- 1School of Public Health, GuangDong Pharmaceutical University, Guangzhou, Guangdong, China
- 2Research Laboratory, The Third People's Hospital of Zhongshan, Zhongshan, Guangdong, China
- 3Department of Radiology, Zhongshan Torch Development Zone People's Hospital, Zhongshan, Guangdong, China
- 4Department of Imaging, The Third People's Hospital of Zhongshan City, Zhongshan, Guangdong, China
- 5Zhongshan Torch High-Tech Industrial Development Zone of Community Health Service Center, Zhongshan, Guangdong, China
- 6Department of Psychiatry, The Third People's Hospital of Zhongshan City, Zhongshan, Guangdong, China
- 7South China Research Center for Acupuncture and Moxibustion, Medical College of Acu-Moxi and Rehabilitation, Guangzhou University of Chinese Medicine, Guangzhou, Guangdong, China
Objective: Cerebrospinal fluid biomarkers are challenging to use for diagnosing mild cognitive impairment (MCI) in large populations, and there is an urgent need for new blood biomarkers. The aim of this study is to investigate whether astrocyte activation is correlated with hippocampal atrophy, and to assess the potential of glial fibrillary acidic protein (GFAP) as a biomarker for diagnosing MCI among community-dwelling older individuals.
Methods: This cross-sectional study included 107 older adults. The levels of GFAP in serum were measured, and the volumetric assessment of gray matter within hippocampal subregions was conducted using Voxel-Based Morphometry (VBM). The relationship between hippocampal subregion volume and blood biomarkers were analyzed using partial correlation. The effectiveness of blood biomarkers in differentiating MCI was assessed using a receiver operating characteristic (ROC) curve.
Results: We found that serum GFAP levels were significantly elevated in the MCI group compared to the cognitively normal (CN) group. Additionally, individuals with MCI exhibited a reduction gray matter volume in specific hippocampal subregions. Notably, the right dentate gyrus (DG) and right cornu ammonis (CA) subregions were found to be effective for distinguishing MCI patients from CN individuals. Serum levels of GFAP demonstrate a sensitivity of 65.9% and a specificity of 75.6% in differentiating patients with MCI from CN individuals.
Conclusion: Specific atrophy within hippocampal subregions has been observed in the brains of community-dwelling elderly individuals. Elevated levels of circulating GFAP may serve as a sensitive peripheral biomarker indicative of hippocampal-specific cognitive alterations in patients with MCI.
1 Introduction
Mild cognitive impairment (MCI) represents a transitional phase between normal aging and Alzheimer's disease (AD) (Song et al., 2021), typically characterized by a subtle decline in memory, thinking, and learning capabilities, along with neurological alterations (Gaur et al., 2023). Long-term studies indicate that approximately 20% of individuals with MCI will eventually progress to AD (Qin et al., 2023). The etiology of the condition remains elusive, and the absence of effective treatment imposes a significant economic burden on patients' families and society at large. Therefore, early diagnosis and intervention during the initial stages of the disease, specifically during the MCI phase, are crucial in mitigating or even averting its progression.
The etiology of MCI hypotheses primarily encompasses the amyloid hypothesis, the tau protein hyperphosphorylation hypothesis, and the neuroinflammation hypothesis (Bradfield, 2023). Exploring the underlying mechanisms of these hypotheses is crucial for further understanding the pathology of MCI and for identifying biomarkers that can facilitate early diagnosis. Biomarkers, including CSF Aβ42/Aβ40, p-Tau181, t-Tau, and neurofilament light chains (NfL), can facilitate the early diagnosis of AD-related MCI and monitor disease progression (Chatterjee et al., 2023). Nevertheless, their high costs and invasive nature limit their utilization in large-scale population screenings, necessitating the search for novel, easily accessible biomarkers effective in diagnosing MCI.
In recent years, with the progress of bioassay technologies, such as immunomagnetic subtraction, single-molecule immunoarray, and immunoprecipitation-mass spectrometry (IP-MS), have intensified the search for peripheral biomarkers. Some biomarkers that are non-invasive, specific, sensitive, reproducible and easy to collect have become an important part of current AD screening and early diagnosis research (Ehrenberg et al., 2020). Recently, glial fibrillary acidic protein (GFAP) has garnered attention as a potentially valuable biomarker for various neurological disorders, including multiple sclerosis, frontotemporal dementia, AD, and Parkinson's disease. Furthermore, GFAP has been found to possess diagnostic significance in predicting the progression of MCI to AD (Cicognola et al., 2021; Abdelhak et al., 2022; Heimfarth et al., 2022). GFAP is distinguished as a specific marker for astrocytes in the central nervous system (CNS) and ranks among the cell-specific markers within the CNS (Kamphuis et al., 2014).
In addition to serving as a cytoskeletal element and a marker of mature astrocytes, GFAP is crucial for astrocytic differentiation and reactivity (Li et al., 2020). Neuroinflammatory processes, such as reactive astrocyte hyperplasia, may be linked to pathological mechanisms underlying neurodegenerative diseases (Osborn et al., 2016). Activated astrocytes are implicated in neuroinflammatory changes in AD through the release of cytokines, inflammatory factors, nitric oxide, and reactive oxygen species (Heneka et al., 2010). Furthermore, research indicates that activated astrocytes are involved in the degradation or clearance of Aβ, hinting at their potential as a novel therapeutic target in AD (Ries and Sastre, 2016). While most studies have focused on comparing control groups with disease groups, fewer have explored the various stages of AD. Blood GFAP levels have been identified as a biomarker for predicting the progression of MCI to AD (Cicognola et al., 2021). Plasma GFAP amplitude changes not only exceed those observed in cerebrospinal fluid but also more accurately distinguish between Aβ-positive and Aβ-negative individuals (Benedet et al., 2021). However, validation and exploration of related mechanisms across diverse ethnic populations remain limited.
Activated astrocytes can influence brain structure, including brain volume and white matter density (Takahashi et al., 2020). These structural changes can be quantitatively assessed using Voxel-based morphometry (VBM) technology. VBM is a neuroimaging technique that analyzes and evaluates structural changes in brain regions by segmenting and extracting the gray and white matter of the brain and detecting volume changes in these tissues (Huang et al., 2023). Brain atrophy is evident in patients with AD, with the most significant atrophy observed in the hippocampal region, which is crucial for memory formation (Ritchie et al., 2018). Functionally, the hippocampus holds a pivotal role in encoding and consolidating both short-term and long-term memories, as well as spatial processing. Structurally, the hippocampus comprises several anatomically and functionally diverse subfields, and analyzing these subregions allows for a more precise elucidation of their relevance to cognitive changes (Xiao et al., 2023). Segmentation of the hippocampus in postmortem AD brains reveals a robust correlation between atrophy of the cornu ammonis (CA)1 and subiculum regions and the diagnosis of AD (Blanken et al., 2017). Additional studies have demonstrated that the dentate gyrus (DG)/CA3 and subiculum regions at the lower end of the hippocampus exhibit more extensive and evident atrophy (de Flores et al., 2015). In this study, we aimed to compare the structural alterations in the hippocampus and its subregions between MCI patients and healthy individuals using VBM, while also exploring the hippocampal subregions that preferentially undergo astrocyte-responsive proliferation in MCI patients.
Consequently, the present study postulated that serum GFAP could function as a biomarker for MCI diagnosis and sought to evaluate its importance alongside other relevant blood biomarkers (specifically, t-Tau, p-Tau181, Aβ42, Aβ40, NfL) in differentiating healthy individuals from those suffering from MCI. By comparing the hippocampal subregion volumes between MCI patients and CN individuals, our objective was to identify hippocampal subregions associated with aforementioned blood biomarkers during the stage of MCI. This research serves as an exploratory foundation for elucidating the pathological mechanisms underlying MCI.
2 Methods
2.1 Research design and participants
In May 2023, a simple random sampling was employed to select one district in Zhongshan, Guangdong Province. A total of 500 community-dwelling older persons aged 60 and above were randomly selected to participate in the questionnaire survey. The inclusion criteria encompassed individuals aged 60 years and above who did not have any known major cerebrovascular diseases, dementia or other pathological cognitive impairments, acute functional mental disorders (including schizophrenia or bipolar disorder), stroke history and traumatic brain injury. Patients with a history of alcohol abuse, drug addiction, and long-term use of medications that affect cognitive function, such as glucocorticoids, antipsychotic drugs, and sedative-hypnotic drugs, are excluded.
Of the 91 people diagnosed with MCI, 52 agreed to participate in the study. A total of 55 participants were matched by sex, age, and education as a control group to the cognitively normal people in the same area. A total of 107 people were actively involved in the study by undergoing blood collection procedures. The APOE ε4 allele frequency was also investigated in participants who provided blood samples. Image data were collected within 7 days after completing the questionnaire. Nevertheless, due to non-cooperation from some participants and contraindications for image collection, MRI data was successfully collected from 86 individuals.
MCI was diagnosed according to guidelines issued by the National Institute on Aging and the Alzheimer's Association (Albert et al., 2011). The subject or their relative has expressed concern about the subject's cognitive abilities and an objective assessment indicated impairment in cognitive function, yet the subject can still maintain independent daily functioning and without dementia, then the subject could be diagnosed with MCI. In this study, we utilized the Mini-Mental Status Examination (MMSE) scale to evaluate cognitive function, the Activities of daily living (ADL) scale to assess daily living functions, and diagnosed dementia in accordance with DSM-5 criteria.
All volunteers provided written informed consent prior to participation, and the Zhongshan Third People's Hospital Ethics Committee, China (reference number SSYLL20220401) provided approval for the study.
2.2 Assessment of cognitive and abilities of daily living
Mini-Mental Status Examination (MMSE) (Winblad et al., 2004; Jia et al., 2021) consists of 30 items, that assess five dimensions: orientation, memory, attention and calculation, recall ability, and language ability. The correct answer gets 1 point, while the wrong answer gets 0 points, for a total of 30 points. A higher score indicates better cognitive function. MCI was identified using education-specific cutoff points for the total scores on the MMSE, which were categorized as 19 or less for illiterate individuals, 22 or less for those with an elementary school education, and 26 or less for individuals with a middle school education or higher.
Activities of daily living scale (ADL) (Wade and Collin, 1988) comprises 14 items, each scored from 1 to 4, with a total score ranging from 14 to 56. If an individual scored 3 or more on two or more items, or had a total score of 22 or higher, they were deemed to be functionally impaired and were excluded.
2.3 Blood sample acquisition and processing
All participants fasted overnight (not <8 h), and blood was collected in both plain and EDTA-treated 5 ml vacutainer tubes and gently inverted and mixed. After collection, the specimens were placed in universal transport boxes, stored at 4°C, and transported to laboratory within 4 h of collection. Plasma was separated from EDTA-treated blood samples and serum was separated from anticoagulant-free blood samples by centrifugation at 3,000 rpm for 10 min at 4°C.
Serum GFAP concentrations were determined by Single molecule immune, using a commercial reagent kit and an AST-Dx90 fully automatic fluorescence immunoassay analyzer from Su zhou Yu Jin Biotechnology. The brief steps were to mix and incubate the sample with magnetic beads indicating the presence of captured antibodies. The antigen in the sample bound to the captured antibody to form an antibody antigen complex. Detection antibodies labeled with fluorescent dyes were added to the antibody antigen complex, and combined with the antibody-antigen complex to form a double antibody sandwich structure. A washing solution was used to remove excess substances such as unbound fluorescent dyes. Finally, the fluorescence intensity of magnetic particles was detected, and the GFAP concentration in the serum of the sample was calculated by comparing the standard curve. The levels of Aβ40, Aβ42, NfL, p-Tau181, and t-Tau in plasma were measured using Simoa® Neurology 3-Plex A Advantage Kit (N3PA, cat: 101995), Simoa® NF-Light v2 Advantage Kit (cat: 104073), Simoa® pTau-181 Advantage V2 Kit (cat: 103714), ALZpath Simoa® p-Tau 217 v2 assay Kit (cat: 104371) in Simoa HD-X Analyzer ™ (Quante Rix Corp.). The coefficient of variation for GFAP was 47.77%, 33.93% for Aβ40, 31.83% for Aβ42, 18.13% for NfL, 52.32% for p-Tau181, 37.9% for t-Tau, respectively.
2.4 Brain imaging
2.4.1 Image acquisition
All subjects underwent MRI scans using the same 3.0 Tesla Vida Siemens scanner (Siemens, Erlangen, Germany) with a 64-channel head coil. We used high-resolution 3D T1-weighted imaging sequences (3D magnetization prepared Fast Gradient Echo, MPRAGE). Scan parameters are as follows: repetition time (TR) = 2,000 ms, echo time (TE) = 2.26 ms, inversion time (TI) = 900 ms, flip angle, (FA) = 8°, field of view (FOV) = 256 × 256 mm, matrix = 256 × 256 mm, thickness = 1.0 mm, and voxel size = 1 × 1 × 1 mm3.
2.4.2 Image processing
Voxel-based morphometry (VBM) analysis was performed using the CAT12 toolbox (https://dbm.neuro.uni-jena.de/vbm/, version 12.7, r1742) and the Statistical Parametric Mapping 12 (SPM12) (http://www.fil.ion.ucl.ac.uk/spm/software/spm12/) with default parameters in MATLAB version R2016b (The MathWorks, Inc., Natick, MA, USA). All scans were visually checked for artifacts and anatomical abnormalities by a senior neuroradiologist prior to pre-processing. Data quality was controlled by two methods: (i) all DICOM format images were reviewed before data processing to exclude images with artifacts or brain parenchymal lesions; and (ii) the weighted average image and preprocessing quality of all data was ensured to be at least B or above. Total intracranial volume (TIV) data were extracted.
During preprocessing, all 3D T1-weighted images were converted from DICOM format to Neuroimaging Informatics Technology Initiative (NIfTI) format. The default settings of the “Segment Data” module in CAT12 were employed, and all steps recommended in the manual were followed. The images were subsequently segmented into gray matter (GM), white matter (WM), and cerebrospinal fluid (CSF). The diffeomorphic anatomical registration through exponentiated lie algebra (DARTEL) algorithm was used for spatial registration, segmentation, and normalization of the images (Ashburner, 2007). DARTEL works by aligning gray and white matter in the image, creats a high-resolution average template, and registers all data to this template. The data were then normalized to a standard brain in the Montreal Neurological Institute (MNI) space. Using the MNI 152 standard space of the DARTEL template, the voxel size of the final standardized image was 1.5 × 1.5 × 1.5 mm. Spatial normalization corrects for differences in overall brain shape between subjects and removes non-brain tissue, thereby facilitating local adaptive segmentation. At the same time, these normalized images correct for signal intensity inhomogeneities. The segmented images are modulated by the Jacobian determinant of the transformation matrix to correct for volume changes.
After normalization, the resulting images were checked for data quality using the CAT12 “Check sample homogeneity” module, which revealed no potential outliers in brain volume. Subsequently, the segmented GM images were smoothed using an 8 mm full width at half maximum (FWHM) Gaussian kernel. Smoothing ensured the validity of the statistical analysis by making the data more normally distributed and reducing the individual differences among all subjects. To eliminate artifacts at the gray matter/white matter boundary, an absolute gray matter threshold of 0.1 was applied.
In this study, we will focus on selecting the hippocampus and its subregions as brain regions of subcortical interest. Based on previous literature (Jung et al., 2021), considering the complex shape and anatomical variation of the hippocampal region, we used the probability diagram of the cell structure of the hippocampal region to divide it into the following five subregions: cornu ammonias (CA), dentate gyrus (DG), entorhinal cortex (EC), subiculum (Subc), and hippocampal-amygdaloid transition area (HATA). In order to minimize the partial volume effect, the subfields were combined as follows: CA1/CA2/CA3 were combined as CA; CA4 and DG were combined as DG (Amunts et al., 2005). A total of 10 hippocampal subregion masks were obtained from the left and right sides, and the Gray Matter Volume of 10 regions of interest was extracted for comparison between groups.
2.5 Statistical analyses
Statistical analysis was performed with GraphPad Prism V.9.0 and IBM SPSS Statistics V.26.0. Continuous variables were described as unadjusted means with SD. Logarithmic was applied to the variables that were not normally distributed. Categorical variables were given as percentages (%). The t-tests and chi-squared tests were used to assess differences between the two groups.
The Analysis of Covariance (ANCOVA) was employed to compare the means of the detected parameters between the MCI and CN groups. We performed a voxel-based morphometric analysis using general linear models in SPM12, which included unrelated covariates such as age, gender, education, and total intracranial volume to reduce potential confounders. The mean volume of hippocampal gray matter and the mean volume of subregion gray matter were compared between the MCI and CN group. The familywise error (FWE) method was applied for multiple comparison correction, with significance established at P < 0.05 and a clustering threshold of k = 10. A partial correlation analysis was performed to assess the relationship between blood biomarkers and hippocampal subregion volume, controlling for age, sex, education, APOE ε4 carrier status and TIV as covariates. The diagnostic performance of biomarkers and hippocampal subregions was compared using receiver operating characteristic (ROC) curve analysis and the sensitivity and specificity refer to the values at the optimal cut-off point, as determined by maximizing the Youden's index. DeLong's test was utilized to compare the Area Under the Curve (AUC) for different models. A P-value of <0.05 was regarded as significant.
3 Results
3.1 Participant characteristics
As can be seen from Table 1, the characteristics of the two groups are evenly distributed. MCI patients were recruited at a mean age of 73.0 ± 7.4 years old, and 82.7% were women. MCI group and CN group were equivalent in age, gender, education, BMI, and total Intracranial volume.
3.2 comparison of blood biomarkers between MCI and CN group
GFAP levels were significantly higher in the MCI group than in the CN group after adjusting for potential risk factors (Li et al., 2020; Marizzoni et al., 2023) for AD such as age, gender, education, and APOE ε4 allele carrier status (F = 4.71, P = 0.038) (Figure 1A). However, no significant group differences were observed in the NfL, p-Tau181, Aβ40 and Aβ42, and t-Tau levels (all P > 0.05) (Figures 1B–F).
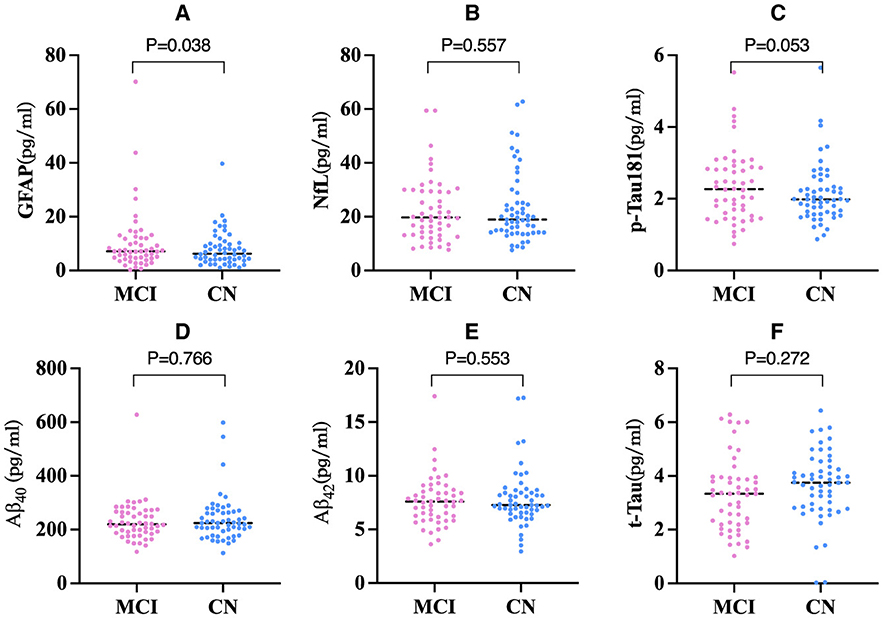
Figure 1. Comparison of blood biomarkers concentrations among two groups (n = 107). (A) Comparison of GFAP concentration among two groups. (B) Comparison of NfL concentration among two groups. (C) Comparison of p-Tau181 concentration among two groups. (D) Comparison of Aβ40 concentration among two groups. (E) Comparison of Aβ42 concentration among two groups. (F) Comparison of t-Tau concentration among two groups. P-values derived from ANCOVA with age, gender, education, and APOE ε4 carriers as covariates. ANCOVA, Analysis of covariance; The line segments depicted in the graph signify the median value of the data. GFAP, Glial Fibrillary Acidic Protein; NfL, Neurofilament Light; t-Tau, total Tau; p-Tau, phosphorylated tau; Aβ, amyloid-β.
3.3 Comparison of hippocampal subregion gray matter volume between MCI and CN group
A voxel-based morphometric analysis was performed using the general linear model in SPM12, with age, sex, education level, and total intracranial volume as covariates of no interest. The mean volumes of hippocampal gray matter and subregion gray matter were compared between the MCI group and control groups. The family-wise error (FWE) correction was applied to account for multiple comparisons. The results showed that all hippocampal subregions in the MCI group had lower volumes than those in the control group, with statistically significant differences observed in most subregions, except for the bilateral EC and rHATA. Please refer to Table 2 and Figure 2 for further details.
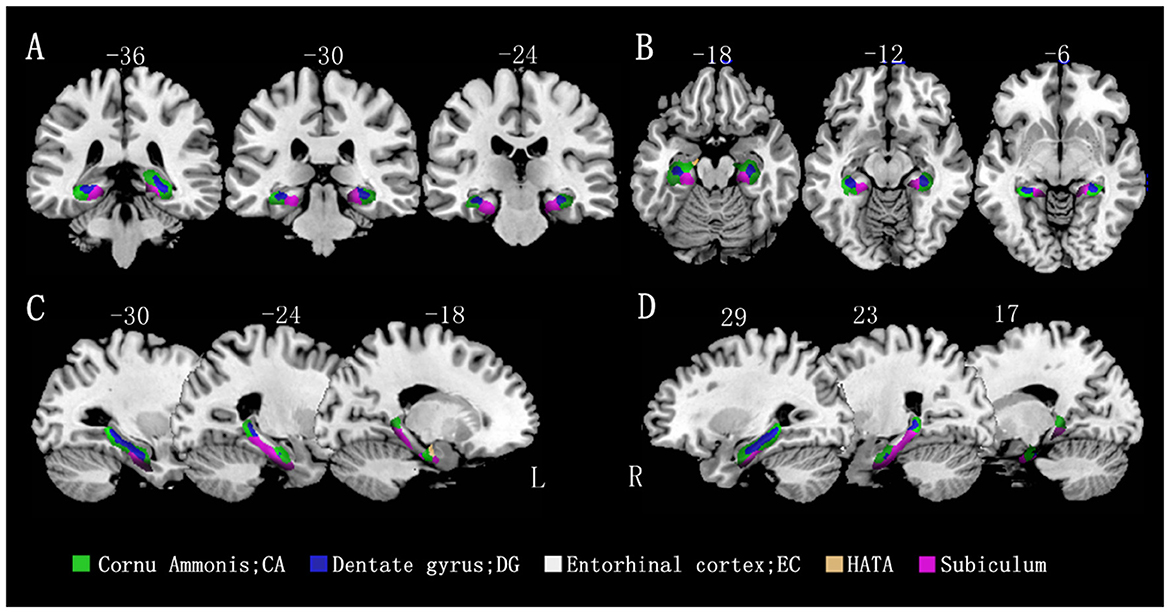
Figure 2. Differences in hippocampal subfield volumes between the two groups (n = 86). Different hippocampal subregions are represented by different colors. (A) Coronal view. (B) Transverse view. (C, D) Sagittal view. L, left; R, right.
3.4 Discriminative power of hippocampal subfield volumes
To evaluate the abillity of hippocampal subregions to distinguish between the two groups, ROC curves were analyzed separately for the left (A) and right (B) subregions (Figure 3). To differentiate between CN and MCI, all subfield volumes had similar AUCs (exceeding 0.556), but only the rCA and rDG subfields significantly distinguished between MCI and CN (P < 0.05) (Table 3).
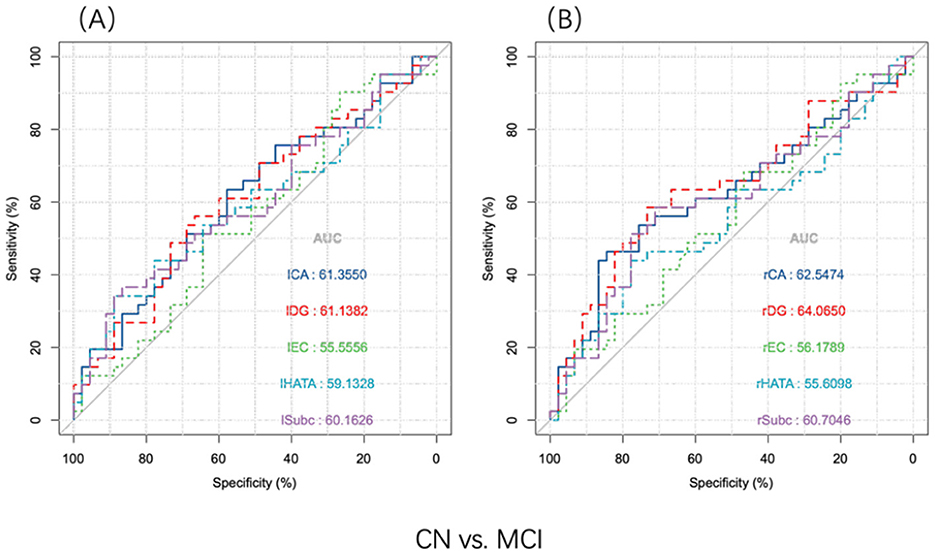
Figure 3. ROC analysis of the hippocampus subregion distinguished MCI and CN participants (n = 86). Discriminative power of hippocampal subfields volume. (A) Discernment of the volume of the left hippocampal subregion. (B) Discernment of the volume of the right hippocampal subregion. CA, cornu ammonis; DG, dentate gyrus; EC, entorhinal cortex; HATA, hippocampal-amygdaloid transition area; Subc, subiculum; l, left; r, right.
3.5 Correlation analysis between hippocampal subregion volume and blood biomarkers
Figure 4 illustrates the partial correlations between hippocampal subregion volumes and blood biomarkers, adjusted for age, sex, APOE ε4 carrier status, and TIV. The findings revealed that GFAP levels were negatively associated with the volumes of the rDG (r = −0.282, P = 0.015), rCA (r = −0.249, P = 0.033), lSubc (r = −0.248, P = 0.021), and lDG (r = −0.230, P = 0.040). NfL levels showed a negatively association with the volume of the lHATA (r = −0.223, P = 0.046). Additionally, p-Tau181 levels were inversely related to the volumes of the rDG (r = −0.265, P = 0.018), rCA (r = −0.246, P = 0.028), lSubc (r = −0.231, P = 0.036), and lDG (r = −0.223, P = 0.045). Aβ40 levels were inversely related to the volumes of the rEC (r = −0.332, P = 0.004), rSubc (r = −0.314, P = 0.005), and rCA (r = −0.253, P = 0.020). The Aβ42/Aβ40 ratio was positively associated with the volume of the lEC (r = 0.271, P = 0.026) and rEC (r = 0.282, P = 0.018). Furthermore, t-Tau levels were positively associated with the volumes of the lCA (r = 0.277, P = 0.014) and lDG (r = 0.253, P = 0.024). No correlation was observed between Aβ42 levels and the volumes of any hippocampal subregions.
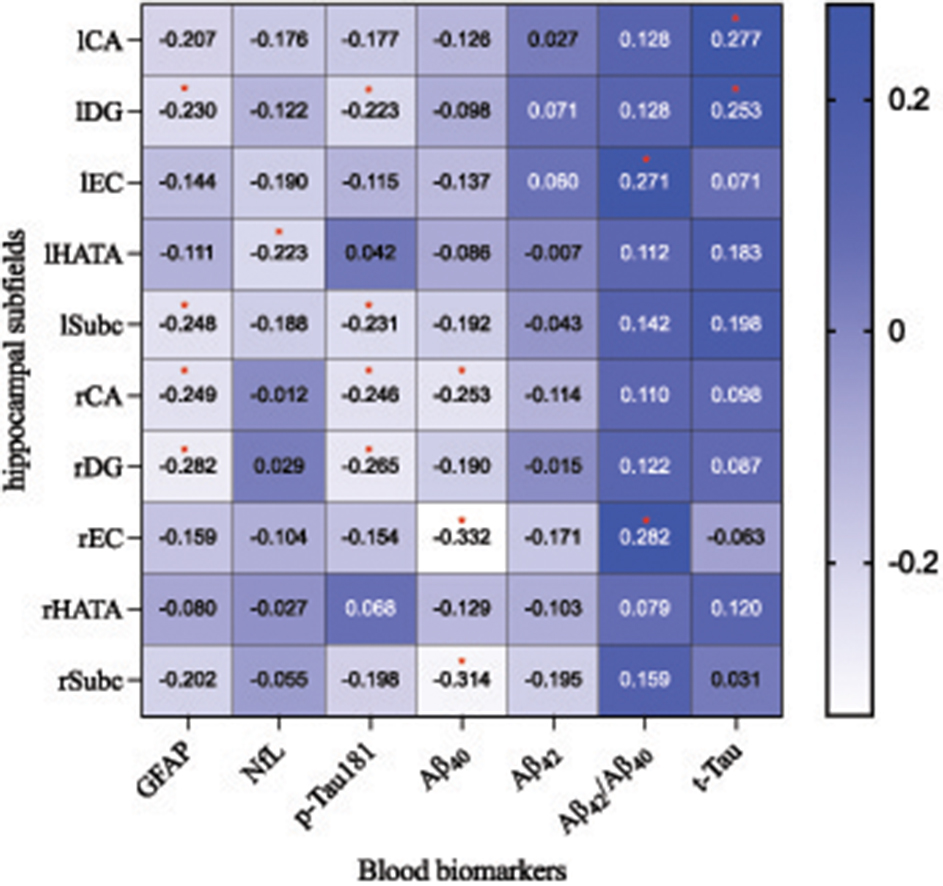
Figure 4. The partial correlation analysis illustrates the relationship between hippocampal subfield volumes and blood biomarker concentrations across all participants (n = 86). Here, blue indicates a positive correlation, with darker shades signifying stronger associations; conversely, white denotes a negative correlation, where a more intense hue corresponds to a more pronounced inverse relationship. *P < 0.05. CA, cornu ammonis; DG, dentate gyrus; EC, entorhinal cortex; HATA, hippocampal-amygdaloid transition area; Subc, subiculum; l, left; r, right. GFAP, glial fibrillary acidic protein; NfL, Neurofilament Light; t-Tau, total Tau; p-Tau, phosphorylated tau; Aβ, amyloid-β.
3.6 Receiver operating characteristic curves for the prediction of MCI vs. CN group
To evaluate the diagnostic efficacy of serum GFAP as potential biomarkers for differentiating MCI from CN individuals, logistic regression analyses were conducted with MCI or CN status as the dependent variable to compute predicted probability values, which were subsequently utilized to generate receiver operating characteristic (ROC) curves. A basic model (BM) was developed, incorporating established risk factors for AD, including age, gender, years of education, and the presence of the APOE ε4 allele; it yielded an AUC of 0.644 (CI 0.540–0.773), with a sensitivity of 31.1%, a specificity of 68.9% (P = 0.012). Upon integrating GFAP into the basic model, the AUC increased to 0.728 (CI 0.621–0.836), with a sensitivity of 65.9% and a specificity of 75.6% (P < 0.001). The DeLong's test indicated no significant difference between the two models (Z = −1.44, P = 0.149). See Figure 5 for details.
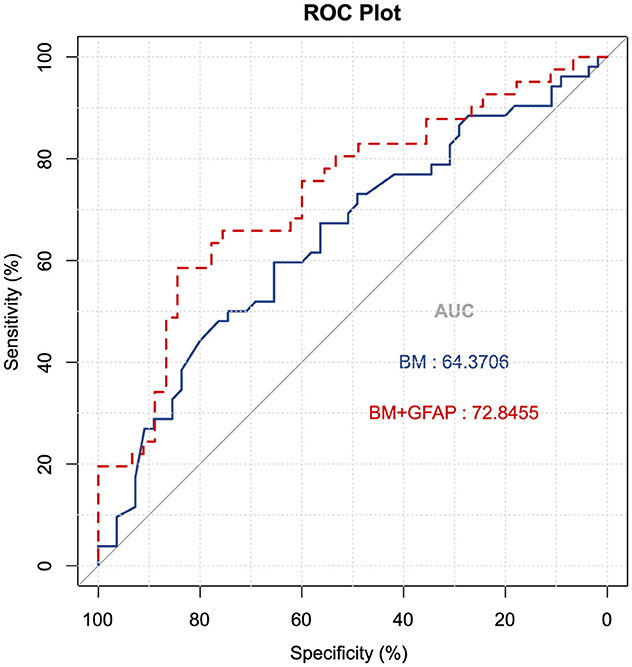
Figure 5. Receiver operating characteristic curves were utilized to predict MCI vs. CN (n = 107). The blue ROC curve represents the “base model” (BM), encompassing factors such as age, gender, education, and APOE ε4 allele status. The red ROC curve corresponds to the base model augmented with GFAP. AUC, area under the curve; GFAP, glial fibrillary acidic protein.
4 Discussion
This research demonstrates that the serum GFAP levels are higher in MCI patients from a community-based elderly cohort, suggesting that GFAP could potentially serve as a hematological marker for early detection of individuals predisposed to MCI prior to the onset of clinical symptoms. Despite the blood-brain barrier's role, which might distort the accuracy of blood biomarker levels as reflections of brain states, a meta-analysis by Gaur et al. (2023) supports these findings by indicating elevated GFAP in both cerebrospinal fluid and peripheral blood in MCI patients relative to a general cohort. Notably, the concentrations of GFAP in the subjects of this study were lower compared to the control populations in other studies. This variation may be attributed to our study's focus on community-dwelling elderly individuals, unlike other studies that primarily used samples from hospital settings or geriatric populations, where comorbidities might elevate GFAP levels. Additionally, elderly participants in such studies may present with multiple AD-related issues. Our participants, randomly selected seniors without self-reported symptoms, provide a baseline for possible widespread use of GFAP measurement. It is crucial to recognize that various factors, including demographics, behaviors, genetic predispositions, and others, could obscure the link between GFAP levels and MCI, with potential confounders impacting the results (Anderson, 2019; Gonzales et al., 2023). Variations in the testing methods also pose considerable influences. Furthermore, other biomarkers, such as Aβ40, Aβ42, NfL, t-Tau, and p-Tau181 were ineffective in distinguishing MCI patients from CN individuals. This ambiguity may arise from the MCI patients' relatively mild condition, resulting in lower biomarker levels in the blood. Additionally, the direct release of GFAP into the bloodstream from astrocytic end-feet at the neurovascular junction might mirror CNS alterations more closely than cerebrospinal fluid levels, underscoring the diagnostic utility of blood-based biomarkers (Pereira et al., 2021). In conclusion, increased GFAP levels in the blood suggest reactive astrocyte proliferation, indicative of early neurodegenerative processes. Therefore, GFAP holds promise as a biomarker to differentiate MCI from CN states.
The findings revealed that the MCI group exhibited a reduction in the volume of the bilateral DG, right CA, and left HATA when compared to the CN group. Furthermore, the volumes of the rCA and rDG subregions were effective in differentiating between individuals with MCI and those in the CN group. Multiple studies comparing MCI with hippocampal subregion volumes in a cognitively normal population are consistent with our results (Pluta et al., 2012; La Joie et al., 2013; Baek et al., 2022). Beak reported hippocampal CA4 and DG volume atrophy associated with increased Aβ deposition (Baek et al., 2022). Cognitive status was found to predict changes in hippocampal volumes, specifically in the CA1 and HATA regions (Becker et al., 2021). Functional magnetic resonance imaging (fMRI) studies conducted by Yassa detected hyperactive signals in the CA3 and DG regions of the hippocampus in aged rats, which were linked to deficits in spatial memory (Yassa et al., 2010). Such cognitive decline is typically reflected as atrophy in these hippocampal subregions, with the rCA and rDG displaying the strongest association coefficients. The recognized asymmetry in the bilateral hippocampal structure of mammals supports these observations (Hou et al., 2013). Disorientation a common symptom in AD and MCI, is associated with the volume of the right hippocampus (Peter et al., 2018). Studies in mice have found that signals from the CA3 of the right hemisphere connect to more robust synapses, playing a role in the stability of long-term memory maintenance (El-Gaby et al., 2015). The volumes of the right CA1 and DG in individuals with MCI are both smaller than those in healthy control groups (Qu et al., 2023). These studies provide compelling evidence for the significant atrophy in volume of the right DG and CA in MCI patients.
Additionally, our research revealed that elevated GFAP levels in the serum of patients with MCI were linked to diminished volumes in specific hippocampal subregions, including the bilateral DG, rCA, and lSubc compared to the control group. This correlation suggests that GFAP-associated pathologies may influence the atrophy of hippocampal subregions. Research by Cirarda corroborates our findings, demonstrating that GFAP levels correlate with volumetric changes in hippocampal subregions and are notably higher in the hippocampus of post-mortem patients (Díez-Cirarda et al., 2023). Neurodegenerative alterations in the CA1 region are linked to astrogliosis and reduced volume (Gonzalez-Rodriguez et al., 2021). However, the exact mechanisms behind the changes in hippocampal volume, whether due to the loss of neurons or glial cells, are still not fully understood. GFAP, a key protein in the astrocyte cytoskeleton, may have increased levels in the blood due to abnormal functional remodeling of astrocytes (Escartin et al., 2021). Microarray analysis also shows downregulation of the astrocytic cytoskeleton, indicating atrophy (Simpson et al., 2011). However, further research is needed to determine whether these changes indicate a neuroprotective response or whether they contribute to the exacerbation of neurodegeneration (Winblad et al., 2004). In summary, variations in the volumes of hippocampal subregions in MCI may reflect underlying changes in astrocytes, illuminating the complex relationship between astrocyte pathology and cognitive decline in MCI.
A ROC curve analysis was conducted to evaluate GFAP's ability to differentiate MCI from controls in this study. The combined diagnostic AUC for the baseline model and GFAP reached 0.728, demonstrating, a sensitivity of 65.9% and a specificity of 75.6%. Tao et al. (2024) reported a similar AUC of 0.714 for serum GFAP in distinguishing MCI from the control population, which aligns with our findings. Integrating GFAP with established AD risk factors significantly enhances the differentiation between MCI and control subjects, suggesting that GFAP levels are closely associated with age, sex, education, and APOE ε4 carrier status. However, predicting MCI based solely on serum GFAP is susceptible to various factors, necessitating the incorporation of multiple multidimensional factors for more accurate MCI prediction.
This study has several limitations. It was designed as a cross-sectional study, therefore, longitudinal tracking of patients could provide deeper insights into the pathophysiological changes associated with GFAP levels and cerebral alterations. Furthermore, we are unable to examine whether the associations of serum GFAP with demographic and clinical factors persist with adjustment for neuropathologic burden. Additionally, the small sample size may limit subgroup analyses, such as evaluating GFAP performance across different genders or lifestyles. Future research should involve larger cohorts to enhance the results' generalizability and applicability to a broader population.
In conclusion, this study demonstrates that GFAP is a promising biomarker for diagnosing MCI, exhibiting substantial sensitivity and specificity in distinguishing MCI from cognitively normal individuals in a community-based elderly cohort. MCI patients show reductions in hippocampal volume and elevated GFAP levels, potentially indicative of cognitive impairment. These findings may also guide future research toward identifying targets for new therapeutic interventions, including astrocyte-protective drugs, which could alleviate the adverse effects on hippocampal structures.
Data availability statement
The datasets presented in this study can be found in online repositories. The names of the repository/repositories and accession number(s) can be found in the article/Supplementary material.
Ethics statement
The studies involving humans were approved by Zhongshan Third People's Hospital Ethics Committee. The studies were conducted in accordance with the local legislation and institutional requirements. The participants provided their written informed consent to participate in this study. Written informed consent was obtained from the individual(s) for the publication of any potentially identifiable images or data included in this article.
Author contributions
YZha: Data curation, Formal analysis, Investigation, Methodology, Visualization, Writing – original draft, Writing – review & editing. JW: Conceptualization, Data curation, Formal analysis, Investigation, Writing – original draft. HZ: Data curation, Investigation, Methodology, Visualization, Writing – review & editing. ZT: Data curation, Formal analysis, Investigation, Writing – review & editing. YZhe: Data curation, Investigation, Software, Writing – review & editing. JP: Data curation, Investigation, Software, Writing – review & editing. JZ: Data curation, Investigation, Software, Writing – review & editing. JL: Data curation, Investigation, Software, Writing – review & editing. LLi: Investigation, Writing – review & editing. LLu: Data curation, Investigation, Writing – review & editing. XL: Funding acquisition, Investigation, Methodology, Project administration, Resources, Writing – review & editing.
Funding
The author(s) declare financial support was received for the research, authorship, and/or publication of this article. This study was funded by the Social Welfare Science and Technology Research Major Project in Zhongshan (grant number 2022B3017).
Acknowledgments
We thank the participants for their cooperation during this study.
Conflict of interest
The authors declare that the research was conducted in the absence of any commercial or financial relationships that could be construed as a potential conflict of interest.
Publisher's note
All claims expressed in this article are solely those of the authors and do not necessarily represent those of their affiliated organizations, or those of the publisher, the editors and the reviewers. Any product that may be evaluated in this article, or claim that may be made by its manufacturer, is not guaranteed or endorsed by the publisher.
Supplementary material
The Supplementary Material for this article can be found online at: https://www.frontiersin.org/articles/10.3389/fnagi.2024.1461556/full#supplementary-material
References
Abdelhak, A., Foschi, M., Abu-Rumeileh, S., Yue, J. K., D'Anna, L., Huss, A., et al. (2022). Blood GFAP as an emerging biomarker in brain and spinal cord disorders. Nat. Rev. Neurol. 18, 158–172. doi: 10.1038/s41582-021-00616-3
Albert, M. S., DeKosky, S. T., Dickson, D., Dubois, B., Feldman, H. H., Fox, N. C., et al. (2011). The diagnosis of mild cognitive impairment due to Alzheimer's disease: recommendations from the National Institute on Aging-Alzheimer's Association workgroups on diagnostic guidelines for Alzheimer's disease. Alzheimers Dement. 7, 270–279. doi: 10.1016/j.jalz.2011.03.008
Amunts, K., Kedo, O., Kindler, M., Pieperhoff, P., Mohlberg, H., Shah, N. J., et al. (2005). Cytoarchitectonic mapping of the human amygdala, hippocampal region and entorhinal cortex: intersubject variability and probability maps. Anat. Embryol. 210, 343–352. doi: 10.1007/s00429-005-0025-5
Anderson, N. D. (2019). State of the science on mild cognitive impairment (MCI). CNS Spectr. 24, 78–87. doi: 10.1017/S1092852918001347
Ashburner, J. (2007). A fast diffeomorphic image registration algorithm. Neuroimage 38, 95–113. doi: 10.1016/j.neuroimage.2007.07.007
Baek, M. S., Lee, N., Kim, J. W., and Hong, J. Y. (2022). Association of hippocampal subfield volumes with amyloid-beta deposition in Alzheimer's disease. J. Clin. Med. 11:1526. doi: 10.3390/jcm11061526
Becker, S., Granert, O., Timmers, M., Pilotto, A., Van Nueten, L., Roeben, B., et al. (2021). Association of hippocampal subfields, CSF biomarkers, and cognition in patients with Parkinson disease without dementia. Neurology 96, e904–e915. doi: 10.1212/WNL.0000000000011224
Benedet, A. L., Milà-Alomà, M., Vrillon, A., Ashton, N. J., Pascoal, T. A., Lussier, F., et al. (2021). Differences between plasma and cerebrospinal fluid glial fibrillary acidic protein levels across the Alzheimer disease continuum. JAMA Neurol. 78, 1471–1483. doi: 10.1001/jamaneurol.2021.3671
Blanken, A. E., Hurtz, S., Zarow, C., Biado, K., Honarpisheh, H., Somme, J., et al. (2017). Associations between hippocampal morphometry and neuropathologic markers of Alzheimer's disease using 7 T MRI. Neuroimage Clin. 15, 56–61. doi: 10.1016/j.nicl.2017.04.020
Bradfield, N. I. (2023). Mild cognitive impairment: diagnosis and subtypes. Clin. EEG Neurosci. 54, 4–11. doi: 10.1177/15500594211042708
Chatterjee, P., Pedrini, S., Doecke, J. D., Thota, R., Villemagne, V. L., Doré, V., et al. (2023). Plasma Aβ42/40 ratio, p-tau181, GFAP, and NfL across the Alzheimer's disease continuum: a cross-sectional and longitudinal study in the AIBL cohort. Alzheimers Dement. 19, 1117–1134. doi: 10.1002/alz.12724
Cicognola, C., Janelidze, S., Hertze, J., Zetterberg, H., Blennow, K., Mattsson-Carlgren, N., et al. (2021). Plasma glial fibrillary acidic protein detects Alzheimer pathology and predicts future conversion to Alzheimer dementia in patients with mild cognitive impairment. Alzheimers Res. Ther. 13:68. doi: 10.1186/s13195-021-00804-9
de Flores, R., La Joie, R., and Chételat, G. (2015). Structural imaging of hippocampal subfields in healthy aging and Alzheimer's disease. Neuroscience 309, 29–50. doi: 10.1016/j.neuroscience.2015.08.033
Díez-Cirarda, M., Yus-Fuertes, M., Sanchez-Sanchez, R., Gonzalez-Rosa, J. J., Gonzalez-Escamilla, G., Gil-Martínez, L., et al. (2023). Hippocampal subfield abnormalities and biomarkers of pathologic brain changes: from SARS-CoV-2 acute infection to post-COVID syndrome. EBioMedicine 94:104711. doi: 10.1016/j.ebiom.2023.104711
Ehrenberg, A. J., Khatun, A., Coomans, E., Betts, M. J., Capraro, F., Thijssen, E. H., et al. (2020). Relevance of biomarkers across different neurodegenerative diseases. Alzheimers Res. Ther. 12:56. doi: 10.1186/s13195-020-00637-y
El-Gaby, M., Shipton, O. A., and Paulsen, O. (2015). Synaptic plasticity and memory: new insights from hippocampal left-right asymmetries. Neuroscientist 21, 490–502. doi: 10.1177/1073858414550658
Escartin, C., Galea, E., Lakatos, A., O'Callaghan, J. P., Petzold, G. C., Serrano-Pozo, A., et al. (2021). Reactive astrocyte nomenclature, definitions, and future directions. Nat. Neurosci. 24, 312–325. doi: 10.1038/s41593-020-00783-4
Gaur, A., Rivet, L., Mah, E., Bawa, K. K., Gallagher, D., Herrmann, N., et al. (2023). Novel fluid biomarkers for mild cognitive impairment: a systematic review and meta-analysis. Ageing Res. Rev. 91:102046. doi: 10.1016/j.arr.2023.102046
Gonzales, M. M., Vela, G., Philip, V., Trevino, H., LaRoche, A., Wang, C.-P., et al. (2023). Demographic and clinical characteristics associated with serum GFAP levels in an ethnically diverse cohort. Neurology 101, e1531–e1541. doi: 10.1212/WNL.0000000000207706
Gonzalez-Rodriguez, M., Villar-Conde, S., Astillero-Lopez, V., Villanueva-Anguita, P., Ubeda-Banon, I., Flores-Cuadrado, A., et al. (2021). Neurodegeneration and astrogliosis in the human CA1 hippocampal subfield are related to hsp90ab1 and bag3 in Alzheimer's disease. Int. J. Mol. Sci. 23:165. doi: 10.3390/ijms23010165
Heimfarth, L., Passos, F. R. S., Monteiro, B. S., de Araújo, A. A., and Quintans Júnior, L. J. (2022). Serum glial fibrillary acidic protein is a body fluid biomarker: a valuable prognostic for neurological disease - A systematic review. Int. Immunopharmacol. 107:108624. doi: 10.1016/j.intimp.2022.108624
Heneka, M. T., Rodríguez, J. J., and Verkhratsky, A. (2010). Neuroglia in neurodegeneration. Brain Res. Rev. 63, 189–211. doi: 10.1016/j.brainresrev.2009.11.004
Hou, G., Yang, X., and Yuan, T.-F. (2013). Hippocampal asymmetry: differences in structures and functions. Neurochem. Res. 38, 453–460. doi: 10.1007/s11064-012-0954-3
Huang, H., Zheng, S., Yang, Z., Wu, Y., Li, Y., Qiu, J., et al. (2023). Voxel-based morphometry and a deep learning model for the diagnosis of early Alzheimer's disease based on cerebral gray matter changes. Cereb. Cortex 33, 754–763. doi: 10.1093/cercor/bhac099
Jia, X., Wang, Z., Huang, F., Su, C., Du, W., Jiang, H., et al. (2021). A comparison of the Mini-Mental State Examination (MMSE) with the Montreal Cognitive Assessment (MoCA) for mild cognitive impairment screening in Chinese middle-aged and older population: a cross-sectional study. BMC Psychiatry 21:485. doi: 10.1186/s12888-021-03495-6
Jung, J., Laverick, R., Nader, K., Brown, T., Morris, H., Wilson, M., et al. (2021). Altered hippocampal functional connectivity patterns in patients with cognitive impairments following ischaemic stroke: a resting-state fMRI study. NeuroImage Clin. 32:102742. doi: 10.1016/j.nicl.2021.102742
Kamphuis, W., Middeldorp, J., Kooijman, L., Sluijs, J. A., Kooi, E.-J., Moeton, M., et al. (2014). Glial fibrillary acidic protein isoform expression in plaque related astrogliosis in Alzheimer's disease. Neurobiol. Aging 35, 492–510. doi: 10.1016/j.neurobiolaging.2013.09.035
La Joie, R., Perrotin, A., de La Sayette, V., Egret, S., Doeuvre, L., Belliard, S., et al. (2013). Hippocampal subfield volumetry in mild cognitive impairment, Alzheimer's disease and semantic dementia. Neuroimage Clin. 3, 155–162. doi: 10.1016/j.nicl.2013.08.007
Li, D., Liu, X., Liu, T., Liu, H., Tong, L., Jia, S., et al. (2020). Neurochemical regulation of the expression and function of glial fibrillary acidic protein in astrocytes. Glia 68, 878–897. doi: 10.1002/glia.23734
Marizzoni, M., Chevalier, C., Paquis, A., Zetterberg, H., Blennow, K., Lathuiliere, A., et al. (2023). Association of AD risk factors with plasma GFAP levels in a memory clinic population. Alzheimers Dement. 19:e076079. doi: 10.1002/alz.076079
Osborn, L. M., Kamphuis, W., Wadman, W. J., and Hol, E. M. (2016). Astrogliosis: an integral player in the pathogenesis of Alzheimer's disease. Prog. Neurobiol. 144, 121–141. doi: 10.1016/j.pneurobio.2016.01.001
Pereira, J. B., Janelidze, S., Smith, R., Mattsson-Carlgren, N., Palmqvist, S., Teunissen, C. E., et al. (2021). Plasma GFAP is an early marker of amyloid-β but not tau pathology in Alzheimer's disease. Brain 144, 3505–3516. doi: 10.1093/brain/awab223
Peter, J., Sandkamp, R., Minkova, L., Schumacher, L. V., Kaller, C. P., Abdulkadir, A., et al. (2018). Real-world navigation in amnestic mild cognitive impairment: the relation to visuospatial memory and volume of hippocampal subregions. Neuropsychologia 109, 86–94. doi: 10.1016/j.neuropsychologia.2017.12.014
Pluta, J., Yushkevich, P., Das, S., and Wolk, D. (2012). In vivo analysis of hippocampal subfield atrophy in mild cognitive impairment via semi-automatic segmentation of T2-weighted MRI. J. Alzheimers Dis. 31, 85–99. doi: 10.3233/JAD-2012-111931
Qin, Y., Han, H., Li, Y., Cui, J., Jia, H., Ge, X., et al. (2023). Estimating bidirectional transitions and identifying predictors of mild cognitive impairment. Neurology 100, e297–e307. doi: 10.1212/WNL.0000000000201386
Qu, H., Ge, H., Wang, L., Wang, W., and Hu, C. (2023). Volume changes of hippocampal and amygdala subfields in patients with mild cognitive impairment and Alzheimer's disease. Acta Neurol. Belg. 123, 1381–1393. doi: 10.1007/s13760-023-02235-9
Ries, M., and Sastre, M. (2016). Mechanisms of Aβ clearance and degradation by glial cells. Front. Aging Neurosci. 8:160. doi: 10.3389/fnagi.2016.00160
Ritchie, K., Carrière, I., Howett, D., Su, L., Hornberger, M., O'Brien, J. T., et al. (2018). Allocentric and egocentric spatial processing in middle-aged adults at high risk of late-onset Alzheimer's disease: the PREVENT dementia study. J. Alzheimers Dis. 65, 885–896. doi: 10.3233/JAD-180432
Simpson, J. E., Ince, P. G., Shaw, P. J., Heath, P. R., Raman, R., Garwood, C. J., et al. (2011). Microarray analysis of the astrocyte transcriptome in the aging brain: relationship to Alzheimer's pathology and APOE genotype. Neurobiol. Aging 32, 1795–1807. doi: 10.1016/j.neurobiolaging.2011.04.013
Song, M., Wang, Y.-M., Wang, R., Xu, S.-J., Yu, L.-L., Wang, L., et al. (2021). Prevalence and risks of mild cognitive impairment of Chinese community-dwelling women aged above 60 years: a cross-sectional study. Arch. Womens Ment. Health 24, 903–911. doi: 10.1007/s00737-021-01137-0
Takahashi, Y., Takeuchi, H., Sakai, M., Yu, Z., Kikuchi, Y., Ito, F., et al. (2020). A single nucleotide polymorphism (-250 A/C) of the GFAP gene is associated with brain structures and cerebral blood flow. Psychiatry Clin. Neurosci. 74, 49–55. doi: 10.1111/pcn.12932
Tao, Q.-Q., Cai, X., Xue, Y.-Y., Ge, W., Yue, L., Li, X.-Y., et al. (2024). Alzheimer's disease early diagnostic and staging biomarkers revealed by large-scale cerebrospinal fluid and serum proteomic profiling. Innovation 5:100544. doi: 10.1016/j.xinn.2023.100544
Wade, D. T., and Collin, C. (1988). The Barthel ADL Index: a standard measure of physical disability? Int. Disabil. Stud. 10, 64–67. doi: 10.3109/09638288809164105
Winblad, B., Palmer, K., Kivipelto, M., Jelic, V., Fratiglioni, L., Wahlund, L.-O., et al. (2004). Mild cognitive impairment–beyond controversies, towards a consensus: report of the International Working Group on Mild Cognitive Impairment. J. Intern. Med. 256, 240–246. doi: 10.1111/j.1365-2796.2004.01380.x
Xiao, Y., Hu, Y., Huang, K., and Alzheimer's Disease Neuroimaging Initiative (2023). Atrophy of hippocampal subfields relates to memory decline during the pathological progression of Alzheimer's disease. Front. Aging Neurosci. 15:1287122. doi: 10.3389/fnagi.2023.1287122
Keywords: mild cognitive impairment, glial fibrillary acidic protein, hippocampus subfield, voxel-based morphometry, community
Citation: Zhang Y, Wang J, Zhang H, Tan Z, Zheng Y, Ping J, Zhang J, Luo J, Li L, Lu L and Liu X (2024) Elevated circulating levels of GFAP associated with reduced volumes in hippocampal subregions linked to mild cognitive impairment among community-dwelling elderly individuals. Front. Aging Neurosci. 16:1461556. doi: 10.3389/fnagi.2024.1461556
Received: 14 July 2024; Accepted: 14 October 2024;
Published: 29 October 2024.
Edited by:
Simone Varrasi, University of Catania, ItalyReviewed by:
Venkata Ajay Narendra Talabattula, Delaware State University, United StatesFernanda Hansen de Moraes, D'Or Institute for Research and Education (IDOR), Brazil
Qiang Qiang, Fudan University, China
Copyright © 2024 Zhang, Wang, Zhang, Tan, Zheng, Ping, Zhang, Luo, Li, Lu and Liu. This is an open-access article distributed under the terms of the Creative Commons Attribution License (CC BY). The use, distribution or reproduction in other forums is permitted, provided the original author(s) and the copyright owner(s) are credited and that the original publication in this journal is cited, in accordance with accepted academic practice. No use, distribution or reproduction is permitted which does not comply with these terms.
*Correspondence: Xinxia Liu, c3lsaXV4aW54aWFAMTYzLmNvbQ==
†These authors have contributed equally to this work and share first authorship