- 1Department of Geriatrics, The Second Affiliated Hospital and Yuying Children’s Hospital of Wenzhou Medical University, Wenzhou, China
- 2Department of Preventive Medicine, School of Public Health, Wenzhou Medical University, Wenzhou, China
Background: Accumulating evidence suggested that Alzheimer’s disease (AD) was associated with altered gut microbiota. However, the relationships between gut microbiota and specific cognitive domains of AD patients have yet been fully elucidated. The aim of this study was to explore microbial signatures associated with global cognition and specific cognitive domains in AD patients and to determine their predictive value as biomarkers.
Methods: A total of 64 subjects (18 mild AD, 23 severe AD and 23 healthy control) were recruited in the study. 16 s rDNA sequencing was performed for the gut bacteria composition, followed by liquid chromatography electrospray ionization tandem mass spectrometry (LC/MS/MS) analysis of short-chain fatty acids (SCFAs). The global cognition, specific cognitive domains (abstraction, orientation, attention, language, etc.) and severity of cognitive impairment, were evaluated by Montreal Cognitive Assessment (MoCA) scores. We further identified characteristic bacteria and SCFAs, and receiver operating characteristic (ROC) curve was used to determine the predictive value.
Results: Our results showed that the microbiota dysbiosis index was significantly higher in the severe and mild AD patients compared to the healthy control (HC). Linear discriminant analysis (LDA) showed that 12 families and 17 genera were identified as key microbiota among three groups. The abundance of Butyricicoccus was positively associated with abstraction, and the abundance of Lachnospiraceae_UCG-004 was positively associated with attention, language, orientation in AD patients. Moreover, the levels of isobutyric acid and isovaleric acid were both significantly negatively correlated with abstraction, and level of propanoic acid was significantly positively associated with the attention. In addition, ROC models based on the characteristic bacteria Lactobacillus, Butyricicoccus and Lachnospiraceae_UCG-004 could effectively distinguished between low and high orientation in AD patients (area under curve is 0.891), and Butyricicoccus and Agathobacter or the combination of SCFAs could distinguish abstraction in AD patients (area under curve is 0.797 and 0.839 respectively).
Conclusion: These findings revealed the signatures gut bacteria and metabolite SCFAs of AD patients and demonstrated the correlations between theses characteristic bacteria and SCFAs and specific cognitive domains, highlighting their potential value in early detection, monitoring, and intervention strategies for AD patients.
1 Introduction
Alzheimer’s disease (AD) is the most common neurodegenerative disease with progressive cognitive decline (Elahi and Miller, 2017). Although many researchers have been exploring the disease’s origins and pathogenesis of this disease, no universally accepted cause has been identified, and no effective strategies for early diagnosis and treatment for this disease were currently available (Kandimalla and Reddy, 2017; Drummond and Wisniewski, 2017; Hansson, 2021). Consequently, early detection and diagnosis were crucial in slowing the progression of the disease and reducing its prevalence and morbidity (Gonçalves et al., 2024; Barron and Molofsky, 2021). In clinical practice, the diagnosis of AD frequently undergoes neuroimaging, examination of cerebrospinal fluid, and assessment of various cognitive scales (Kazmi and Hsiao, 2023; Harris, 2023). Montreal Cognitive Assessment (MoCA) was the most common tool to evaluate cognitive function in AD patients (Pinto et al., 2019), however, it was noteworthy that the differences in the education level, cultural background, examiner’s skills and experience, examination environment, and the emotional and mental state of the subjects could interfere with the accuracy of the assessment of cognitive function (Boxer and Sperling, 2023; Migliore and Coppede, 2022). Although memory impairment is the most common symptom in AD patients, some people may exhibit atypical symptoms, such as visual–spatial, language, executive and behavioral, and motor dysfunction (Graff-Radford et al., 2021), which increases the difficulty in recognizing AD in patients without prominent memory deficits (Scheltens et al., 2021). A retrospective review reported a misdiagnosis rate as high as 53% in young-onset AD, while the rate was only 4% in patients with typical symptoms (Balasa et al., 2011). Due to the heterogeneity of clinical manifestations and the complexity of disease neuropathology, there were great differences in clinical practice, resulting in complex identification of cognitive impairment in the early stage. Currently, these tests are time-consuming, cumbersome, and require a high degree of expertise from practitioners, suggesting an urgent need for an effective tool to accurately identify cognitive decline and different cognitive domains in older adults.
Accumulating evidence supported the significant alterations in the gut microbiota composition and function of AD patients (Loh et al., 2024), and abnormal gut bacteria were closely related to the development and progression of AD (Skalny et al., 2024; Zhu et al., 2022). Our previous studies revealed the increases of pro-inflammatory bacteria and the decrease of producing-short chain fatty acids (SCFAs) bacteria in APP/PS1 mice compared to the WT mice (Pan et al., 2024; Sun et al., 2019). In a clinically randomized controlled trial (RCT), the improvement of immediate memory and delayed memory functions in healthy older population were correlated with the supplementation of Bifidobacterium longum BB68S (Shi et al., 2022). Bifidobacterium was observed in the senescence-accelerated mice, as the memory deficits were improved after receiving supplementation of a probiotic preparation comprising of B. lactis, Lactobacillus casei, B. bifidum, and L. acidophilus (Yang et al., 2020). It was reported that probiotic treatment could improve memory in traumatic brain injury mice (Amaral et al., 2024). The abundance of Lactobacillus in mice with impaired memory was negatively correlated with the activation of NF-ĸB signaling pathway (Jang et al., 2018), and Ruminococcaceae_UCG_004 was prominently associated with attention deficit symptom in attention-deficit/hyperactivity disorder patients (Szopinska-Tokov et al., 2020), suggesting that the changes of different bacteria might be related to the alterations of different cognitive domains. Metataxonomic analyses showed a decrease in butyrate-producing bacterial communities and a concurrent reduction in SCFA butyrate production in the 3 × Tg-AD mice (Chilton et al., 2024). Multi-strain probiotics treatment could significantly improve cognitive impairment in the SAMP8 mice, and the mechanism may be related to the regulation of SCFAs, such as valeric acid, isovaleric acid, and hexanoic acid (Xiao-Hang et al., 2024). Although gut microbiota might be involved in the occurrence and development of AD, the relationships between key bacteria and specific cognitive domains have not been explored.
In this study, we aimed to investigate the features of the gut microbiota and metabolite SCFAs in patients with AD, and the potential correlations between characteristic gut bacteria and SCFAs and specific cognitive domains. This study provided insight into the application of gut microbiota and SCFAs signatures in early detection and intervention strategies in patients with AD.
2 Materials and methods
2.1 Study population
This study was conducted in the Department of Geriatrics, the Second Affiliated Hospital of Wenzhou Medical University from January 2022 to May 2024. The AD was diagnosed according to the guidelines established by National Institute on Aging-Alzheimer’s Association workgroups (McKhann et al., 2011). Exclusion criteria: MRI evidence of stroke, hippocampal sclerosis, local space-occupying lesion, or any cognitive ability-related disease of central nervous system (CNS); The psychiatric disorders (except mild depression) or currently treated with related medication; alcohol abuse within the last 2 years; The application of epileptic medication or hypnotic; The untreated thyroid disease or deficiency of vitamin B12 or folate; The presence of hearing or visual impairments that could influence the completion of scales; The self-reported using of probiotics or antibiotics or dieting within the last 3 months; The comorbidities such as malignant tumors, acute enteritis and severe respiratory failure. A total of 41 AD patients were included in the study and stratified based on the severity of cognitive impairment into mild AD (mAD, n = 18, 10 ≤ MoCA <18) and severe AD (sAD, n = 23, MoCA <10) groups, with consideration given to their educational levels. A group of healthy control participants (HC, n = 23) were also recruited during the same period. The exclusion criteria mentioned above were applied to control participants as well.
2.2 16 s rRNA sequencing
Fresh fecal samples were collected and then promptly stored in −80°C until analysis. Microbial DNA was extracted using OMEGA-soil DNA Kit (Omega Bio-Tek, United States). The concentration and purity the extracted DNA were detected using NanoDrop2000 UV–vis spectrophotometer (Thermo Scientific, Wilmington, United States). The V3-V4 hypervariable regions of the microbial 16 s rRNA gene were amplified using primers 338F9 (ACTCCTACGGGAGGCAGCAG) and 806R (GGACTACHVGGGTWTCTAAT). Subsequently, sequencing was performed on the Illumina MiSeq System (Illumina, United States) by Majorbio Bio-Pharm Technology Co. Ltd. (Shanghai, China). Alpha diversity was assessed using the Simpson index. The difference in alpha and beta diversity among the three groups were analyzed using the Kruskal-Wallis test. Principal coordinates analysis (PCoA), based on abund-jaccard distance algorithm, was employed to analyze variation in microbiota composition, the differences among the 3 groups were evaluated using the Analysis of Similarities (ANOSIM). Linear discriminant analysis (LDA) effect size (LEfSe) based on Kruskal-Wallis test was utilized to detect differences in species abundance among groups. Subsequently, LDA was employed to estimate the impact of these different genera on intergroup differences. Genera with LDA score > 2 were diagrammed on the bar plots. The Wilcoxon rank sun test was also used to identify significantly different taxa among groups, followed by post-hoc tests for pairwise comparisons between groups using welch-uncorrected test.
2.3 SCFAs analysis
Fresh fecal samples (200 mg) were collected from subjects during hospitalization, outpatient or health screening center, and added in a 2 mL centrifuge tube with internal standard (L-2-chlorophenylalanine) for grinding and low-temperature ultrasonic extraction. After centrifuging for 15 min (4°C, 13,000 g), the supernatant was used for LC–MS/MS analysis on a Thermo UHPLC-Q Exactive HF-X system equipped with an ACQUITY HSS T3 column (100 mm × 2.1 mm i.d., 1.8 μm, Waters, United States) at Majorbio Bio-Pharm Technology Co. Ltd. (Shanghai, China). Followed by the pretreatment of LC/MS raw data in Progenesis QI software (Waters Corporation, Milford, United States), the metabolites were identified by searching database including the HMDB,1 Metlin2 and Majorbio Database3. The final concentrations of SCFAs were expressed as micrograms per milligram of feces (μg/g).
2.4 Statistical analysis
The differences in both the total score and individual item scores of MoCA between groups of AD patients were analyzed using Wilcoxon rank sum test. The p value <0.05 was considered statistically significant. The microbiota dysbiosis index was calculated by Wilcoxon rank sun test and visualized through a violin plot. Several important genera, with gradient alteration among groups or extruding in comparation of groups or correlating with scores had been selected and presented separately by bar plot. Furthermore, Spearman’s correlation analysis was performed to reveal the relationships of microbiota and SCFAs with cognitive functions. The receiver operator characteristic (ROC) curves were plotted to estimate the distinguishability of differential microbiota and SCFAs in terms of abstraction and orientation capabilities.
3 Results
3.1 Alterations of gut microbial diversity in AD patients
The microbiota dysbiosis index was significantly higher in both the sAD patients and mAD patients compared to the HC (Figure 1A, sAD vs. HC: p = 0.0001; mAD vs. HC: p = 0.0034). There was no significant difference in the Simpson index among the HC, mAD patients and sAD patients (Figure 1B). Moreover, PCoA revealed a significant difference among three groups (Figure 1C, p = 0.0220). Furthermore, there was significant difference in β diversity among three groups (Figure 1D, p = 0.0013).
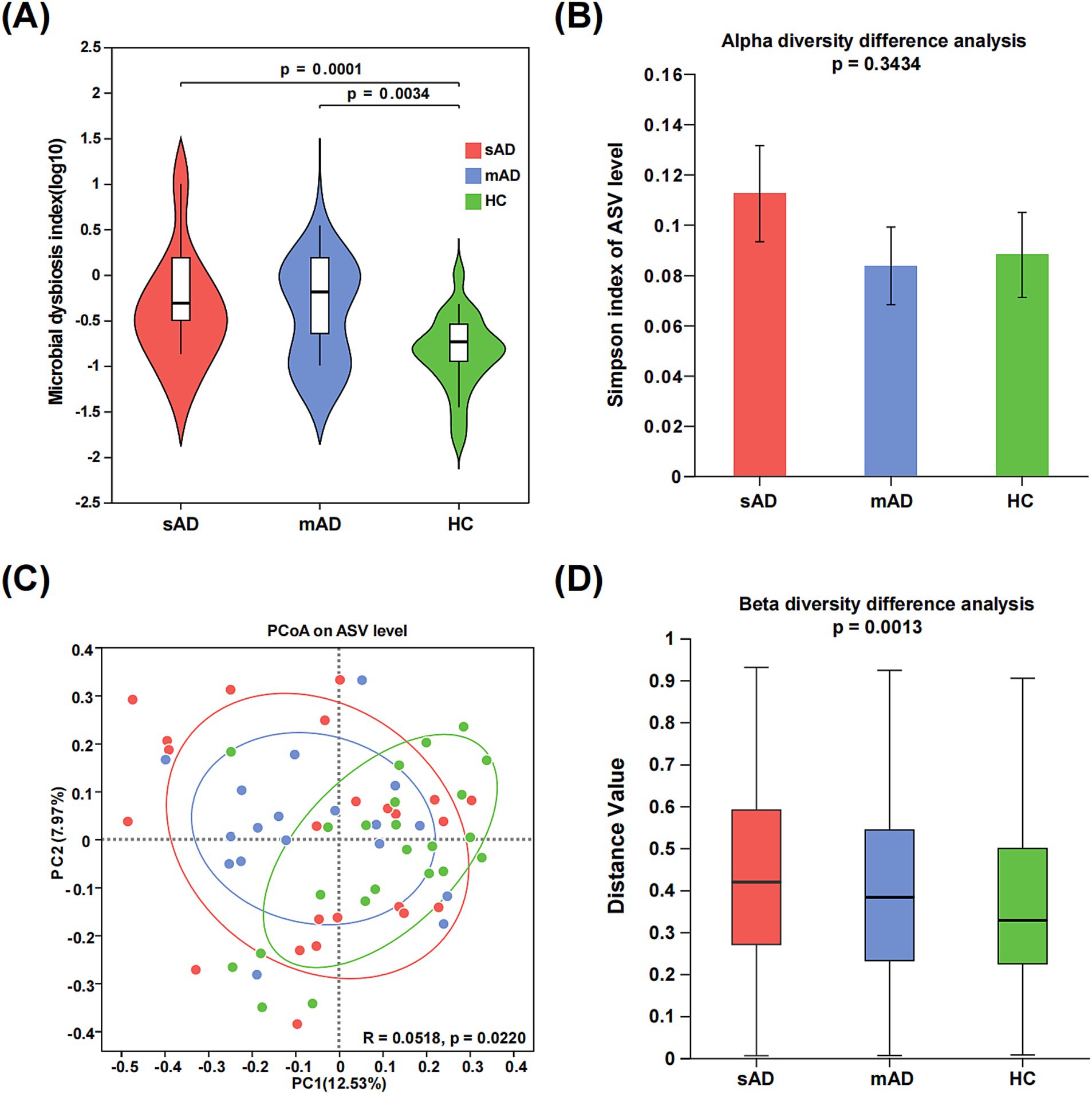
Figure 1. Alterations of gut microbial diversity in AD patients. (A) The MDI of the gut microbiota among sAD patients, mAD patients and HC groups. (B) The alpha diversities of gut microbiota among three groups were represented through Simpson index. (C) PCoA of gut microbiota based on abund_jaccard showed that most of the microbial samples were clustered by disease status (PC1 = 12.53%, PC2 = 7.97%). (D) The beta diversities of fecal microbiota among three groups (Green, HC; blue, mAD; red, sAD).
3.2 Characteristic gut bacteria in AD patients
The cladogram of distinguished bacterial populations was plotted in Figure 2A and there were 2 taxa, 10 taxa and 22 taxa, respectively, enriched in sAD, mAD and HC groups from phylum to genus levels. As shown in Figure 2B, LDA effect size analysis showed that 12 families and 17 genera were identified as key microbiota among three groups. Among the 29 taxa, 5 taxa were exclusively enriched in sAD patients, 9 taxa were exclusively enriched in mAD patients, and 15 taxa were exclusively enriched in HC. Significant bacteria of sAD group mainly included g_Sellimonas, and mAD group mainly included g_Bacillus, and g_Leuconostoc, while g_Agathobacter, g_Phascolarctobacterium, g_Butyricicoccus, g_Fusobacterium, g_Haemophilus, g_Lachnospiraceae_UCG-001, g_Parasutterella, g_Lachnospiraceae_ND3007_group, g_Lachnospira, and g_Lachnospiraceae_UCG-004 were involved in HC group.
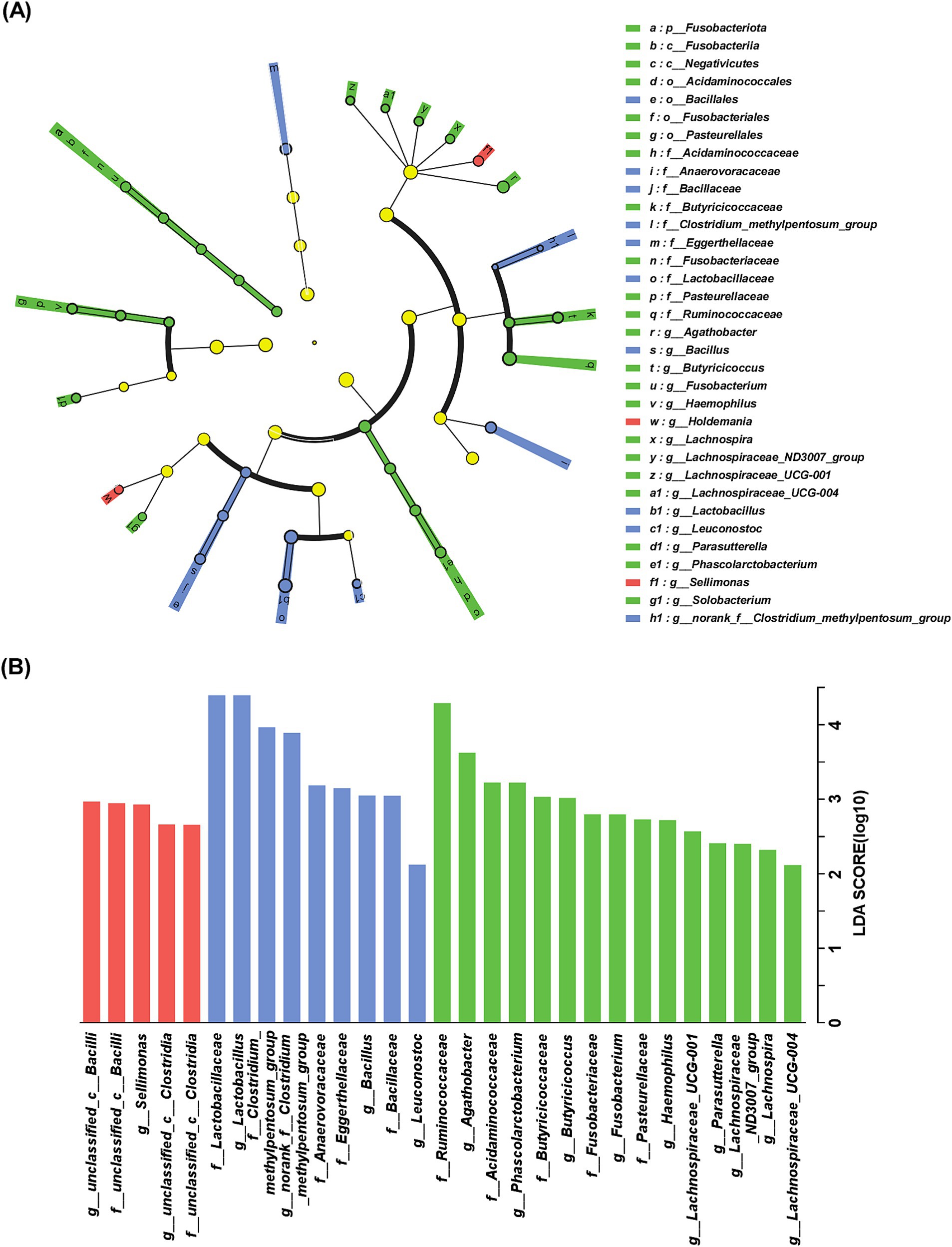
Figure 2. Characteristic of gut bacteria in AD patients. (A) Cladogram of distinguished bacterial populations among of mAD patients, sAD patients and HC groups from phylum to genus. (B) LDA scores of gut microbiota among sAD patients, mAD patients and HC groups. Only taxa with LDA score > 2 and p < 0.05 are listed. The red bar chart represented the bacteria that were more abundant in sAD patients, the blue bar chart represented a higher abundance of bacteria in mAD patients and the green bar chart represented a higher abundance of bacteria in HC groups.
3.3 Associations of gut bacteria and SCFAs with specific cognitive domains of AD patients
As shown in Figure 3, the abundance of Butyricicoccus was positively associated with abstraction but negatively associated with orientation in mAD patients (p < 0.05). The abundance of Lachnospiraceae_UCG-004 was positively associated with attention, language, orientation in AD patients (p < 0.05). Furthermore, the abundance of Sellimonas exhibited negative correlations with MoCA items in sAD patients, while showed opposite correlation in mAD patients. As for SCFAs, the levels of isobutyric acid and isovaleric acid were both significantly negatively correlated with abstraction. Additionally, the attention of AD patients was significantly positively associated with level of propanoic acid.
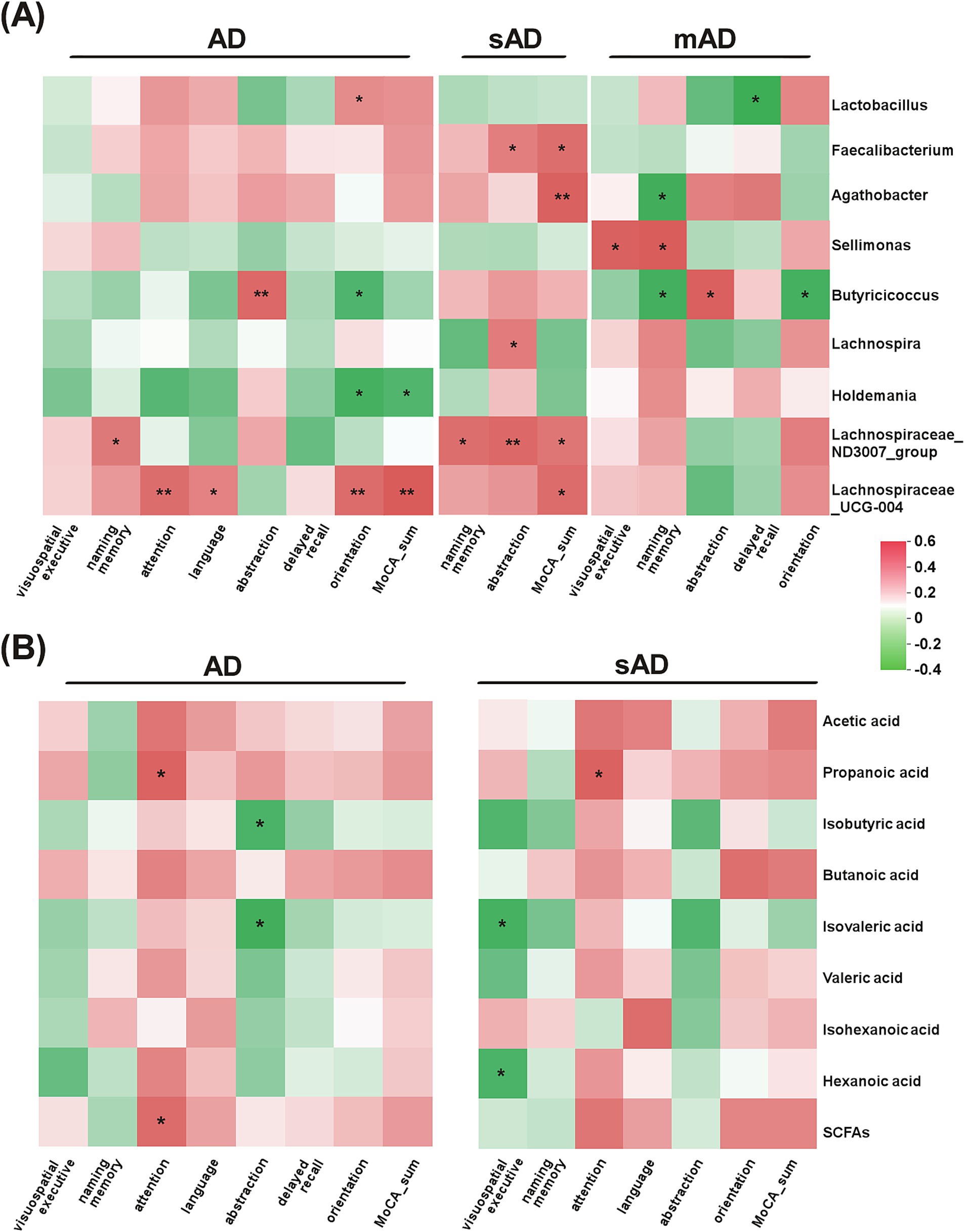
Figure 3. Associations of gut bacteria and SCFAs with specific cognitive domains of AD patients. (A) The heatmap of Spearman rank correlation analysis between the subitems of MoCA and gut microbiota in AD patients, sAD patients and mAD patients, respectively. (B) The heatmap of Spearman rank correlation analysis between the subitems of MoCA and metabolites in AD patients and sAD patients, respectively. Red indicated positive associations and green indicated negative associations. *p < 0.05, **p < 0.01.
3.4 Prediction of specific cognitive domains in AD patients
As shown in Figure 4, the discriminating models based on the characteristic and correlated bacteria, such as Lactobacillus, Butyricicoccus and Lachnospiraceae_UCG-004 could effectively distinguish low orientation from high orientation (AUC = 0.891, 95% CI: 0.77–1.0, p < 0.01). Moreover, Butyricicoccus and Agathobacter showed a good distinction between high and low abstraction in AD patients (AUC: 0.797, 95% CI: 0.643–0.952, p = 0.007), when combining isobutyric acid and isovaleric acid, the AUC improved to 0.839 (95% CI: 0.667–1.0, p = 0.002) (See Supplementary Figures 1–3).
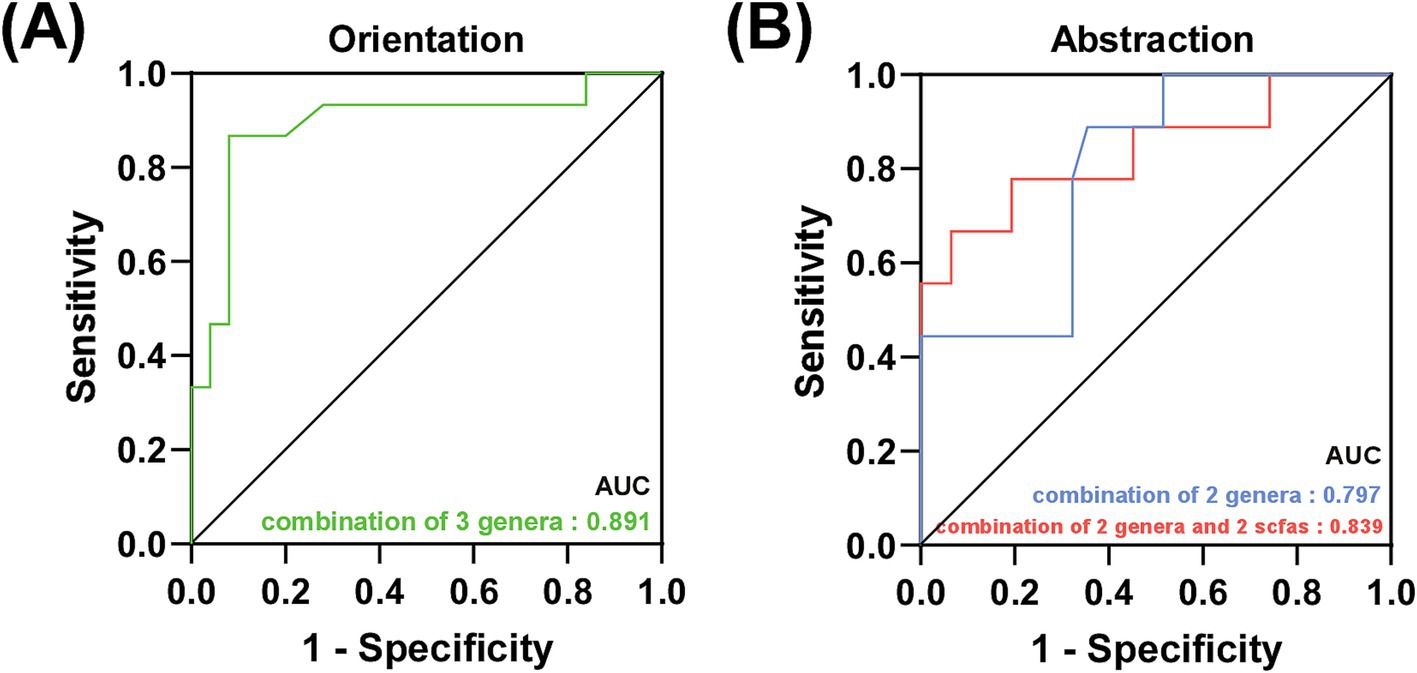
Figure 4. Prediction of specific cognitive domains in AD patients. (A) Green line represented the combination of 3 characteristic bacteria to distinguish the low orientation score from the high orientation score in AD patients. (B) Blue line represented the combination of 2 characteristic bacteria and red line represented the combination of 2 characteristic bacteria and 2 SCFAs to distinguish the low abstraction score from the high abstraction score in AD patients.
4 Discussion
In this study, we revealed the characteristics of gut microbiota and SCFAs in AD patients and demonstrated the relationships between characteristic bacteria and SCFAs and specific cognitive domains. Noteworthy, the abundance of Butyricicoccus was positively associated with abstraction, and the abundance of Lachnospiraceae_UCG-004 was positively associated with attention, language, orientation in AD patients. Moreover, the levels of isobutyric acid and isovaleric acid were both significantly negatively correlated with abstraction, and level of propanoic acid was significantly positively associated with the attention. Furthermore, ROC models based on the characteristic bacteria and SCFAs exhibited good sensitivity and specificity in predicting specific cognitive domains. These results suggested that gut microbiota and metabolite SCFAs could be used as convenient biomarkers to efficiently identify the global cognition and specific cognitive domains in AD patients.
An increasing number of studies have shown that the structure and composition of gut microbiota in subjects with cognitive impairment have undergone significant changes (Zhuang et al., 2018). A previous study revealed that the feces collected from patients with mild cognitive impairment (MCI) exhibited similar variation in alpha and beta diversities (Li et al., 2019). An animal study investigating antibiotics-induced gut dysbiosis built bridges between significantly decreased Chao1 index, depleted SCFAs relative abundance and impaired object recognition memory (Fu et al., 2024), implying casual relationships between disruption of gut microbiota and specific cognitive function. Additionally, mouse models with diabetes-induced cognitive impairment showed a significant increase in alpha diversity, and improved spatial learning and memory capabilities following intermittent fasting (Liu et al., 2020). These findings suggest a link between gut dysbiosis and cognitive impairment, warranting further exploration into the precise nature of their interaction and the underlying mechanisms involved.
In this study, the abundance of Butyricicoccus and Lachnospiraceae_UCG-004 could effectively distinguish between low and high orientation in AD patients, and Butyricicoccus and Agathobacter showed a good distinction between high and low orientation in AD patients. As cognitive impairment worsened, the abundance of Lachnospiraceae_UCG-004 gradually decreased (Yamashiro et al., 2024), consistent with our results. As for Lachnospiraceae_UCG-004, its changes in abundance were correlated with MoCA scores in this study. While previous studies had not directly linked Lachnospiraceae_UCG-004 with cognitive function, its decreased abundance in PD (Nishiwaki et al., 2020), varying levels in different subtypes of schizophrenia patients (Kowalski et al., 2023), and increased richness after supplementation of prebiotics (Rodriguez et al., 2020) suggested the contributing role of Lachnospiraceae_UCG_004 in maintaining microecological balance and overall health. In this study, Lachnospiraceae_UCG_004 showed significantly positive correlations with the sum of MoCA in both AD patients and sAD patients with consistent trends in mAD group, indicating the potential of Lachnospiraceae_UCG-004 as a biomarker for cognitive impairment. In this study, Agathobacter and Butyricicoccus had discriminative abilities in abstraction capability. Although there was a lack of direct research on the role of Butyricicoccus in abstraction capability, our heatmap analysis had indicated a significant effect of Butyricicoccus on cognitive abilities, particularly abstraction and orientation capabilities in mAD group.
Recent studies have emphasized the importance of SCFAs in regulating brain diseases, including AD (Yan et al., 2024; Ya-Xi et al., 2024). SCFAs are microbial metabolites, which are mainly composed of acetic acid, propionic acid and butyric acid, formate, valerate and caproate, and its production can be regulated by the gut microbiota (LeBlanc et al., 2017). A growing body of evidence suggested that altered gut microbiota could lead to changes in SCFAs levels, which were closely related to the pathogenesis and clinical manifestations of AD. The changes in SCFAs production were directly associated with alterations in the gut microbiota (Cipe et al., 2015; Zhu et al., 2011). In this study, the levels of isobutyric acid and isovaleric acid were significantly negatively correlated with abstraction, and the level of propanoic acid was significantly positively associated with attention of AD patients. In addition, the diagnostic model of AD was established using characteristic bacteria or the combination of SCFAs could distinguish abstraction in AD patients, and the AUC area had satisfactory prediction effect. As for isovaleric acid, a previous study had revealed the impaired Na+/K+-ATPase activity in cerebral cortex in the pathogenesis of isovaleric acidemia (Ribeiro et al., 2007) and the level of isovaleric acid was re-reduced after supplementation of bioactive peptide extracted from walnut protein with amended cognitive function in dementia mice (Wang et al., 2019). It was reported that the levels of propionate and isobutyric acid were decreased in AD mice (Zheng et al., 2019). A growing number of studies have demonstrated a link between increased propionate and brain disorders. Propionate had adverse effects on autism (Frye et al., 2017), depression (Szczesniak et al., 2016) and social behavior in a rodent model of autism spectrum disorder (Shams et al., 2019). SCFAs, generally considered beneficial to human physiology and exert beneficial effects through various mechanisms, linked the brain function via the microbiota-gut-brain axis. Considering that SCFAs are only related to specific cognitive domains, such as abstraction, orientation and attention, further study to explore the detailed causative relationship between SCFAs and cognitive domains is needed.
Several limitations of this study should be noted. Firstly, the sample size was small, potentially impacting the reliability of the conclusions drawn. Secondly, due to practical constraints, it was challenging to categorize patients based on individual cognitive domains. Thirdly, our study solely focused on assessing the concentration of SCFAs in fecal samples, without incorporating blood samples. Despite these limitations, our study still identified several genera correlated with specific cognitive function and showed the potential diagnostic and therapeutic ability of gut microbiota.
To conclude, these findings revealed the signatures gut bacteria and metabolite SCFAs of AD patients, and demonstrated the correlations between theses characteristic bacteria and SCFAs and specific cognitive domains, highlighting that gut microbiota and SCFAs could be used as potential biomarkers to efficiently identify the global cognition and specific cognitive domains in AD patients.
Data availability statement
The datasets presented in this study can be found in online repositories. The names of the repository/repositories and accession number(s) can be found at: https://www.ncbi.nlm.nih.gov/, PRJNA1174882.
Ethics statement
The studies involving humans were approved by Ethics Committee of the Second Affiliated Hospital of Wenzhou Medical University. The studies were conducted in accordance with the local legislation and institutional requirements. The participants provided their written informed consent to participate in this study.
Author contributions
QC: Writing – original draft, Investigation. JSh: Investigation, Writing – original draft. GY: Data curation, Software, Writing – review & editing. HX: Data curation, Software, Writing – review & editing, Validation. SY: Writing – review & editing, Investigation. JX: Investigation, Writing – review & editing. JL: Writing – review & editing, Writing – original draft. JSu: Funding acquisition, Writing – original draft.
Funding
The author(s) declare that financial support was received for the research, authorship, and/or publication of this article. This work was supported by Key Technology Research and Development Project of Wenzhou Municipality (No. ZY2022004).
Conflict of interest
The authors declare that the research was conducted in the absence of any commercial or financial relationships that could be construed as a potential conflict of interest.
Publisher’s note
All claims expressed in this article are solely those of the authors and do not necessarily represent those of their affiliated organizations, or those of the publisher, the editors and the reviewers. Any product that may be evaluated in this article, or claim that may be made by its manufacturer, is not guaranteed or endorsed by the publisher.
Supplementary material
The Supplementary material for this article can be found online at: https://www.frontiersin.org/articles/10.3389/fnagi.2024.1478557/full#supplementary-material
Footnotes
References
Amaral, W. Z., Kokroko, N., Treangen, T. J., Villapol, S., and Gomez-Pinilla, F. (2024). Probiotic therapy modulates the brain-gut-liver microbiota axis in a mouse model of traumatic brain injury. Biochim. Biophys. Acta Mol. basis Dis. 1870:167483. doi: 10.1016/j.bbadis.2024.167483
Balasa, M., Gelpi, E., Antonell, A., Rey, M. J., Sánchez-Valle, R., Molinuevo, J. L., et al. (2011). Clinical features and APOE genotype of pathologically proven early-onset Alzheimer disease. Neurology 76, 1720–1725. doi: 10.1212/WNL.0b013e31821a44dd
Barron, J. J., and Molofsky, A. V. (2021). A protective signal between the brain's supporting cells in Alzheimer's disease. Nature 595, 651–652. doi: 10.1038/d41586-021-01870-7
Boxer, A., and Sperling, R. (2023). Accelerating Alzheimer's therapeutic development: the past and future of clinical trials. Cell 186, 4757–4772. doi: 10.1016/j.cell.2023.09.023
Chilton, P. M., Ghare, S. S., Charpentier, B. T., Myers, S. A., Rao, A. V., Petrosino, J. F., et al. (2024). Age-associated temporal decline in butyrate-producing bacteria plays a key pathogenic role in the onset and progression of neuropathology and memory deficits in 3×Tg-AD mice. Gut Microbes 16:2389319. doi: 10.1080/19490976.2024.2389319
Cipe, G., Idiz, U. O., Firat, D., and Bektasoglu, H. (2015). Relationship between intestinal microbiota and colorectal cancer. World J. Gastrointest. Oncol. 7, 233–240. doi: 10.4251/wjgo.v7.i10.233
Drummond, E., and Wisniewski, T. (2017). Alzheimer's disease: experimental models and reality. Acta Neuropathol. 133, 155–175. doi: 10.1007/s00401-016-1662-x
Elahi, F. M., and Miller, B. L. (2017). A clinicopathological approach to the diagnosis of dementia. Nat. Rev. Neurol. 13, 457–476. doi: 10.1038/nrneurol.2017.96
Frye, R. E., Nankova, B., Bhattacharyya, S., Rose, S., Bennuri, S. C., and Mac Fabe, D. F. (2017). Modulation of immunological pathways in autistic and neurotypical lymphoblastoid cell lines by the enteric microbiome metabolite propionic acid. Front. Immunol. 8:1670. doi: 10.3389/fimmu.2017.01670
Fu, J., Qin, Y., Xiao, L., and Dai, X. (2024). Causal relationship between gut microflora and dementia: a Mendelian randomization study. Front. Microbiol. 14:1306048. doi: 10.3389/fmicb.2023.1306048
Gonçalves, P. B., Sodero, A. C. R., and Cordeiro, Y. (2024). Natural products targeting amyloid-β oligomer neurotoxicity in Alzheimer's disease. Eur. J. Med. Chem. 276:116684. doi: 10.1016/j.ejmech.2024.116684
Graff-Radford, J., Yong, K. X. X., Apostolova, L. G., Bouwman, F. H., Carrillo, M., Dickerson, B. C., et al. (2021). New insights into atypical Alzheimer's disease in the era of biomarkers. Lancet Neurol. 20, 222–234. doi: 10.1016/s1474-4422(20)30440-3
Hansson, O. (2021). Biomarkers for neurodegenerative diseases. Nat. Med. 27, 954–963. doi: 10.1038/s41591-021-01382-x
Harris, E. (2023). Brain cell biomarker for preclinical Alzheimer disease identified. JAMA 329, 2118–2119. doi: 10.1001/jama.2023.9928
Jang, S. E., Lim, S. M., Jeong, J. J., Jang, H. M., Lee, H. J., Han, M. J., et al. (2018). Gastrointestinal inflammation by gut microbiota disturbance induces memory impairment in mice. Mucosal Immunol. 11, 369–379. doi: 10.1038/mi.2017.49
Kandimalla, R., and Reddy, P. H. (2017). Therapeutics of neurotransmitters in Alzheimer's disease. J. Alzheimers Dis. 57, 1049–1069. doi: 10.3233/JAD-161118
Kazmi, S., and Hsiao, E. (2023). Extending genetic risk for Alzheimer's disease from host to holobiont. Cell 186, 690–692. doi: 10.1016/j.cell.2023.01.004
Kowalski, K., Żebrowska-Różańska, P., Karpiński, P., Kujawa, D., Łaczmański, Ł., Samochowiec, J., et al. (2023). Profiling gut microbiota signatures associated with the deficit subtype of schizophrenia: findings from a case-control study. Prog. Neuro-Psychopharmacol. Biol. Psychiatry 127:110834. doi: 10.1016/j.pnpbp.2023.110834
LeBlanc, J. G., Chain, F., Martin, R., Bermudez-Humaran, L. G., Courau, S., and Langella, P. (2017). Beneficial effects on host energy metabolism of short-chain fatty acids and vitamins produced by commensal and probiotic bacteria. Microb. Cell Factories 16:79. doi: 10.1186/s12934-017-0691-z
Li, B., He, Y., Ma, J., Huang, P., Du, J., Cao, L., et al. (2019). Mild cognitive impairment has similar alterations as Alzheimer's disease in gut microbiota. Alzheimers Dement. 15, 1357–1366. doi: 10.1016/j.jalz.2019.07.002
Liu, Z., Dai, X., Zhang, H., Shi, R., Hui, Y., Jin, X., et al. (2020). Gut microbiota mediates intermittent-fasting alleviation of diabetes-induced cognitive impairment. Nat. Commun. 11:855. doi: 10.1038/s41467-020-14676-4
Loh, J., Mak, W., Tan, L., Ng, C., Chan, H., Yeow, S., et al. (2024). Microbiota-gut-brain axis and its therapeutic applications in neurodegenerative diseases. Signal Transduct. Target. Ther. 9:37. doi: 10.1038/s41392-024-01743-1
McKhann, G. M., Knopman, D. S., Chertkow, H., Hyman, B. T., Jack, C. R., Kawas, C. H., et al. (2011). The diagnosis of dementia due to Alzheimer's disease: recommendations from the National Institute on Aging-Alzheimer's Association workgroups on diagnostic guidelines for Alzheimer's disease. Alzheimers Dement. 7, 263–269. doi: 10.1016/j.jalz.2011.03.005
Migliore, L., and Coppede, F. (2022). Gene-environment interactions in Alzheimer disease: the emerging role of epigenetics. Nat. Rev. Neurol. 18, 643–660. doi: 10.1038/s41582-022-00714-w
Nishiwaki, H., Ito, M., Ishida, T., Hamaguchi, T., Maeda, T., Kashihara, K., et al. (2020). Meta-analysis of gut dysbiosis in Parkinson's disease. Mov. Disord. 35, 1626–1635. doi: 10.1002/mds.28119
Pan, S., Zhang, Y., Ye, T., Kong, Y., Cui, X., Yuan, S., et al. (2024). A high-tryptophan diet alleviated cognitive impairment and neuroinflammation in APP/PS1 mice through activating aryl hydrocarbon receptor via the regulation of gut microbiota. Mol. Nutr. Food Res. 68:e2300601. doi: 10.1002/mnfr.202300601
Pinto, T. C. C., Machado, L., Bulgacov, T. M., Rodrigues-Júnior, A. L., Costa, M. L. G., Ximenes, R. C. C., et al. (2019). Is the Montreal cognitive assessment (MoCA) screening superior to the Mini-mental state examination (MMSE) in the detection of mild cognitive impairment (MCI) and Alzheimer's disease (AD) in the elderly? Int. Psychogeriatr. 31, 491–504. doi: 10.1017/s1041610218001370
Ribeiro, C. A., Balestro, F., Grando, V., and Wajner, M. (2007). Isovaleric acid reduces Na+, K+-ATPase activity in synaptic membranes from cerebral cortex of young rats. Cell. Mol. Neurobiol. 27, 529–540. doi: 10.1007/s10571-007-9143-3
Rodriguez, J., Neyrinck, A. M., Zhang, Z., Seethaler, B., Nazare, J. A., Robles Sánchez, C., et al. (2020). Metabolite profiling reveals the interaction of chitin-glucan with the gut microbiota. Gut Microbes 12:1810530. doi: 10.1080/19490976.2020.1810530
Scheltens, P., De Strooper, B., Kivipelto, M., Holstege, H., Chételat, G., Teunissen, C. E., et al. (2021). Alzheimer's disease. Lancet 397, 1577–1590. doi: 10.1016/s0140-6736(20)32205-4
Shams, S., Foley, K. A., Kavaliers, M., MacFabe, D. F., and Ossenkopp, K. P. (2019). Systemic treatment with the enteric bacterial metabolic product propionic acid results in reduction of social behavior in juvenile rats: contribution to a rodent model of autism spectrum disorder. Dev. Psychobiol. 61, 688–699. doi: 10.1002/dev.21825
Shi, S., Zhang, Q., Sang, Y., Ge, S., Wang, Q., Wang, R., et al. (2022). Probiotic Bifidobacterium longum BB68S improves cognitive functions in healthy older adults: a randomized, double-blind, placebo-controlled trial. Nutrients 15:51. doi: 10.3390/nu15010051
Skalny, A. V., Aschner, M., Santamaria, A., Filippini, T., Gritsenko, V. A., Tizabi, Y., et al. (2024). The role of gut microbiota in the neuroprotective effects of selenium in Alzheimer's disease. Mol. Neurobiol. doi: 10.1007/s12035-024-04343-w Epub ahead of print.
Sun, J., Xu, J., Ling, Y., Wang, F., Gong, T., Yang, C., et al. (2019). Fecal microbiota transplantation alleviated Alzheimer’s disease-like pathogenesis in APP/PS1 transgenic mice. Transl. Psychiatry 9:189. doi: 10.1038/s41398-019-0525-3
Szczesniak, O., Hestad, K. A., Hanssen, J. F., and Rudi, K. (2016). Isovaleric acid in stool correlates with human depression. Nutr. Neurosci. 19, 279–283. doi: 10.1179/1476830515Y.0000000007
Szopinska-Tokov, J., Dam, S., Naaijen, J., Konstanti, P., Rommelse, N., Belzer, C., et al. (2020). Investigating the gut microbiota composition of individuals with attention-deficit/hyperactivity disorder and association with symptoms. Microorganisms 8:406. doi: 10.3390/microorganisms8030406
Wang, M., Amakye, W. K., Guo, L., Gong, C., Zhao, Y., Yao, M., et al. (2019). Walnut-derived peptide PW5 ameliorates cognitive impairments and alters gut microbiota in APP/PS1 transgenic mice. Mol. Nutr. Food Res. 63:e1900326. doi: 10.1002/mnfr.201900326
Xiao-Hang, Q., Si-Yue, C., and Hui-Dong, T. (2024). Multi-strain probiotics ameliorate Alzheimer's-like cognitive impairment and pathological changes through the AKT/GSK-3β pathway in senescence-accelerated mouse prone 8 mice. Brain Behav. Immun. 119, 14–27. doi: 10.1016/j.bbi.2024.03.031
Yamashiro, K., Takabayashi, K., Kamagata, K., Nishimoto, Y., Togashi, Y., Yamauchi, Y., et al. (2024). Free water in gray matter linked to gut microbiota changes with decreased butyrate producers in Alzheimer's disease and mild cognitive impairment. Neurobiol. Dis. 193:106464. doi: 10.1016/j.nbd.2024.106464
Yan, H., Yi Feng, W., Jing, M., Rui Fang, Z., and Jin, Y. L. (2024). Short-chain fatty acids: important components of the gut-brain axis against AD. Biomed. Pharmacother. 175:116601. doi: 10.1016/j.biopha.2024.116601
Yang, X., Yu, D., Xue, L., Li, H., and Du, J. (2020). Probiotics modulate the microbiota-gut-brain axis and improve memory deficits in aged SAMP8 mice. Acta Pharm. Sin. B 10, 475–487. doi: 10.1016/j.apsb.2019.07.001
Ya-Xi, L., Ling-Ling, Y., and Xiu-Qing, Y. (2024). Gut microbiota-host lipid crosstalk in Alzheimer's disease: implications for disease progression and therapeutics. Mol. Neurodegener. 19:35. doi: 10.1186/s13024-024-00720-0
Zheng, J., Zheng, S. J., Cai, W. J., Yu, L., Yuan, B. F., and Feng, Y. Q. (2019). Stable isotope labeling combined with liquid chromatography-tandem mass spectrometry for comprehensive analysis of short-chain fatty acids. Anal. Chim. Acta 1070, 51–59. doi: 10.1016/j.aca.2019.04.021
Zhu, Z., Ma, X., Wu, J., Xiao, Z., Wu, W., Ding, S., et al. (2022). Altered gut microbiota and its clinical relevance in mild cognitive impairment and Alzheimer's disease: Shanghai aging study and Shanghai memory study. Nutrients 14:3959. doi: 10.3390/nu14193959
Zhu, M. L., Ni, Z. H., Yan, Y. C., Gu, Y., Xue, J., Chen, N., et al. (2011). Impact of therapeutic time on the prognosis in critically ill patients with acute renal failure who needed renal replacement therapy. Zhongguo Wei Zhong Bing Ji Jiu Yi Xue 23, 421–425. doi: 10.1007/s10570-010-9464-0
Keywords: Alzheimer’s disease, gut microbiota, short chain fatty acids, cognitive domains, microbial biomarkers
Citation: Chen Q, Shi J, Yu G, Xie H, Yu S, Xu J, Liu J and Sun J (2024) Gut microbiota dysbiosis in patients with Alzheimer’s disease and correlation with multiple cognitive domains. Front. Aging Neurosci. 16:1478557. doi: 10.3389/fnagi.2024.1478557
Edited by:
Shaohua Wang, Ohio University, United StatesReviewed by:
Jie Zhang, Huazhong University of Science and Technology, ChinaSantosh Kumar Prajapati, University of South Florida, United States
Copyright © 2024 Chen, Shi, Yu, Xie, Yu, Xu, Liu and Sun. This is an open-access article distributed under the terms of the Creative Commons Attribution License (CC BY). The use, distribution or reproduction in other forums is permitted, provided the original author(s) and the copyright owner(s) are credited and that the original publication in this journal is cited, in accordance with accepted academic practice. No use, distribution or reproduction is permitted which does not comply with these terms.
*Correspondence: Jiaming Liu, d3pqaWFtaW5nX2xpdUAxNjMuY29t; Jing Sun, c3Vuand6QDEyNi5jb20=
†These authors have contributed equally to this work