- 1Department of Biomedical Engineering and Science, Florida Institute of Technology, Melbourne, FL, United States
- 2Rotman Research Institute, Baycrest Health Sciences, Toronto, ON, Canada
- 3School of Biomedical Engineering, McMaster University, Hamilton, ON, Canada
- 4School of Computer Science, McGill University, Hamilton, ON, Canada
Blink-related oscillations (BROs) are newly discovered neurophysiological brainwave responses associated with spontaneous blinking, and represent environmental monitoring and awareness processes as the brain evaluates new visual information appearing after eye re-opening. BRO responses have been demonstrated in healthy young adults across multiple task states and are modulated by both task and environmental factors, but little is known about this phenomenon in aging. To address this, we undertook the first large-scale evaluation of BRO responses in healthy aging using the Cambridge Centre for Aging and Neuroscience (Cam-CAN) repository, which contains magnetoencephalography (MEG) data from a large sample (N = 457) of healthy adults across a broad age range (18–88) during the performance of a simple target detection task. The results showed that BRO responses were present in all age groups, and the associated effects exhibited significant age-related modulations comprising an increase in sensor-level global field power (GFP) and source-level theta and alpha spectral power within the bilateral precuneus. Additionally, the extent of cortical activations also showed an inverted-U relationship with age, consistent with neurocompensation with aging. Crucially, these age-related differences were not observed in the behavioral measures of task performance such as reaction time and accuracy, suggesting that blink-related neural responses during the target detection task are more sensitive in capturing aging-related brain function changes compared to behavioral measures alone. Together, these results suggest that BRO responses are not only present throughout the adult lifespan, but the effects can also capture brain function changes in healthy aging—thus providing a simple yet powerful avenue for evaluating brain health in aging.
1 Introduction
Spontaneous blinking occurs 15–20 times per minute, producing about 110 milliseconds of visual blackout each time (Tsubota et al., 1999; Volkmann et al., 1980). It is mediated by reciprocal activity of the orbicularis oculi and levator palpebrae superioris muscles in the face, creating a rapid closing and re-opening of the eyelids (Manning et al., 1983; Riggs et al., 1981). Although blinking has traditionally not been considered to be important in cognition, behavioral and neuroimaging studies are increasingly pointing to a potential link between the two: humans tend to blink when the attentional demand is low, such as at the ends of sentences when reading (Orchard and Stern, 1991) and during speaker pauses when listening to speech (Nakano and Kitazawa, 2010); adults have been shown to modulate their spontaneous blink behavior depending on environmental task demands (Hoppe et al., 2018; Oh et al., 2012a; Oh et al., 2012b); and blinking also activates key cortical regions associated with attentional switching in the brain (Nakano et al., 2013). These findings all suggest that spontaneous blinking also has important implications for cognitive processing.
Blink-related oscillations (BROs) are recently discovered neurophysiological responses associated with spontaneous blinking, and are believed to represent endogenous neural processes related to environmental monitoring and awareness as the brain evaluates new visual information that appears after eye re-opening (Bonfiglio et al., 2013, 2014; Liu et al., 2017, 2020; Liu et al., 2019b). BRO responses are distinct from the well-known blink oculomotor effects, and the BRO time domain activity is characterized by an increase in the delta-band (0.5–4 Hz) signal peaking approximately 250–300 ms after the blink (Liu et al., 2017, 2020). The associated cortical activations involve a wide network of posterior brain regions, including the dorsal and ventral visual streams engaged in sensory and perceptual processing (Hebart and Hesselmann, 2012), the hippocampus and parahippocampal gyri involved in spatial and episodic memory (Aminoff et al., 2013; Burgess et al., 2002), as well as the precuneus associated with numerous high-level cognitive processes such as episodic memory retrieval, visuospatial imagery, and self-related processing and awareness (Cavanna and Trimble, 2006; Gilboa et al., 2004; Kjaer et al., 2001; Liu et al., 2017; Liu et al., 2019b). In addition, BRO spectral effects encompass an early increase in signal power within the beta/low gamma (13–35 Hz) band, followed by a later and more prolonged reduction in the theta (4–8 Hz) and alpha (8–12 Hz) bands. These have been postulated to represent early sensory processing of visual information produced by blink events, followed by later higher-level episodic memory and information processing effects (Liu et al., 2017, 2020; Liu et al., 2019b).
To date, BRO responses have been demonstrated using both electroencephalography (EEG) and magnetoencephalography (MEG) across multiple task states, including resting (Liu et al., 2017; Liu et al., 2019a; Sattari et al., 2023), cognitive loading (Ghosh Hajra et al., 2021; Liu et al., 2019b), different sensory stimulation conditions (Liu et al., 2020), and complex task environments such as simulated flight (Page et al., 2024; Ziccardi et al., 2024). BRO effects have also been shown to be modulated by both task and environmental factors, as blinking during cognitive loading leads to reduced cortical activations compared to blinking during rest (Liu et al., 2019b). On the other hand, spontaneous blinking under different external sensory environments (e.g., ongoing visual vs. auditory inputs) results in altered temporal and spectral BRO response features that are consistent with the brain’s dynamic adaptation of blink processing in order to accommodate differential sensory requirements (Liu et al., 2020). Although prior studies had pointed to the potential usefulness of BRO responses in providing information about brain function, they all utilized small, relatively homogenous samples of healthy young participants, and little is known about how BRO responses may change in normal aging. Given that normal aging is known to have significant impact on brain structure and function (Harada et al., 2013; Oschwald et al., 2019)—and the precuneus region activated by BRO responses is especially sensitive to aging-related deficits such as cortical atrophy (Fjell et al., 2014), metabolic reduction (Choi et al., 2018), and decreased perfusion (Lee et al., 2009)—it is crucial to investigate BRO responses in aging in order to better understand this phenomenon. Notably, a prior study examining BRO responses in pilots during simulated flight maneuvers had demonstrated that BRO response characteristics were sensitive in detecting the effects of age in pilot performance (Ziccardi et al., 2024), but the use of a relatively low-density 14-channel EEG system limited the ability of that study to perform any in-depth characterization of age-related trajectories of BRO responses.
The present study aimed to investigate BRO effects in normal aging using a large sample of healthy adults across a broad age range. Specifically, we used data from the publicly available Cambridge Centre for Aging and Neuroscience (Cam-CAN) repository, which contains data for 700 cognitively normal healthy adults ranging in age from 18 to 88 (Shafto et al., 2014; Taylor et al., 2017). We hypothesized that BRO responses would be present and detectable throughout the adult lifespan, and that their characteristics would reflect brain changes in healthy aging. Additionally, given that BRO responses correspond to brain activity directly, we also hypothesized that BRO-based measurements would be superior to behavioral measurements such as reaction time and accuracy in detecting brain changes in healthy aging.
2 Methods
2.1 Participants
Data for this study were obtained from the Cam-CAN repository (Shafto et al., 2014; Taylor et al., 2017). Approximately 700 healthy adults were recruited for Phase 2 of the Cam-CAN study, ranging in age from 18 to 88. Volunteer recruitment was targeted to be gender-balanced between males and females, with equal distribution of individuals per age decile. A total of 631 participants had MEG data available; of these, we report results from 457 individuals (representing 72.4% of the total) following exclusions due to left-handedness (n = 63), noisy or corrupt MEG or electrooculogram (EOG) data (n = 18), missing structural MRI (n = 14), lack of demographic metrics such as vision test results (n = 18), and other exclusions related to blink behavior to be further detailed in Methods. Additional demographic and behavioral measures were also provided in the Cam-CAN data, including age, gender, number of years of continuous education, Mini-Mental State Exam (MMSE) score (Cummings et al., 2002), visual acuity using a modified Snellen eye test (Shafto et al., 2014), as well as reaction time and accuracy of task performance.
Participants were divided into four groups by age, comprising the youngest (YG, age 18−30), middle-young (MY, age 31−50), middle-old (MO, age 51−70), and oldest (OL, age 71−90) (Table 1). Demographic measures were compared across groups using one-way ANOVA, with Bonferroni correction for post-hoc multiple comparisons.
2.2 Experimental paradigm
The experimental paradigm consisted of a sensorimotor target detection task, which has been described elsewhere (Shafto et al., 2014). Briefly, participants viewed a screen with a central fixation cross, while short auditory and visual stimuli were presented either bimodally (93.8% of trials) or unimodally (6.2% of trials). Auditory stimuli consisted of 300-ms binaural tones at one of three frequencies (300, 600, or 1,200 Hz), while visual stimuli comprised bilateral checkerboards that appeared for 34 ms on either side of the fixation cross. The participants pressed a button with their right index finger whenever they detected the appearance of a target. The inter-stimulus interval ranged from 2 s to 26 s, and the duration of the overall task was 8 min 40 s.
2.3 Data acquisition
Data acquisition parameters for the Cam-CAN repository have also been detailed elsewhere (Taylor et al., 2017). Briefly, MEG data acquisition utilized a 306-channel Vectorview system (Elekta Neuromag, Helsinki, Finland), with participants in a seated position. Data were sampled at 1000 Hz with a bandpass filter of 0.03−330 Hz. Anatomical landmarks including the nasion, inion, and bilateral pre-auricular points were digitized to allow for co-registration between the MEG and MRI coordinate systems, and head position was also continuously monitored to allow for offline correction of head motion. Concomitant recordings of vertical and horizontal electrooculogram (vEOG and hEOG) were made, along with electrocardiogram (ECG) and task response times. High-resolution structural MRI was collected using a T1-weighted magnetization prepared rapid gradient echo (MPRAGE) sequence on a 3 T Siemens TIM Trio system with a 32-channel head coil, with 1 mm isotropic voxels.
2.4 Blink identification and behavioral assessments
Raw, continuous vEOG data were down-sampled to 250 Hz and visually inspected to remove artifactual channels, and blink identification was performed using a semi-automated, template matching procedure in line with prior works (Liu et al., 2017, 2020; Liu et al., 2019b). The vEOG signal was first bandpass-filtered at 0.1−20 Hz, and one blink instance that best represented a stereotypical blink was manually selected as template. This template was then convolved with the entire vEOG signal, and amplitude thresholding was applied to identify potential blink instances. In order to minimize contamination from adjacent blink events, temporal thresholding was also applied to quantify the time interval between adjacent blinks, then exclude any blink events that were <3 s apart. The total number of blinks was quantified prior to temporal thresholding to enable behavioral evaluation of blink rate. To ensure a sufficient number of blink trials for BRO extraction, only participants with more than three trials following temporal thresholding were included in further analysis, in accordance with prior studies (Liu et al., 2020; Liu et al., 2019b). A total of 63 participants were excluded due to frequent blinking, as insufficient number of blink trials remained following temporal thresholding.
Blink behavior was assessed via both qualitative and quantitative methods in line with previous literature (Liu et al., 2017, 2020; Liu et al., 2019b). For qualitative assessment, individual-level trial-averaged vEOG waveforms were normalized by their respective maximum amplitudes before averaging across subjects in each group to minimize bias due to differential voltages in the raw blink signal. For quantitative measures, morphological features were extracted corresponding to the height and width of different regions in the un-normalized individual-level blink waveforms (Figure 1A). The Cam-CAN dataset also provided task performance measurements in the form of reaction time and target detection accuracy, and these were compared across age groups using one-way ANOVA with Bonferroni correction for post-hoc multiple comparisons.
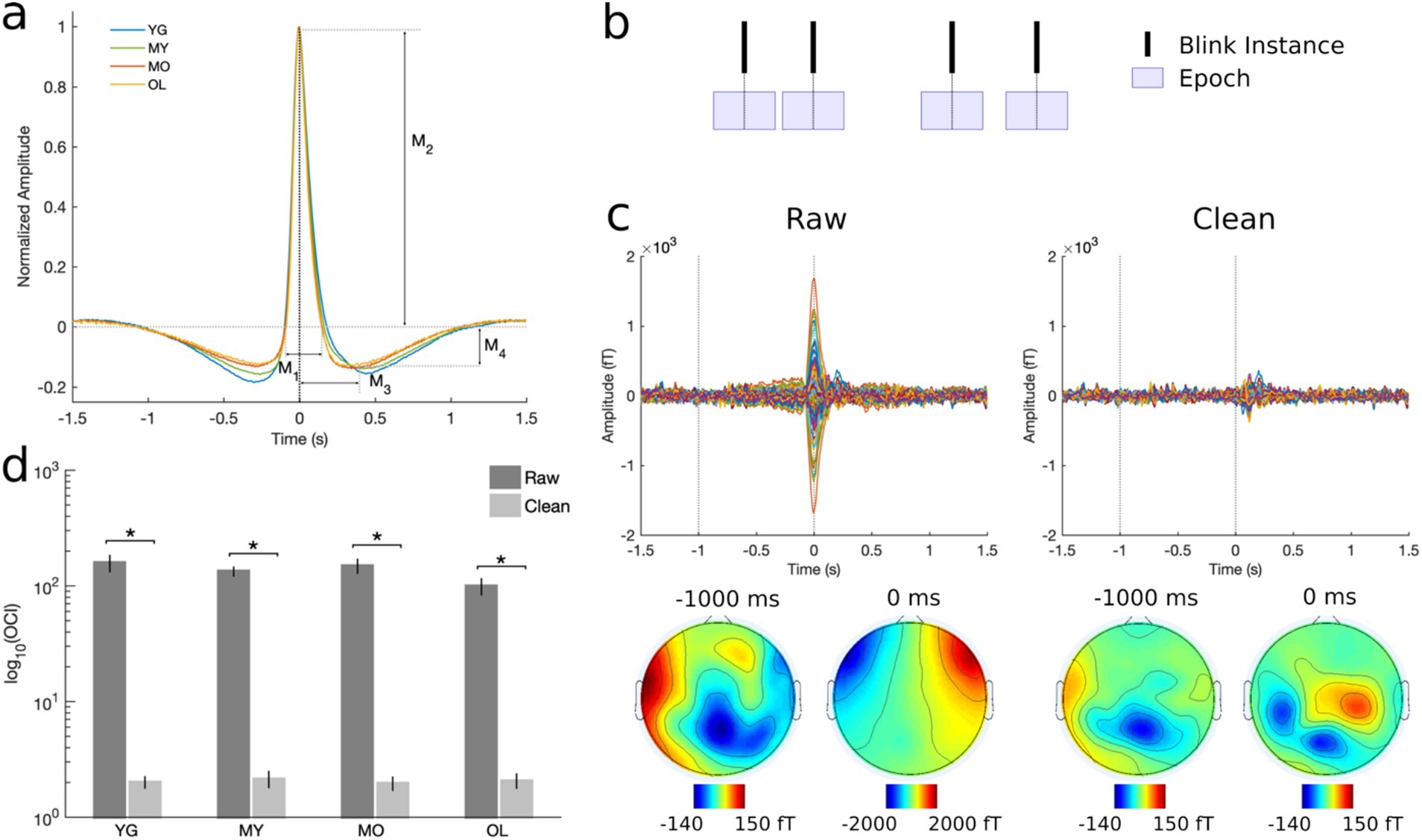
Figure 1. Preprocessing and artifact removal results. (A) Grand-averaged normalized vEOG blink traces showing the quantitative morphological features used to assess blink behavioral characteristics. M1 = positive peak width; M2 = positive peak amplitude; M3 = negative peak time; M4 = negative peak amplitude. Black dotted line at 0 ms denotes the moment of complete eye closure or T0. (B) Schematic illustration of data segmentation technique. BRO effects were assessed by segmenting data into 3-s epochs centered at 0 ms latency or T0. (C) Representative subject MEG data before and after artifact removal. Top panel shows all-channel BRO waveforms after averaging across all blink trials, while bottom panel shows corresponding scalp topographies. Dotted lines denote the latencies of maximum blink amplitude (0 ms) and pre-blink baseline (−1000 ms). (D) Ocular contamination index (OCI) results show 98% reduction in blink signal contribution after artifact removal. *p < 0.0001.
2.5 MEG preprocessing
We utilized the preprocessed MEG data from the Cam-CAN repository, in which temporal signal space separation (tSSS) had been applied to remove noise from both external sources and head position indicator coils, head motion was corrected, noisy channels reconstructed, and each dataset had been transformed to a common head position (Taulu et al., 2005; Taylor et al., 2017). Subsequent analyses utilized a combination of SPM12 (Henson et al., 2019), EEGLAB (Delorme and Makeig, 2004), and custom scripts in MATLAB (The Mathworks Inc.).
Continuous MEG data were down-sampled to 250 Hz and visually inspected to remove artifactual channels. Data were then notch-filtered at 50 Hz and 100 Hz to remove mains power (5 Hz bandwidth), and bandpass-filtered at 0.1−80 Hz using a zero-phase 4th-order butterworth filter. Independent component analysis (ICA) was performed using the InfoMax algorithm to identify and remove contamination due to environmental and physiological sources such as blinks, saccades, cardiac activity, muscle contraction, breathing, and movement based on their stereotypical characteristics (Delorme and Makeig, 2004; Ghosh Hajra et al., 2018; Hajra et al., 2020; Liu et al., 2019b; Makeig et al., 1996). For instance, the blink artifact involves a large positive spike in the time-domain event-related field signal occurring at blink latency, with the largest signals concentrated near the frontal eye regions. Following artifact removal, the cleaned continuous MEG data were subsequently segmented into 3-s epochs centered on the latency of maximum blink amplitude, or T0, to enable BRO extraction (Figure 1B).
2.6 Effectiveness of artifact removal
To ensure complete removal of artifact prior to BRO extraction, the effectiveness of the ICA-based artifact removal procedure was rigorously assessed using both quantitative and qualitative techniques (Liu et al., 2017, 2020; Liu et al., 2019b). Qualitative evaluation involved visual inspection of the trial-averaged, individual-level data before and after artifact removal to ensure elimination of temporal and topographical features corresponding to ocular artifact. Quantitative assessment was performed by computing the ocular contamination index (OCI) for each dataset as the ratio of ocular signal contribution at the maximum blink latency (0 ms) relative to pre-blink baseline (−1000 ms) in accordance with previously published methods (Liu et al., 2020). Statistical comparisons were made using two-tailed, paired t-test between the raw and cleaned data.
2.7 Global field power
Time-domain BRO effects at the sensor level were measured using global field power (GFP) to quantify the spatial variance across channels (Liu et al., 2017; Skrandies, 1990). Cleaned continuous data were bandpass-filtered into the delta band (0.5−4 Hz), segmented into 3-s blink epochs, averaged across trials, and GFP was derived for each participant before grand-averaging across subjects. Windows of interest were identified corresponding to salient features in the grand-averaged waveform (Figure 2A), including the two post-blink peaks (P1, 50−180 ms and P2, 220−350 ms), a pre-blink baseline (B1, -1300 to -1100 ms), and a blink preparation interval just before blink onset (B2, -350 to -150 ms). Mean GFP magnitudes were computed within these windows of interest for each individual. The latencies of the P1 and P2 peaks were measured for each participant and averaged across subjects for each group. For individuals with only one post-blink GFP peak, only P2 latency was determined based on the grand-averaged GFP waveform, while P1 was excluded from group averaging. Quantitative measures were statistically compared across groups using one-way ANOVA with Bonferroni correction.
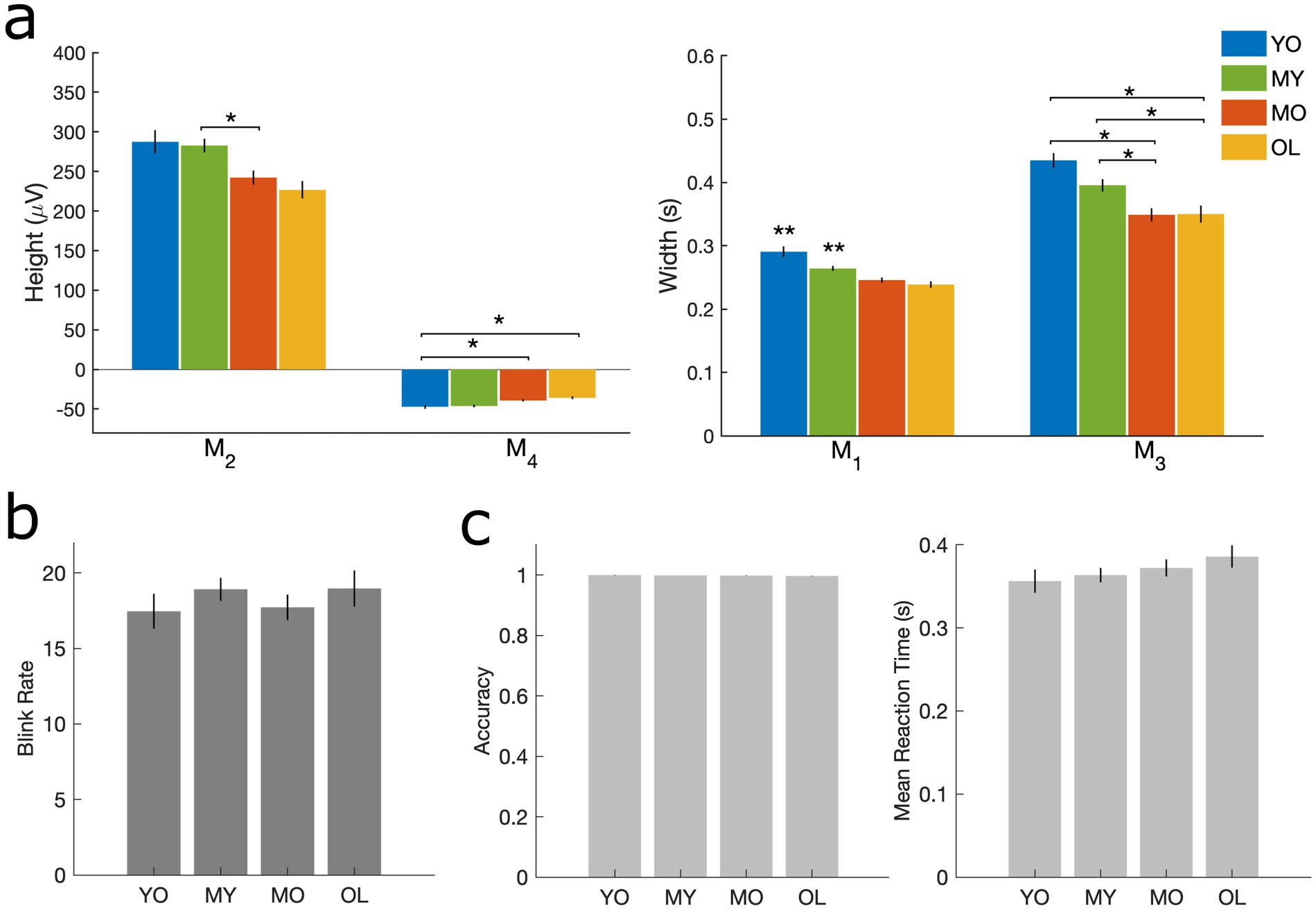
Figure 2. Measures corresponding to blink morphology and task performance. (A) Height and width of vEOG waveform. M2 = positive peak amplitude; M4 = negative peak amplitude; M1 = positive peak width; M3 = negative peak time. (B) Number of blinks per minute. (C) Target detection task performance, including accuracy (left) and mean reaction time (right). All results are computed at the individual level and presented as mean ± SE across participants. *p < 0.05 as indicated. **p < 0.05 compared to all other groups.
2.8 Source localization
Source localization was performed using whole-brain analysis in SPM12 according to previously published procedures (Liu et al., 2017; Liu et al., 2019b). Following standard forward modeling using a single-shell spherical head model, source reconstruction was performed using minimum norm estimates with group constraints during inversion to improve source reliability across subjects (Hauk, 2004; Litvak and Friston, 2008). Source reconstruction used denoised, segmented, and trial-averaged data (0.5–80 Hz) over the entire 3-s epoch, and time-frequency contrast images were generated by averaging the estimated source activity over the delta frequency band and across the previously selected windows of interest corresponding to the two post-blink GFP peaks (P1, P2) and the pre-blink baseline (−1300 to −1100 ms). These contrast images were projected to a 3-dimensional source space, smoothed using a Gaussian kernel with 8 mm full-width at half-maximum, and entered into a mixed-effects general linear model (GLM) using two-way ANOVA, with time (i.e., B1 vs. P1, B1 vs. P2, B1 vs. B2) as a within-subject factor and age group as a between-subject factor. Individual participant age and visual acuity were incorporated as covariates in the model to account for inter-individual differences in participant vision and age.
2.9 Source-level spectral effects
Source-level timeseries were extracted using virtual electrodes positioned at coordinates centered within activation clusters located in the bilateral precuneus [MNI coordinates (8 −72 38), (−8 −76 46)]. Voxel time courses were smoothed over a spherical volume of interest with 5-mm radius, filtered to 0.5–45 Hz, and event-related spectral perturbation (ERSP) was computed using continuous wavelet transform (CWT) with the Morlet function and 6 cycles (Liu et al., 2017; Liu et al., 2019b; Makeig, 1993). CWT was performed for each trial and virtual electrode, and the log power was computed as the logarithm of the squared absolute values of the wavelet coefficients. Baseline correction was performed by subtracting from each trial the mean log power of the pre-blink baseline window defined as -1500 to -500 ms in line with prior works (Liu et al., 2017, 2020). Results were then trial-averaged for each individual and grand-averaged in each group.
Blink-related effects were statistically assessed using a nonparametric permutation approach based on Monte Carlo estimates (Liu et al., 2020; Maris and Oostenveld, 2007). A group-level paired t-test was first computed between the trial-averaged log spectral power in the pre-blink and post-blink intervals at each frequency and time point, with p < 0.05 for two-tailed significance threshold. The data were then randomly permuted between the two intervals for each frequency, and new T-statistic values were derived. This was repeated 1,000 times, and a distribution of permuted T-values was generated. The true T statistic between the pre-blink and post-blink intervals was then compared to the permuted distribution to determine probabilities, and results were deemed significant if p < 0.05. This process was carried out separately for each age group to assess statistically significant blink-related spectral features.
Quantitative comparisons of spectral features in each frequency band were made by selecting pre- and post-blink intervals corresponding to known BRO effects, including those in the pre-blink interval [i.e., (−1000 −600), (−600 −300), and (−300 0) ms], and the post-blink interval [i.e., (0–300), (300–1000) ms]. Mean spectral values were calculated for each individual within each time window and frequency, and grand-averaged across participants. Statistical assessment was conducted using one-way ANOVA with age as a between-subject factor, with Bonferroni correction for post-hoc multiple comparisons.
3 Results
3.1 Artifact removal
Qualitative examination of BRO signals before and after artifact removal revealed that features such as a large signal spike at blink latency (0 ms or T0) and frontally concentrated topography that are consistent with ocular artifact were eliminated after denoising (Figure 1C). Additional quantitative results using OCI showed that the maximal blink signal contribution was reduced by more than 98% following artifact removal (Figure 1D). This is consistent with prior BRO literature (Liu et al., 2017, 2020), and indicates that these procedures effectively removed ocular contamination due to blinking.
3.2 Behavior
Behavioral assessments showed that blink morphology exhibited age-related reductions in both the height (M2, M4) and width (M1, M3) of the vEOG waveform (Figure 2A). These features correspond approximately to the speed (M1) and amplitude (M2) of the blink itself, as well as the speed (M3) and amplitude (M4) of post-blink recovery (Iwasaki et al., 2005). Such reductions in blink kinematics are consistent with aging-related blepharoptosis or droopiness of the eyelid, which reduces the palpebral fissure width and limits the amplitude and peak velocity of blink-induced eyelid closure in older adults (Sun et al., 1997). However, no age effects were found in blink rate or task behavioral measures of mean reaction time and accuracy (Figures 2B,C), consistent with prior studies (Gruber et al., 2014; Sforza et al., 2008). These results indicate that normal aging did not alter the rate of blinking or performance in the target detection task, and the observed blink kinematic differences are likely the passive consequences of aging-related weakening in the eyelid muscles (Sun et al., 1997).
3.3 Sensor-level time-domain effects
GFP analysis was performed to examine BRO temporal effects at the sensor level. Results showed that BRO responses were present in all age groups, with morphological features consistent with prior literature (Bonfiglio et al., 2013; Liu et al., 2017, 2020; Liu et al., 2019a; Liu et al., 2019b). Three of the four groups exhibited a bifurcated morphology with two post-blink peaks P1 and P2 maximal at approximately 130 ms and 320 ms, respectively, while the youngest (YG) group exhibited a single peak at a comparable latency to that of P2 (Figure 3A). GFP amplitudes for P1, P2, and B2 were all increased compared to the B1 in each group (p < 0.0001), indicating that the BRO effects were significantly different from baseline for all ages (Figure 3B). In addition, all peaks showed age-related increase in GFP amplitude except B1, indicating that BRO sensor activity increased with age during both the post-blink peaks (P1 and P2) as well as the blink preparation interval (B2), and these effects were not due to potential age differences in baseline brain activity (B1, Figure 3B). Peak latency did not differ across groups for either of the post-blink peaks, indicating that the speed of BRO processing did not change with age (Figure 3C).
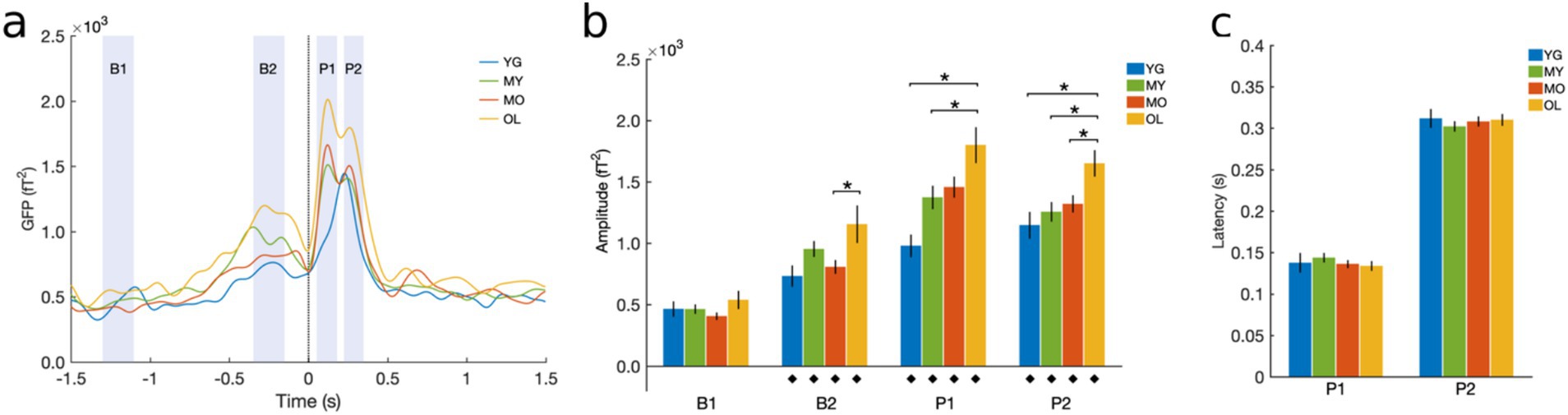
Figure 3. Sensor-level GFP results. (A) Grand-averaged GFP waveform. Black dotted line denotes latency of blink maximum or T0. Shaded regions denote windows of interest spanning different features of the BRO waveform, including post-blink BRO peaks (P1 and P2), blink preparation interval (B2), and pre-blink baseline (B1). (B) Mean GFP amplitude within the specified intervals of interest. (C) Latency of the post-blink peaks. Results computed for each participant and presented as mean ± SE across subjects. ♦p < 0.0001 compared to B1; *p < 0.05 as indicated.
3.4 Source-level effects
To determine blink-related cortical activations and the impact of aging, source localization was performed for all age groups. Results showed that BRO responses were present in all age groups, and the blink-related activations are in line with prior literature (Liu et al., 2017; Liu et al., 2019b). Compared to pre-blink baseline, BRO responses during the first window post-blink (ΔP1, 50–180 ms) led to increased activity in the bilateral occipital and posterior temporal regions along with the hippocampus, parahippocampal gyri, and fusiform (Figure 4A), while the second window (ΔP2, 220–350 ms) showed increased activity in the bilateral occipital, temporal, and posterior parietal regions along with the precuneus (Figure 4B). Minimal BRO-related activations were observed in the YG group, with only small occipital clusters in P1 which expanded in P2. The extent of BRO-induced cortical activations exhibited an inverted-U relationship with age, with the total number of activated voxels peaking in MO (Figure 5). No significant effect of age or visual acuity was found, indicating that the observed effects were not due to individual variations in age or vision. Additional comparisons examining blink-related activations during the blink preparation interval (i.e., B2–B1 contrast) did not produce any suprathreshold clusters, suggesting that blink preparatory neural processes did not lead to cortical activations significantly different from baseline, despite the observed sensor-level differences.
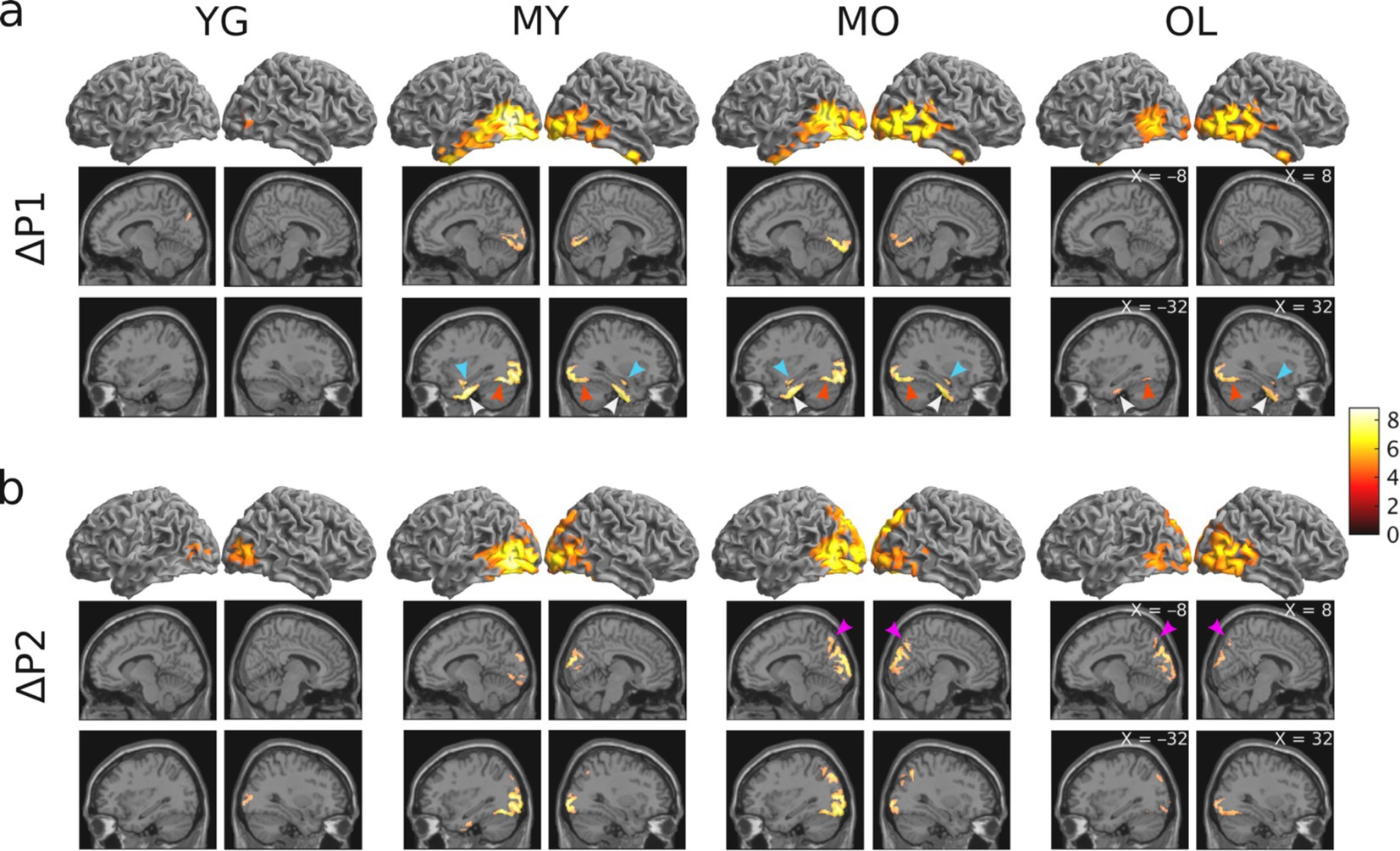
Figure 4. Results of whole-brain source localization analysis showing blink-related increase in brain activation for each age group (p < 0.05 FWE). (A) Blink-related cortical activations during the P1 peak compared to baseline (ΔP1 = P1 – B1 contrast). (B) Blink-related cortical activations during the P2 peak compared to baseline (ΔP2 = P2 – B1 contrast). Color bar denotes T-statistic values. Cyan arrows = hippocampus; white arrows = parahippocampal gyri; red arrows = fusiform; magenta arrows = precuneus.
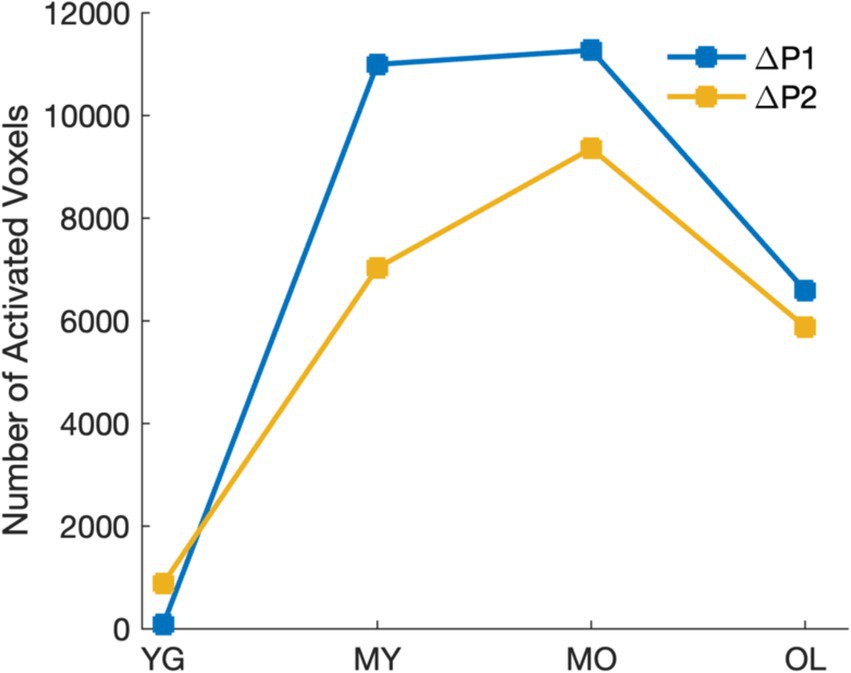
Figure 5. Extent of cortical activations in each post-blink peak showing inverted-U relationship with age.
Time-frequency analysis was undertaken to examine source-level BRO spectral effects within the bilateral precuneus. Results revealed an early blink-related increase in signal power, or event-related synchronization (ERS), in the beta/low gamma bands (13–35 Hz) during the 0–300 ms interval post blink. This is followed by a later and more prolonged power reduction, or event-related desynchronization (ERD), in the theta (4–8 Hz) and alpha (8–12 Hz) bands during the 400–1000 ms interval (Figure 6A). There was also a pre-blink ERD in the theta/alpha bands during the −600 to 0 ms interval before blink onset, with low-magnitude extension into the higher frequency bands. These effects were observed in all age groups and consistent with prior literature (Liu et al., 2020; Liu et al., 2019b), suggesting that BRO effects were present within the bilateral precuneus throughout the lifespan.
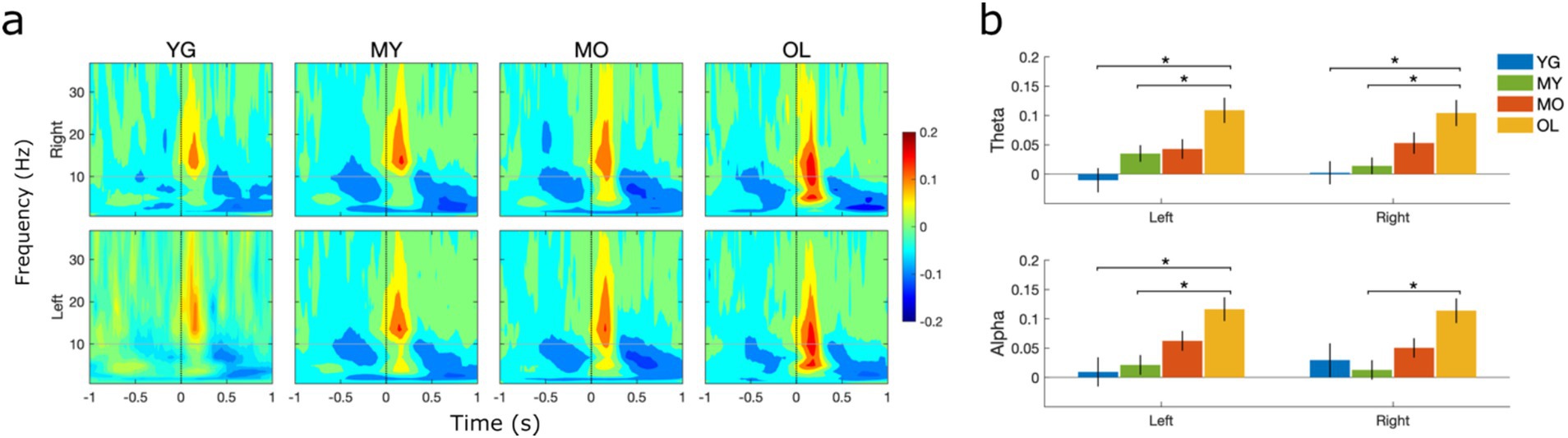
Figure 6. Source-level spectral effects within the left and right precuneus. (A) Grand-averaged log spectral power. Black dotted line denotes T0 or latency of blink maximum. Color bar denotes log power values. (B) Mean spectral power within the theta and alpha bands during the 0–300 ms post-blink interval. Values were computed at the individual level and presented as mean ± SE across participants. *p < 0.05.
To evaluate age differences in spectral effects within the bilateral precuneus, the mean spectral power in different frequency bands and time windows of interest were compared using one-way ANOVA. Results showed significant age-related increase in BRO signal power within the theta and alpha bands during the early post-blink interval (0–300 ms) in both the left and right precuneus (Figure 6B), while no age-related differences were observed in other intervals or frequency bands. These results suggest that the early post-blink theta- and alpha-band BRO effects are sensitive to brain changes in normal aging. Additionally, to ensure that the observed effects were not due to the particular grouping of participants by age in this study, further analyses were conducted to compare the precuneus spectral effects by dividing participants into both 10-year (Supplementary Figure S1) and 5-year age groupings (Supplementary Figure S2). The same age-related increase was found in both the other groupings.
4 Discussion
In this study, we conducted the first investigation of BRO responses in healthy aging using a large, cross-sectional sample of cognitively normal healthy adults across a wide age range (ages 18–88). Our results demonstrate the presence of BRO responses throughout the adult lifespan, and also show age-related modulations in BRO effects that suggest these blink-related neural responses can capture brain changes in healthy aging.
4.1 BRO responses are present across the adult lifespan
We first examined BRO effects at the sensor level using GFP to capture time-domain activity across all sensors. Results showed that GFP activity exhibited increased amplitude during two post-blink intervals compared to the pre-blink baseline, and the effects were observed in all age groups (Figure 3). This is consistent with prior literature (Liu et al., 2017, 2020; Liu et al., 2019a; Liu et al., 2019b), and confirms the presence of BRO responses across all age groups.
To determine the neuroanatomical origins of the BRO response, we performed source localization to assess cortical activations underlying the two post-blink peaks in GFP. Our results showed that BRO effects engaged brain regions including: (1) the bilateral occipital, posterior parietal, and inferior temporal regions consistent with the dorsal and ventral visual processing pathways (Hebart and Hesselmann, 2012); (2) the bilateral precuneus known to be associated with many aspects of high-level cognition including visuospatial processing, episodic memory retrieval, and self-related processing (Cavanna and Trimble, 2006; Gilboa et al., 2004; Kjaer et al., 2002; Wenderoth et al., 2005); (3) the hippocampus and parahippocampal gyri corresponding to spatial and non-spatial episodic memory and association processing (Aminoff et al., 2013; Burgess et al., 2002); (4) the fusiform gyri associated with complex image encoding and face perception (Machielsen et al., 2000; Weiner and Zilles, 2016); and (5) the bilateral anterior temporal lobes (ATL) associated with semantic cognition and the mental representation of meaning (Visser et al., 2010) (Figure 4). These effects are consistent with prior literature (Bonfiglio et al., 2013; Liu et al., 2017; Liu et al., 2019b), and demonstrate the presence of the BRO response throughout the adult lifespan.
At the source level, our analysis focused on spectral effects within the bilateral precuneus as these regions are known to be involved in BRO processing (Liu et al., 2017; Liu et al., 2019b). Our results showed that BRO responses exhibited beta/low gamma (13–35 Hz) band ERS during the early time window immediately after the blink (0–300 ms), followed by theta (4–8 Hz) and alpha (8–12 Hz) ERD during the later interval (300–1000 ms). These effects are also in line with prior studies (Liu et al., 2017, 2020; Liu et al., 2019a; Liu et al., 2019b), and confirm the presence of BRO responses within the precuneus in all age groups (Figure 6). Together, these results demonstrate that BRO responses are present throughout the adult lifespan, and the associated effects are detectable across the sensor and source levels.
4.2 BRO response amplitudes increase with healthy aging
We assessed age-related effects in BRO processing by examining sensor-level GFP as well as source-level activity within the bilateral precuneus as these regions are highly implicated in aging-related brain changes such as cortical atrophy and metabolic reduction (Fjell et al., 2009; Kalpouzos et al., 2009). Our results showed that both GFP amplitudes and BRO spectral power within the precuneus exhibited age-related increase (Figures 3, 6), while GFP peak latencies were not different with age. These results suggest that BRO responses are sensitive to brain function changes in healthy aging, but these changes did not alter the speed of information processing following blinking.
In the spectral domain, age-related increases were found in BRO theta and alpha ERS during the early post-blink interval (0–300 ms, Figure 6B). Blink-related theta and alpha oscillations have been postulated to represent episodic memory and information processing effects, respectively (Greenberg et al., 2015; Klimesch, 2012; Liu et al., 2019b; Matsumoto et al., 2013). In particular, alpha ERD is believed to index reduced cortical inhibition or increased neuronal excitability associated with active information processing (Kelly et al., 2006; Klimesch, 2012; Sauseng et al., 2009), while precuneus theta ERD has been shown to be crucial in facilitating associative episodic memory (Greenberg et al., 2015). These findings are in line with the postulated role of precuneus theta and alpha oscillations in BRO-related episodic memory and information processing effects. Given that the theta and alpha ERD both occur during the late post-blink interval (300–1000 ms), the preceding theta and alpha ERS during the early interval (0–300 ms) may indicate neural preparatory efforts in anticipation of upcoming blink processing. As such, the observed age-related increase in the early theta and alpha ERS may correspond to greater preparatory efforts being needed as a result of decreased neural efficiency with aging (Buckner, 2004; Gold et al., 2013; Mather and Harley, 2016; Nyberg et al., 2009, 2012; Vogel et al., 2005), which has been shown to compromise cognitive performance (Colcombe et al., 2005) and may thus impair the ability of the brain to process blink-related information. Such reduction in neural efficiency may also reflect underlying aging-related neurodegeneration such as cortical thinning, which is known to be prominent in the precuneus (Fjell et al., 2014; Storsve et al., 2014). Interestingly, the late theta and alpha ERD itself does not change with age (Supplementary Figure S5), suggesting that the blink-related neural processing remains stable with healthy aging.
No significant age-related differences were found in the pre-blink spectral effects. However, the observed pre-blink beta ERD is consistent with a previous study examining BRO effects during ongoing visual stimulation (Liu et al., 2020), in which beta ERD was postulated to represent the suppression of ongoing visual processes in preparation for processing the upcoming blink. The visual stimulus presentation employed in the target detection task in the current study likely also resulted in similar suppression of ongoing visual processes prior to blink onset. In a similar manner, the pre-blink alpha ERD in our study likely also reflects suppression of inhibition prior to blink onset, in preparation for processing the upcoming blink (Klimesch, 2012). The pre-blink theta ERD in our study may be related to expectation of upcoming sensory input, as a prior study also found similar effects preceding the onset of pain stimulus when individuals were expecting the input (Taesler and Rose, 2016). The absence of significant age differences in pre-blink effects suggests that these pre-blink BRO processes remain stable with healthy aging.
4.3 BRO cortical activations exhibit inverted-U relationship with age
We observed an inverted-U relationship with age in BRO cortical activations, such that the activation extent in both P1 and P2 intervals increases with age from the youngest group, peaks in the MO group (age 51–70), then decreases again in the oldest group (Figure 5). This suggests greater cortical recruitment for BRO processing with age, which is likely due to decreased neural efficiency with aging (Gold et al., 2013). These observed effects are consistent with the Scaffolding Theory of Aging and Cognition (STAC), in which the brain recruits additional neural resources as a compensatory mechanism in order to maintain cognitive performance when faced with age-related brain degeneration (Oschwald et al., 2019; Park and Reuter-Lorenz, 2009; Stern, 2002). As gray matter volume is known to decrease linearly with age beginning around the age of 20 (Fjell et al., 2013; Giorgio et al., 2010), this may help to account for the gradual expansion of BRO cortical activations with age. However, as the amount of functional loss that can be mitigated through neural compensation is also constrained by the total neural resources available at any given time (Oschwald et al., 2019; Park and Reuter-Lorenz, 2009; Stern, 2002), the accumulation of age-related neurodegeneration over time in the oldest group likely reduced the overall neural resources available in that group compared to MO, leading to decreased activations. This is also in line with the cognitive performance results from MMSE, in which OL showed lower scores compared to all other groups (Table 1). Additionally, the observed inverted-U relationship with age in cortical recruitment is also consistent with prior studies demonstrating a similar pattern of aging-related functional and structural changes in the brain, including episodic memory capacity (Rönnlund et al., 2005), cerebral white matter volume (Fjell et al., 2013), as well as hippocampal volume (Fjell et al., 2013). Although this inverted-U relationship is different than the age-related increase seen in the GFP and spectral effects, we postulate that the age-related increase may be due to the increased cortical “effort” in BRO processing as a result of decreased neural efficiency with aging (Colcombe et al., 2005), while the inverted-U relationship with age may reflect contributions from both decreased neural efficiency as well as functional neurocompensation in aging (Oschwald et al., 2019). The observed patterns of BRO changes with age in our study thus encompass both the neural “effort” in processing information, as well as the availability of neural resources which constrains the cumulative cortical effect. Nevertheless, further studies are needed to better elucidate the precise mechanisms of age-related changes in BRO processing.
Besides the age-related expansion of BRO cortical activations, there is also a staggered recruitment of cortical regions across the different age groups, in that the hippocampal and parahippocampal activation begins in MY (age 31–50) and remains present up to the oldest group, while the precuneus activation begins in MO (age 51–70) and remains present up to the oldest group (Figure 4). This suggests a potential systematic expansion of the neural recruitment for BRO processing with age, such that specific neural resources are gradually brought onboard to compensate for the accumulation of neurodegeneration with aging.
It is interesting that no significant cortical activations were observed in the precuneus, hippocampus, or parahippocampal regions for the youngest group (age 18–30) in our study. This is contrary to prior studies of BRO responses which had reported significant cortical engagement in these regions (Liu et al., 2017; Liu et al., 2019b). Nonetheless, there are experimental differences between those studies and ours which may have led to the observed differences. For instance, those studies utilized passive task paradigms with either no sensory input (Liu et al., 2017; Liu et al., 2019b), or only auditory or visual inputs in isolation (Liu et al., 2020), and none had required active task performance to maintain vigilance. On the other hand, the current study utilized data collected under dynamic environmental conditions with multisensory inputs, and also required active responses to indicate detection of short-duration targets. This ensures a higher level of participant vigilance in our study, and may have altered the neural resource allocation involved in BRO processing relative to tasks with less complex sensory inputs and lower vigilance states. Interestingly, although prominent age-related effects were seen in BRO processing during this task, the behavioral measures of task performance such as reaction time and accuracy were not different across age groups (Figure 2). This suggests that BRO neural processing during the target detection task has superior sensitivity in detecting changes due to normal aging compared to the task itself.
Finally, it should be noted that the use of minimum norm estimates for source localization in this study has some limitations, in that the technique has a tendency to bias towards the cortical surface due to its regularization parameter which requires minimum source power (Litvak and Friston, 2008). However, this approach has the advantage of requiring minimal assumptions about cortical sources (Hauk, 2004), and is in line with previous BRO studies (Liu et al., 2017; Liu et al., 2019b). Nonetheless, future studies should confirm the source activation findings using alternative approaches, such as different regularization techniques for minimum norm estimates (Hincapié et al., 2016; Vallarino et al., 2023) as well as spatial filtering with beamformer (Hillebrand et al., 2005; Hu et al., 2017). Additionally, given the present study is the first investigation of aging-related changes in BRO responses and uses a single cohort of a large sample of healthy adults, the observed findings should also be validated in future studies using other cohorts of participants.
5 Conclusion
We conducted the first investigation of blink-related oscillations in healthy aging using a large sample of healthy adults across a wide age range (age 18–88). Our results demonstrate that BRO responses are not only present throughout the adult lifespan, but there are also substantial age-related modulations that reflect underlying neural compensation. These age effects are not present in behavioral measures of task performance such as reaction time and accuracy, indicating that BRO responses have greater sensitivity in capturing brain changes in healthy aging compared to behavior alone. These findings significantly advance our understanding of the BRO phenomenon, and demonstrate the potential of blink-related neural processing for detecting brain changes in normal aging.
Data availability statement
Publicly available datasets were analyzed in this study. This data can be found at: Cambridge Centre for Aging and Neuroscience, https://cam-can.mrc-cbu.cam.ac.uk/dataset/.
Ethics statement
The studies involving humans were approved by the Cambridgeshire Research Ethics Committee (reference: 10/H0308/50). The studies were conducted in accordance with the local legislation and institutional requirements. The participants provided their written informed consent to participate in this study.
Author contributions
SGH: Conceptualization, Formal analysis, Methodology, Writing – original draft, Writing – review & editing. JM: Conceptualization, Methodology, Writing – review & editing. PK: Investigation, Writing – review & editing. CP: Investigation, Writing – review & editing. AS: Writing – review & editing. CL: Data curation, Conceptualization, Formal analysis, Funding acquisition, Investigation, Methodology, Project administration, Resources, Software, Supervision, Validation, Visualization, Writing – original draft, Writing – review & editing.
Group members of Cam-CAN Group
Cambridge Centre for Aging and Neuroscience, University of Cambridge, Cambridge, United Kingdom.
Funding
The author(s) declare that financial support was received for the research, authorship, and/or publication of this article. This study was supported by faculty startup funding for CL at the Florida Institute of Technology, and the Soupcoff Family Research Grant (grant # 737201815). CL was also partially supported by a fellowship from the Natural Sciences and Engineering Research Council of Canada (NSERC, grant # PDF 557827-21). Publication of this article was funded in part by the Open Access Subvention Fund and the John H. Evans Library.
Acknowledgments
The authors would like to thank the Cambridge Centre for Aging and Neuroscience for allowing us to use their data for this study, and also A. Fournier and T. Tian for their technical assistance.
Conflict of interest
The authors declare that the research was conducted in the absence of any commercial or financial relationships that could be construed as a potential conflict of interest.
Publisher’s note
All claims expressed in this article are solely those of the authors and do not necessarily represent those of their affiliated organizations, or those of the publisher, the editors and the reviewers. Any product that may be evaluated in this article, or claim that may be made by its manufacturer, is not guaranteed or endorsed by the publisher.
Supplementary material
The Supplementary material for this article can be found online at: https://www.frontiersin.org/articles/10.3389/fnagi.2024.1473178/full#supplementary-material
References
Aminoff, E. M., Kveraga, K., and Bar, M. (2013). The role of the parahippocampal cortex in cognition. Trends Cogn. Sci. 17, 379–390. doi: 10.1016/j.tics.2013.06.009
Bonfiglio, L., Olcese, U., Rossi, B., Frisoli, A., Arrighi, P., Greco, G., et al. (2013). Cortical source of blink-Related Delta oscillations and their correlation with levels of consciousness. Hum. Brain Mapp. 34, 2178–2189. doi: 10.1002/hbm.22056
Bonfiglio, L., Piarulli, A., Olcese, U., Andre, P., Arrighi, P., Frisoli, A., et al. (2014). Spectral parameters modulation and source localization of blink-related alpha and low-Beta oscillations differentiate minimally conscious state from vegetative state/unresponsive wakefulness syndrome. PLoS One 9:e93252. doi: 10.1371/journal.pone.0093252
Buckner, R. L. (2004). Memory and executive function in aging and ad: multiple factors that cause decline and reserve factors that compensate. Neuron 44, 195–208. doi: 10.1016/j.neuron.2004.09.006
Burgess, N., Maguire, E. A., and O’Keefe, J. (2002). The human Hippocampus and spatial and episodic memory. Neuron 35, 625–641. doi: 10.1016/S0896-6273(02)00830-9
Cavanna, A. E., and Trimble, M. R. (2006). The precuneus: a review of its functional anatomy and behavioural correlates. Brain J. Neurol. 129, 564–583. doi: 10.1093/brain/awl004
Choi, H., Kang, H., and Lee, D. S. (2018). Predicting aging of brain metabolic topography using variational autoencoder. Front. Aging Neurosci. 10:212. doi: 10.3389/FNAGI.2018.00212/BIBTEX
Colcombe, S. J., Kramer, A. F., Erickson, K. I., and Scalf, P. (2005). The implications of cortical recruitment and brain morphology for individual differences in inhibitory function in aging humans. Psychol. Aging 20, 363–375. doi: 10.1037/0882-7974.20.3.363
Cummings, J. L., Frank, J. C., Cherry, D., Kohatsu, N. D., Kemp, B., Hewett, L., et al. (2002). Guidelines for managing Alzheimer’s disease: part I. Assessment. Am. Fam. Physician 65, 2263–2272
Delorme, A., and Makeig, S. (2004). EEGLAB: an open source toolbox for analysis of single-trial EEG dynamics including independent component analysis. J. Neurosci. Methods 134, 9–21. doi: 10.1016/j.jneumeth.2003.10.009
Fjell, A. M., Walhovd, K. B., Fennema-Notestine, C., Mcevoy, L. K., Hagler, D. J., Holland, D., et al. (2009). Behavioral/systems/cognitive one-year brain atrophy evident in healthy aging. J. Neurosci. 29, 15223–15231. doi: 10.1523/JNEUROSCI.3252-09.2009
Fjell, A. M., Westlye, L. T., Grydeland, H., Amlien, I., Espeseth, T., Reinvang, I., et al. (2014). Accelerating cortical thinning: unique to dementia or universal in aging? Cereb. Cortex 24, 919–934. doi: 10.1093/CERCOR/BHS379
Fjell, A. M., Westlye, L. T., Grydeland, H., Amlien, I., Espeseth, T., Reinvang, I., et al. (2013). Critical ages in the life course of the adult brain: nonlinear subcortical aging. Neurobiol. Aging 34, 2239–2247. doi: 10.1016/J.NEUROBIOLAGING.2013.04.006
Ghosh Hajra, S., Liu, C. C., and Law, A. (2021). “Neural responses to spontaneous blinking capture differences in working memory load: assessing blink related oscillations with N-back task,” in Interntional Neuroergonomics Conference.
Ghosh Hajra, S., Liu, C. C., Song, X., Fickling, S. D., Cheung, T. P. L., and D’Arcy, R. C. N. (2018). Accessing knowledge of the “here and now”: a new technique for capturing electromagnetic markers of orientation processing. J. Neural Eng. 16:016008. doi: 10.1088/1741-2552/aae91e
Gilboa, A., Winocur, G., Grady, C. L., Hevenor, S. J., and Moscovitch, M. (2004). Remembering our past: functional neuroanatomy of recollection of recent and very remote personal events. Cereb. Cortex 14, 1214–1225. doi: 10.1093/cercor/bhh082
Giorgio, A., Santelli, L., Tomassini, V., Bosnell, R., Smith, S., De Stefano, N., et al. (2010). Age-related changes in grey and white matter structure throughout adulthood. NeuroImage 51:943. doi: 10.1016/J.NEUROIMAGE.2010.03.004
Gold, B. T., Kim, C., Johnson, N. F., Kryscio, R. J., and Smith, C. D. (2013). Lifelong bilingualism maintains neural efficiency for cognitive control in aging. J. Neurosci. 33, 387–396. doi: 10.1523/JNEUROSCI.3837-12.2013
Greenberg, J. A., Burke, J. F., Haque, R., Kahana, M. J., and Zaghloul, K. A. (2015). Decreases in theta and increases in high frequency activity underlie associative memory encoding. NeuroImage 114, 257–263. doi: 10.1016/j.neuroimage.2015.03.077
Gruber, N., Müri, R. M., Mosimann, U. P., Bieri, R., Aeschimann, A., Zito, G. A., et al. (2014). Effects of age and eccentricity on visual target detection. Front. Aging Neurosci. 5:101. doi: 10.3389/fnagi.2013.00101
Hajra, S. G., Gopinath, S., Liu, C. C., Pawlowski, G., Fickling, S. D., and Song, X. (2020). “Enabling event-related potential assessments using low-density electrode arrays: a new technique for denoising individual channel EEG data,” in IEMTRONICS 2020 - International IOT, Electronics and Mechatronics Conference, Proceedings.
Harada, C. N., Love, M. C. N., and Triebel, K. (2013). Normal cognitive aging. Clin. Geriatr. Med. 29:737. doi: 10.1016/J.CGER.2013.07.002
Hauk, O. (2004). Keep it simple: a case for using classical minimum norm estimation in the analysis of EEG and MEG data. NeuroImage 21, 1612–1621. doi: 10.1016/j.neuroimage.2003.12.018
Hebart, M. N., and Hesselmann, G. (2012). What visual information is processed in the human dorsal stream? J. Neurosci. 32:8107. doi: 10.1523/JNEUROSCI.1462-12.2012
Henson, R. N., Abdulrahman, H., Flandin, G., and Litvak, V. (2019). Multimodal integration of M/EEG and f/MRI data in SPM12. Front. Neurosci. 13:300. doi: 10.3389/FNINS.2019.00300/BIBTEX
Hillebrand, A., Singh, K. D., Holliday, I. E., Furlong, P. L., and Barnes, G. R. (2005). A new approach to neuroimaging with magnetoencephalography. Hum. Brain Mapp. 25, 199–211. doi: 10.1002/hbm.20102
Hincapié, A. S., Kujala, J., Mattout, J., Daligault, S., Delpuech, C., Mery, D., et al. (2016). MEG connectivity and power detections with minimum norm estimates require different regularization parameters. Comput. Intell. Neurosci. 2016:3979547. doi: 10.1155/2016/3979547
Hoppe, D., Helfmann, S., and Rothkopf, C. A. (2018). Humans quickly learn to blink strategically in response to environmental task demands. Proc. Natl. Acad. Sci. 115, 2246–2251. doi: 10.1073/pnas.1714220115
Hu, Y., Lin, Y., Yang, B., Tang, G., Liu, T., Wang, Y., et al. (2017). Deep source localization with magnetoencephalography based on sensor Array decomposition and beamforming. Sensors 17:1860. doi: 10.3390/S17081860
Iwasaki, M., Kellinghaus, C., Alexopoulos, A. V., Burgess, R. C., Kumar, A. N., Han, Y. H., et al. (2005). Effects of eyelid closure, blinks, and eye movements on the electroencephalogram. Clin. Neurophysiol. 116, 878–885. doi: 10.1016/J.CLINPH.2004.11.001
Kalpouzos, G., Chételat, G., Baron, J. C., Landeau, B., Mevel, K., Godeau, C., et al. (2009). Voxel-based mapping of brain gray matter volume and glucose metabolism profiles in normal aging. Neurobiol. Aging 30, 112–124. doi: 10.1016/J.NEUROBIOLAGING.2007.05.019
Kelly, S. P., Lalor, E. C., Reilly, R. B., and Foxe, J. J. (2006). Increases in alpha oscillatory power reflect an active retinotopic mechanism for distracter suppression during sustained visuospatial attention. J. Neurophysiol. 95, 3844–3851. doi: 10.1152/jn.01234.2005
Kjaer, T. W., Nowak, M., Kjaer, K. W., Lou, A. R., and Lou, H. C. (2001). Precuneus-prefrontal activity during awareness of visual verbal stimuli. Conscious. Cogn. 10, 356–365. doi: 10.1006/ccog.2001.0509
Kjaer, T. W., Nowak, M., and Lou, H. C. (2002). Reflective self-awareness and conscious states: PET evidence for a common midline parietofrontal core. NeuroImage 17, 1080–1086. doi: 10.1006/nimg.2002.1230
Klimesch, W. (2012). Alpha-band oscillations, attention, and controlled access to stored information. Trends Cogn. Sci. 16, 606–617. doi: 10.1016/j.tics.2012.10.007
Lee, C., Lopez, O. L., Becker, J. T., Raji, C., Dai, W., Kuller, L. H., et al. (2009). Imaging cerebral blood flow in the cognitively Normal aging brain with arterial spin labeling: implications for imaging of neurodegenerative disease. J. Neuroimaging 19, 344–352. doi: 10.1111/J.1552-6569.2008.00277.X
Litvak, V., and Friston, K. (2008). Electromagnetic source reconstruction for group studies. NeuroImage 42, 1490–1498. doi: 10.1016/j.neuroimage.2008.06.022
Liu, C. C., Ghosh Hajra, S., Cheung, T. P. L., Song, X., and D’Arcy, R. C. N. (2017). Spontaneous blinks activate the Precuneus: characterizing blink-related oscillations using magnetoencephalography. Front. Hum. Neurosci. 11:489. doi: 10.3389/fnhum.2017.00489
Liu, C. C., Ghosh Hajra, S., Fickling, S., Pawlowski, G., Song, X., and D’Arcy, R. C. N. (2019a). Novel signal processing technique for capture and isolation of blink-related oscillations using a low-density electrode Array for bedside evaluation of consciousness. IEEE Trans. Biomed. Eng. 67, 453–463. doi: 10.1109/TBME.2019.2915185
Liu, C. C., Ghosh Hajra, S., Pawlowski, G., Fickling, S. D., Song, X., and D’Arcy, R. C. N. (2020). Differential neural processing of spontaneous blinking under visual and auditory sensory environments: an EEG investigation of blink-related oscillations. NeuroImage 218:116879. doi: 10.1016/j.neuroimage.2020.116879
Liu, C. C., Ghosh Hajra, S., Song, X., Doesburg, S. M., Cheung, T. P. L., and D’Arcy, R. C. N. (2019b). Cognitive loading via mental arithmetic modulates effects of blink-related oscillations on precuneus and ventral attention network regions. Hum. Brain Mapp. 40, 377–393. doi: 10.1002/hbm.24378
Machielsen, W. C., Rombouts, S. A., Barkhof, F., Scheltens, P., and Witter, M. P. (2000). FMRI of visual encoding: reproducibility of activation. Hum. Brain Mapp. 9, 156–164. doi: 10.1002/(SICI)1097-0193(200003)9:3<156::AID-HBM4>3.0.CO;2-Q
Makeig, S. (1993). Auditory event-related dynamics of the EEG spectrum and effects of exposure to tones. Electroencephalogr. Clin. Neurophysiol. 86, 283–293.
Makeig, S., Bell, A. J., Jung, T.-P., and Sejnowski, T. J. (1996). “Independent component analysis of electroencephalographic data” in Advances in neural information processing systems. eds. D. Touretzky, M. Mozer, and M. Hasselmo, vol. 8 (New York, NY: The MIT Press), 145–151.
Manning, K. A., Riggs, L. A., and Komenda, J. K. (1983). Reflex eyeblinks and visual suppression. Percept. Psychophys. 34, 250–256. doi: 10.3758/BF03202953
Maris, E., and Oostenveld, R. (2007). Nonparametric statistical testing of EEG- and MEG-data. J. Neurosci. Methods 164, 177–190. doi: 10.1016/j.jneumeth.2007.03.024
Mather, M., and Harley, C. W. (2016). The locus Coeruleus: essential for maintaining cognitive function and the aging brain. Trends Cogn. Sci. 20:214. doi: 10.1016/J.TICS.2016.01.001
Matsumoto, J. Y., Stead, M., Kucewicz, M. T., Matsumoto, A. J., Peters, P. A., Brinkmann, B. H., et al. (2013). Network oscillations modulate interictal epileptiform spike rate during human memory. Brain J. Neurol. 136, 2444–2456. doi: 10.1093/brain/awt159
Nakano, T., Kato, M., Morito, Y., Itoi, S., and Kitazawa, S. (2013). Blink-related momentary activation of the default mode network while viewing videos. Proc. Natl. Acad. Sci. U. S. A. 110, 702–706. doi: 10.1073/pnas.1214804110
Nakano, T., and Kitazawa, S. (2010). Eyeblink entrainment at breakpoints of speech. Exp. Brain Res. 205, 577–581. doi: 10.1007/s00221-010-2387-z
Nyberg, L., Dahlin, E., Stigsdotter Neely, A., and Bäckman, L. (2009). Neural correlates of variable working memory load across adult age and skill: dissociative patterns within the fronto-parietal network. Scand. J. Psychol. 50, 41–46. doi: 10.1111/J.1467-9450.2008.00678.X
Nyberg, L., Lövdén, M., Riklund, K., Lindenberger, U., and Bäckman, L. (2012). Memory aging and brain maintenance. Trends Cogn. Sci. 16, 292–305. doi: 10.1016/J.TICS.2012.04.005
Oh, J., Han, M., Peterson, B. S., and Jeong, J. (2012a). Spontaneous eyeblinks are correlated with responses during the Stroop task. PLoS One 7:e34871. doi: 10.1371/journal.pone.0034871
Oh, J., Jeong, S.-Y., and Jeong, J. (2012b). The timing and temporal patterns of eye blinking are dynamically modulated by attention. Hum. Mov. Sci. 31, 1353–1365. doi: 10.1016/j.humov.2012.06.003
Orchard, L. N., and Stern, J. A. (1991). Blinks as an index of cognitive activity during Reading. Integr. Physiol. Behav. Sci. 26, 108–116. doi: 10.1007/BF02691032
Oschwald, J., Guye, S., Liem, F., Rast, P., Willis, S., Röcke, C., et al. (2019). Brain structure and cognitive ability in healthy aging: a review on longitudinal correlated change. Rev. Neurosci. 31:96. doi: 10.1515/REVNEURO-2018-0096
Page, C., Liu, C. C., Meltzer, J., and Ghosh Hajra, S. (2024). Blink-related oscillations provide naturalistic assessments of brain function and cognitive workload within complex real-world multitasking environments. Sensors 24:1082. doi: 10.3390/S24041082
Park, D. C., and Reuter-Lorenz, P. (2009). The adaptive brain: aging and neurocognitive scaffolding. Annu. Rev. Psychol. 60, 173–196. doi: 10.1146/annurev.psych.59.103006.093656
Riggs, L. A., Volkmann, F. C., and Moore, R. K. (1981). Suppression of the blackout due to blinks. Vis. Res. 21, 1075–1079. doi: 10.1016/0042-6989(81)90012-2
Rönnlund, M., Nyberg, L., Bäckman, L., and Nilsson, L.-G. (2005). Stability, growth, and decline in adult life span development of declarative memory: cross-sectional and longitudinal data from a population-based study. Psychol. Aging 20, 3–18. doi: 10.1037/0882-7974.20.1.3
Sattari, S., Kenny, R., Liu, C. C., Hajra, S. G., Dumont, G. A., and Virji-Babul, N. (2023). Blink-related EEG oscillations are neurophysiological indicators of subconcussive head impacts in female soccer players: a preliminary study. Front. Hum. Neurosci. 17:1208498. doi: 10.3389/FNHUM.2023.1208498/BIBTEX
Sauseng, P., Klimesch, W., Heise, K. F., Gruber, W. R., Holz, E., Karim, A. A., et al. (2009). Brain oscillatory substrates of visual short-term memory capacity. Curr. Biol. 19, 1846–1852. doi: 10.1016/j.cub.2009.08.062
Sforza, C., Rango, M., Galante, D., Bresolin, N., and Ferrario, V. F. (2008). Spontaneous blinking in healthy persons: an optoelectronic study of eyelid motion. Ophthalmic Physiol. Opt. 28:577. doi: 10.1111/j.1475-1313.2008.00577.x
Shafto, M. A., Tyler, L. K., Dixon, M., Taylor, J. R., Rowe, J. B., Cusack, R., et al. (2014). The Cambridge Centre for Ageing and Neuroscience (Cam-CAN) study protocol: a cross-sectional, lifespan, multidisciplinary examination of healthy cognitive ageing. BMC Neurol. 14:204. doi: 10.1186/s12883-014-0204-1
Skrandies, W. (1990). Global field power and topographic similarity. Brain Topogr. 3, 137–141. doi: 10.1007/BF01128870
Stern, Y. (2002). What is cognitive reserve? Theory and research application of the reserve concept. J. Int. Neuropsychol. Soc. 8, 448–460. doi: 10.1017/S1355617702813248
Storsve, A. B., Fjell, A. M., Tamnes, C. K., Westlye, L. T., Overbye, K., Aasland, H. W., et al. (2014). Differential longitudinal changes in cortical thickness, surface area and volume across the adult life span: regions of accelerating and decelerating change. J. Neurosci. 34, 8488–8498. doi: 10.1523/JNEUROSCI.0391-14.2014
Sun, W. S., Baker, R. S., Chuke, J. C., Rouholiman, B. R., Hasan, S. A., Gaza, W., et al. (1997). Age-related changes in human blinks: passive and active changes in eyelid kinematics. Investig. Ophthalmol. Vis. Sci. 38, 92–99.
Taesler, P., and Rose, M. (2016). Prestimulus Theta oscillations and connectivity modulate pain perception. J. Neurosci. 36, 5026–5033. doi: 10.1523/JNEUROSCI.3325-15.2016
Taulu, S., Simola, J., and Kajola, M. (2005). Applications of the signal space separation method. IEEE Trans. Signal Process. 53, 3359–3372. doi: 10.1109/TSP.2005.853302
Taylor, J. R., Williams, N., Cusack, R., Auer, T., Shafto, M. A., Dixon, M., et al. (2017). The Cambridge Centre for Ageing and Neuroscience (Cam-CAN) data repository: structural and functional MRI, MEG, and cognitive data from a cross-sectional adult lifespan sample. NeuroImage 144, 262–269. doi: 10.1016/j.neuroimage.2015.09.018
Tsubota, K., Kwong, K. K., Lee, T. Y., Nakamura, J., and Cheng, H. M. (1999). Functional MRI of brain activation by eye blinking. Exp. Eye Res. 69, 1–7. doi: 10.1006/exer.1999.0660
Vallarino, E., Hincapié, A. S., Jerbi, K., Leahy, R. M., Pascarella, A., Sorrentino, A., et al. (2023). Tuning minimum-norm regularization parameters for optimal MEG connectivity estimation. NeuroImage 281:120356. doi: 10.1016/J.NEUROIMAGE.2023.120356
Visser, M., Jefferies, E., and Lambon Ralph, M. A. (2010). Semantic processing in the anterior temporal lobes: a meta-analysis of the functional neuroimaging literature. J. Cogn. Neurosci. 22, 1083–1094. doi: 10.1162/JOCN.2009.21309
Vogel, E. K., McCollough, A. W., and Machizawa, M. G. (2005). Neural measures reveal individual differences in controlling access to working memory. Nature 438, 500–503. doi: 10.1038/nature04171
Volkmann, F. C., Riggs, L. A., and Moore, R. K. (1980). Eyeblinks and visual suppression. Science 207, 900–902.
Weiner, K. S., and Zilles, K. (2016). The anatomical and functional specialization of the fusiform gyrus. J. Neuropsychol. 83, 48–62. doi: 10.1016/j.neuropsychologia.2015.06.033
Wenderoth, N., Debaere, F., Sunaert, S., and Swinnen, S. P. (2005). The role of anterior cingulate cortex and precuneus in the coordination of motor behaviour. Eur. J. Neurosci. 22, 235–246. doi: 10.1111/j.1460-9568.2005.04176.x
Ziccardi, A., Van Benthem, K., Liu, C. C., Herdman, C. M., and Ghosh Hajra, S. (2024). Towards ubiquitous and nonintrusive measurements of brain function in the real world: assessing blink-related oscillations during simulated flight using portable low-cost EEG. Front. Neurosci. 17:1286854. doi: 10.3389/FNINS.2023.1286854
Keywords: aging, blinking, neurophysiology and brainwaves, blink-related oscillations (BROs), magnetoencephalography (MEG), precuneus
Citation: Ghosh Hajra S, Meltzer JA, Keerthi P, Pappas C, Sekuler ABCam-CAN Group and Liu CC (2025) Spontaneous blinking and brain health in aging: Large-scale evaluation of blink-related oscillations across the lifespan. Front. Aging Neurosci. 16:1473178. doi: 10.3389/fnagi.2024.1473178
Edited by:
Adrian W. Gilmore, University of Delaware, United StatesReviewed by:
Feng Liu, Stevens Institute of Technology, United StatesDeborah Zelinsky, Mind-Eye Institute, United States
Copyright © 2025 Ghosh Hajra, Meltzer, Keerthi, Pappas, Sekuler, Cam-CAN Group and Liu. This is an open-access article distributed under the terms of the Creative Commons Attribution License (CC BY). The use, distribution or reproduction in other forums is permitted, provided the original author(s) and the copyright owner(s) are credited and that the original publication in this journal is cited, in accordance with accepted academic practice. No use, distribution or reproduction is permitted which does not comply with these terms.
*Correspondence: Careesa Chang Liu, bGl1Y0BmaXQuZWR1