of education among the cognitive trajectory groups, the high stability group had generally higher educational attainment compared to the slow decline and rapid decline groups, with the rapid decline group having the lowest educational levels. To our knowledge, the influence of education on dietary behavior is likely related to the ability to acquire, understand, and implement knowledge about ideal dietary behaviors. Individuals with low educational attainment have consistently shown lower overall dietary quality levels, possibly due to a lack of nutrition knowledge, cooking skills, or the ability to use preventive information. Those who have received a certain level of schooling are capable of acquiring these skills, thereby meeting the essential requirements for adopting healthy dietary behaviors and improving their diet. This group, with a certain educational foundation and situated at the boundary of having or lacking the ability to change dietary behaviors, is likely to exhibit characteristics of slow cognitive decline. The reason why the impact of education on cognition exists as a longitudinal association only in the high stability cognitive function group might be that this group has relatively higher education levels, while the education levels of the other two groups are too low to influence cortical functions and other behaviors mediating cognitive function, or it may be that their relationship is offset by other factors. These conclusions require further in-depth research and verification, but they also provide a new research perspective for related fields.
Finally, the results of our study demonstrated that FV has a significant mediating effect on the relationship between years of education and changes in cognitive function. The above findings are consistent with several studies. Firstly, individuals with higher education levels are more likely to maintain a healthy diet. Secondly, healthy dietary patterns and adequate variety in food intake can improve cognitive function by affecting metabolism (Galbete et al., 2018), inflammation (Azadbakht et al., 2005; Miller et al., 1992), and microvascular function (Yaffe et al., 2014). This suggests that FV may partially mediate the relationship between education and cognitive function.
This study has several strengths. First, we are the first to demonstrate the bidirectional association between educational attainment, dietary patterns, and cognitive function among individuals with different cognitive function trajectories, using data from a nationally representative long-term cohort. This provides strong support for the associations described in our study. Second, we utilized multi-time point cohort data spanning 10 years to explore longitudinal associations, thereby gaining a profound understanding of the relationships between education, diet, and cognitive function. This will provide guidance for interventions aimed at maintaining cognitive function in the elderly. Third, we assessed the potential mechanisms underlying this association, showing that the relationship between educational attainment and cognitive function is partially mediated by food variety, which may further enhance health interventions.
Despite its contributions, this study has several limitations. First, due to resource limitations, cognitive function was assessed solely using the MMSE scale. As a rapid screening tool, the MMSE may not capture the full complexity of cognitive function, potentially limiting the precision of cognitive assessments. However, MMSE has been widely validated as a reliable instrument for large-scale population studies. Second, both education level and dietary information were self-reported by the participants, which may introduce potential biases. Furthermore, the lack of more detailed and representative assessment metrics represents an inherent limitation of this study. More comprehensive evaluations are crucial for future in-depth research. Nevertheless, the measurement methods employed in this study have been validated as effective in previous studies (Zhang et al., 2022; Qi et al., 2023; Yi and Vaupel, 2010). Third, our study examined only one potential mediating mechanism through food variety (FV). While the mediating effect of FV was statistically significant, it accounted for only a small portion of the total effect, indicating that other unexamined mediators likely exist and require further exploration. Fourth, some participants were excluded due to missing data or loss to follow-up, which may introduce selection bias and affect the representativeness of the findings. Future research will involve combining multiple representative cohort datasets to better understand the relationships between education, diet, and cognition. Additionally, when feasible, we aim to construct cohort datasets for related research topics to conduct more accurate and representative assessments of population cognition, educational levels, and dietary patterns, thus enhancing the reliability of the associations observed. Finally, regarding the dietary assessment in this study, the lack of information on the proportions of various food components represents a key area for future research. Investigating the role of food component proportions in the identified associations will be an important direction for subsequent studies.
5 Conclusion
In conclusion, this study indicated that there was a longitudinal association between educational attainment and cognitive function over time among older adults in China, and this prospective education-cognition relationship was partially mediated by FV. Given these findings, healthcare providers can focus more on older adults with lower educational levels and inadequate food variety intake, taking effective measures to mitigate cognitive function disparities caused by low educational attainment and addressing cognitive decline by enriching food variety.
Data availability statement
Publicly available datasets were analyzed in this study. This data can be found here: https://opendata.pku.edu.cn/dataverse/CHADS (accessed on 27 April 2024).
Ethics statement
The studies involving humans were approved by Biomedical Ethics Committee of Peking University. The studies were conducted in accordance with the local legislation and institutional requirements. The participants provided their written informed consent to participate in this study.
Author contributions
XW: Conceptualization, Data curation, Methodology, Software, Writing – original draft. YQ: Data curation, Writing – original draft. YZ: Data curation, Writing – original draft. YC: Data curation, Writing – review & editing. HR: Visualization, Writing – review & editing. CH: Visualization, Writing – review & editing. LQ: Conceptualization, Project administration, Supervision, Writing – review & editing.
Funding
The author (s) declare financial support was received for the research, authorship, and/or publication of this article. This research was supported by a grant from the National Natural Science Foundation of China (grant no: 81973155).
Acknowledgments
Thanks to the Chinese Longitudinal Healthy Longevity Survey (CLHLS) for providing the data and allowing us to use it for free.
Conflict of interest
The authors declare that the research was conducted in the absence of any commercial or financial relationships that could be construed as a potential conflict of interest.
Publisher's note
All claims expressed in this article are solely those of the authors and do not necessarily represent those of their affiliated organizations, or those of the publisher, the editors and the reviewers. Any product that may be evaluated in this article, or claim that may be made by its manufacturer, is not guaranteed or endorsed by the publisher.
Supplementary material
The Supplementary Material for this article can be found online at: https://www.frontiersin.org/articles/10.3389/fnagi.2024.1498454/full#supplementary-material
References
Arabshahi, S., Lahmann, P. H., Williams, G. M., Marks, G. C., and van der Pols, J. C. (2011). Longitudinal change in diet quality in Australian adults varies by demographic, socio-economic, and lifestyle characteristics. J. Nutr. 141, 1871–1879. doi: 10.3945/jn.111.140822
Azadbakht, L., Mirmiran, P., and Azizi, F. (2005). Dietary diversity score is favorably associated with the metabolic syndrome in Tehranian adults. Int. J. Obesity 29, 1361–1367. doi: 10.1038/sj.ijo.0803029
Berendsen, A. A. M., Kang, J. H., van de Rest, O., Feskens, E. J. M., de Groot, L., Grodstein, F., et al. (2017). The dietary approaches to stop hypertension diet, cognitive function, and cognitive decline in american older women. J. Am. Med. Dir. Assoc. 18, 427–432. doi: 10.1016/j.jamda.2016.11.026
Boss, L., Kang, D. H., and Branson, S. (2015). Loneliness and cognitive function in the older adult: a systematic review. Int. Psychogeriatr. 27, 541–553. doi: 10.1017/S1041610214002749
Chan, R. C., Hoosain, R., and Lee, T. M. (2002). Reliability and validity of the Cantonese version of the Test of Everyday Attention among normal Hong Kong Chinese: a preliminary report. Clin. Rehabil. 16, 900–909. doi: 10.1191/0269215502cr574oa
Chen, Y. C., Jung, C. C., Chen, J. H., Chiou, J. M., Chen, T. F., Chen, Y. F., et al. (2017). Association of dietary patterns with global and domain-specific cognitive decline in Chinese elderly. J. Am. Geriatr. Soc. 65, 1159–1167. doi: 10.1111/jgs.14741
Foubert-Samier, A., Catheline, G., Amieva, H., Dilharreguy, B., Helmer, C., Allard, M., et al. (2012). Education, occupation, leisure activities, and brain reserve: a population-based study. Neurobiol. Aging. 33, 423.e15–25. doi: 10.1016/j.neurobiolaging.2010.09.023
Galbete, C., Schwingshackl, L., Schwedhelm, C., Boeing, H., and Schulze, M. B. (2018). Evaluating mediterranean diet and risk of chronic disease in cohort studies: an umbrella review of meta-analyses. Eur. J. Epidemiol. 33, 909–931. doi: 10.1007/s10654-018-0427-3
Hamaker, E. L., Kuiper, R. M., and Grasman, R. P. (2015). A critique of the cross-lagged panel model. Psychol. Methods 20, 102–116. doi: 10.1037/a0038889
Haring, B., Wu, C., Mossavar-Rahmani, Y., Snetselaar, L., Brunner, R., Wallace, R. B., et al. (2016). No association between dietary patterns and risk for cognitive decline in older women with 9-year follow-up: data from the women's health initiative memory study. J. Acad. Nutr. Dietet. 116, 921–930.e1. doi: 10.1016/j.jand.2015.12.017
Harrington, J. M., Dahly, D. L., Fitzgerald, A. P., Gilthorpe, M. S., and Perry, I. J. (2014). Capturing changes in dietary patterns among older adults: a latent class analysis of an ageing Irish cohort. Public Health Nutr. 17, 2674–2686. doi: 10.1017/S1368980014000111
Haslbeck, J. M. B., and Waldorp, L. J. (2015). mgm: estimating time-varying mixed graphical models in high-dimensional data. J. Stat. Sftw. 93, 1–46. doi: 10.18637/jss.v093.i08
Iacobucci, D. (2012). Mediation analysis and categorical variables: the final frontier. J. Cons. Psychol. 22, 582–594. doi: 10.1016/j.jcps.2012.03.006
Iuliano, S., Poon, S., Robbins, J., Bui, M., Wang, X., De Groot, L., et al. (2021). Effect of dietary sources of calcium and protein on hip fractures and falls in older adults in residential care: cluster randomised controlled trial. BMJ 375:n2364. doi: 10.1136/bmj.n2364
Jennings, A., Berendsen, A. M., de Groot, L., Feskens, E. J. M., Brzozowska, A., Sicinska, E., et al. (2019). Mediterranean-style diet improves systolic blood pressure and arterial stiffness in older adults. Hypertension 73, 578–586. doi: 10.1161/HYPERTENSIONAHA.118.12259
Jia, L., Du, Y., Chu, L., Zhang, Z., Li, F., Lyu, D., et al. (2020). Prevalence, risk factors, and management of dementia and mild cognitive impairment in adults aged 60 years or older in China: a cross-sectional study. Lancet Public Health 5, e661–e71. doi: 10.1016/S2468-2667(20)30185-7
Kant, A. K. (2004). Dietary patterns and health outcomes. J. Am. Diet. Assoc. 104, 615–635. doi: 10.1016/j.jada.2004.01.010
Kennedy, G. L. (2009). Evaluation of dietary diversity scores for assessment of micronutrient intake and food security in developing countries (Doctoral thesis). University of Wageningen, Netherlands.
Kennedy, P. G., Ballard, T., and Dop, M. (2011). Guidelines for Measuring Household and Individual Dietary Diversity. Rome: Food and Agriculture Organization of the United Nations.
Li, L. W., Ding, D., Wu, B., and Dong, X. (2017). Change of cognitive function in u.s. chinese older adults: a population-based study. J. Gerontol. Ser. A 72(suppl_1):S5–10. doi: 10.1093/gerona/glx004
Lin, C. C., Shih, M. H., Chen, C. D., and Yeh, S. L. (2021). Effects of adequate dietary protein with whey protein, leucine, and vitamin D supplementation on sarcopenia in older adults: an open-label, parallel-group study. Clin. Nutr. 40, 1323–1329. doi: 10.1016/j.clnu.2020.08.017
Lök, N., Bademli, K., and Selçuk-Tosun, A. (2019). The effect of reminiscence therapy on cognitive functions, depression, and quality of life in Alzheimer patients: Randomized controlled trial. Int. J. Geriatr. Psychiatry 34, 47–53. doi: 10.1002/gps.4980
Loughrey, D. G., Lavecchia, S., Brennan, S., Lawlor, B. A., and Kelly, M. E. (2017). The impact of the mediterranean diet on the cognitive functioning of healthy older adults: a systematic review and meta-analysis. Adv. Nutr. 8, 571–586. doi: 10.3945/an.117.015495
Malek-Ahmadi, M. (2016). Reversion from mild cognitive impairment to normal cognition: a meta-analysis. Alzheimer Dis. Assoc. Disord. 30, 324–330. doi: 10.1097/WAD.0000000000000145
Marioni, R. E., van den Hout, A., Valenzuela, M. J., Brayne, C., and Matthews, F. E. (2012). Active cognitive lifestyle associates with cognitive recovery and a reduced risk of cognitive decline. J. Alzheimers Dis. 28, 223–230. doi: 10.3233/JAD-2011-110377
Miller, W. L., Crabtree, B. F., and Evans, D. K. (1992). Exploratory study of the relationship between hypertension and diet diversity among Saba Islanders. Public Health Rep. 107, 426–432.
Min, J. W. A. (2018). longitudinal study of cognitive trajectories and its factors for Koreans aged 60 and over: a latent growth mixture model. Int. J. Geriatr. Psychiatry 33, 755–762. doi: 10.1002/gps.4855
Ministry of Civil Affairs People's Republic of China. (2022).The Communique on the Development of the National Cause for Aging in 2022 was released [EB/OL]. Available at: https://www.mca.gov.cn/n152/n165/c1662004999980001752/content.html (accessed December 21, 2024).
Mishra, G. D., McNaughton, S. A., Bramwell, G. D., and Wadsworth, M. E. (2006). Longitudinal changes in dietary patterns during adult life. Br. J. Nutr. 96, 735–744. doi: 10.1079/BJN20061871
Nagin, D. S. (1999). Analyzing developmental trajectories: a semiparametric, group-based approach. Psychol. Methods 4, 139–157. doi: 10.1037/1082-989X.4.2.139
Nakazaki, E., Mah, E., Sanoshy, K., Citrolo, D., and Watanabe, F. (2021). Citicoline and memory function in healthy older adults: a randomized, double-blind, placebo-controlled clinical trial. J. Nutr. 151, 2153–2160. doi: 10.1093/jn/nxab119
Neelakantan, N., Koh, W. P., Yuan, J. M., and van Dam, R. M. (2018). Diet-quality indexes are associated with a lower risk of cardiovascular, respiratory, and all-cause mortality among Chinese adults. J. Nutr. 148, 1323–1332. doi: 10.1093/jn/nxy094
Nucci, M., Mapelli, D., and Mondini, S. (2012). Cognitive Reserve Index questionnaire (CRIq): a new instrument for measuring cognitive reserve. Aging Clin. Exp. Res. 24, 218–226. doi: 10.1007/BF03654795
Petersen, R. C., Smith, G. E., Waring, S. C., Ivnik, R. J., Tangalos, E. G., Kokmen, E., et al. (1999). Mild cognitive impairment: clinical characterization and outcome. Arch. Neurol. 56, 303–308. doi: 10.1001/archneur.56.3.303
Qi, R., Yang, Y., Sheng, B., Li, H., and Zhang, X. (2023). Plant-based diet indices and their association with frailty in older adults: a CLHLS-based cohort study. Nutrients 15:245120. doi: 10.3390/nu15245120
Rujing, R., Peng, Y., Zhihui, W., Jinlei, Q., Ran, T., Jintao, W., et al. (2021). China Alzheimer disease report 2021. J. Diagn. Concepts Pract. 20, 317–337. doi: 10.16150/j.1671-2870.2021.04.001
Satija, A., Bhupathiraju, S. N., Rimm, E. B., Spiegelman, D., Chiuve, S. E., Borgi, L., et al. (2016). Plant-based dietary patterns and incidence of type 2 diabetes in US men and women: results from three prospective cohort studies. PLoS Med. 13:e1002039. doi: 10.1371/journal.pmed.1002039
Satija, A., Bhupathiraju, S. N., Spiegelman, D., Chiuve, S. E., Manson, J. E., Willett, W., et al. (2017). Healthful and unhealthful plant-based diets and the risk of coronary heart disease in U.S. adults. J. Am. Coll. Cardiol. 70, 411–422. doi: 10.1016/j.jacc.2017.05.047
Satija, A., Malik, V., Rimm, E. B., Sacks, F., Willett, W., Hu, F. B., et al. (2019). Changes in intake of plant-based diets and weight change: results from 3 prospective cohort studies. Am. J. Clin. Nutr. 110, 574–582. doi: 10.1093/ajcn/nqz049
Seow, A., Shi, C. Y., Franke, A. A., Hankin, J. H., Lee, H. P., Yu, M. C., et al. (1998). Isoflavonoid levels in spot urine are associated with frequency of dietary soy intake in a population-based sample of middle-aged and older Chinese in Singapore. Cancer Epidemiol. Biomarkers Prev. 7, 135–140.
Smyth, A., Dehghan, M., O'Donnell, M., Anderson, C., Teo, K., Gao, P., et al. (2015). Healthy eating and reduced risk of cognitive decline: a cohort from 40 countries. Neurology 84, 2258–2265. doi: 10.1212/WNL.0000000000001638
Tabung, F. K., Steck, S. E., Zhang, J., Ma, Y., Liese, A. D., Tylavsky, F. A., et al. (2016). Longitudinal changes in the dietary inflammatory index: an assessment of the inflammatory potential of diet over time in postmenopausal women. Eur. J. Clin. Nutr. 70, 1374–1380. doi: 10.1038/ejcn.2016.116
Tampubolon, G. (2015). Cognitive ageing in great britain in the new century: cohort differences in episodic memory. PLoS ONE 10:e0144907. doi: 10.1371/journal.pone.0144907
Tangney, C. C., Li, H., Wang, Y., Barnes, L., Schneider, J. A., Bennett, D. A., et al. (2014). Relation of DASH- and mediterranean-like dietary patterns to cognitive decline in older persons. Neurology 83, 1410–1416. doi: 10.1212/WNL.0000000000000884
Thorpe, M. G., Milte, C. M., Crawford, D., and McNaughton, S. A. (2019). Education and lifestyle predict change in dietary patterns and diet quality of adults 55 years and over. Nutr. J. 18:67. doi: 10.1186/s12937-019-0495-6
Tu, L., Lv, X., Yuan, C., Zhang, M., Fan, Z., Xu, X., et al. (2020). Trajectories of cognitive function and their determinants in older people: 12 years of follow-up in the Chinese Longitudinal Healthy Longevity Survey. Int. Psychogeriatr. 32, 765–775. doi: 10.1017/S1041610220000538
Wengreen, H., Munger, R. G., Cutler, A., Quach, A., Bowles, A., Corcoran, C., et al. (2013). Prospective study of Dietary Approaches to Stop Hypertension- and Mediterranean-style dietary patterns and age-related cognitive change: the Cache County Study on Memory, Health and Aging. Am. J. Clin. Nutr. 98, 1263–1271. doi: 10.3945/ajcn.112.051276
Wu, J., Song, X., Chen, G. C., Neelakantan, N., van Dam, R. M., Feng, L., et al. (2019). Dietary pattern in midlife and cognitive impairment in late life: a prospective study in Chinese adults. Am. J. Clin. Nutr. 110, 912–920. doi: 10.1093/ajcn/nqz150
Yaffe, K., Vittinghoff, E., Pletcher, M. J., Hoang, T. D., Launer, L. J., Whitmer, R., et al. (2014). Early adult to midlife cardiovascular risk factors and cognitive function. Circulation 129, 1560–1567. doi: 10.1161/CIRCULATIONAHA.113.004798
Yi, Z., and Vaupel, J. W. (2010). functional capacity and self–evaluation of health and life of oldest old in China. J. Soc. Issues 58, 733–748. doi: 10.1111/1540-4560.00287
Zeng, Y., Feng, Q., Gu, D., and Vaupel, J. W. (2017). Demographics, phenotypic health characteristics and genetic analysis of centenarians in China. Mech. Ageing Dev. 165(Pt B):86–97. doi: 10.1016/j.mad.2016.12.010
Zhang, J., Wang, Q., Hao, W., and Zhu, D. (2022). Long-term food variety and dietary patterns are associated with frailty among Chinese older adults: a cohort study based on CLHLS from 2014 to 2018. Nutrients 14:479. doi: 10.3390/nu14204279
Keywords: older adults, cognitive trajectories, network analysis, cross-lagged panel model, mediation analysis
Citation: Wang X, Qiao Y, Zhang Y, Cui Y, Ren H, Hao C and Qiu L (2025) Cognitive trajectories and their relationships with education and diets among older adults: a network-based 10-year cohort study. Front. Aging Neurosci. 16:1498454. doi: 10.3389/fnagi.2024.1498454
Received: 19 September 2024; Accepted: 27 December 2024;
Published: 15 January 2025.
Edited by:
Kristin Rene Krueger, Rush University Medical Center, United StatesReviewed by:
Donato Melchionda, Azienda Ospedaliero-Universitaria Ospedali Riuniti di Foggia, ItalyAnna Cierniak-Emerych, Wroclaw University of Economics, Poland
Copyright © 2025 Wang, Qiao, Zhang, Cui, Ren, Hao and Qiu. This is an open-access article distributed under the terms of the Creative Commons Attribution License (CC BY). The use, distribution or reproduction in other forums is permitted, provided the original author(s) and the copyright owner(s) are credited and that the original publication in this journal is cited, in accordance with accepted academic practice. No use, distribution or reproduction is permitted which does not comply with these terms.
*Correspondence: Lixia Qiu, cWx4XzExMjZAMTYzLmNvbQ==