- 1Department of Neurology Nursing Unit Ward 17, The First Affiliated Hospital of Wenzhou Medical University, Wenzhou, Zhejiang, China
- 2Department of Neurology Nursing Unit Ward 361, The First Affiliated Hospital of Wenzhou Medical University, Wenzhou, Zhejiang, China
- 3Department of Neurology Nursing Unit Ward 362, The First Affiliated Hospital of Wenzhou Medical University, Wenzhou, Zhejiang, China
Objective: This study aimed to analyse the relevant factors that may affect post-stroke fatigue (PSF) in patients with stroke and further explore the correlation between family functioning and PSF.
Methods: Patients who had experienced a first episode of stroke and were admitted to the Department of Neurology of the First Affiliated Hospital of Wenzhou Medical University were rigorously screened. The general data and family functioning of the patients on admission were collected, and their family adaptation, partnership, growth, affection and resolve scores and their PSF on the 5th day of admission were collected using the fatigue severity scale (FSS). Multiple linear regression analysis was then utilized to explore the factors affecting PSF in patients with stroke.
Results: A total of 220 questionnaires were distributed, and 220 were returned, with 212 valid questionnaires and a valid return rate of 96.4%. These 212 patients had a family functioning score of 6.58 ± 2.00 and an FSS score of 36.62 ± 10.96. Spearman’s correlation analysis showed negative correlations between the FSS scores and the adaptation, partnership, growth, affection, resolve and family functioning scores (r = −0.380, −0.505, −0.470, −0.303, −0.281 and −0.712, respectively; p < 0.001). Furthermore, multiple linear regression analysis showed that family functioning (β′ = −0.516), marital status (β′ = −0.244), household income (β′ = −0.185), literacy (β′ = −0.181) and activities of daily living (β′ = −0.084) were influential factors for PSF in patients with stroke (p < 0.05).
Conclusion: There is a significant negative correlation between family functioning and PSF, suggesting that better family functioning may help mitigate the severity of post-stroke fatigue. Healthcare providers should identify interventions to help patients and families address fatigue, boost disease recovery, promote patients’ physical and mental health and improve their quality of life.
1 Introduction
Stroke is the second leading cause of death and is a major contributor to disability worldwide (Campbell and Khatri, 2020). Epidemiology studies have revealed a substantial increase in annual stroke incidence and deaths between 1990 and 2019 despite a significant decrease in age-standardized rates of stroke, particularly among those aged > 70 years (GBD 2019 Stroke Collaborators, 2021; Wu et al., 2019). Rehabilitation after stroke is a complex process that includes the recovery of physical function, improvement of cognitive function and psychosocial adaptation. However, post-stroke fatigue (PSF) has been shown in recent years to be a common and serious sequela affecting patients’ recovery and quality of life (Lanctôt et al., 2020; Vincent-Onabajo and Adamu, 2014). Fatigue, a subjective feeling, is often described as persistent physical and/or mental exhaustion that goes beyond the usual sense of tiredness. Post-stroke fatigue is characterized by persistent physical, cognitive and/or emotional fatigue, which severely affects the patient’s daily life and functioning. It is a symptom that frequently occurs in patients after the occurrence of stroke and causes them to have painful feelings, manifesting as both physical pain and emotional distress. The number of patients with stroke in China is increasing with the aging of the population, although, in recent years, the incidence of stroke in younger people has increased. This has led to the growing challenge of achieving an improved and faster recovery among patients after stroke. Family is of paramount importance for patients with stroke, as 80% of their care is provided by the family during the acute phase of their illness (World Health Organization, 2011). A family is viewed as a system with an efficacy that is reflected in the ability its members to interact, communicate emotionally with each other and respond together to stressful events (Juárez-Belaúnde et al., 2024). Family functioning refers to the interrelationships, communication and collaboration among family members and their ability to cope with stress and adversity. Family functioning may be subject to a variety of factors including, but not limited to, familial support, family dynamics and the mental health status of family members. Olson’s family systems theory (Juárez-Belaúnde et al., 2024) suggests that it is an essential function of the family to assist family members with chronic diseases to improve their health behaviors (Yahav, 2002; Franklin and Streeter, 1993). Favorable family functioning promotes the implementation of healthy behaviors in patients and enhances their ability to cope with the disease, which is of great significance for disease recovery (Krauth, 2017; Pesantes et al., 2018). These findings underscore the importance of family functioning in promoting effective coping mechanisms and enhancing recovery in patients with chronic diseases, including stroke (Dunbar et al., 2008; Trief et al., 2006). Although limited, there is emerging literature suggesting a potential correlation between family functioning and post-stroke fatigue (PSF). Several studies have demonstrated that family dynamics, such as communication, emotional support, and caregiving roles, play a significant role in the recovery process for stroke patients, which may indirectly influence PSF. For example, studies have shown that higher levels of family cohesion and support are associated with improved recovery outcomes and lower fatigue levels in stroke survivors (Dunbar et al., 2016; Indelicato et al., 2020). In the present study, we investigate the effect of family functioning on PSF in patients in the early stages of stroke by surveying the current status of PSF to provide theoretical support for clinical care.
2 Participants and methods
2.1 Participants
To ensure the study had sufficient power to detect the anticipated correlations between family functioning and PSF, the required sample size was calculated. Based on a hypothesized correlation coefficient (r) of 0.3, a power of 80% and a significance level (α) of 0.05, it was determined that 85 participants were necessary. Accounting for an anticipated dropout rate of 10%, this figure was adjusted to 95 participants to maintain statistical integrity throughout the study’s duration. The formula used to calculate the sample size for correlation studies, which factors in these parameters, was derived from Cohen’s statistical power analysis for the behavioral sciences (Chase and Chase, 1976).
Patients with a first episode of stroke admitted to the Department of Neurology of the First Affiliated Hospital of Wenzhou Medical University between October 2021 and July 2022 were rigorously screened using convenience sampling; this method was chosen because of time and resource constraints and in consideration of the fact that stroke is a specific disease. The inclusion criteria were as follows: patients whose clinical manifestations met the diagnostic criteria for stroke (Campbell et al., 2019) and whose stroke was confirmed through imaging (cranial computed tomography or cranial magnetic resonance imaging); those with a first episode of stroke and in a stable condition; those who were conscious and able to cooperate with the investigation; and those who gave informed consent and volunteered to participate in the study. The inclusion criteria were as follows: patients whose clinical manifestations met the diagnostic criteria for stroke (Campbell et al., 2019), confirmed through imaging (cranial computed tomography or cranial magnetic resonance imaging); those with a first episode of stroke and in stable condition; those who were conscious and able to cooperate with the investigation; and those who gave informed consent and volunteered to participate in the study. The exclusion criteria were as follows: patients with severe aphasia; those with severe cognitive impairment; those with malignant tumors; those with degenerative diseases of the central nervous system such as Parkinson’s disease; those with a previous history of mental illness; those with other recent major stressful life events such as divorce or the death of a loved one; and those with pre-stroke fatigue (PrSF). Patients with PrSF were excluded to isolate the stroke’s direct impact on fatigue levels. Pre-stroke fatigue was assessed using the fatigue severity scale (FSS), with a cut-off score set above the clinical threshold to identify significant fatigue. A total of 15 individuals meeting these criteria were excluded.
This study was conducted in accordance with the Declaration of Helsinki and approved by the Ethics Committee of the First Affiliated Hospital of Wenzhou Medical University (Approval number: 2022171). An informed consent form was signed by all participants in this study.
2.2 Methods
2.2.1 Survey tools
2.2.1.1 General patient data
All enrolled participants completed a general data questionnaire. The questionnaire was designed specifically to investigate the type of disease, gender, age, marital status, education level, household monthly income per capita (referred to as household income), medical payment methods, employment, living arrangement, activities of daily living (ADLs), number of comorbid chronic diseases, smoking history and alcohol history of the participants. The ADLs were measured using the modified Barthel index (MBI) (Wang et al., 2023), a tool most commonly used to assess the ability of patients with stroke to perform ADLs. The measurement consists of 10 items, namely feeding, bed-to-wheelchair transfer, personal hygiene, toileting, bathing, walking, walking up and down stairs, dressing and bowel control, with a total score of 100. The lower the MBI score is, the more severe the dysfunction and the greater the dependence. The degree of dependence was categorized into four grades, with ≤ 40 points categorized as severe dependence (all items required care from others – poor), 41–60 points categorized as moderate dependence (most items required care from others – fair) and 61–90 points and 90–100 points (Juárez-Belaúnde et al., 2024), categorized as mild or no dependence, respectively (few or no items required care from others – good). These categories were based on established scales, as reported (Duffy et al., 2013). Smoking was defined as an average of at least one cigarette per day for > 1 year; alcohol consumption was defined as an average of at least 50 ml of alcoholic beverages with a standard alcohol content (or other liquors of equivalent alcoholic content) per day for > 1 year.
2.2.1.2 Family adaptation, partnership, growth, affection and resolve score
The family adaptation, partnership, growth, affection and resolve (APGAR) score, designed by Smilkstein et al. (1982) in 1978 and based on family functioning characteristics, was applied to assess patients’ satisfaction with family functioning. It was developed to measure the five dimensions of adaptation, partnership, growth, affection and resolve in five separate questions. Each question was assigned a score of 0–2, with a total score of 0–10 points. The degree of family dysfunction was based on the total score, with 7–10 points defined as good family function, 4–6 points as moderate family dysfunction and 0–3 points as severe family dysfunction, with a Cronbach’s α coefficient of 0.94 (Liu et al., 2015). The family APGAR score delivers an assessment of familial support and functioning from a medical perspective and is now widely used in patients with cerebrovascular disease (Mei et al., 2023).
2.2.1.3 Post-stroke fatigue
Post-stroke fatigue was scored using the FSS (Lenaert et al., 2022), which is mainly designed to assess the severity of physical and mental fatigue felt by patients as a result of the disease. When assessing patients with stroke using the FSS, some scholars have diagnosed a score of ≥ 36 points as indicative of PSF (Wu and Wang, 2007). The FSS is comprised of a total of nine entries, each of which is scored on a 7-point Likert scale ranging from 1 to 7. A score of 1 indicates a ‘strongly disagree’ response to the corresponding entry, and a score of 7 represents a ‘strongly agree’ response to the corresponding entry. The final FSS score for the patient was obtained by summing the total score of the nine entries. The higher the score is, the more severe the patient’s fatigue (Zhang et al., 2021). The FSS score was highly reliable, with a Cronbach’s α coefficient of 0.90.
2.2.1.4 Sleep disturbances
The Athens Insomnia Scale (AIS) was used to measure the sleep quality of patients during the study period (Soldatos et al., 2000). The AIS is an internationally recognized self-assessment scale for sleep quality, consisting of eight items. The scale is a 4-point Likert scale, scored 0, 1, 2 or 3, with 0 representing ‘not at all’ and 3 representing a ‘severe’ impact. The total score is 24 points. Scores ranging from 0 to 4 indicate no sleep disturbances, 4–6 suggest possible insomnia and scores above 6 represent insomnia. The higher the score is, the poorer the sleep quality and the more severe the sleep problems. The Cronbach’s alpha coefficient for this scale is 0.875.
2.2.1.5 Anxiety and depression
The Hospital Anxiety and Depression Scale was developed to screen anxiety and depression in hospital patients (Cassiani-Miranda et al., 2022). It is composed of 14 items divided into two subscales: the depression subscale (7 items) and the anxiety subscale (7 items). Scores from 0 to 7 indicate no symptoms, 8 to 10 suggest possible symptoms and 11 to 21 indicate definite symptoms. The overall Cronbach’s alpha coefficient for the scale, as well as those of the anxiety and depression subscales, are 0.88 and 0.81, respectively.
2.2.2 Data collection
Patients were screened at the time of admission and assessed for family functioning after enrolment. A fatigue severity survey was conducted on the 5th day of enrolment, and all patients gave informed consent to be surveyed face-to-face by the investigator. In addition, the case data of patients suffering a first episode of stroke were retrieved with the cooperation of medical workers in the department. During the survey, the content of the questionnaire was first interpreted in detail, and then the requirements for completing the questionnaire were explained to ensure that the respondents understood it completely and could complete the questionnaire independently. Patients with dyslexia or those who were unable to complete the questionnaire by themselves due to hemiplegia completed the questionnaire one by one according to their circumstances after the investigator had read the questionnaire to them to make sure that they understood it completely. After the interviews, the questionnaires were checked and returned immediately to the investigator.
2.2.3 Quality control
The questionnaire survey was performed by two nurse practitioners and three charge nurses in the Department of Neurology who were uniformly trained and communicated using the same terminology. Before the survey, the investigator was required to explain the knowledge of stroke-related illnesses to facilitate favorable contact and communication with the patients. During the survey, the patients were selected in strict accordance with the inclusion and exclusion criteria. When communicating with the patients, unified guidance language was used to explain the items that patients did not understand objectively and accurately. Patients completed the questionnaires face-to-face with the investigator answering independently and without hints; the questionnaires were then checked and returned immediately. Questionnaires, if completed by the investigator instead of the patient, were verified with the patient after completion. At the entry stage, the questionnaires were rechecked and entered into the computer after ensuring that there were no errors, and qualifying questionnaires were numbered. A double-checking entry method was used to avoid human-generated data errors, and all data were entered into an electronic database and statistically analysed.
2.3 Statistical analysis
All valid raw data were numbered and managed using Excel software (Microsoft Corporation, Redmond, WA, USA). Count data, such as general clinical and sociological data and scores of each scale, were expressed as percentages and frequencies, and those conforming to normal distribution were expressed as mean ± standard deviation. For quantitative data with a non-normal distribution, the distribution was described using the median (first quartile, third quartile) (M[P25, P75]). The Kruskal–Wallis H test or Mann–Whitney U test was used to compare differences between groups. For multiple groups where the difference was statistically significant, the Mann–Whitney U test was used for pairwise comparisons between groups, and the Bonferroni method was used to correct the test results. Multiple linear regression analysis was utilized to explore the relationship between the variables of age, family income, marital status and literacy and PSF in patients who have experienced a first episode of stroke. Furthermore, Spearman’s correlation analysis was employed to analyse the potential correlation between family functioning and PSF in patients who have experienced a first episode of stroke. All data in this study were processed using the SPSS 25.0 data statistical software package (IBM Corporation, Armonk, NY, USA), with α = 0.05 as the test level.
3 Results
3.1 General data and disease-related characteristics of patients
A total of 220 questionnaires were distributed, and 220 were returned, with a recovery rate of 100.0%. Eight invalid questionnaires were excluded, and 212 valid questionnaires were included, with a valid recovery rate of 96.4%. See Figure 1 and Table 1 for details.
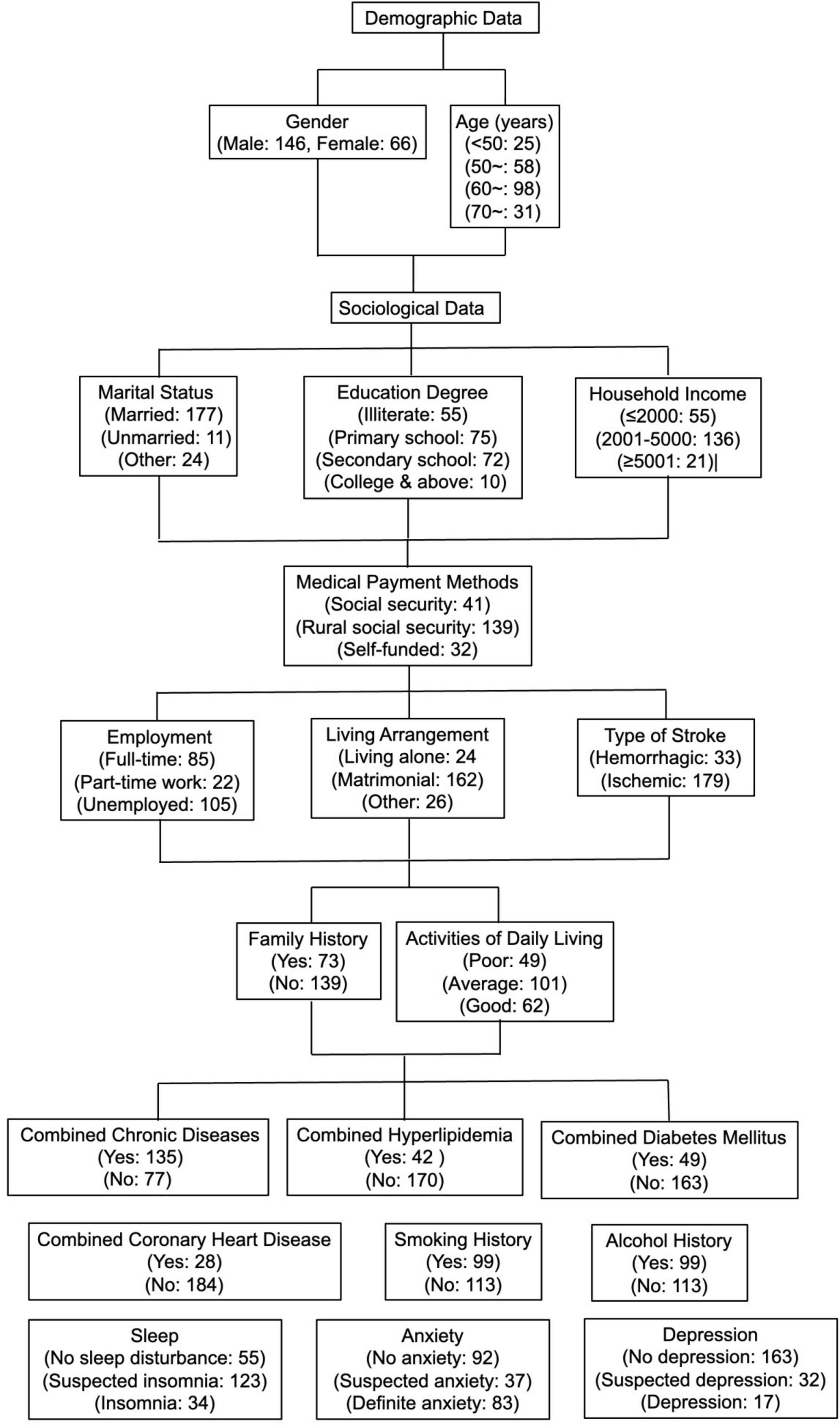
Figure 1. Flow chart of general demographic sociological and disease-related data of 212 first-episode stroke patients.
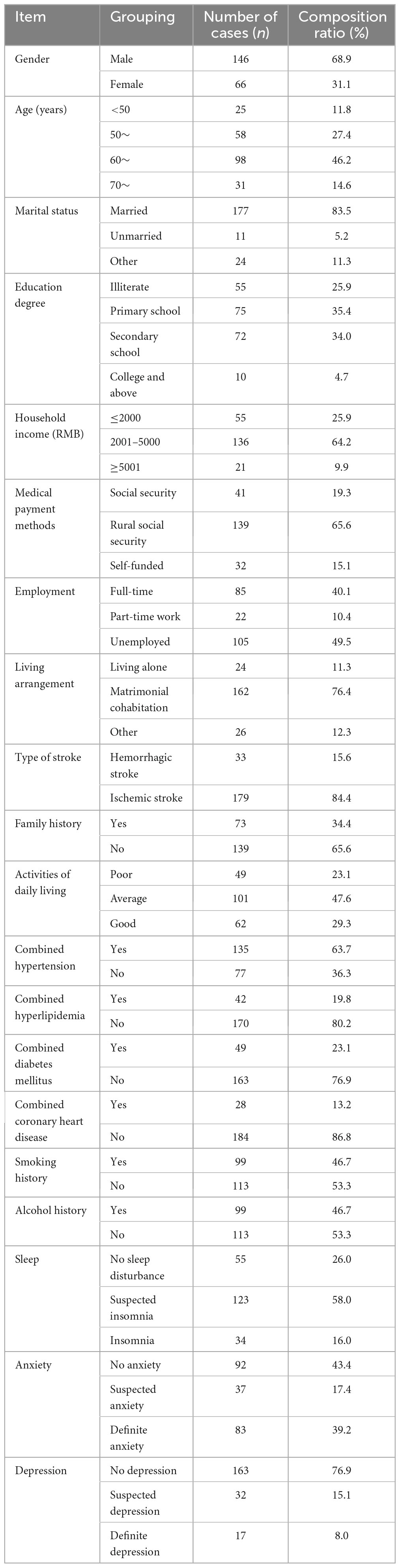
Table 1. General demographic sociological and disease-related data of 212 first-episode stroke patients.
3.2 Status and comparison of post-stroke fatigue in patients with stroke
3.2.1 Status of post-stroke fatigue in patients with stroke
The FSS scores of the 212 patients ranged from 14 to 60, with a mean of 36.62 ± 10.96. They were categorized into two groups: 102 (48.1%) patients had PSF (the ‘fatigue group’), and 110 (51.9%) patients had no fatigue (the “non-fatigue group”). In the fatigue group, there were 64 men and 38 women, with a mean age of 64.2 ± 8.3 years; in the non-fatigue group, there were 82 men and 28 women, with a mean age of 57.2 ± 8.9 years. No statistically significant association was found between the two groups in terms of gender (χ2 = 3.437, p = 0.063); however, there was a statistically significant difference in terms of age comparison (t = 5.937, p < 0.001, mean difference = 7.007).
3.2.2 Comparison of fatigue severity scale scores among patients with different clinical data
No statistically significant difference was found in the comparison of FSS scores for stroke type, family history, types of comorbid chronic diseases, smoking history and alcohol history (p > 0.05). However, there was a statistically significant difference in the comparison of FSS scores for gender, age, marital status, education level, household income, medical payment methods, employment, living arrangements, ADLs, sleep, anxiety and depression (p < 0.05, see Table 2).
3.3 Family functioning score of patients with stroke
The family APGAR scores of the 212 patients ranged from 2 to 10, with a mean of 6.58 ± 2.00. Each entry was scored as follows: adaptation 1.26 ± 0.75, partnership 1.27 ± 0.74, growth 1.43 ± 0.64, affection 1.45 ± 0.65 and resolve 1.18 ± 0.64. Table 3 provides the scores of the different functional groups.
3.4 Correlation analysis of family functioning and post-stroke fatigue
Spearman’s correlation analysis was used to conduct a two-by-two correlation analysis between the scores of each entry, the total family functioning score and the FSS score of the 212 patients with stroke. The data in Table 4 reveals that PSF in patients with stroke was negatively correlated with each entry and the total family functioning score.
3.5 Multiple linear regression analysis of the post-stroke fatigue status of patients with stroke
A total of 13 variables were included, with FSS scores as the dependent variable and variables with statistically significant differences (p < 0.05) or correlations in univariate analysis as the independent variables. Table 5 details the assignment of the variables. Multiple linear regression analysis revealed that marital status, literacy, household income, ADLs and the family functioning score were negatively correlated influences on PSF in patients with stroke (p < 0.05). Based on the standardized regression coefficients and their absolute values, the degree of influence of the independent variables on PSF in patients with stroke was determined, in which the factor with the greatest influence on PSF was family functioning (β′ = −0.516), followed by marital status (β′ = −0.244), household income (β′ = −0.185), literacy (β′ = −0.181) and ADLs (β′ = −0.084) (Table 6).
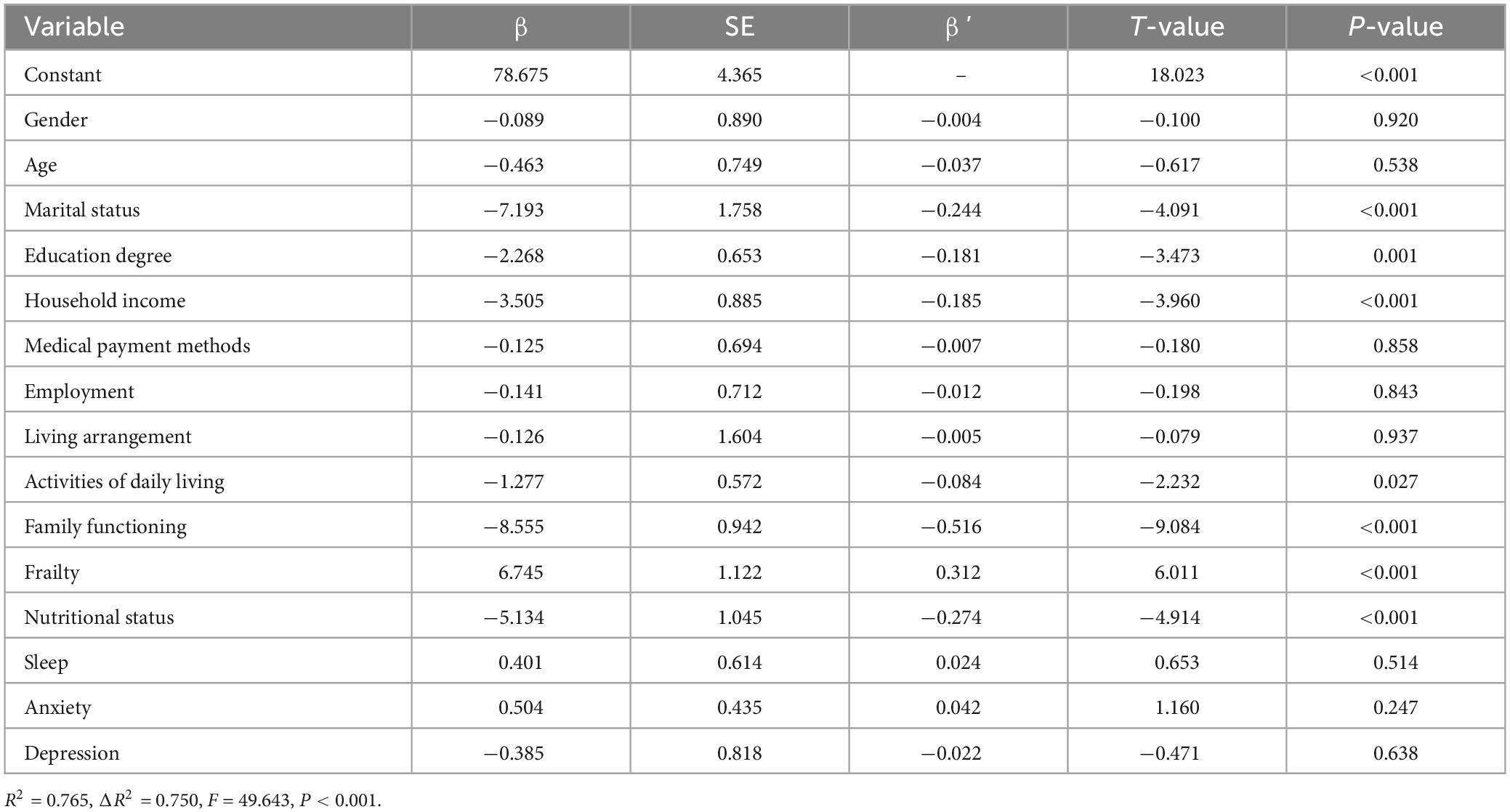
Table 6. Multiple linear regression analysis of factors influencing post-traumatic growth in stroke patients.
3.6 Influence of frailty on post-stroke fatigue
The mean FSS scores for patients who were non-frail, pre-frail and frail were 30.12 ± 8.45, 38.54 ± 9.32 and 45.67 ± 10.21, respectively. Multiple linear regression analysis indicated that frailty (β′ = 0.312, p < 0.01) was an independent predictor of PSF. Nutritional status was assessed using the Mini Nutritional Assessment (MNA). Patients were categorized into three groups: well-nourished (MNA ≥ 24), at risk of malnutrition (MNA 17–23.5) and malnourished (MNA < 17). The results revealed that nutritional status was significantly associated with fatigue severity. The mean FSS scores for patients who were well-nourished, at risk and malnourished were 32.78 ± 9.14, 40.56 ± 10.23 and 48.33 ± 11.45, respectively. Multiple linear regression analysis demonstrated that nutritional status (β′ = −0.274, p < 0.01) was an independent predictor of PSF (Table 7). The Clinical Frailty Scale (CFS) was used to assess the frailty of patients. The CFS scores ranged from 1 (very fit) to 9 (terminally ill). Patients were categorized into three groups: non-frail (CFS 1–3), pre-frail (CFS 4–5) and frail (CFS 6–9). The results revealed that frailty was significantly associated with higher FSS scores (Table 7).
4 Discussion
Our findings reveal significant differences in fatigue severity among patients with stroke based on gender, age, marital status, education level, income, employment status, living arrangements, ADLs, sleep quality, anxiety and depression. The absence of significant differences in fatigue severity among patients with stroke based on the type of stroke, family history and other comorbidities suggests that addressing fatigue requires a broader, more holistic approach rather than focusing solely on clinical factors. Tailoring interventions to accommodate these socioeconomic and psychological dimensions can enhance patient care, emphasizing the need for comprehensive strategies that incorporate mental health support, lifestyle adjustments and social assistance. This integrated approach could improve patient outcomes and quality of life in post-stroke recovery.
The findings of our study demonstrate a significant negative correlation between family functioning and PSF in patients who have experienced a first episode of stroke. This relationship highlights the potential of family dynamics as a modifiable factor in the management and recovery of patients with stroke. Our results are consistent with the hypothesis that improved family support systems can significantly reduce PSF severity, which aligns with recent studies emphasizing the role of social and familial support in post-stroke recovery processes (World Health Organization, 2011; Hagan et al., 2023). This emphasizes the need for healthcare strategies that incorporate family counseling and support mechanisms as integral components of stroke rehabilitation programmes.
This study demonstrates that the level of family functioning varies widely among patients with stroke, ranging from severe dysfunction to effective functioning, with many patients falling within the low-to-medium range. This variation in family functioning highlights the importance of considering family dynamics in post-stroke care and recovery strategies. Low family functioning, characterized by poor communication, lack of emotional exchange, and insufficient mutual support, was particularly notable in areas such as partnership and resolve. These deficiencies not only hinder the patient’s emotional recovery but also exacerbate the psychological burden caused by the disease, as patients face economic pressures and changes in their roles within the family, leading to irritability, depression, and negative emotions. To address these challenges, interventions that improve family functioning are critical for enhancing post-stroke recovery. Special focus should be placed on fostering better communication and emotional support among family members. Educating family caregivers about the importance of active involvement in the patient’s recovery—such as supervising medication, diet, and rehabilitation—can strengthen family partnerships and resolve. This approach will help to alleviate the patient’s psychological burden, reduce post-stroke fatigue, and improve overall recovery outcomes. By addressing the emotional and communicative deficiencies within the family, healthcare providers can foster a supportive environment that accelerates rehabilitation and enhances the quality of life for stroke survivors. Feigin et al. (2015) also investigated this aspect, suggesting that family dysfunction and vulnerability to conflict among family members are common phenomena for patients with stroke. In this survey, family dysfunction was noted, underlining the importance of interventions to improve family functioning in patients with stroke.
Post-stroke fatigue is common and easily overlooked in patients with stroke. It occurs in 30%–72% of these patients and may persist for a long period, affecting patients’ memory and cognitive functions. It further affects the rehabilitation and long-term prognosis of patients, increasing the recurrence and mortality rates of patients with stroke; it is one of the most intolerable sequelae among these patients (Choi-Kwon et al., 2017; Lagogianni et al., 2018; Mandliya et al., 2016). The current study reveals a relatively high incidence of PSF, suggesting that healthcare providers should monitor patients who have experienced a first episode of stroke and promptly assess the presence of PSF to minimize and, if possible, avoid PSF.
This study revealed a statistically significant difference in PSF scores between patients in the good and dysfunctional family functioning groups (all p < 0.001); adaptation, partnership, growth, affection and resolve were negatively correlated with PSF (p < 0.001). Further multiple linear regression analysis demonstrated that family functioning was one of the main factors affecting PSF, suggesting that a low level of family functioning is directly or indirectly responsible for the occurrence of PSF. Stroke takes a toll on patients’ physical and psychological well-being and their family health patterns and, consequently, negatively impacts coping and recovery from the disease. The family is the strongest support system for the patient in the aftermath of stroke and largely influences the patient’s psychology. Dunbar et al. (2016) found that family functioning affects patients’ emotional and physical states, and Indelicato et al. (2020) concluded that patients with diabetes and with less family closeness had correspondingly poorer glycaemic control. The family plays a decisive role in the physical and mental health and emotional and behavioral well-being of family members (Miller et al., 2000).
Our study’s finding that improved family functioning correlates negatively with PSF corroborates the results of similar studies in the field. For instance, a study by Yang et al. observed that patients with stroke who had strong familial support exhibited significantly lower levels of PSF compared with those with minimal family interaction (Yang et al., 2021). Additionally, Ablewhite et al. reported that active family involvement in everyday care activities was associated with a faster decline in PSF symptoms over 6 months (Ablewhite et al., 2022).
Furthermore, our results demonstrated that frailty significantly impacts PSF. Patients categorized as frail had higher FSS scores than patients who were non-frail or pre-frail. This finding underscores the importance of addressing frailty in patients with stroke, particularly among the elderly, as frailty can exacerbate the overall burden of the disease and hinder recovery. Integrating frailty assessments into the rehabilitation plan can help tailor interventions that specifically address the needs of patients who are frail. This aligns with the findings that frailty significantly influences cardiovascular disease outcomes, highlighting the critical role of caregivers and family support in managing such conditions (Liperoti et al., 2021). Furthermore, nutritional status was found to be a significant predictor of PSF. Patients who were malnourished had higher FSS scores than those who were well-nourished or at risk of malnutrition. Proper nutritional support is, therefore, vital in the management of PSF. Addressing malnutrition and ensuring adequate nutritional intake can significantly enhance recovery outcomes and reduce fatigue levels in patients with stroke. This is supported by studies emphasizing the importance of nutrition in post-stroke recovery and the role of malabsorption in exacerbating fatigue symptoms (Giovannini et al., 2024; Gimovsky and Berghella, 2022).
These consistent observations across studies highlight the critical role of family dynamics in the rehabilitation process of stroke survivors. Our study adds to this body of knowledge by quantifying the strength of the relationship and emphasizing specific aspects of family functioning, such as emotional support and communication, which were found to be particularly influential. This consistency in findings across different cohorts and geographical settings suggests a robust, generalisable link between family functioning and reduced PSF, underscoring the potential benefits of integrating family-centered approaches into post-stroke care protocols.
The clinical relevance of our study lies in demonstrating how improved family functioning significantly reduces PSF in patients who have experienced a first episode of stroke. This suggests that incorporating family dynamics into stroke rehabilitation could enhance patient outcomes. Clinicians should consider family assessments and interventions, such as family therapy and education, as part of comprehensive care plans. These approaches can empower families to support the patient’s recovery effectively, potentially accelerating rehabilitation and improving their quality of life. Emphasizing family involvement in care strategies not only addresses physical recovery but also bolsters the emotional support crucial for overcoming the challenges of post-stroke adjustments.
Nonetheless, there are some limitations to this study. First, only a cross-sectional survey was conducted in the acute phase after the patients’ first stroke and when their conditions were in a stable state. Due to limited time and insufficient funds, we did not conduct a long-term and in-depth follow-up of the study participants, which resulted in an inability to assess the dynamic changes of PSF in patients who have experienced a first episode of stroke. Second, a small sample was included in this study, with only 212 valid questionnaires. Despite being an acceptable sample size, small sample sizes may limit the accuracy and reliability of the results. This may have led to limitations in the quality of statistical analyses, making it unlikely that small effects could be detected or that more refined data analyses could be performed. Third, the samples included in this study were from a single center, which limits the representativeness of the sample and leads to unlikely generalization to other regions or different types of patient populations. As a result, the external validity of the results may have been affected. For a stronger analysis of the effect of family functioning on patients who have experienced a stroke and its correlation with PSF, in future studies, multiple time points could be used as the basis for investigations to compare and analyse the dynamic changes in family functioning and PSF over time. In addition, operational and scientifically feasible nursing interventions should be developed to improve the quality of life of patients who have survived a stroke.
This study has several limitations. First, the cross-sectional design of the study limits the ability to establish causality between family functioning and post-stroke fatigue (PSF). While our findings demonstrated a significant negative association between family functioning and PSF, the study did not specifically explore whether this association was direct or indirect. It is possible that other mediating factors, such as psychological health, social support, or the patient’s physical condition, could influence the relationship between family functioning and PSF. Future longitudinal studies are needed to clarify the causal pathways and determine whether improvements in family functioning directly reduce PSF or if other factors mediate this relationship. Additionally, the sample size was relatively small and limited to a single hospital, which may restrict the generalizability of the results. The study also focused on patients during the acute phase of stroke recovery, and long-term follow-up was not conducted to assess the dynamic changes in family functioning and PSF over time. Future research should aim to establish whether the relationship between family functioning and PSF is direct or mediated by other factors, such as psychological health, social support, or physical comorbidities. Longitudinal studies are needed to explore the dynamic changes in family functioning and PSF over time, particularly during the chronic phase of stroke recovery. Additionally, future studies could examine the effectiveness of specific family-based interventions—such as family therapy, caregiver training, and psychosocial support programs—in reducing PSF and improving patient outcomes. Expanding the study to include more diverse populations and settings could also help generalize the findings and identify culturally appropriate interventions. Finally, exploring the interaction between family functioning, frailty, and nutritional status may provide deeper insights into comprehensive care strategies for stroke survivors.
5 Conclusion
This study demonstrates a significant negative correlation between family functioning and post-stroke fatigue (PSF), indicating that patients with better family dynamics experience less severe PSF. Beyond the role of family functioning, factors such as marital status, household income, education level, and activities of daily living also contribute to the severity of PSF. These findings suggest that comprehensive rehabilitation strategies should incorporate family-centered approaches to improve not only physical recovery but also the emotional and psychological well-being of stroke survivors. By enhancing communication, emotional support, and practical assistance within the family unit, healthcare providers can help reduce the burden of PSF and promote better recovery outcomes. Furthermore, targeted interventions addressing socioeconomic and educational disparities may help mitigate the impact of PSF in certain populations
Data availability statement
The original contributions presented in the study are included in the article/supplementary material, further inquiries can be directed to the corresponding author.
Ethics statement
The studies involving humans were approved by the Ethics Committee of the First Affiliated Hospital of Wenzhou Medical University. The studies were conducted in accordance with the local legislation and institutional requirements. The participants provided their written informed consent to participate in this study.
Author contributions
RZ: Writing – review and editing, Writing – original draft, Supervision, Funding acquisition, Formal analysis, Conceptualization. HH: Writing – review and editing, Investigation, Data curation. YY: Writing – review and editing, Investigation, Data curation. SB: Writing – review and editing, Investigation, Data curation. NL: Writing – review and editing, Investigation, Data curation. MS: Writing – review and editing, Investigation, Formal analysis, Data curation.
Funding
The author(s) declare financial support was received for the research, authorship, and/or publication of the article. Wenzhou Science and Technology Bureau Wenzhou City Project (No. Y20220101).
Conflict of interest
The authors declare that the research was conducted in the absence of any commercial or financial relationships that could be construed as a potential conflict of interest.
Publisher’s note
All claims expressed in this article are solely those of the authors and do not necessarily represent those of their affiliated organizations, or those of the publisher, the editors and the reviewers. Any product that may be evaluated in this article, or claim that may be made by its manufacturer, is not guaranteed or endorsed by the publisher.
References
Ablewhite, J., Nouri, F., Whisker, A., Thomas, S., Jones, F., das Nair, R., et al. (2022). How do stroke survivors and their caregivers manage post-stroke fatigue? A qualitative study. Clin. Rehabil. 36, 1400–1410. doi: 10.1177/02692155221107738
Campbell, B., and Khatri, P. (2020). Stroke. Lancet 396, 129–142. doi: 10.1016/S0140-6736(20)31179-X
Campbell, B., De Silva, D., Macleod, M., Coutts, S., Schwamm, L., Davis, S., et al. (2019). Ischaemic stroke. Nat. Rev Dis. Prim. 5:70. doi: 10.1038/s41572-019-0118-
Cassiani-Miranda, C., Scoppetta, O., and Cabanzo-Arenas, D. (2022). Validity of the hospital anxiety and depression scale (HADS) in primary care patients in Colombia. Gen. Hosp. Psychiatry 74, 102–109. doi: 10.1016/j.genhosppsych.2021.01.014
Chase, L., and Chase, R. B. (1976). A statistical power analysis of applied psychological research. J. Appl. Psychol. 61, 234–237. doi: 10.1037/0021-9010.61.2.234
Choi-Kwon, S., Ko, M., Jun, S., Kim, J., Cho, K., Nah, H., et al. (2017). Post -stroke fatiguemay be associated with the promoter region of a monoamineoxidase a gene polymorphism. Cerebrovasc. Dis. 19, 54–58. doi: 10.1159/000450894
Duffy, L., Gajree, S., Langhorne, P., Stott, D., and Quinn, T. (2013). Reliability (inter-rater agreement) of the Barthel Index for assessment of stroke survivors: Systematic review and meta-analysis. Stroke 44, 462–468. doi: 10.1161/STROKEAHA.112.678615
Dunbar, S., Clark, P., Quinn, C., Gary, R., and Kaslow, N. (2008). Family influences on heart failure self-care and outcomes. J. Cardiovasc. Nurs. 23, 258–265. doi: 10.1097/01.JCN.0000305093.20012.b8
Dunbar, S., Clark, P., Stamp, K., Reilly, C., Gary, R., Higgins, M., et al. (2016). Family partnership and education interventions to reduce dietary sodium by patients with heart failure differ by family functioning. Heart Lung 45, 311–318. doi: 10.1016/j.hrtlng.2016.04.001
Feigin, V., Krishnamurthi, R., Parmar, P., Norrving, B., Mensah, G., Bennett, D., et al. (2015). Update on the global burden of ischemic and hemorrhagic stroke in 1990-2013: The GBD 2013 study. Neuroepidemiology 45, 161–176. doi: 10.1159/000441085
Franklin, C., and Streeter, C. (1993). Validity of the 3-D circumplex model for family assessment. Res. Soc Work Pract. 3, 258–275. doi: 10.1177/104973159300300302
GBD 2019 Stroke Collaborators (2021). Global, regional, and national burden of stroke and its risk factors, 1990-2019: A systematic analysis for the global burden of disease study 2019. Lancet Neurol. 20, 795–820. doi: 10.1016/S1474-4422(21)00252-0
Gimovsky, A., and Berghella, V. (2022). Evidence-based labor management: Second stage of labor (part 4). Am. J. Obstet. Gynecol. 4:100548. doi: 10.1016/j.ajogmf.2021.100548
Giovannini, S., Iacovelli, C., Loreti, C., Lama, E., Morciano, N., Frisullo, G., et al. (2024). The role of nutritional supplement on post-stroke fatigue: A pilot randomized controlled trial. J. Nutr. Health Aging 28:100256. doi: 10.1016/j.jnha.2024.100256
Hagan, J., Sipe, M., Tyer-Viola, L., Corless, I., Quinn, L., Hall, K., et al. (2023). Investigation of social support as a mediator of the relationship between physical and psychological health among hospitalised patients. J. Clin. Nurs. 32, 7812–7821. doi: 10.1111/jocn.16868
Indelicato, L., Calvo, V., Dauriz, M., Negri, A., Negri, C., Trombetta, M., et al. (2020). Depressive symptoms and glycaemic control in adults with type 1 diabetes: An exploratory study on the role of family functioning. Acta Diabetol. 57, 23–30. doi: 10.1007/s00592-019-01356-z
Juárez-Belaúnde, A., Soto-León, V., Dileone, M., Orcajo, E., León-Álvarez, N., Muñoz, A., et al. (2024). Early poststroke clinically significant fatigue predicts functional independence: A prospective longitudinal study. Front. Neurol. 15:1364446. doi: 10.3389/fneur.2024.1364446
Krauth, K. (2017). Family-oriented rehabilitation (FOR) and rehabilitation of adolescents and young adults (AYA) in pediatric oncology. Oncol. Res. Treat. 40, 752–758. doi: 10.1159/000484609
Lagogianni, C., Thomas, S., and Lincoln, N. (2018). Examining the relationship between fatigue and cognition after stroke: A systematic review. Neuropsychol. Rehabil. 28, 57–116. doi: 10.1080/09602011.2015.1127820
Lanctôt, K., Lindsay, M., Smith, E., Sahlas, D., Foley, N., Gubitz, G., et al. (2020). Canadian stroke best practice recommendations: Mood, cognition and fatigue following stroke, 6th edition update 2019. Int. J. Stroke 15, 668–688. doi: 10.1177/1747493019847334
Lenaert, B., van Kampen, N., van Heugten, C., and Ponds, R. (2022). Real-time measurement of post-stroke fatigue in daily life and its relationship with the retrospective Fatigue Severity Scale. Neuropsychol. Rehabil. 32, 992–1006. doi: 10.1080/09602011.2020.1854791
Liperoti, R., Vetrano, D., Palmer, K., Targowski, T., Cipriani, M., Lo Monaco, M., et al. (2021). Association between frailty and ischemic heart disease: A systematic review and meta-analysis. BMC Geriatr. 21:357. doi: 10.1186/s12877-021-02304-9
Liu, D., You, S., Li, S., Liu, G., and Zhang, D. (2015). Relationship between self-management behaviors and family functions among elderly patients with diabetes in community. Chongq. Med. 44, 893–895. doi: 10.3969/j.issn.1671-8348.2015.07.010
Mandliya, A., Das, A., Unnikrishnan, J., Amal, M., Sarma, P., and Sylaja, P. (2016). Post-stroke fatigue is an independent predictor of post-stroke disability and burden of care: A path analysis study. Top. Stroke Rehabil. 23, 1–7. doi: 10.1080/10749357.2015.1110273
Mei, Y., Xiang, D., Zhang, Z., Twumwaah Budu, J., Lin, B., and Chen, S. (2023). Family function, self-efficacy, care hours per day, closeness and benefit finding among stroke caregivers in China: A moderated mediation model. J. Clin. Nurs. 32, 506–516. doi: 10.1111/jocn.16290
Miller, I., Ryan, C., Keitner, G., Bishop, D., and Epstein, N. (2000). The McMaster approach to families: Theory, assessment, treatment and research. J. Fam. Ther. 22, 168–189. doi: 10.1111/1467-6427.00145
Pesantes, M., Del Valle, A., Diez-Canseco, F., Bernabé-Ortiz, A., Portocarrero, J., Trujillo, A., et al. (2018). Family support and diabetes: Patient’s experiences from a public hospital in Peru. Qual. Health Res. 28, 1871–1882. doi: 10.1177/1049732318784906
Smilkstein, G., Ashworth, C., and Montano, D. (1982). Validity and reliability of the family APGAR as a test of family function. J. Fam. Pract. 15, 303–311.
Soldatos, C., Dikeos, D., and Paparrigopoulos, T. (2000). Athens insomnia scale: Validation of an instrument based on ICD-10 criteria. J. Psychosom. Res. 48, 555–560. doi: 10.1016/s0022-3999(00)00095-7
Trief, P., Morin, P., Izquierdo, R., Teresi, J., Eimicke, J., Goland, R., et al. (2006). Depression and glycemic control in elderly ethnically diverse patients with diabetes: The IDEATel project. Diabetes Care 29, 830–835. doi: 10.2337/diacare.29.04.06.dc05-1769
Vincent-Onabajo, G., and Adamu, A. (2014). Impact of poststroke fatigue on health-related quality of life of Nigerian stroke survivors. J. Stroke 16, 195–201. doi: 10.5853/jos.2014.16.3.195
Wang, Y., Chang, P., Chen, Y., Lee, Y., Huang, S., Chen, M., et al. (2023). Comparison of responsiveness of the Barthel index and modified Barthel index in patients with stroke. Disabil. Rehabil. 45, 1097–1102. doi: 10.1080/09638288.2022.2055166
World Health Organization (2011). World report on disability chapter 5: Assistance and support. Geneva: World Health Organization.
Wu, C., and Wang, D. (2007). Clinical application and assessment of the Chinese version of Fatigue Severity Scale in stroke patients. Chin. J. Phys. Med. Rehabil. 29, 608–611. doi: 10.3760/j.issn:0254-1424.2007.09.009
Wu, S., Wu, B., Liu, M., Chen, Z., Wang, W., Anderson, C., et al. (2019). Stroke in China: Advances and challenges in epidemiology, prevention, and management. Lancet Neurol. 18, 394–405. doi: 10.1016/S1474-4422(18)30500-3
Yahav, R. (2002). External and internal symptoms in children and characteristics of the family system: A comparison of the linear and circumplex models. Am. J. Fam. Ther. 30, 39–56. doi: 10.1080/019261802753455633
Yang, L., Zhao, Q., Liu, H., Zhu, X., Wang, K., and Man, J. (2021). Family functioning mediates the relationship between activities of daily living and poststroke depression. Nurs. Res. 70, 51–57. doi: 10.1097/NNR.0000000000000472
Keywords: stroke, post-stroke fatigue, family functioning, clinical care, fatigue severity scale
Citation: Zhu R, Huang H, Yu Y, Bao S, Lin N and Shu M (2024) Post-stroke fatigue and its correlation with family functioning in patients who have experienced a first episode of stroke. Front. Aging Neurosci. 16:1440163. doi: 10.3389/fnagi.2024.1440163
Received: 29 May 2024; Accepted: 24 September 2024;
Published: 21 October 2024.
Edited by:
Silvia Giovannini, Catholic University of the Sacred Heart, ItalyReviewed by:
Claudia Loreti, Agostino Gemelli University Polyclinic (IRCCS), ItalyYang Zhang, Shandong University, China
Copyright © 2024 Zhu, Huang, Yu, Bao, Lin and Shu. This is an open-access article distributed under the terms of the Creative Commons Attribution License (CC BY). The use, distribution or reproduction in other forums is permitted, provided the original author(s) and the copyright owner(s) are credited and that the original publication in this journal is cited, in accordance with accepted academic practice. No use, distribution or reproduction is permitted which does not comply with these terms.
*Correspondence: Meichun Shu, shumeichun_mch@126.com