- 1Department of Gerontology and Geriatrics, The First Affiliated Hospital of China Medical University, Shenyang, Liaoning, China
- 2Department of Anesthesiology, The Fourth Affiliated Hospital of China Medical University, Shenyang, Liaoning, China
Background: The gut microbiota (GM) is hypothesized to play roles in Alzheimer’s disease (AD) pathogenesis. In recent years, many GM composition and abundance investigations in AD patients have been conducted; however, despite this work, some results remain controversial. Therefore, we conducted a systematic review and meta-analysis using 16S ribosomal RNA (16S rRNA) sequencing to explore GM alterations between patients with AD spectrum and healthy controls (HCs).
Methods: A systematic and comprehensive literature search of PubMed, Web of Science, Embase, the Cochrane Library, China National Knowledge Infrastructure, China Biology Medicine disc database, WanFang database and Social Sciences Citation Index databases was conducted from inception to January 2023. Inclusion and exclusion criteria were strictly defined, and two researchers independently screened and extracted information from selected studies. Data quality were evaluated according to the “Cochrane system evaluator manual” and pooled data were comprehensively analyzed using Stata 14 software with standardized mean differences (SMDs) and 95% confidence intervals (95% CIs) used to measure effect sizes. Also, geographical heterogeneity effects (related to cohorts) on GM abundance were examined based on subgroup meta-analyses if sufficient studies reported outcomes. Finally, publication bias was assessed using funnel plots.
Results: Out of 1566 articles, 13 studies involving 581 patients with AD spectrum and 445 HCs were deemed eligible and included in our analysis. In summary, a decreased microbiota alpha diversity and a significantly distinct pattern of clustering with regard to beta diversity were observed in AD spectrum patients when compared with HCs. Comparative analyses revealed a decreased Ruminococcus, Faecalibacterium, Lachnospira, Dialister, Lachnoclostridium, and Roseburia abundance in AD spectrum patients while Phascolarctobacterium, Lactobacillus, and Akkermansia muciniphila were more enriched in patients when compared to HCs. Furthermore, regional variations may have been in play for intestinal microbes such as Bacteroides, Bifidobacterium, and Alistipes.
Conclusion: Our meta-analysis identified alterations in GM abundance in patients with AD spectrum, with 12 genera from four major phyla significantly associated with AD. Moreover, we provided evidence for region-specific alterations in Bacteroides, Bifidobacterium, and Alistipes abundance. These findings may have profound implications for the development of innovative GM-based strategies to prevent and treat AD.
Systematic review registration: https://doi.org/10.37766/inplasy2024.6.0067, identifier INPLASY202460067.
Introduction
Alzheimer’s disease (AD) is a neurodegenerative disorder characterized by progressive cognitive and memory decline, making it the predominant dementia type. With global aging trends, the number of individuals affected by dementia is projected to reach 78 million by 2030 (Gauthier et al., 2021). In recent years, AD has emerged as a significant public health concern impacting overall wellbeing and quality of life metrics across global populations (Alzheimer’s, 2023). Therefore, it is crucial to diagnose, prevent, and treat AD during its early or even preclinical stages.
AD pathophysiological mechanisms and clinical manifestations suggest that the disease should be conceptualized as a continuum or spectrum, encompassing three distinct phases: preclinical AD (including subjective cognitive decline, SCD), mild cognitive impairment (MCI), and Dementia of Alzheimer’s type, also known as AD (Fernandes et al., 2020; Alzheimer’s, 2023). The characteristic histopathological hallmark of AD is the extraneuronal aggregation of amyloid-β (Aβ) peptide fragments into plaques, along with intraneuronal abnormal tau protein accumulation, which both serve as AD biomarkers (Long and Holtzman, 2019). Although no cure exists for AD, recent evidence has highlighted the potential role of the gut microbiota (GM) in disease development or exacerbation (Bonfili et al., 2018; Kim et al., 2020). Furthermore, several studies have reported promising results in terms of enhancing cognition via non-pharmacological interventions such as fecal microbial transplantation (Sun et al., 2019) and probiotics at early disease stages (Hwang et al., 2019).
The GM is a complex and abundant microbial community in the human gastrointestinal tract, and has important physiological roles regulating immune function, promoting food catabolism and metabolism, secreting metabolites, and limiting pathogen abundance (Rolhion and Chassaing, 2016). Numerous studies have confirmed the significant involvement of the GM in the occurrence and development of central nervous system diseases, such as anxiety disorders (Cox and Weiner, 2018), autism (Finegold et al., 2010), depression (Valles-Colomer et al., 2019), and Parkinson’s disease (PD) (Scheperjans et al., 2015). Recently, several investigations reported a correlation between intestinal flora and AD, suggesting altered GM composition and abundance among AD patients. Evidence has also indicated that neuroinflammation plays fundamental roles in AD onset and progression, while activation of the human innate immune system by gut microorganisms may drive brain inflammation (Cheng et al., 2019; Kowalski and Mulak, 2019; Sochocka et al., 2019). Gut microorganisms secrete increased amyloid protein and lipopolysaccharide (LPS) that modulate signaling pathways to potentially increase amyloid aggregation, and lead to inflammation via inflammatory cytokine production and immunogenic changes within the brain (Hill and Lukiw, 2015; Taylor and Matthews, 2015; Sochocka et al., 2019). Emerging studies indicate that metabolites generated by GM play a role in regulating the differentiation, maturation, and activation of reactive astrogliosis and microgliosis, which provide support and protection to neurons, clearing dead cells and foreign particles to maintain homeostasis in the brain. However, intestinal dysbiosis abnormally activates glial cells, potentially propagating Aβ toxicity, increasing Aβ accumulation, or releasing proinflammatory cytokines and reactive oxygen species, all of which are harmful to neurons, facilitate tau pathology, and further exacerbate inflammation, leading to neuronal damage and the progression of AD pathology (Yang and David, 2018; Ashley et al., 2022).
Recently, GM exploration as a biomarker and potential target to prevent and treat AD has gained popularity due to simple specimen acquisition, easy detection, and targeted regulation. In bacteria, 16S ribosomal RNA (16S rRNA) corresponds to the DNA sequence encoding rRNA, which is universally present in bacterial genomes (Gao et al., 2021). 16S rRNA-based sequencing is a rapid, cost-effective, and minimal labor-intensive approach for microbial detection; it allows for the comprehensive analysis of whole microbial composition and significantly enhances bacterial identification and resolution (Forde and O’Toole, 2013). Thus, in recent years, 16S rRNA sequencing techniques have emerged as predominant methods for investigating microbial ecosystem composition. To date, several clinical studies (Ling et al., 2020; Guo et al., 2021; Liu et al., 2021; Kaiyrlykyzy et al., 2022; Ferreiro et al., 2023) have used gut flora 16S rRNA high-throughput sequencing to analyze GM relative abundance in patients with AD. However, due to variations in bacterial detection techniques and inconsistent results from 16S rRNA sequencing, accurate and specific comparisons across studies are often limited. Several observational studies have found inconsistencies in the composition and diversity of GM associated with AD. For instance, Guo et al. (2021) identified genera such as Prevotella, Bacteroides, and Lachnospira as differing between AD patients and HCs, while Liu et al. (2021) found differences in genera including Blautia, Bacteroides, and Ruminococcus. Meantime, Guo et al. (2021) reported a higher relative abundance of Bacteroides in AD patients, whereas (Liu et al., 2019) arrived at the opposite conclusion. Additionally, Sheng et al. (2021) observed a trend toward a gradual decrease in GM abundance and diversity from individuals with SCD to MCI. However, a meta-analysis including AD and MCI patients indicated a slight increase in GM diversity and abundance among MCI patients. In this study, we conducted a systematic review and meta-analysis to investigate altered GM abundance in AD spectrum patients (i.e., SCD, MCI, and AD) based on 16S rRNA sequencing.
Materials and methods
The systematic review was performed according to the PRISMA guidelines (Preferred Reporting Items for Systematic Reviews and Meta-Analyses), and the protocol was registered at INPLASY (International Platform for Registered Protocols for Systematic Reviews and Meta-Analyses) under registration number INPLASY202460067.1
Search strategy
To identify information/data related to intestinal microbial profiles associated with AD, an extensive search was conducted across different medical databases, including PubMed, Web of Science, Embase, the Cochrane Library, China National Knowledge Infrastructure, China Biology Medicine disc database, WanFang database and Social Sciences Citation Index databases from inception to January 2023. The completed search strategy for Embase was: (“rna, ribosomal, 16s”/exp OR “16s rrna”:ab,ti OR “rrna 16s”:ab,ti OR “16s ribosomal rna”:ab,ti OR “rna, 16s ribosomal”:ab,ti OR “ribosomal rna, 16s”:ab,ti OR “16s ribosome rna”:ab,ti OR “16s rdna”:ab,ti OR “rdna 16s”:ab,ti OR “16s ribosomal dna”:ab,ti OR “dna, 16s ribosomal”:ab,ti OR “ribosomal dna, 16s”:ab,ti OR “16s ribosome dna”:ab,ti OR “16s rrna gene”:ab,ti) AND (“alzheimer disease”/exp OR “dementia”/exp OR alzheimer:ab,ti OR “alzheimers disease”:ab,ti OR dementia:ab,ti OR cognitive:ab,ti OR cogntion:ab,ti OR amentia: ab,ti OR mci:ab,ti OR “mild cognitive impairment”:ab,ti OR “mild cognitive defect”:ab,ti OR scd:ab,ti OR “subjective cognitive decline”:ab,ti OR “subjective cognitive impairment”:ab,ti). Furthermore, we also reviewed cited references of the retrieved articles to identify additional published and unpublished studies.
Inclusion and exclusion criteria
Inclusion criteria were as follows: (1) AD spectrum patients diagnosed using validated criteria (Diagnostic and Statistical Manual of Mental Disorders or National Institute on Aging and Alzheimer’s Association guidelines) (First et al., 2002; Hyman et al., 2012); (2) GM comparisons conducted between AD spectrum and HCs using 16S rRNA sequencing; (3) GM samples came from stool samples; (4) Accessible raw data were, such as relative GM abundance at distinct levels, microbial composition, community structures, and diversity indices; (5) Studies including randomized controlled trials (RCTs), cohort studies, and case-control studies; and (6) Studies not limited by language type (i.e., English, Chinese).
Exclusion criteria were as follows: (1) Studies without HCs; (2) Microbial analyses using other microbial detection methods; (3) Ambiguous literature and corresponding data and results that could not be extracted; (4) Studies with insufficient or overlapping data (the most recent and complete data were chosen); and (5) Systematic reviews, animal studies, conference abstracts, case reports, and commentaries.
Study selection and data extraction
Using our retrieval strategy, two independent investigators extracted data and discussed any post-extraction discrepancies. Disagreements were resolved by discussion with a third reviewer. If necessary, the corresponding authors of selected studies were contacted (e-mail) to request any missing data. Data collected from selected studies included: general information (first author and publication year, study design, and region), participant characteristics (sample size, age, and sex ratio), adjusted confounding factors, and information pertaining to GM alterations (relative GM abundance by taxonomic, microbial composition, community structure levels, and diversity index).
Risk of bias and quality assessment
Based on extracted data, the Cochrane Risk of Bias Assessment Tool (Higgins et al., 2011) was used for RCTs which had five domains: randomization, deviation from intervention, missing data, outcome measurements, and selective reporting. The Newcastle–Ottawa scale (Wells et al., 2000) was used for observational/non-randomized studies with domain selection, comparability, and exposure/outcomes.
Statistical analysis
This meta-analysis was conducted using Stata 14.0 software. Standardized mean differences (SMD) and 95% confidence intervals (CI) came from fixed effects or random effects models for quantitative synthesis. Heterogeneity was evaluated using the Cochran Q statistic and quantified by I2 tests, with an I2 > 50% indicating moderate-to-high heterogeneity (Higgins et al., 2003). Subgroup analysis was performed to investigate sources of heterogeneity, while sensitivity analyses conducted if necessary. Publication bias was visually assessed using funnel plots (Sterne et al., 2011).
Results
Literature searches and study characteristics
The initial search yielded 1,566 articles. After excluding 681 duplicates, two independent reviewers assessed titles and abstracts to exclude 860 irrelevant articles. Subsequently, full-text reviews were conducted on 25 articles, and finally 13 studies were included in the meta-analysis (Figure 1). Studies comprised 10 case-control (Vogt et al., 2017; Zhuang et al., 2018; Li et al., 2019; Liu et al., 2019, 2021; Ling et al., 2020; Guo et al., 2021; Sheng et al., 2021; Zhou et al., 2021; Khedr et al., 2022) and two longitudinal studies (Haran et al., 2019; Zhang et al., 2021), and also one RCT study (Nagpal et al., 2019). The geographical location of studies included China (Zhuang et al., 2018; Li et al., 2019; Liu et al., 2019, 2021; Ling et al., 2020; Guo et al., 2021; Sheng et al., 2021; Zhang et al., 2021; Zhou et al., 2021), USA (Vogt et al., 2017; Haran et al., 2019; Nagpal et al., 2019), and Egypt (Khedr et al., 2022). All studies used 16SrRNA sequencing to evaluate GM samples.
Our analysis comprised 1026 subjects (445 HCs and 581 AD spectrum patients), with a majority of female subjects (59.9%) and a mean age > 65 years. Most studies adjusted for important confounders, including age, sex, education, and body mass index (BMI). The most frequently utilized estimator for alpha diversity was the Shannon index, followed by the Simpson index and Chao1 index, although three studies did not provide information on which index was employed. 12 studies reported beta diversity indices mainly include the Bray-Curtis, weighted and unweighted UniFrac. GM alterations primarily focused on dominant phyla and also different specific bacterial genera or subspecies, such as A. muciniphila, Faecalibacterium, Bifidobacterium, Bacteroides, and Alistipes (Table 1).
Microbiota diversity in patients with AD spectrum
The richness, evenness, and alpha diversity can all be used to express the diversity of species. Of the 13 included studies, ten studies investigated the bacterial diversity in AD spectrum patients versus HCs, although different indexes were utilized. At the individual study level, results on the difference in alpha diversity between AD spectrum patients and HCs were discrepant: the Shannon index was observed to be significantly lower in patients with AD spectrum compared to HCs in five studies (Vogt et al., 2017; Liu et al., 2019; Ling et al., 2020; Sheng et al., 2021; Zhang et al., 2021), and showed not significant difference in the remaining five studies (Zhuang et al., 2018; Li et al., 2019; Nagpal et al., 2019; Guo et al., 2021; Zhou et al., 2021). Sheng et al. (2021) found on statistically significant reduction in alpha diversity indices for SCD individuals compared to HCs and significantly decreased alpha diversity among CI individuals. Li et al. (2019) found significantly lower diversity (Shannon index) among AD group compared to those of the controls only in blood microbiota samples, but no significant differences among stool microbiota samples. The Simpson index, reported in six studies, was found to be significantly lower in AD spectrum patients compared HCs in four studies (Liu et al., 2019; Ling et al., 2020; Sheng et al., 2021; Zhang et al., 2021) and to be non-significantly different in two studies (Liu et al., 2021; Zhou et al., 2021). Another seven studies used the Chao1 between samples to assess richness and it was found to be significantly lower in AD spectrum patients than HCs in three studies (Liu et al., 2019; Ling et al., 2020; Sheng et al., 2021) and non-significant in four studies (Li et al., 2019; Nagpal et al., 2019; Liu et al., 2021; Zhou et al., 2021).
Beta diversity represents the dissimilarity between the two gut communities. A total of 11 studies (Vogt et al., 2017; Zhuang et al., 2018; Haran et al., 2019; Li et al., 2019; Liu et al., 2019; Nagpal et al., 2019; Ling et al., 2020; Guo et al., 2021; Sheng et al., 2021; Zhang et al., 2021; Zhou et al., 2021) in this review have analyzed the beta diversity of GM, and nine of them consistently identified that the GM of AD spectrum patients showed a remarkably distinct clustering pattern compared with that of HCs (Vogt et al., 2017; Zhuang et al., 2018; Haran et al., 2019; Li et al., 2019; Liu et al., 2019; Ling et al., 2020; Guo et al., 2021; Zhang et al., 2021; Zhou et al., 2021).
The relative abundance of bacterial genera at different phyla levels
In terms of relative abundance, Firmicutes and Bacteroidetes represented the dominant phyla, accounting for 90% of all the GM. Firmicutes consists of > 200 different genera comprising Gram-positive aerobic and anaerobic bacteria (Rinninella et al., 2019). Among selected studies, statistical analyses were conducted on 10 distinct genera in Firmicutes. We observed that Ruminococcus, Phascolarctobacterium, Faecalibacterium, Lachnospira, Dialister, Lactobacillus, Lachnoclostridium and Roseburia exhibited distinct intestinal bacterial profiles between AD spectrum patients and HCs. Specifically in AD spectrum patients, a decrease in the relative abundance of Ruminococcus (SMD = −0.48, 95% CI: −0.75 to −0.22; I2for heterogeneity = 0%) (Figure 2A), Faecalibacterium (SMD = −0.62, 95% CI: −0.82 to −0.43; I2for heterogeneity = 21.7%) (Figure 2B), Lachnospira (SMD = −0.42, 95% CI: −0.71 to −0.13; I2for heterogeneity = 25.1%) (Figure 2C), Dialister (SMD = −2.32, 95% CI: −2.66 to −1.97; I2for heterogeneity = 35.0%) (Figure 2D), Lachnoclostridium (SMD = −0.36, 95% CI: −0.68 to −0.04; I2for heterogeneity = 10.2%) (Figure 2E), and Roseburia (SMD = −0.71, 95% CI: −1.28 to −0.15; I2for heterogeneity = 64.0%) (Figure 2F) was observed.
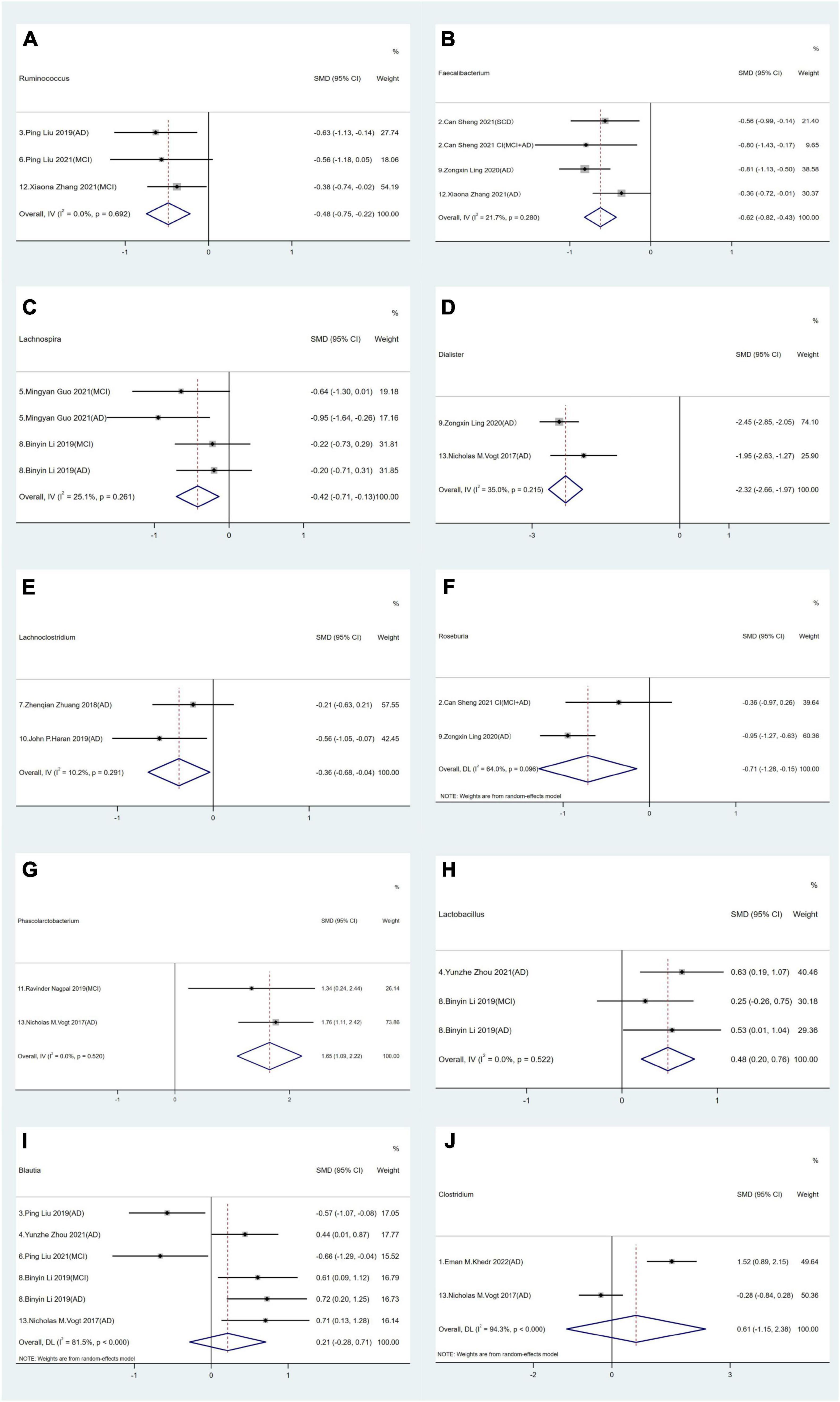
Figure 2. Forest plots of alterations of gut microbiota in the Firmicutes phylum compare AD spectrum patients to healthy controls (HCs): (A) Ruminococcus, (B) Faecalibacterium, (C) Lachnospira, (D) Dialister, (E) Lachnoclostridium, (F) Roseburia, (G) Phascolarctobacterium, (H) Lactobacillus, (I) Blautia, (J) Clostridium.
Conversely, an increase in the relative abundance of Phascolarctobacterium (SMD = 1.65, 95% CI: 1.09 to 2.22; I2for heterogeneity = 0%) (Figure 2G) and Lactobacillus (SMD = 0.48, 95% CI: 0.20 to 0.76; I2for heterogeneity = 0%) (Figure 2H) was noted in AD spectrum cases versus HCs. Furthermore, no significant differences in Blautia (SMD = −0.21, 95% CI: −0.28 to 0.71; I2for heterogeneity = 81.5%) (Figure 2I) and Clostridium (SMD = 0.61, 95% CI: −1.15 to 2.38; I2for heterogeneity = 94.3%) were found (Figure 2J).
The relative abundance of three genera in the Bacteroidetes phylum was assessed in eight studies. The pooled effect size for Bacteroides did not exhibit statistical significance when comparing AD spectrum patients and HCs (SMD = −0.17, 95% CI: −0.91 to 0.58; I2for heterogeneity = 92.9%). However, a subgroup meta-analysis based on geographical location revealed an increased abundance in American and Egyptian cohorts with AD spectrum (SMD = 0.79, 95% CI: 0.29 to 1.28; I2for heterogeneity = 58%), while no similar trends were observed in Chinese cohorts (SMD = −0.75, 95% CI: −1.58 to 0.07; I2for heterogeneity = 90.4%) (Figure 3A). Notably, we observed an increased abundance of Alistipes across US AD cohorts (SMD = 0.49, 95% CI: 0.12 to 0.86; I2for heterogeneity = 0%), but a diminished presence in Chinese cohorts (SMD = −0.78, 95% CI: −1.28 to −0.29; I2for heterogeneity = 69.2%) (Figure 3B). Four cohorts from three studies showed no significant differences in Prevotella abundance between AD spectrum patients and HCs (SMD = 0.52, 95% CI: −0.76 to 1.8; I2for heterogeneity = 94.5%) (Figure 3C).
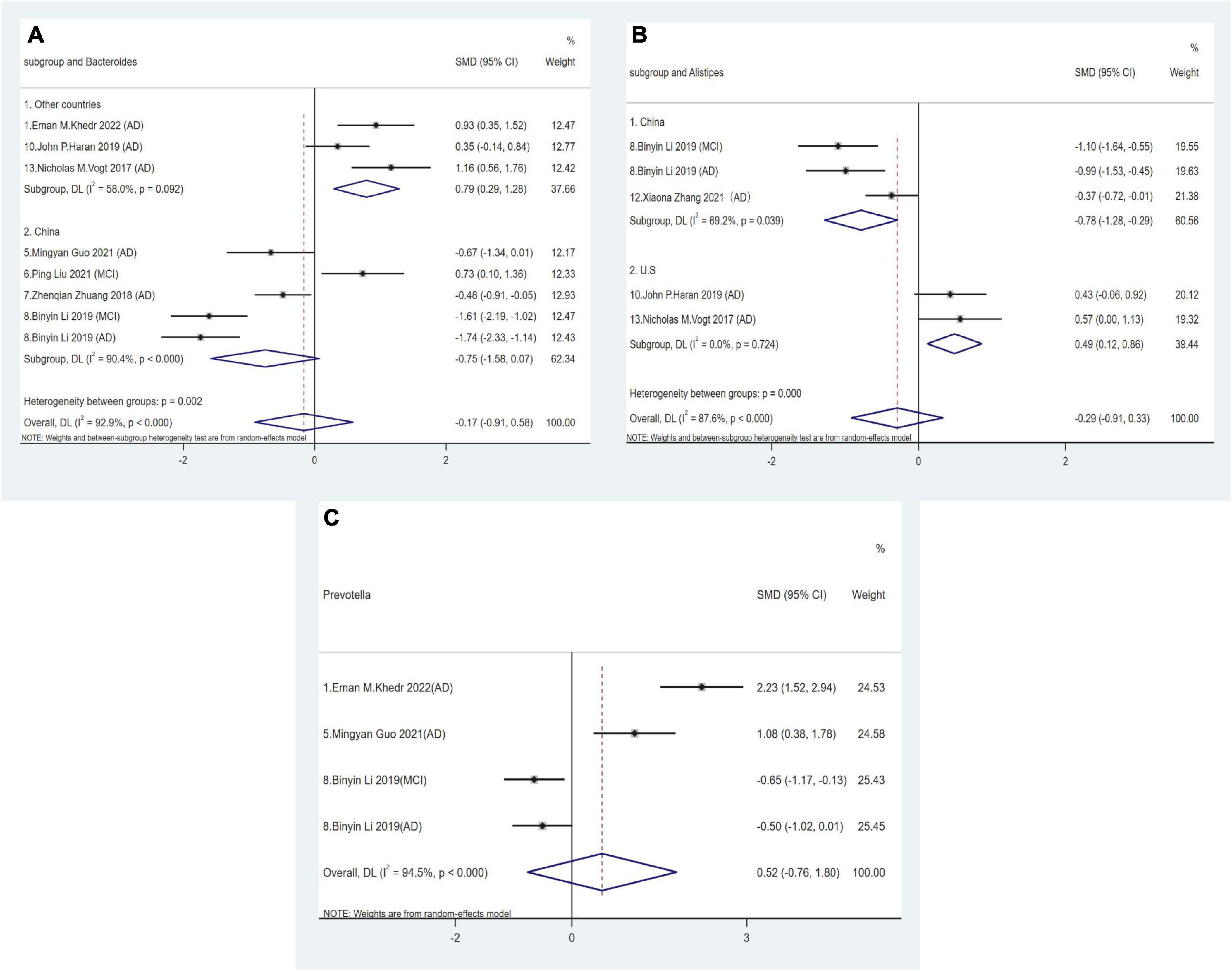
Figure 3. Forest plots of alterations of gut microbiota in the Bacteroidetes phylum compare AD spectrum patients to HCs: (A) Bacteroides, (B) Alistipes, (C) Prevotella.
The Actinobacteria phylum is relatively less abundant in elderly individuals and is predominantly represented by the Bifidobacterium genus (Vaiserman et al., 2017). However, the pooled effect size for Bifidobacterium showed no statistical significance in comparisons between AD spectrum patients and HCs (SMD = 0.1, 95% CI: −042 to 0.63; I2for heterogeneity = 86.5%). Nevertheless, after adjusting for country, Chinese cohorts revealed a positive correlation between AD and relative Bifidobacterium abundance at the genus level (SMD = 0.47, 95% CI: 0.23 to 0.70; I2for heterogeneity = 19%) (Figure 4A).
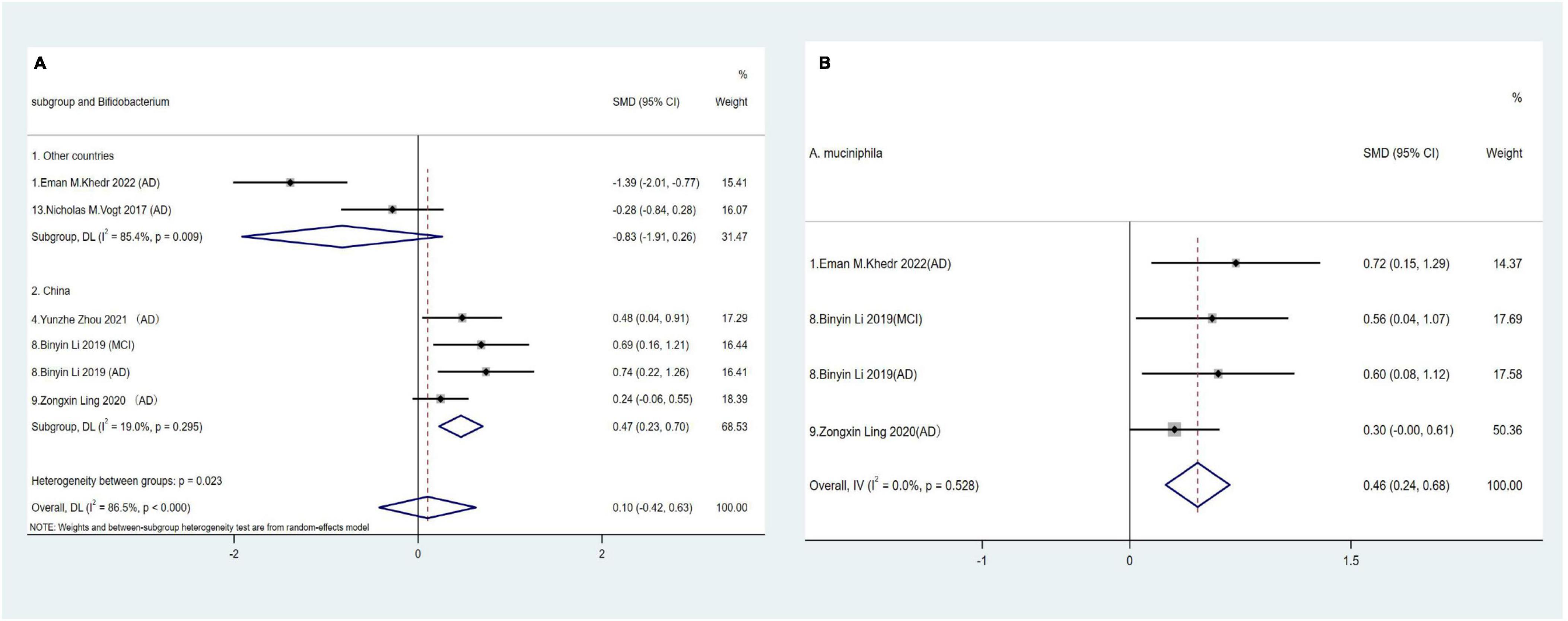
Figure 4. (A) Forest plots of alterations of Bifidobacterium in the Actinobacteria phylum compare AD spectrum patients to HCs. (B) Forest plots of alterations of A. muciniphila in the Verrucobacterium phylum compare AD spectrum patients to HCs.
A. muciniphila is a strictly anaerobic Gram-negative bacterium and the sole representative of the Verrucobacterium phylum in human fecal samples (Cani et al., 2022). Three studies across four cohorts showed consistently significant increases in relative A. muciniphila abundance in AD spectrum patients (SMD = 0.46, 95% CI: 0.24 to 0.68; I2for heterogeneity = 0%) (Figure 4B).
Risk of bias assessments
The critical appraisal of selected studies revealed that for the majority of areas, rigorous methodologies were used. However, some case-controlled studies lacked clear control group selection and provided insufficient detail regarding non-response rates or loss to follow-up (Supplementary Table 1).
Funnel plots were used to evaluate publication bias across indicators. No significant asymmetry was observed in funnel plots, indicating no evidence of publication bias across indicators (Figure 5).
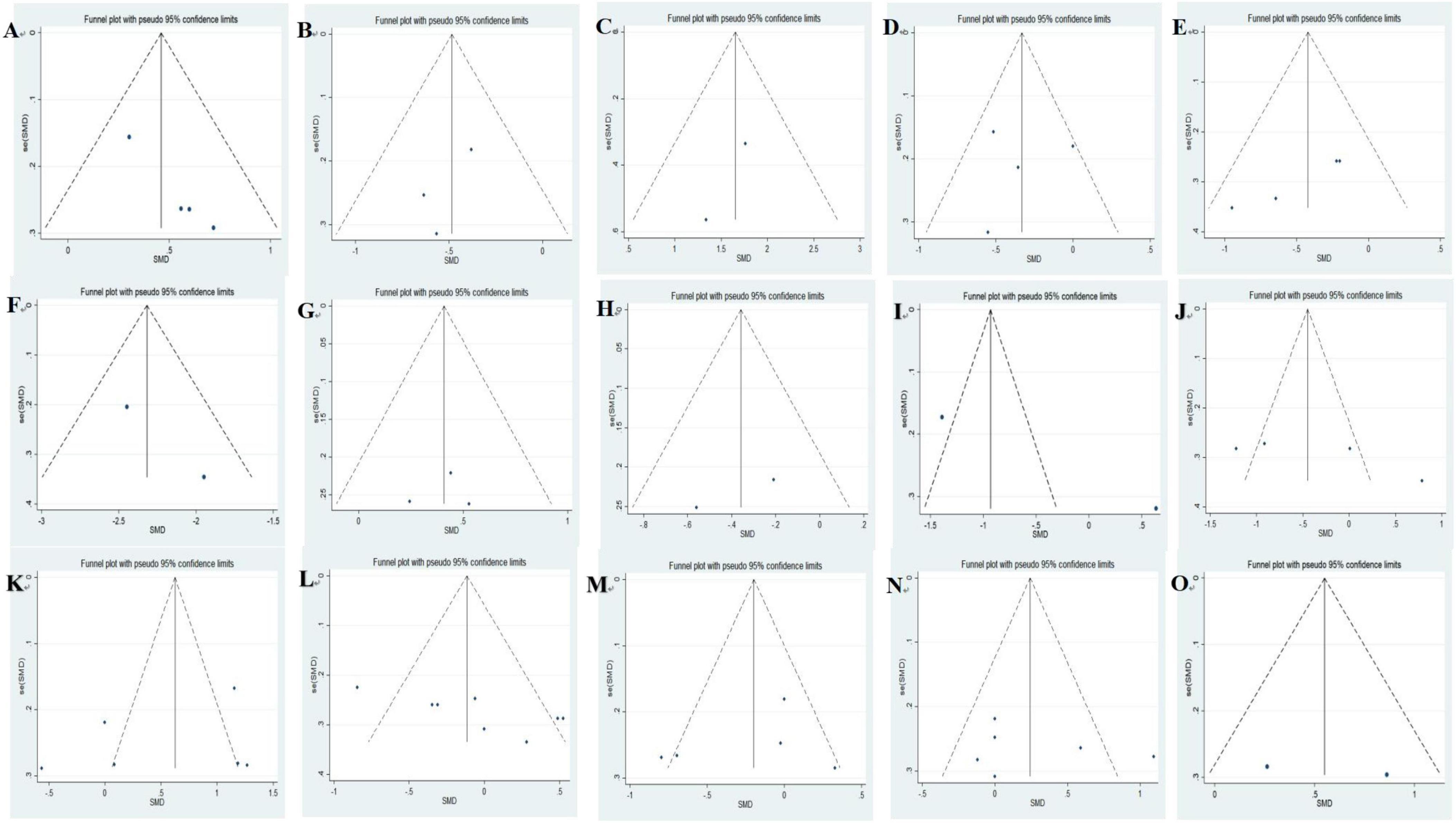
Figure 5. Funnel plots of included studies analyzing alterations of gut microbiota in AD vs. HCs: (A) A. muciniphila, (B) Ruminococcus, (C) Phascolarctobacterium, (D) Faecalibacterium, (E) Lachnospira, (F) Dialister, (G) Lactobacillus, (H) Lachnoclostridium, (I) Roseburia, (J) Prevotella, (K) Bifidobacterium, (L) Bacteroides, (M) Alistipes, (N) Blautia, (O) Clostridium.
Discussion
In recent years, both experimental and observational studies have extensively investigated GM alterations in patients with AD, as the identification of such microbial signatures may provide novel insights into AD pathogenesis and potential therapeutic strategies. In this study, we used 16S rRNA sequencing to explore shifts in GM composition among AD spectrum patients. We observed significant GM composition changes at various taxonomic levels between patients and HCs, and also investigated differential GM abundance in relation to geographical location. Statistically significant lower alpha diversity in AD spectrum patients versus HCs was observed in a substantial proportion of studies, i.e., half for the Shannon index (five out of ten studies), more than half for the Simpson index (four out of six studies), and less than half for Chao1 (three out of seven). Microbial dissimilarities (beta diversity) were observed the gut communities of the two groups.
In the qualitative synthesis, our investigation reveals a statistically significant lower alpha diversity in AD spectrum patients compared with HCs in a substantial proportion of studies, and microbial dissimilarities (beta diversity) between these two groups, were consistently observed across the majority of the studies included, indicating distinct microbial population abundances in the gut of AD spectrum and non-AD spectrum individuals. A previous meta-analysis (Hung et al., 2022) that incorporated eight studies using high-throughput technologies in the 16S rRNA gene showed inconsistent trends in alpha diversity (assessed by the Shannon index and Simpson index) across different stages of AD (MCI and AD), suggesting reduced gut microbiota diversity particularly in the later stages of AD. Another meta-analysis (Jemimah et al., 2023) that re-analyzed he studies included in the Huang Hung et al. (2022) study using QIIME (Quantitative Insights Into Microbial Ecology) software for 16S rRNA gene sequence data yielded results similar to our findings. Among the nine studies included in this analysis, a slight but significant decrease in Shannon diversity was observed, with MCI patients showing increasing trends—although these were non-significant. Moreover, there was stronger consensus in terms of beta diversity, with most studies reporting significant differences among AD, MCI, and cognitively normal cohorts.
In the present systematic review, we found evidence that the composition of GM of AD spectrum persons differs from the non-AD spectrum at the genus level among four phyla. In the quantitative analysis, lower relative proportions of Ruminococcus, Faecalibacterium, Lachnospira, Dialister, Lachnoclostridium, and Roseburia (Firmicutes phylum) were found in the AD spectrum group compared to HCs, whereas the genera of Phascolarctobacterium, Lactobacillus (Firmicutes phylum), and Akkermansia muciniphila (Verrucomicrobia phylum) were found to be significantly higher in patients. Furthermore, regional variations may have been in play for intestinal microbes such as Bacteroides, Alistipes (Bacteroidetes phylum) and Bifidobacterium (Actinobacteria phylum).
Mechanistic explanations for changes in GM composition at various taxonomic levels between AD spectrum and non-AD spectrum individuals may be provided by studies investigating the host-gut microbiota relationship through correlating GM composition with circulating metabolites (van der Hee and Wells, 2021). For example, short-chain fatty acids (SCFAs) are generated by gut commensal microbes during indigestible dietary fiber fermentation, including acetate, propionate and butyrate, which possess immunomodulatory potential (Rescigno, 2014). Our study found that almost genera within the phylum Firmicutes, such as Ruminococcus, Faecalibacterium, Lachnospira, Dialister, Lachnoclostridium, and Roseburia, are positively correlated with SCFAs (Rescigno, 2014; Sakamoto et al., 2020; Pal et al., 2021; Cai et al., 2022; Ordoñez-Rodriguez et al., 2023), which supports the hypothesis that AD spectrum persons have a distinct microbial profile that is more efficient in fermenting substrates and in producing higher fecal SCFAs concentrations than that of cognitively normal counterparts (Seo and Holtzman, 2024). Phascolarctobacterium have been previously reported to be negatively associated with cognitive function indicators, suggesting that mitochondrial damage, calcium homeostasis imbalance, and inflammation may play a role in AD (Ling et al., 2020; Diviccaro et al., 2023; Jemimah et al., 2023). A. muciniphila is a mucin-degrading bacterium which uses mucin as its primary energy source; it also exacerbates inflammation by degrading protective mucus layers and exposing immune cells to increased microbial antigen and toxin levels (Jian et al., 2023). In line with our findings, Ling et al. (2020) who used 16S rRNA sequencing to characterize the GM and observed increased A. muciniphila abundance in Chinese- and Kazakh-based AD cohorts. In addition, Li et al. (2019) reported a negative correlation between A. muciniphila abundance and Mini-Mental State Examination scores, suggesting potential associations with hippocampal atrophy. Interestingly, contrary to earlier findings, we observed a positive association between Lactobacillus species abundance and AD risk in our analyses. Although commonly used as probiotics with beneficial host health effects (Huang et al., 2022), previous studies reported that Lactobacillus produced γ-aminobutyric acid (GABA) via glutamate metabolism, which regulated GABA levels in the cerebral cortex and improved cognition, mood, and behavior. In addition, Lactobacillus reduced kynurenine concentrations and subsequently enhanced cognitive function in depressed patients (Rudzki et al., 2019). Significantly, a dysregulated kynurenine pathway in the tryptophan metabolic route was proposed as a prominent contributor to AD (Almulla et al., 2022; Liang et al., 2022). However, not all cognitive effects due to Lactobacillus are positive. For instance, an increased relative Lactobacillus abundance was implicated in obesity and diabetes pathophysiology in both mice and patients (Karlsson et al., 2013; Zeng et al., 2013; Arora et al., 2017). These findings highlight the complexity of GM ecosystem and suggest that a lower relative abundance of the phylum Firmicutes and a greater relative abundance of Verrucomicrobia in the AD do not necessarily translate into a common pattern across all genera within these phyla, since several genera from the same phylum may be found in higher or in lower proportions in AD spectrum individuals.
Overall, discrepancies found for regional variations in the abundance of Bacteroides, Alistipes and Bifidobacterium when comparing AD spectrum with HCs may reflect differences in dietary pattern and lifestyle. For example, Dilmore et al. (2023) exploring dietary intervention effects on the microbiome in adults at risk for AD showed that the low-fat American Heart Association Diet increased GABA production in MCI individuals by increasing GABA-producing Alistipes. This finding contradicted previous studies reporting dysfunction of GABA signaling aggravating AD pathology and showing lower levels of GABA in postmortem brain tissue from AD patients (Govindpani et al., 2017; Andersen et al., 2022). A previous meta-analysis study has found that an overgrowth of Bacteroides in US-based AD cohorts, while this pattern was not observed in Chinese cohorts. The discrepancy might be due to geographical differences in diet, patient populations and in the RNA sequencing area. A large amount of study-specific variation in GM composition and diversity can likely be attributed to interpersonal variability (Ursell et al., 2012). It has been reported that if taxonomic compositions of two individuals’ gut microbiota are quite different, they may still exhibit similar functional activities (Seo and Holtzman, 2024). This current recognition of “functional redundancy” underscores the importance of studying the functional activity and the metabolic potential of GM rather than solely relying on taxonomic composition (Fetzer et al., 2015). Therefore, in order to strengthen the robustness of current evidence, additional individual-level meta-analyses are warranted to elucidate the overall composition, diversity, and stability of the gut microbial network and understand its functional interactions in AD. These analyses should adjust for confounders in a comparable fashion covering critical factors influencing the gut microbiome composition, such as sex, age, diet, physical activity, drug interventions, RNA sequencing area, and standardized microbiome data processing.
Our study strength lies in its comprehensive range of outcomes and rigorous methodology. Furthermore, for better comparability, we only included studies that analyzed the gut microbiome composition by means of high-throughput sequencing techniques. However, several limitations are worth mentioning. First, our study mainly included a cross-sectional design, which only allowed for the identification of associations rather than causal relationships between GM and AD development. Second, despite a thorough and systematic search across databases, selected studies predominantly came from three countries with relatively small sample sizes; thus caution should be exercised when generalizing our findings to other populations. Third, due to limited available study outcomes, we could not analyze dementia subtypes so the impact of GM may vary among different subtypes. Fourth, while we used mature 16S rRNA sequencing in our study, combining metagenomic sequencing with multiomics approaches could potentially provide more comprehensive and accurate microbial information while minimizing methodological bias. Lastly, although we adjusted for important potential confounders, such as age, sex, BMI, and diabetes, it is important to acknowledge that statistical adjustments may not have completely addressed these issues. Notably, dietary factors were shown to influence GM composition, making it difficult to draw consistent GM profiles in AD patients and even in HCs.
Conclusion
We systematically evaluated studies investigating altered GM composition using 16S rRNA sequencing in AD spectrum patients when compared to HCs. In summary, a reduced microbiota diversity and a significantly distinct pattern of clustering with regard to beta diversity were observed in AD spectrum patients when compared with those in HCs. Additionally, consistent with previous findings, patients with AD spectrum exhibited significant intestinal flora changes. Specifically, decreased Ruminococcus, Faecalibacterium, Lachnospira, Dialister, Lachnoclostridium, and Roseburia abundance was recorded in patients while Phascolarctobacterium, Lactobacillus, and A. muciniphila were more enriched when compared to HCs. No significant differences in the relative abundance of other microbial taxa were identified. Furthermore, regional variations may have been in play for the GM, such as Bacteroides, Bifidobacterium, and Alistipes. Currently, debates are ongoing regarding GM pathogenicity and associated metabolites; therefore, well-designed studies using innovative approaches are required to gain a better understanding of the GM in AD development. Such insights may pave the way for novel GM-based strategies to prevent and treat AD.
Data availability statement
The original contributions presented in this study are included in this article/Supplementary material, further inquiries can be directed to the corresponding authors.
Author contributions
HL: Data curation, Formal analysis, Investigation, Methodology, Project administration, Software, Visualization, Writing – original draft. XC: Data curation, Software, Visualization, Writing – original draft. YL: Methodology, Software, Supervision, Validation, Visualization, Writing – original draft. FH: Formal analysis, Investigation, Project administration, Visualization, Writing – original draft. AT: Conceptualization, Supervision, Writing – review & editing. RZ: Conceptualization, Project administration, Supervision, Writing – review & editing.
Funding
The authors declare that financial support was received for the research, authorship, and/or publication of this article. This work was supported by STI2030-Major Projects (Grant number: 2021ZD0201802).
Conflict of interest
The authors declare that the research was conducted in the absence of any commercial or financial relationships that could be construed as a potential conflict of interest.
Publisher’s note
All claims expressed in this article are solely those of the authors and do not necessarily represent those of their affiliated organizations, or those of the publisher, the editors and the reviewers. Any product that may be evaluated in this article, or claim that may be made by its manufacturer, is not guaranteed or endorsed by the publisher.
Supplementary material
The Supplementary Material for this article can be found online at: https://www.frontiersin.org/articles/10.3389/fnagi.2024.1422350/full#supplementary-material
Footnotes
References
Almulla, A., Supasitthumrong, T., Amrapala, A., Tunvirachaisakul, C., Jaleel, A., Oxenkrug, G., et al. (2022). The tryptophan catabolite or kynurenine pathway in Alzheimer’s disease: A systematic review and meta-analysis. J. Alzheimers Dis. 88, 1325–1339. doi: 10.3233/jad-220295
Alzheimer’s (2023). 2023 Alzheimer’s disease facts and figures. Alzheimers Dement. 19, 1598–1695. doi: 10.1002/alz.13016
Andersen, J., Schousboe, A., and Verkhratsky, A. (2022). Astrocyte energy and neurotransmitter metabolism in Alzheimer’s disease: Integration of the glutamate/GABA-glutamine cycle. Prog. Neurobiol. 217:102331. doi: 10.1016/j.pneurobio.2022.102331
Arora, T., Seyfried, F., Docherty, N., Tremaroli, V., le Roux, C., Perkins, R., et al. (2017). Diabetes-associated microbiota in fa/fa rats is modified by Roux-en-Y gastric bypass. ISME J. 11, 2035–2046. doi: 10.1038/ismej.2017.70
Ashley, N. B., Adrien, P., Tarik, S. O., and Nicola, J. A. (2022). Astrocyte contribution to dysfunction, risk and progression in neurodegenerative disorders. Nat. Rev. Neurosci. 24, 23–39. doi: 10.1038/s41583-022-00641-1
Bonfili, L., Cecarini, V., Cuccioloni, M., Angeletti, M., Berardi, S., Scarpona, S., et al. (2018). SLAB51 probiotic formulation activates SIRT1 pathway promoting antioxidant and neuroprotective effects in an AD mouse model. Mol. Neurobiol. 55, 7987–8000. doi: 10.1007/s12035-018-0973-4
Cai, Y., Huang, F., Lao, X., Lu, Y., Gao, X., Alolga, R., et al. (2022). Integrated metagenomics identifies a crucial role for trimethylamine-producing Lachnoclostridium in promoting atherosclerosis. NPJ Biofilms Microb. 8:11. doi: 10.1038/s41522-022-00273-4
Cani, P., Depommier, C., Derrien, M., Everard, A., and de Vos, W. (2022). Akkermansia muciniphila: Paradigm for next-generation beneficial microorganisms. Nat. Rev. Gastroenterol. Hepatol. 19, 625–637. doi: 10.1038/s41575-022-00631-9
Cheng, H., Ning, M., Chen, D., and Ma, W. (2019). Interactions between the gut microbiota and the host innate immune response against pathogens. Front. Immunol. 10:607. doi: 10.3389/fimmu.2019.00607
Cox, L., and Weiner, H. (2018). Microbiota signaling pathways that influence neurologic disease. Neurotherapeutics 15, 135–145. doi: 10.1007/s13311-017-0598-8
Dilmore, A., Martino, C., Neth, B., West, K., Zemlin, J., Rahman, G., et al. (2023). Effects of a ketogenic and low-fat diet on the human metabolome, microbiome, and foodome in adults at risk for Alzheimer’s disease. Alzheimers Dement. 19, 4805–4816. doi: 10.1002/alz.13007
Diviccaro, S., Cioffi, L., Piazza, R., Caruso, D., Melcangi, R., and Giatti, S. (2023). Neuroactive steroid-gut microbiota interaction in T2DM diabetic encephalopathy. Biomolecules 13:1325. doi: 10.3390/biom13091325
Fernandes, A., Tábuas-Pereira, M., Duro, D., Lima, M., Gens, H., Santiago, B., et al. (2020). C-reactive protein as a predictor of mild cognitive impairment conversion into Alzheimer’s disease dementia. Exp. Gerontol. 138:111004. doi: 10.1016/j.exger.2020.111004
Ferreiro, A., Choi, J., Ryou, J., Newcomer, E., Thompson, R., Bollinger, R., et al. (2023). Gut microbiome composition may be an indicator of preclinical Alzheimer’s disease. Sci. Transl. Med. 15:eabo2984. doi: 10.1126/scitranslmed.abo2984
Fetzer, I., Johst, K., Schäwe, R., Banitz, T., Harms, H., and Chatzinotas, A. (2015). The extent of functional redundancy changes as species’ roles shift in different environments. Proc. Natl. Acad. Sci. U.S.A. 112, 14888–14893. doi: 10.1073/pnas.1505587112
Finegold, S., Dowd, S., Gontcharova, V., Liu, C., Henley, K., Wolcott, R., et al. (2010). Pyrosequencing study of fecal microflora of autistic and control children. Anaerobe 16, 444–453. doi: 10.1016/j.anaerobe.2010.06.008
First, M. B., Frances, A., and Pincus, H. A. (2002). DSM-IV-TR handbook of differential diagnosis. Arlington, VA: American Psychiatric Publishing, Inc.
Forde, B., and O’Toole, P. (2013). Next-generation sequencing technologies and their impact on microbial genomics. Briefings Funct. Genom. 12, 440–453. doi: 10.1093/bfgp/els062
Gao, B., Chi, L., Zhu, Y., Shi, X., Tu, P., Li, B., et al. (2021). An introduction to next generation sequencing bioinformatic analysis in gut microbiome studies. Biomolecules 11:530. doi: 10.3390/biom11040530
Gauthier, S., Rosa-Neto, P., Morais, J., and Webster, C. (2021). World Alzheimer report 2021: Journey through the diagnosis of dementia. London: Alzheimer’s Disease International.
Govindpani, K., Calvo-Flores Guzmán, B., Vinnakota, C., Waldvogel, H., Faull, R., and Kwakowsky, A. (2017). Towards a better understanding of GABAergic remodeling in Alzheimer’s disease. Int. J. Mol. Sci. 18:1813. doi: 10.3390/ijms18081813
Guo, M., Peng, J., Huang, X., Xiao, L., Huang, F., and Zuo, Z. (2021). Gut microbiome features of chinese patients newly diagnosed with Alzheimer’s disease or mild cognitive impairment. J. Alzheimers Dis. 80, 299–310. doi: 10.3233/jad-201040
Haran, J., Bhattarai, S., Foley, S., Dutta, P., Ward, D., Bucci, V., et al. (2019). Alzheimer’s disease microbiome is associated with dysregulation of the anti-inflammatory P-glycoprotein pathway. mBio 10:e00632–19. doi: 10.1128/mBio.00632-19
Higgins, J., Altman, D., Gøtzsche, P., Jüni, P., Moher, D., Oxman, A., et al. (2011). The Cochrane Collaboration’s tool for assessing risk of bias in randomised trials. Br. Med. J. 343:d5928. doi: 10.1136/bmj.d5928
Higgins, J., Thompson, S., Deeks, J., and Altman, D. (2003). Measuring inconsistency in meta-analyses. Br. Med. J. 327, 557–560. doi: 10.1136/bmj.327.7414.557
Hill, J., and Lukiw, W. (2015). Microbial-generated amyloids and Alzheimer’s disease (AD). Front. Aging Neurosci. 7:9. doi: 10.3389/fnagi.2015.00009
Huang, R., Wu, F., Zhou, Q., Wei, W., Yue, J., Xiao, B., et al. (2022). Lactobacillus and intestinal diseases: Mechanisms of action and clinical applications. Microbiol. Res. 260:127019. doi: 10.1016/j.micres.2022.127019
Hung, C., Chang, C., Huang, C., Nouchi, R., and Cheng, C. (2022). Gut microbiota in patients with Alzheimer’s disease spectrum: A systematic review and meta-analysis. Aging 14, 477–496. doi: 10.18632/aging.203826
Hwang, Y., Park, S., Paik, J., Chae, S., Kim, D., Jeong, D., et al. (2019). Lactobacillus plantarum efficacy and safety of C29-fermented soybean (DW2009) in individuals with mild cognitive impairment: A 12-week, multi-center, randomized, double-blind, placebo-controlled clinical trial. Nutrients 11:305. doi: 10.3390/nu11020305
Hyman, B., Phelps, C., Beach, T., Bigio, E., Cairns, N., Carrillo, M., et al. (2012). National institute on aging-Alzheimer’s association guidelines for the neuropathologic assessment of Alzheimer’s disease. Alzheimers Dement. 8, 1–13. doi: 10.1016/j.jalz.2011.10.007
Jemimah, S., Chabib, C., Hadjileontiadis, L., and AlShehhi, A. (2023). Gut microbiome dysbiosis in Alzheimer’s disease and mild cognitive impairment: A systematic review and meta-analysis. PLoS One 18:e0285346. doi: 10.1371/journal.pone.0285346
Jian, H., Liu, Y., Wang, X., Dong, X., and Zou, X. (2023). Akkermansia muciniphila as a next-generation probiotic in modulating human metabolic homeostasis and disease progression: A role mediated by gut-liver-brain axes? Int. J. Mol. Sci. 24:3900. doi: 10.3390/ijms24043900
Kaiyrlykyzy, A., Kozhakhmetov, S., Babenko, D., Zholdasbekova, G., Alzhanova, D., Olzhayev, F., et al. (2022). Study of gut microbiota alterations in Alzheimer’s dementia patients from Kazakhstan. Sci. Rep. 12:15115. doi: 10.1038/s41598-022-19393-0
Karlsson, F., Tremaroli, V., Nookaew, I., Bergström, G., Behre, C., Fagerberg, B., et al. (2013). Gut metagenome in European women with normal, impaired and diabetic glucose control. Nature 498, 99–103. doi: 10.1038/nature12198
Khedr, E., Omeran, N., Karam-Allah Ramadan, H., Ahmed, G., and Abdelwarith, A. (2022). Alteration of gut microbiota in Alzheimer’s disease and their relation to the cognitive impairment. J. Alzheimers Dis. 88, 1103–1114. doi: 10.3233/jad-220176
Kim, M., Kim, Y., Choi, H., Kim, W., Park, S., Lee, D., et al. (2020). Transfer of a healthy microbiota reduces amyloid and tau pathology in an Alzheimer’s disease animal model. Gut 69, 283–294. doi: 10.1136/gutjnl-2018-317431
Kowalski, K., and Mulak, A. (2019). Brain-gut-microbiota axis in Alzheimer’s disease. J. Neurogastroenterol. Motil. 25, 48–60. doi: 10.5056/jnm18087
Li, B., He, Y., Ma, J., Huang, P., Du, J., Cao, L., et al. (2019). Mild cognitive impairment has similar alterations as Alzheimer’s disease in gut microbiota. Alzheimers Dement. 15, 1357–1366. doi: 10.1016/j.jalz.2019.07.002
Liang, Y., Xie, S., He, Y., Xu, M., Qiao, X., Zhu, Y., et al. (2022). Kynurenine pathway metabolites as biomarkers in Alzheimer’s disease. Dis. Mark. 2022:9484217. doi: 10.1155/2022/9484217
Ling, Z., Zhu, M., Yan, X., Cheng, Y., Shao, L., Liu, X., et al. (2020). Structural and functional dysbiosis of fecal microbiota in chinese patients with Alzheimer’s disease. Front. Cell. Dev. Biol. 8:634069. doi: 10.3389/fcell.2020.634069
Liu, P., Jia, X., Chen, Y., Yu, Y., Zhang, K., Lin, Y., et al. (2021). Gut microbiota interacts with intrinsic brain activity of patients with amnestic mild cognitive impairment. CNS Neurosci. Ther. 27, 163–173. doi: 10.1111/cns.13451
Liu, P., Wu, L., Peng, G., Han, Y., Tang, R., Ge, J., et al. (2019). Altered microbiomes distinguish Alzheimer’s disease from amnestic mild cognitive impairment and health in a Chinese cohort. Brain Behav. Immun. 80, 633–643. doi: 10.1016/j.bbi.2019.05.008
Long, J., and Holtzman, D. (2019). Alzheimer disease: An update on pathobiology and treatment strategies. Cell 179, 312–339. doi: 10.1016/j.cell.2019.09.001
Nagpal, R., Neth, B., Wang, S., Craft, S., and Yadav, H. (2019). Modified Mediterranean-ketogenic diet modulates gut microbiome and short-chain fatty acids in association with Alzheimer’s disease markers in subjects with mild cognitive impairment. EBioMedicine 47, 529–542. doi: 10.1016/j.ebiom.2019.08.032
Ordoñez-Rodriguez, A., Roman, P., Rueda-Ruzafa, L., Campos-Rios, A., and Cardona, D. (2023). Changes in gut microbiota and multiple sclerosis: A systematic review. Int. J. Environ. Res. Public Health 20:4624. doi: 10.3390/ijerph20054624
Pal, D., Naskar, M., Bera, A., and Mukhopadhyay, B. (2021). Chemical synthesis of the pentasaccharide repeating unit of the O-specific polysaccharide from Ruminococcus gnavus. Carbohydr. Res. 507:108384. doi: 10.1016/j.carres.2021.108384
Rescigno, M. (2014). Intestinal microbiota and its effects on the immune system. Cell Microbiol. 16, 1004–1013. doi: 10.1111/cmi.12301
Rinninella, E., Raoul, P., Cintoni, M., Franceschi, F., Miggiano, G. A. D., Gasbarrini, A., et al. (2019). What is the healthy gut microbiota composition? A changing ecosystem across age, environment, diet, and diseases. Microorganisms 7:14. doi: 10.3390/microorganisms7010014
Rolhion, N., and Chassaing, B. (2016). When pathogenic bacteria meet the intestinal microbiota. Philos. Trans. R. Soc. Lond. B Biol. Sci. 371:504. doi: 10.1098/rstb.2015.0504
Rudzki, L., Ostrowska, L., Pawlak, D., Małus, A., Pawlak, K., Waszkiewicz, N., et al. (2019). Probiotic Lactobacillus plantarum 299v decreases kynurenine concentration and improves cognitive functions in patients with major depression: A double-blind, randomized, placebo controlled study. Psychoneuroendocrinology 100, 213–222. doi: 10.1016/j.psyneuen.2018.10.010
Sakamoto, M., Ikeyama, N., Toyoda, A., Murakami, T., Mori, H., Iino, T., et al. (2020). Dialister hominis sp. nov., isolated from human faeces. Int. J. Syst. Evol. Microbiol. 70, 589–595. doi: 10.1099/ijsem.0.003797
Scheperjans, F., Aho, V., Pereira, P., Koskinen, K., Paulin, L., Pekkonen, E., et al. (2015). Gut microbiota are related to Parkinson’s disease and clinical phenotype. Mov. Disord. 30, 350–358. doi: 10.1002/mds.26069
Seo, D., and Holtzman, D. (2024). Current understanding of the Alzheimer’s disease-associated microbiome and therapeutic strategies. Exp. Mol. Med. 56, 86–94. doi: 10.1038/s12276-023-01146-2
Sheng, C., Lin, L., Lin, H., Wang, X., Han, Y., and Liu, S. (2021). Altered gut microbiota in adults with subjective cognitive decline: The SILCODE study. J. Alzheimers Dis. 82, 513–526. doi: 10.3233/jad-210259
Sochocka, M., Donskow-Łysoniewska, K., Diniz, B., Kurpas, D., Brzozowska, E., and Leszek, J. (2019). The gut microbiome alterations and inflammation-driven pathogenesis of Alzheimer’s disease-a critical review. Mol. Neurobiol. 56, 1841–1851. doi: 10.1007/s12035-018-1188-4
Sterne, J., Sutton, A., Ioannidis, J., Terrin, N., Jones, D., Lau, J., et al. (2011). Recommendations for examining and interpreting funnel plot asymmetry in meta-analyses of randomised controlled trials. Br. Med. J. 343:d4002. doi: 10.1136/bmj.d4002
Sun, J., Xu, J., Ling, Y., Wang, F., Gong, T., Yang, C., et al. (2019). Fecal microbiota transplantation alleviated Alzheimer’s disease-like pathogenesis in APP/PS1 transgenic mice. Transl. Psychiatry 9:189. doi: 10.1038/s41398-019-0525-3
Taylor, J., and Matthews, S. (2015). New insight into the molecular control of bacterial functional amyloids. Front. Cell. Infect. Microbiol. 5:33. doi: 10.3389/fcimb.2015.00033
Ursell, L., Clemente, J., Rideout, J., Gevers, D., Caporaso, J., and Knight, R. (2012). The interpersonal and intrapersonal diversity of human-associated microbiota in key body sites. J. Allergy Clin. Immunol. 129, 1204–1208. doi: 10.1016/j.jaci.2012.03.010
Vaiserman, A., Koliada, A., and Marotta, F. (2017). Gut microbiota: A player in aging and a target for anti-aging intervention. Ageing Res. Rev. 35, 36–45. doi: 10.1016/j.arr.2017.01.001
Valles-Colomer, M., Falony, G., Darzi, Y., Tigchelaar, E., Wang, J., Tito, R., et al. (2019). The neuroactive potential of the human gut microbiota in quality of life and depression. Nat. Microbiol. 4, 623–632. doi: 10.1038/s41564-018-0337-x
van der Hee, B., and Wells, J. (2021). Microbial regulation of host physiology by short-chain fatty acids. Trends Microbiol. 29, 700–712. doi: 10.1016/j.tim.2021.02.001
Vogt, N., Kerby, R., Dill-McFarland, K., Harding, S., Merluzzi, A., Johnson, S., et al. (2017). Gut microbiome alterations in Alzheimer’s disease. Sci. Rep. 7:13537. doi: 10.1038/s41598-017-13601-y
Wells, G., Shea, B., O’connell, D., Peterson, J., Welch, V., Losos, M., et al. (2000). The Newcastle-Ottawa scale (NOS) for assessing the quality of nonrandomised studies in meta-analyses. Available online at: http://www.ohri.ca/programs/clinical_epidemiology/oxford.asp (accessed March 11, 2024).
Yang, S., and David, M. H. (2018). Interplay between innate immunity and Alzheimer disease: APOE and TREM2 in the spotlight. Nat. Rev. Immunol. 18, 759–772. doi: 10.1038/s41577-018-0051-1
Zeng, H., Liu, J., Jackson, M., Zhao, F., Yan, L., and Combs, G. (2013). Fatty liver accompanies an increase in lactobacillus species in the hind gut of C57BL/6 mice fed a high-fat diet. J. Nutr. 143, 627–631. doi: 10.3945/jn.112.172460
Zhang, X., Wang, Y., Liu, W., Wang, T., Wang, L., Hao, L., et al. (2021). Diet quality, gut microbiota, and microRNAs associated with mild cognitive impairment in middle-aged and elderly Chinese population. Am. J. Clin. Nutr. 114, 429–440. doi: 10.1093/ajcn/nqab078
Zhou, Y., Wang, Y., Quan, M., Zhao, H., and Jia, J. (2021). Gut microbiota changes and their correlation with cognitive and neuropsychiatric symptoms in Alzheimer’s disease. J. Alzheimers Dis. 81, 583–595. doi: 10.3233/jad-201497
Keywords: Alzheimer’s disease, gut microbiota, 16S rRNA sequencing, biomarkers, meta-analysis
Citation: Li H, Cui X, Lin Y, Huang F, Tian A and Zhang R (2024) Gut microbiota changes in patients with Alzheimer’s disease spectrum based on 16S rRNA sequencing: a systematic review and meta-analysis. Front. Aging Neurosci. 16:1422350. doi: 10.3389/fnagi.2024.1422350
Received: 23 April 2024; Accepted: 22 July 2024;
Published: 08 August 2024.
Edited by:
Gianluigi Forloni, Mario Negri Pharmacological Research Institute (IRCCS), ItalyReviewed by:
Prabhakar Singh, Sathyabama Institute of Science and Technology, IndiaSharad Purohit, Augusta University, United States
Copyright © 2024 Li, Cui, Lin, Huang, Tian and Zhang. This is an open-access article distributed under the terms of the Creative Commons Attribution License (CC BY). The use, distribution or reproduction in other forums is permitted, provided the original author(s) and the copyright owner(s) are credited and that the original publication in this journal is cited, in accordance with accepted academic practice. No use, distribution or reproduction is permitted which does not comply with these terms.
*Correspondence: Rongwei Zhang, rongweizhang@126.com; Ayong Tian, tianayong@126.com
†These authors have contributed equally to this work and share first authorship