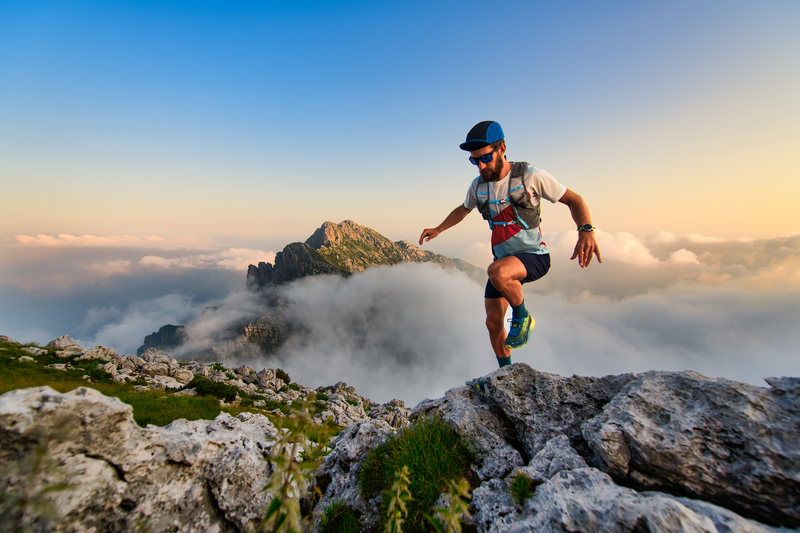
95% of researchers rate our articles as excellent or good
Learn more about the work of our research integrity team to safeguard the quality of each article we publish.
Find out more
ORIGINAL RESEARCH article
Front. Aging Neurosci. , 12 September 2024
Sec. Alzheimer's Disease and Related Dementias
Volume 16 - 2024 | https://doi.org/10.3389/fnagi.2024.1412735
Background: The relationship between white matter hyperintensities (WMH) and the core features of Alzheimer’s disease (AD) remains controversial. Further, due to the prevalence of co-pathologies, the precise role of WMH in cognition and neurodegeneration also remains uncertain.
Methods: Herein, we analyzed 1803 participants with available WMH volume data, extracted from the ADNI database, including 756 cognitively normal controls, 783 patients with mild cognitive impairment (MCI), and 264 patients with dementia. Participants were grouped according to cerebrospinal fluid (CSF) pathology (A/T profile) severity. Linear regression analysis was applied to evaluate the factors associated with WMH volume. Modeled by linear mixed-effects, the increase rates (Δ) of the WMH volume, cognition, and typical neurodegenerative markers were assessed. The predictive effectiveness of WMH volume was subsequently tested using Cox regression analysis, and the relationship between WMH/ΔWMH and other indicators such as cognition was explored through linear regression analyses. Furthermore, we explored the interrelationship among amyloid-β deposition, cognition, and WMH using mediation analysis.
Results: Higher WMH volume was associated with older age, lower CSF amyloid-β levels, hypertension, and smoking history (all p ≤ 0.001), as well as cognitive status (MCI, p < 0.001; dementia, p = 0.008), but not with CSF tau levels. These results were further verified in any clinical stage, except hypertension and smoking history in the dementia stage. Although WMH could not predict dementia conversion, its increased levels at baseline were associated with a worse cognitive performance and a more rapid memory decline. Longitudinal analyses showed that baseline dementia and positive amyloid-β status were associated with a greater accrual of WMH volume, and a higher ΔWMH was also correlated with a faster cognitive decline. In contrast, except entorhinal cortex thickness, the WMH volume was not found to be associated with any other neurodegenerative markers. To a lesser extent, WMH mediates the relationship between amyloid-β and cognition.
Conclusion: WMH are non-specific lesions that are associated with amyloid-β deposition, cognitive status, and a variety of vascular risk factors. Despite evidence indicating only a weak relationship with neurodegeneration, early intervention to reduce WMH lesions remains a high priority for preserving cognitive function in the elderly.
Alzheimer’s disease (AD) is a leading cause of dementia and one of the major causes of disability, dependency, and even death among older people worldwide. However, the disease still lacks significantly effective disease-modifying therapy methods and cannot be cured (Long and Holtzman, 2019; Zheng and Wang, 2024). At present, AD is considered a distinctive entity, and is defined pathologically by the presence of the following specific neuropathological profiles: extracellular deposition of amyloid-β (Aβ) and intraneuronal presence of aggregated hyperphosphorylated tau proteins (Jack et al., 2018; Long and Holtzman, 2019; Zheng and Wang, 2024). In addition to these core features, vascular pathologies are important and should not be disregarded. According to several postmortem investigations, dementia with pure AD pathologies is uncommon, with the majority of patients exhibiting mixed brain pathological alterations (Schneider et al., 2007; Grinberg et al., 2013; Toledo et al., 2013). By employing data-driven analytical techniques, several researchers have identified vascular dysregulation as the earliest and strongest pathological biomarker of late-onset AD (Iturria-Medina et al., 2016). Recently, our and other research groups have outlined the relationship between AD and cerebrovascular injury (Love and Miners, 2016; Klohs, 2019; Cortes-Canteli and Iadecola, 2020; Li and Liu, 2022; Rost et al., 2022). Given the lack of effective therapies, determining the correlation between cerebrovascular lesions and AD could potentially guide interventions tailored to specific risks, and preserve cognitive function in the elderly population.
White matter hyperintensities (WMH), a classical imaging hallmark of cerebral small vessel disease (CSVD), are visualized as elevated intensities on T2-weighted or fluid-attenuated inversion recovery (FLAIR) magnetic resonance imaging (MRI), and their importance has been reemphasized in the latest consensus guidelines (STRIVE-2) (Duering et al., 2023). Similar to macroscopic infarcts, the contribution of cerebral microangiopathy to dementia is also substantial (Troncoso et al., 2008). However, it seems inappropriate to regard WMH purely as vascular issues (Garnier-Crussard et al., 2023). Notably, a recent investigation of autosomal dominant inherited AD revealed that increased WMH volume is an early imaging feature of preclinical AD (Lee et al., 2016). In terms of ordering, WMH was visible following amyloid and tau pathologies and prior to symptom onset, with a volume accumulation that may be due to alterations in these indicators (Lee et al., 2016; Luo et al., 2023). Whether amyloid and tau pathologies are linked to the occurrence of WMH is controversial. While some studies have found an associated between higher amyloid burden and larger WMH volumes in patients with dementia (van Westen et al., 2016; Walsh et al., 2020; van Waalwijk van Doorn et al., 2021), mild cognitive impairment (MCI) (Marnane et al., 2016; Wei et al., 2019; Walsh et al., 2020), and cognitively normal controls (NCs) (Kester et al., 2014; Marnane et al., 2016; Osborn et al., 2018; Graff-Radford et al., 2019; Wei et al., 2019; Walsh et al., 2020), other studies did not detect any such a relationship (Hedden et al., 2012; Marchant et al., 2013; Park et al., 2014; Luo et al., 2023; Ortner et al., 2023; Ying et al., 2023). Mixed results have also been obtained from different meta-analyses (Roseborough et al., 2017; Liu et al., 2018; Twait et al., 2023). In contrast, most studies assessing the association between WMH and tau biomarkers have failed to demonstrate any positive associations at any clinical stage (Osborn et al., 2018; Wei et al., 2019; Walsh et al., 2020; van Waalwijk van Doorn et al., 2021; Garnier-Crussard et al., 2023; Ortner et al., 2023), while only some studies on imaging pathology have offered positive results (Erten-Lyons et al., 2013; McAleese et al., 2015). It should further be emphasized that age effects may account for some of these cross-sectional associations (Luo et al., 2023). Further evaluation of these interactions is required to understand the role of WMH in the internal pathology and extrinsic phenotype of AD.
The connection between WMH, cognitive decline, and dementia has been well established in several large meta-analyses (Debette and Markus, 2010; Debette et al., 2019; Hu et al., 2021). Further, cross-sectional studies have suggested that WMH are more prevalent in patients with dementia (Yoshita et al., 2006; Gordon et al., 2015), and are associated with cognitive function across all major domains (van den Berg et al., 2018). However, previous studies have predominantly used clinical criteria for AD diagnosis without biomarkers, which can lead to misdiagnosis. The clinical phenotype involves a concentrated expression of intrinsic pathological changes (Li et al., 2022b). Thus, another caveat is that determining the contribution of WMH to cognitive dysfunction may be confounded by the core features of AD. Concurrent cerebrovascular lesions lower the threshold for the symptomatic manifestations of AD (Cortes-Canteli and Iadecola, 2020; Rost et al., 2022); however, whether the effects of WMH and AD pathologies on cognition are additive or synergistic remains unclear.
Herein, based on a large sample of well-characterized participants, we aimed to (1) explore the relationships between WMH and AD cerebrospinal fluid (CSF) core biomarkers along the cognitive continuum; (2) clarify the association between WMH and cognitive function among non-dementia participants who have the greatest therapeutic potential (Long and Holtzman, 2019; Zheng and Wang, 2024); (3) reveal the interactions among AD pathologies, WMH, and cognition; and (4) evaluate the relationship between WMH and neurodegeneration.
Data used in the preparation of this article were obtained from the Alzheimer’s Disease Neuroimaging Initiative (ADNI) database (adni.loni.usc.edu). The ADNI was launched in 2003 as a public-private partnership, led by Principal Investigator Michael W. Weiner, MD. The primary goal of ADNI has been to test whether serial MRI, positron emission tomography (PET), other biological markers, and clinical and neuropsychological assessment can be combined to measure the progression of MCI and early AD. For up-to-date information, see http://www.adni-info.org.
As of May 2023, this study enrolled 1803 individuals with available baseline WMH volume data. The ADNI database classified these individuals clinically as NCs (n = 756; Mini-Mental State Examination [MMSE] score: ≥ 24, Clinical Dementia Rating Scale [CDR] score: 0), patients with MCI (n = 783; MMSE score: ≥ 24, CDR score: 0.5, and objective memory loss measured using education-adjusted scores on delayed recall of logical memory), or patients with AD dementia (n = 264) following predefined criteria (McKhann et al., 1984). Among them, 1,182 individuals had available CSF data. On the other hand, 1,056 of the above 1,539 non-dementia participants (68.6%) had complete demographic information and underwent one or more clinical follow-ups and WMH reexaminations within the next 48 months. Among this cohort, CSF data were available for 876 individuals. Figure 1 illustrates the screening process, and the clinical information of the enrolled participants is presented in Supplementary Tables S1–S5 and Table 1.
Figure 1. The screening flowchart of participants in this study. ADNI, Alzheimer’s Disease Neuroimaging Initiative; CSF, cerebrospinal fluid; MEM, memory sub-domain; EF, executive function sub-domain; WMH, white matter hyperintensity.
The included participants provided detailed clinical information, including demographic data, apolipoprotein E (APOE) status, history of chronic diseases, smoking history, and neuropsychological test results. The tests included the CDR, MMSE, Montreal Cognitive Assessment, 13-Item Alzheimer’s Disease Assessment Scale-Cognitive Subscale, and Logical Memory test. Composite scores for memory (MEM), executive function (EF), language, and visuospatial function derived from the ADNI were also obtained (Crane et al., 2012). The corresponding tables illustrate the available data.
AD core biomarkers, including the levels of CSF Aβ42, p-tau (phosphorylated at threonine 181), and t-tau, were measured using fully automated Roche Elecsys® immunoassays, as described previously (Bittner et al., 2016). The enrolled participants were classified as having high brain Aβ loads (A+) or fibrillar tau (T+), according to a priori principles that utilized established cutoff values of <977 pg./mL for CSF Aβ42 and > 27 pg./mL for p-tau (Li et al., 2022a; Zhang et al., 2022). Growth-associated protein 43 (GAP43), a presynaptic protein indicative of nerve damage, was assayed using an in-house enzyme-linked immunoassay method that has been previously described in detail (Zhang et al., 2022).
Details regarding CSF collection and detection are available at http://adni.loni.usc.edu/.
WMH measurement was performed in the DeCarli Lab (UC-Davis), using a Bayesian approach for the segmentation of high-resolution 3D T1 and FLAIR sequences (Carmichael et al., 2012). In brief, images were processed to (1) exclude non-brain tissues, (2) spatially align, and (3) remove MRI field artifacts. The images were then warped to a standard template space in which the prior probability of WMH occurrence and the FLAIR signal characteristics of WMH were modeled at every location in the cerebral white matter. This prior information, together with the signal intensities of the FLAIR images in question, was used to identify WMH. To obtain structural indicators, including hippocampal volume, cerebral gray matter volume, and thickness of the entorhinal cortex, a region-of-interest (ROI)-based analysis was performed using FreeSurfer.1 The sum of the bilateral hippocampal volumes and the average bilateral thickness were used for subsequent analyses. For [18F]fluoro-2-deoxyglucose (18F-FDG) PET, the metaROI standardized uptake value ratio (SUVR) was calculated as representative of global glucose metabolism. The meta-ROI results from a set of predefined ROIs based on coordinates frequently cited in other AD-related FDG studies were used (Landau et al., 2011).
Details regarding imaging acquisition protocols and processing are also available online.2
Basic participant characteristics are summarized as numbers (%) or means ± standard deviations for categorical and continuous variables, respectively. Chi-square tests were used for categorical variables, and one-way ANOVA followed by Tukey’s tests (or independent two-sample t-tests) were used for continuous variables. Notably, the WMH values were normalized by their respective total intracranial volumes (TIVs), and subsequently natural log-transformed to achieve a normal distribution because the values were skewed (Shapiro–Wilk test, p < 0.05). The natural log-transformed TIV-normalized WMH volume variable was used in subsequent analyses.
With reference to previous methods (Burt et al., 2019; Mattsson et al., 2019), we applied a linear mixed-effects model to assess how WMH volume changed over time across the different groups. Fixed effects included age, sex, educational level, and APOE ε4 status, TIV, baseline WMH volume, and a predefined group × time interaction, which, if statistically significant, indicated a difference of WMH change over time. The random effects included time, in which both the intercept and slope could vary. On the other hand, the change slope of WMH volume (ΔWMH), ADNI_MEM (ΔMemory), ADNI_EF (ΔEF), typical neurodegeneration markers such as hippocampal volume were estimated for subsequent analysis with the fixed effect of time. Spearman correlation analyses were further applied to evaluate the correlation between WMH volume and other indices, such as scores on typical neuropsychological scales, and the results were presented in the form of a heatmap. Furthermore, linear regression models were constructed to explore the factors associated with WMH volume, and to evaluate the relationships between WMH (or ΔWMH) and cognition or neurodegeneration. The covariates are displayed in the corresponding tables.
The predictive effectiveness of baseline WMH volume was verified by using Cox-proportional hazard regression models. The analyses included only NCs and MCI participants, with follow-up up to 48 months. The outcome of the model was time to dementia change. For each individual, time “0” was defined as the date of the baseline WMH assessment. For converters, the survival time was defined as the time from the baseline assessment to the diagnosis of dementia. The proportional hazards assumption was tested using the scaled Schoenfeld residuals.
Finally, mediation effect analysis was applied to explore the interrelationship among Aβ deposition, cognition, and WMH. The mediation was interpreted based on the estimated average direct effect (ADE), average causal mediation effect (ACME) and average total effect (ATE). The ADE represents the effect of the Aβ deposition on the outcome measure, and the ACME represents the effect of the Aβ deposition on the outcome through the mediator variable (WMH volume). The ATE is a summarisation of ADE and ACME. We calculated bias-corrected 95% confidence intervals for the size of the mediating effects using bootstrapping (k = 500 samples).
The significance threshold was set at p < 0.05 (corrected for multiple comparisons using Tukey’s test). The above-mentioned analyses were performed using GraphPad Prism (version 9.4.0) or R programming language (version 4.2.1; package “lmerTest,” “survival,” “mediation,” etc.).
A total of 1803 participants, including 756 NCs, 783 patients with MCI, and 264 patients with dementia, were enrolled. As shown in Supplementary Table S1, the dementia group had a higher baseline age than the other two groups (75.4 ± 8.21 vs. 72.0 ± 7.25 [NC] and 72.4 ± 7.85 [MCI], both p < 0.001). Further, the NC group had a lower proportion of men and a slightly higher educational level than the other two groups (men: 41.3% vs. 55.1% [MCI] and 57.4% [dementia], both p < 0.001; education: 16.6 ± 2.42 vs. 16.2 ± 2.60 [MCI] and 15.6 ± 2.74 [dementia], p < 0.01 and 0.001, respectively). However, there were no differences in the frequency of chronic diseases such as hypertension and diabetes among the three groups, while patients with cognitive impairment were more likely to smoke (31.2% [MCI] and 32.3% [dementia] vs. 24.1% [NC], both p < 0.01). As expected, the MCI and dementia groups both showed worse performance scores in the neuropsychological tests and higher proportions of APOE ε4 carriers than the NC group (all p < 0.001); the AD CSF core biomarkers were also more altered in these two groups than in the NC group (all p < 0.001). Additionally, glucose metabolism, represented by the metaROI FDG SUVR, as well as several typical morphological parameters, including hippocampal volume, cerebral gray matter volume, and thickness of the entorhinal cortex, all gradually changed across the cognitive continuum (all p < 0.001).
A subset of the above cohort (n = 1,182) was further grouped by AD CSF core features. Details regarding group characteristics are presented in Supplementary Table S2 (by “A” status), Supplementary Table S3 (by “T” status), and Supplementary Table S4 (by both “A/T” profiles). Another subset included 1,056 non-dementia participants with complete demographic and follow-up information; of these, Supplementary Table S5 shows group characteristics and the numbers of participants with follow-up WMH/ADNI_MEM/ADNI_EF data within the next 48 months. Table 1 presents the clinical information of 876 individuals with CSF data, including 391 NCs and 485 patients with MCI. The differences between the two groups were consistent with those described above.
As shown in Supplementary Table S1 and Figure 2A, WMH volume gradually increased across the cognitive continuum, with the lowest in the NC group, an increase in the MCI group (p < 0.001), and reaching the highest in the dementia group (vs. NC or MCI, both p < 0.001), suggesting that WMH volume may increase in relation to cognitive status. Correlation matrices displayed the Spearman correlation coefficients between WMH volume and scores on typical neuropsychology scales (Supplementary Figure S1; |R| = 0.151–0.332, p < 0.001), reinforcing the above suggestion. Next, the groups were further subdivided to explore the differences under different “A/T” profiles (Jack et al., 2018). After stratification of the clinically diagnosed groups additionally by Aβ status, the WMH volume was relatively higher in the A+ groups than in the corresponding A- groups (all p < 0.05; Supplementary Table S2 and Figure 2B). After stratification by p-tau status, no differences were observed between the paired groups (all p > 0.05; Supplementary Table S3 and Figure 2C). Following additional stratification by both Aβ and p-tau statuses, we observed that the transition of the p-tau condition did not influence the WMH volume under the same cognitive and Aβ statuses (all p > 0.05). In contrast, the A+ condition led to an increase in WMH volume in the T- NC groups (A− vs. A+, p < 0.05), T- MCI groups (A− vs. A+, p < 0.01), and T- dementia groups (A- vs. A+, p < 0.05). Aβ did not affect WMH in the T+ groups (A−T+ NC/MCI/dementia vs. corresponding A + T+ NC/MCI/dementia, all p > 0.05). The details are presented in Supplementary Table S4 and Figure 2D.
Figure 2. WMH volume in different diagnostic groups. (A) Participants were grouped by clinical diagnosis (n = 1803; see Supplementary Table S1). (B) Participants were grouped by clinical diagnosis and CSF-determined Aβ status (n = 1,182; see Supplementary Table S2). (C) Participants were grouped by clinical diagnosis and CSF-determined p-tau status (n = 1,182; see Supplementary Table S3). (D) Participants were grouped by clinical diagnosis and CSF-determined Aβ and p-tau statuses (n = 1,182; see Supplementary Table S4). According to a previous standard, we set the cutoff value at 977 pg./mL for Aβ and 27 pg./mL for p-tau to select participants with Aβ deposition (< 977 pg./mL; A+) and fibrillar tau (> 27 pg./mL; T+). The WMH volume was total intracranial volume-normalized and log-transformed (Supplementary Figure S2 shows the distribution of original WMH values and log-transformed WMH values). The results are presented in improved box charts; the red lines indicate the median values; the upper straight lines indicate the upper quartiles and the lower straight lines indicate the lower quartiles. Statistical analysis was conducted using one-way ANOVA followed by Tukey’s test (adjusted p value). Comparisons among groups: *, < 0.05; **, < 0.01; ***, < 0.001; #, > 0.05. WMH, white matter hyperintensity; NC, cognitively normal control; MCI, mild cognitive impairment; CSF, cerebrospinal fluid; Aβ, β-amyloid; p-tau, phosphorylated tau; ANOVA, analysis of variance.
In addition to the cognitive status and Aβ levels, the above results indicated that there may be other factors influencing WMH volume. The regression analysis results further suggested that age (β: 0.029, p < 0.001), education level (β: −0.013, p = 0.038), hypertension (β: 0.120, p < 0.001), smoking history (β: 0.104, p = 0.001), cognitive impairment (β: 0.127 for MCI, p < 0.001; β: 0.136 for dementia, p = 0.008), and CSF Aβ42 levels (β: −0.0002, p < 0.001) were related to WMH. Meanwhile, sex, APOE ε4 status, CSF p-tau levels, and other chronic diseases (diabetes, atrial fibrillation, hyperlipidemia, cardiovascular and cerebrovascular diseases) were not associated with WMH (all p > 0.05; Table 2). In the subgroup analyses, age and CSF Aβ42 levels were still significant, while hypertension and smoking history were only significant in the non-dementia groups (Supplementary Table S6).
As shown in Table 3, higher WMH volume was associated with lower scores of ADNI_MEM (p = 0.040) and ADNI_EF (p = 0.002). The association was also significant for AD CSF core features. Furthermore, we found there were no interactive effects between WMH and CSF Aβ42 on both ADNI_MEM and ADNI_EF (p = 0.510 and 0.336, respectively; data not shown). When this interaction term was additionally included as a covariate, WMH was only significantly associated with ADNI_EF (p = 0.014), not ADNI_MEM (p = 0.129).
Among the 876 participants summarized in Table 1, 119 (13.6%) developed dementia within the next 48 months, while the others developed MCI (4.3%), returned to a healthy status (4.8%), or remained stable (77.3%). Cox regression indicated that baseline WMH volume was not a risk factor for future dementia conversion (p = 0.840), unlike CSF Aβ42 or p-tau (both p < 0.001) (Supplementary Table S7). Schoenfeld residuals were computed to test proportional hazard assumption (Supplementary Table S8). However, linear regression analysis revealed that a higher WMH volume at baseline was associated with decreased levels in ΔMemory (Figure 3A and Supplementary Table S9), indicating that a larger baseline WMH volume was associated with a faster cognitive decline. Specifically, the effect of WMH on ΔMemory was not affected by demographic information, APOE ε4 status, baseline cognitive status, or previous disease status including hypertension and smoking (p < 0.001 and 0.010 for model 1 and model 2, respectively). In model 3, the CSF Aβ42 and p-tau levels were additionally included as covariates and the results were not altered (p < 0.05). In model 4, we observed no interactive effects between baseline WMH volume and CSF Aβ42 levels on ΔMemory (p = 0.611), while the WMH trended towards a significant increase the likelihood of lower ΔMemory (p = 0.105). In contrast, the WMH volume was not associated with ΔEF (Figure 3B and Supplementary Table S9). The association between AD CSF core features, especially CSF p-tau, and Δcognition was always significant.
Figure 3. Forest plot of the association between baseline WMH volume and cognitive function slopes. (A) The association between baseline WMH volume and ΔMemory. (B) The association between baseline WMH volume and ΔEF. The cognitive function slopes were calculated by using linear mixed-effects models among non-dementia participants with at least one follow-up ADNI_MEM/EF score within the next 48 months (n = 1,056, see Supplementary Table S5). In model 1, baseline WMH volume, plus age, sex, education, APOE ε4 status, and cognitive status were used as predictors of cognitive function slopes. In model 2, hypertension and smoking status were used as additional predictors on the basis of model 1. In model 3, CSF core biomarkers, including Aβ42 and p-tau levels, were used as additional predictors on the basis of model 2. In model 4, the interaction term of CSF Aβ42 and WMH were used as additional predictors on the basis of model 3. The WMH volume was total intracranial volume-normalized and log-transformed. CSF t-tau was not included due to its extremely high correlation with p-tau (R > 0.900, p < 0.001). For effect estimates with exact 95% CI and statistical significance values, see Supplementary Table S9. MCI, mild cognitive impairment; WMH, white matter hyperintensity; CSF, cerebrospinal fluid; Aβ, β-amyloid; p-tau, phosphorylated tau; t-tau, total tau; APOE, apolipoprotein E; MEM, memory sub-domain; EF, executive function; ADNI, Alzheimer’s Disease Neuroimaging Initiative; CI, confidence interval.
Some participants had longitudinal WMH data at the 12th, 24th, 36th, and 48th months (Supplementary Tables S1, S5). The data distribution and changes in WMH volume are displayed in Figure 4. Group × time interactions were significant in participants with dementia (vs. NC or MCI, p < 0.05) and in participants with A+ and non-dementia profiles (A+ NC vs. A− NC, p < 0.05; A+ MCI vs. A− MCI, p < 0.001). Compared to their respective controls, the WMH volume of these participants increased faster over time (Figure 4; Supplementary Tables S10, S11). However, there were no differences of group × time interactions between the A+ dementia and A-dementia groups, or between the T+ groups and the corresponding T- groups (all p > 0.05; data not shown).
Figure 4. Data distribution and change in WMH volume within the next 48 months. (A–D) Data distribution of WMH volume (left column) and estimated means of WMH volume (right column) in different diagnostic groups. Raw values above 50/40/30/30 cm3 were discarded in A/B/C/D (left column) for the sake of aesthetics, with n = 24/15/55/13, respectively. Due to limited numbers, the follow-up WMH values cannot be predicted in the dementia group at the 36th and 48th month. The horizontal line in the middle of each box (left column) indicates the median, the top and bottom borders of the box mark the 75th and 25th percentiles. The modeled data (right column) show the mean 95% CIs for the predicted values using a linear mixed-effects model adjusted for age, sex, educational level, APOE ε4 status, total intracranial volume, and baseline WMH volume. p values indicate the group × time interaction effect. For effect estimates and statistical significance values, see Supplementary Tables S10, S11. NC, cognitively normal control; MCI, mild cognitive impairment; WMH, white matter hyperintensity; CSF, cerebrospinal fluid; Aβ, β-amyloid; APOE, apolipoprotein E.
Next, we explored the relationship between ΔWMH and Δcognition. As shown in Figure 5 and Supplementary Table S12, a higher ΔWMH was associated with decreased levels of ΔMemory and ΔEF (p < 0.001 and p = 0.001, respectively), indicating that a faster baseline WMH volume increase was associated with a more rapid cognitive decline. This association was not influenced by demographic information, APOE ε4 status, baseline cognitive status, or previous disease status including hypertension and smoking, and AD CSF core features.
Figure 5. Forest plot of the association between ΔWMH volume and cognitive function slopes. The slopes (ΔWMH, ΔMemory and ΔEF) were calculated by using linear mixed-effects models among non-dementia participants with at least one follow-up WMH data, ADNI_MEM/EF score within the next 48 months (n = 1,056, see Supplementary Table S5). The ΔWMH volumes, plus diagnostic status, age, sex, education, APOE ε4 status, hypertension and smoking status, and CSF core biomarkers, including Aβ42 and p-tau levels were used as predictors of cognitive function slopes (n = 876, see Table 1). CSF t-tau was not included due to its extremely high correlation with p-tau (R > 0.900, p < 0.001). For effect estimates with exact 95% CI and statistical significance values, see Supplementary Table S12. MCI, mild cognitive impairment; WMH, white matter hyperintensity; CSF, cerebrospinal fluid; Aβ, β-amyloid; p-tau, phosphorylated tau; t-tau, total tau; APOE, apolipoprotein E; MEM, memory sub-domain; EF, executive function; ADNI, Alzheimer’s Disease Neuroimaging Initiative; CI, confidence interval.
Considering the close relationship between CSF Aβ42 and WMH, as well as their impacts on cognitive function, we wondered whether WMH may mediate the relationship between brain Aβ deposition and cognition. Subsequent mediation analyses revealed both direct (i.e., ADE), WMH-mediated (i.e., ACME) and total effects of brain Aβ deposition on baseline cognitive performance (Figure 6). However, although significant, the mediation effects accounted for only 10.50% (p = 0.004) and 14.13% (p < 0.001) of the total effects in the MEM and EF cognitive domains, respectively. Further, we found that WMH also exerted significant mediating effects on the correlation between brain Aβ deposition and ΔMemory (proportion: 7.09%, p < 0.05), but not on ΔEF (p > 0.05; Supplementary Figure S3).
Figure 6. Mediation analyses of brain Aβ deposition on cognitive function. (A) The interrelationship among Aβ deposition, ADNI_MEM, and WMH. (B) The interrelationship among Aβ deposition, ADNI_EF, and WMH. Baseline WMH volume was used as the mediating variable; age, sex, educational level, and APOE ε4 status, were used as the covariates; the CSF Aβ levels were used as independent variable, and the ADNI_MEM or ADNI_EF score was used as dependent variable. All paths are presented in standardized regression coefficients (β). The WMH volume was total intracranial volume-normalized and log-transformed. The analysis was performed in 876 participants in Table 1. WMH, white matter hyperintensity; CSF, cerebrospinal fluid; Aβ, β-amyloid; APOE, apolipoprotein E; MEM, memory sub-domain; EF, executive function; ADNI, Alzheimer’s Disease Neuroimaging Initiative; ACME, average causal mediation effect; ADE, average direct effect; ATE, average total effect.
CSF GAP43 and t-tau, FDG SUVR, and structural indicators, including hippocampal volume, cerebral gray matter volume, and entorhinal cortex thickness, are commonly considered as markers of neurodegeneration, reflecting nerve damage from different perspectives (Jack et al., 2018; Zhang et al., 2022). Herein, we explored their relationship with WMH. At the cross-sectional level, the results indicated that baseline WMH volume had a weak but significant correlation with these markers, except for CSF GAP43 (Supplementary Figure S4; |R| = 0.078–0.295; all p < 0.05). Longitudinal analysis further showed that WMH was only correlated with the slope of hippocampal volume and entorhinal cortex thickness (Supplementary Figure S4; R = −0.067 and − 0.228, respectively; p = 0.030 and p < 0.001, respectively). The correlation between CSF AD core features and these indicators was generally more prominent. Further linear regression was corrected for the influence of confounding factors, and the results indicated that WMH could still independently related to the thickness of the entorhinal cortex (baseline level, p = 0.016; change slope, approaching significance, p = 0.096; see Figure 7 and Supplementary Table S13 for details), but not other indicators (data not shown). Similar results were obtained in individuals without dementia (Supplementary Figure S4).
Figure 7. Forest plot of the association between WMH volume and entorhinal cortex thickness. (A) The association between baseline WMH volume and entorhinal cortex thickness. (B) The association between baseline WMH volume and the changes slope of entorhinal cortex thickness. Baseline WMH volume, plus diagnostic status, age, sex, education, APOE ε4 status, hypertension and smoking status, and CSF core biomarkers, including Aβ42 and p-tau levels were used as predictors of cognitive function slopes. The WMH volume was total intracranial volume-normalized and log-transformed. CSF t-tau was not included due to its extremely high correlation with p-tau (R > 0.900, p < 0.001). For effect estimates with exact 95% CI and statistical significance values, see Supplementary Table S13. MCI, mild cognitive impairment; WMH, white matter hyperintensity; CSF, cerebrospinal fluid; Aβ, β-amyloid; p-tau, phosphorylated tau; t-tau, total tau; APOE, apolipoprotein E; MEM, memory sub-domain; EF, executive function; ADNI, Alzheimer’s Disease Neuroimaging Initiative; CI, confidence interval.
In this study, we used data from a large cohort of participants along the cognitive continuum, to investigate WMH in detail. Overall, our analysis revealed the following results: (i) age and CSF Aβ42 levels were the most important factors related to WMH volume, and these results remained largely consistent across all clinical stages, although hypertension and smoking history were only associated with WMH in non-dementia participants; (ii) higher WMH volume at baseline was associated with worse cognitive performance and a more rapid memory decline, however, baseline WMH volume cannot predict future dementia conversion; (iii) participants with dementia, or non-dementia participants harboring cerebral Aβ both exhibited a greater accrual of WMH volume, and a greater increase in WMH volume was found to be linked with a more rapid cognitive decline; (iv) to a lesser extent, the correlation between cerebral Aβ and cognition was affected by the mediating effects of WMH; (v) among different neurodegeneration markers, the WMH was only independently and significantly associated with the thickness of entorhinal cortex.
WMH are generally considered to be of vascular origin, and have been related to blood–brain barrier abnormalities and neuroinflammation (Garnier-Crussard et al., 2023; Ying et al., 2023). Previous studies have confirmed the correlation between WMH and age (Yoshita et al., 2006; Wei et al., 2019; Walsh et al., 2020; Luo et al., 2023; Ortner et al., 2023), as well as overall vascular risk factors (Yoshita et al., 2006; Cox et al., 2019; Alban et al., 2023). In several significantly large cohorts, researchers have indicated that arterial hypertension is associated with a larger WMH volume and accelerated WMH progression (Goldstein et al., 2005; Gottesman et al., 2010; Verhaaren et al., 2013; Bernal et al., 2023). The association between smoking and elevated WMH has also been confirmed in a large study of the UK Biobank samples (Gray et al., 2020). However, it should be noted that these studies mainly focused on participants free of dementia. This study did not identify any link between WMH and hypertension or smoking in the dementia group, emphasizing the importance of early intervention. Smoking and blood pressure may exert their effects through endothelial damage, oxidative stress and inflammatory mechanisms, and may have potential for dementia prevention due to their modifiable nature (Silva et al., 2022). Although diabetes, atrial fibrillation, and hyperlipidemia are all potential risk factors for atherosclerosis and brain infarction, the present study did not identify any relationship with WMH. In fact, several prior studies have shown no or only a weak association between diabetes and WMH (Prins and Scheltens, 2015; Moroni et al., 2020). Regarding hyperlipidemia, both positive and negative correlations have been observed with WMH (Moroni et al., 2020). Stroke may promote the occurrence of WMH (Loos et al., 2018; Duering et al., 2023), but this study did not find any association between cardiovascular and cerebrovascular diseases and WMH. From our perspective, these inconsistent results may be related to the study design and cohort differences. For example, people with stroke and other cardiovascular diseases were generally excluded from ADNI and thus it has less cardio- and cerebrovascular disease burden than the general older adults. Further research is still needed.
Cortical amyloid deposition is another key contributor to the development of WMH. Despite contradictions by some studies (Hedden et al., 2012; Marchant et al., 2013; Park et al., 2014; Luo et al., 2023; Ortner et al., 2023; Ying et al., 2023), we believe that our results were reliable because the correlations between CSF Aβ42 levels and WMH volume were not affected by confounding factors, and were significant at all clinical stages, suggesting that WMH could also be AD origin and another core feature of AD (Iturria-Medina et al., 2016; Lee et al., 2016). Recent studies have further found that the cerebral Aβ burden was associated with WMH located in specific posterior regions, indicating a region-specific effect (Weaver et al., 2019; Bernal et al., 2023). Furthermore, a potentially self-reinforcing mechanism has been proposed, indicating that vascular pathologies may initiate Aβ deposition on the one hand, while on the other hand, Aβ deposition may also increase the susceptibility to developing vascular lesions (Klohs, 2019). Specifically, vascular dysfunction could promote oxidative stress and neuroinflammation, thereby stimulating the increased production and limiting the clearance of Aβ (Rost et al., 2022). To some extent, this effect can be achieved through platelet activation (Li and Liu, 2022). Conversely, due to the toxic effects on pericytes and endothelial cells, elevations in Aβ have been shown to cause vasoconstriction, reduce cerebral perfusion, and prevent functional hyperemia and autoregulation, finally resulting in lesions including WMH (Love and Miners, 2016; Cortes-Canteli and Iadecola, 2020).
There is significant evidence to show that cerebrovascular disease accelerates the onset of dementia in AD patients (Snowdon et al., 1997; Cortes-Canteli and Iadecola, 2020; Rost et al., 2022). Individuals with mixed findings of vascular and AD pathologies are almost three times more likely to develop dementia than those with a single pathological diagnosis (Schneider et al., 2007). This study found that higher WMH volume at baseline was associated with worse cognitive performance and a faster memory decline. However, the Cox regression results suggested that WMH volume cannot predict future dementia conversion, which was inconsistent with previous studies (Debette and Markus, 2010; Gordon et al., 2015; Hu et al., 2021). Our negative findings may be related to the short follow-up time. Furthermore, we made longitudinal analyses of WMH volume, which showed that baseline features of AD (including dementia diagnosis and positive Aβ status) were associated with future increase in WMH volume, and that longitudinal WMH volume were correlated with further change in cognition. Specifically, faster WMH volume aggregation reflects a more rapid deterioration of future cognition. These results suggest that longitudinal WMH volume can be used to dynamically track neurodegeneration throughout the preclinical stage and different clinical stages of AD. Co-pathologies lead to uncertainty regarding the role of WMH. As such, we corrected for confounding factors including CSF Aβ and p-tau. After these analyses, the relationships between WMH/ΔWMH and cognition was still significant, indicating that the long-term effects occurred independent of AD pathologies. In contrast, previous studies either did not consider confounding factors, or corrected only for demographic information (Debette and Markus, 2010; Hu et al., 2021). Our recent review suggested that Aβ could damage cognitive function independent of tau pathology and neurodegeneration; however, the specific mechanism remains unclear (Li et al., 2022b). This study found that WMH mediates the correlation between brain Aβ deposition and cognition. It should be noted that although these mediating effects were statistically significant, the degree was very weak, indicating that Aβ acts primarily through other mechanisms (Jack et al., 2018). Recent imaging studies have indicated that WMH may cause cognitive impairment by interrupting the connection between the cortex and subcortical nucleus (Zhu et al., 2023). In addition, WMH-associated microglial activation plays a role in the development of AD (Prins and Scheltens, 2015; Moroni et al., 2020).
How WMH relate to different markers of neurodegeneration is currently only poorly understood, as previous studies either did not pay much attention to the potential interactions of AD core pathologies, or focused on only a single marker instead of investigating multiple neurodegenerative markers using various modalities. Herein, we analyzed markers from the CSF, as well as those extracted from PET and structural MRI. Overall, our results showed that the correlations between markers and WMH were affected by Aβ or tau pathologies to a certain extent, and WMH was only independently associated with the thickness of entorhinal cortex. Previously, Bos et al. found that WMH and Aβ are independent determinants of medial temporal lobe atrophy and that their effects are additive (Bos et al., 2017). However, the authors regarded both WMH and Aβ as binary variables, rather than continuous variables, and estimated atrophy subjectively rather than using objective data, which may have resulted in a poor robustness. Similar to our results, Kurz et al. found that elevated t-tau levels in patients with vascular damage could occur as a result of coexisting Aβ pathology (Kurz et al., 2003). In one small sample, researchers also found no correlation between WMH volume and the FDG SUVR (Ortner et al., 2023). The correlation mechanism between WMH and cortical thickness needs further exploration.
This study had several limitations. First, we defined previous chronic diseases and smoking history based on a retrospective screening of medical records, which could have missed newly diagnosed patients, and we did not consider the severity and control of disease (or smoking). Secondly, considering its rarity, we did not screen for mutations related to CSVD. Third, this study focused on the total volume of WMH, rather than its pattern (punctuated or confluent) or location (deep or periventricular), although some prior studies have shown that WMH from different sources may have distinct patterns (Keller et al., 2023) or locations (Weaver et al., 2019). Fourth, based on the ADNI’s inclusion/exclusion criteria, participants were more likely to identify their race as White, highly educated, and APOE ε4 positive, and had relatively low vascular risk burdens (Gianattasio et al., 2021). The impact of race on the burden of AD pathologies may be severely underestimated (Royse et al., 2021). Thus, findings from our study may not be assumed to be directly generalizable to other populations. Future research should therefore include participants with greater racial diversity and educational attainments. Fifth, loss to follow-up remains a concern in longitudinal study, which may have introduced selection bias. Sixth, longitudinal mediation studies are warranted to underpin the relations among variables over time and establish causality. Seventh, WMH’s mechanism of action of WMHs was not investigated. Finally, compared with subjective ratings (Gordon et al., 2015; Marnane et al., 2016; van Westen et al., 2016), this study used an automated quantitative method to calculate WMH volume; as such, it should be noted that different evaluation methods may lead to different results.
WMH are non-specific lesions associated with both vascular and AD factors. Increased baseline volume and accruals were both found to be associated with cognitive decline. Importantly, we found that their impacts on cognition were independent of Aβ, and these two pathologies are not simply additive. WMH mediates the correlation between Aβ and cognition, and there is likely to be a mutually reinforcing relationship (Klohs, 2019; Cortes-Canteli and Iadecola, 2020; Rost et al., 2022). For neurodegeneration, we confirmed that WMH volume was only associated with the thickness of entorhinal cortex, not other markers. Owing to the particular impact of WMH on cognition, the present study highlights the value of incorporating vascular pathologies into AD biomarkers. Both vascular and AD pathologies should be considered when developing therapeutic targets. Given the limited treatment options for dementia, early intervention to reduce vascular lesions is a high priority.
Publicly available datasets were analyzed in this study. This data can be found here: http://adni.loni.usc.edu/. Please note that access is contingent on adherence to the ADNI Data Use Agreement and the publications’ policies.
The studies involving humans were approved by Alzheimer’s Disease Neuroimaging Initiative. The studies were conducted in accordance with the local legislation and institutional requirements. The participants provided their written informed consent to participate in this study.
T-RL: Conceptualization, Data curation, Formal analysis, Methodology, Resources, Supervision, Writing – original draft, Writing – review & editing. B-LL: Methodology, Writing – review & editing. X-RX: Writing – review & editing, Methodology. JZ: Methodology, Writing – review & editing. T-SW: Writing – review & editing. F-QL: Conceptualization, Funding acquisition, Methodology, Project administration, Resources, Supervision, Writing – review & editing.
Data used in the preparation of this article were obtained from the Alzheimer’s Disease Neuroimaging Initiative (ADNI) database (http://adni.loni.usc.edu). As such, the investigators within the ADNI contributed to the design and implementation of ADNI and/or provided data but did not participate in analysis or writing of this report. A complete listing of ADNI investigators can be found at: http://adni.loni.usc.edu/wp-content/uploads/how_to_apply/ADNI_Acknowledgement_List.pdf.
The author(s) declare that financial support was received for the research, authorship, and/or publication of this article. This work was supported by grants “82300149” from the National Natural Science Foundation of China, grants "2023M741462" from the China Postdoctoral Science Foundation, grants "MXJL202211" from the First Affiliated Hospital of Nanjing Medical University, grants "PY2023018" from the Young Scholars Fostering Fund of the First Affiliated Hospital of Nanjing Medical University, and grants "2023ZB308" and "2023ZB182" from the Department of Human Resources and Social Security of Jiangsu Province.
Data collection and sharing for this project was funded by the Alzheimer’s Disease Neuroimaging Initiative (ADNI) (National Institutes of Health Grant U01AG024904) and DOD ADNI (Department of Defense award number W81XWH-12-2-0012). ADNI is funded by the National Institute on Aging, the National Institute of Biomedical Imaging and Bioengineering, and through generous contributions from the following: AbbVie, Alzheimer’s Association; Alzheimer’s Drug Discovery Foundation; Araclon Biotech; BioClinica, Inc.; Biogen; Bristol- Myers Squibb Company; CereSpir, Inc.; Cogstate; Eisai Inc.; Elan Pharmaceuticals, Inc.; Eli Lilly and Company; EuroImmun; F. Hoffmann-La Roche Ltd. and its affiliated company Genentech, Inc.; Fujirebio; GE Healthcare; IXICO Ltd.; Janssen Alzheimer Immunotherapy Research & Development, LLC.; Johnson & Johnson Pharmaceutical Research & Development LLC.; Lumosity; Lundbeck; Merck & Co., Inc.; Meso Scale Diagnostics, LLC.; NeuroRx Research; Neurotrack Technologies; Novartis Pharmaceuticals Corporation; Pfizer Inc.; Piramal Imaging; Servier; Takeda Pharmaceutical Company; and Transition Therapeutics. The Canadian Institutes of Health Research is providing funds to support ADNI clinical sites in Canada. Private sector contributions are facilitated by the Foundation for the National Institutes of Health (http://www.fnih.org). The grantee organization is the Northern California Institute for Research and Education, and the study is coordinated by the Alzheimer’s Therapeutic Research Institute at the University of Southern California. ADNI data are disseminated by the Laboratory for Neuro Imaging at the University of Southern California. The authors wish to acknowledge the group of Professor Shao-Wen Tang (Department of epidemiology, School of Public Health, Nanjing Medical University) for their assistance in statistical methods for this study.
The authors declare that the research was conducted in the absence of any commercial or financial relationships that could be construed as a potential conflict of interest.
All claims expressed in this article are solely those of the authors and do not necessarily represent those of their affiliated organizations, or those of the publisher, the editors and the reviewers. Any product that may be evaluated in this article, or claim that may be made by its manufacturer, is not guaranteed or endorsed by the publisher.
The Supplementary material for this article can be found online at: https://www.frontiersin.org/articles/10.3389/fnagi.2024.1412735/full#supplementary-material
Alban, S. L., Lynch, K. M., Ringman, J. M., Toga, A. W., Chui, H. C., Sepehrband, F., et al. (2023). The association between white matter Hyperintensities and amyloid and tau deposition. Neuroimage Clin. 38:103383. doi: 10.1016/J.Nicl.2023.103383
Bernal, J., Schreiber, S., Menze, I., Ostendorf, A., Pfister, M., Geisendörfer, J., et al. (2023). Arterial hypertension and Β-amyloid accumulation have spatially overlapping effects on posterior white matter Hyperintensity volume: a cross-sectional study. Alzheimers Res. Ther. 15:97. doi: 10.1186/S13195-023-01243-4
Bittner, T., Zetterberg, H., Teunissen, C. E., Ostlund, R. E. Jr., Militello, M., Andreasson, U., et al. (2016). Technical performance of a novel, fully automated Electrochemiluminescence immunoassay for the quantitation of Β-amyloid (1-42) in human cerebrospinal fluid. Alzheimers Dement. 12, 517–526. doi: 10.1016/J.Jalz.2015.09.009
Bos, I., Verhey, F. R., Ramakers, I., Jacobs, H., Soininen, H., Freund-Levi, Y., et al. (2017). Cerebrovascular and amyloid pathology in Predementia stages: the relationship with neurodegeneration and cognitive decline. Alzheimers Res. Ther. 9:101. doi: 10.1186/S13195-017-0328-9
Burt, L. A., Billington, E. O., Rose, M. S., Raymond, D. A., and Hanley, D. A., And Boyd, S. K. (2019). Effect of high-dose vitamin D supplementation on volumetric bone density and bone strength: a randomized clinical trial. JAMA 322, 736–745. doi: 10.1001/Jama.2019.11889
Carmichael, O., Mungas, D., Beckett, L., Harvey, D., Tomaszewski Farias, S., Reed, B., et al. (2012). Mri predictors of cognitive change in a diverse and carefully characterized elderly population. Neurobiol. Aging 33, 83–95.e2. doi: 10.1016/J.Neurobiolaging.2010.01.021
Cortes-Canteli, M., And Iadecola, C. (2020). Alzheimer's Disease and vascular aging: Jacc focus seminar. J. Am. Coll. Cardiol. 75, 942–951. doi: 10.1016/J.Jacc.2019.10.062
Cox, S. R., Lyall, D. M., Ritchie, S. J., Bastin, M. E., Harris, M. A., Buchanan, C. R., et al. (2019). Associations between vascular risk factors and brain Mri indices in Uk biobank. Eur. Heart J. 40, 2290–2300. doi: 10.1093/Eurheartj/Ehz100
Crane, P. K., Carle, A., Gibbons, L. E., Insel, P., Mackin, R. S., Gross, A., et al. (2012). Development and assessment of a composite score for memory in the Alzheimer's Disease Neuroimaging Initiative (Adni). Brain Imaging Behav. 6, 502–516. doi: 10.1007/S11682-012-9186-Z
Debette, S., And Markus, H. S. (2010). The clinical importance of white matter Hyperintensities on brain magnetic resonance imaging: systematic review and Meta-analysis. BMJ 341,:C3666. doi: 10.1136/Bmj.C3666
Debette, S., Schilling, S., Duperron, M. G., and Larsson, S. C., And Markus, H. S. (2019). Clinical significance of magnetic resonance imaging markers of vascular brain injury: a systematic review and Meta-analysis. JAMA Neurol. 76, 81–94. doi: 10.1001/Jamaneurol.2018.3122
Duering, M., Biessels, G. J., Brodtmann, A., Chen, C., Cordonnier, C., De Leeuw, F. E., et al. (2023). Neuroimaging standards for research into small vessel Disease-advances since 2013. Lancet Neurol. 22, 602–618. doi: 10.1016/S1474-4422(23)00131-X
Erten-Lyons, D., Woltjer, R., Kaye, J., Mattek, N., Dodge, H. H., Green, S., et al. (2013). Neuropathologic basis of white matter Hyperintensity accumulation with advanced age. Neurology 81, 977–983. doi: 10.1212/Wnl.0b013e3182a43e45
Garnier-Crussard, A., Cotton, F., and Krolak-Salmon, P., And Chételat, G. (2023). White matter Hyperintensities in Alzheimer's Disease: beyond vascular contribution. Alzheimers Dement. doi: 10.1002/Alz.13057, 19, 3738–3748.
Gianattasio, K. Z., Bennett, E. E., Wei, J., Mehrotra, M. L., Mosley, T., Gottesman, R. F., et al. (2021). Generalizability of findings from a clinical sample to a community-based sample: a comparison of Adni and Aric. Alzheimers Dement. 17, 1265–1276. doi: 10.1002/Alz.12293
Goldstein, I. B., Bartzokis, G., and Guthrie, D., And Shapiro, D. (2005). Ambulatory blood pressure and the brain: a 5-year follow-up. Neurology 64, 1846–1852. doi: 10.1212/01.Wnl.0000164712.24389.Bb
Gordon, B. A., Najmi, S., Hsu, P., Roe, C. M., and Morris, J. C., And Benzinger, T. L. (2015). The effects of white matter Hyperintensities and amyloid deposition on Alzheimer dementia. Neuroimage Clin. 8, 246–252. doi: 10.1016/J.Nicl.2015.04.017
Gottesman, R. F., Coresh, J., Catellier, D. J., Sharrett, A. R., Rose, K. M., Coker, L. H., et al. (2010). Blood pressure and white-matter Disease progression in a Biethnic cohort: atherosclerosis risk in communities (Aric) study. Stroke 41, 3–8. doi: 10.1161/Strokeaha.109.566992
Graff-Radford, J., Arenaza-Urquijo, E. M., Knopman, D. S., Schwarz, C. G., Brown, R. D., Rabinstein, A. A., et al. (2019). White matter Hyperintensities: relationship to amyloid and tau burden. Brain 142, 2483–2491. doi: 10.1093/Brain/Awz162
Gray, J. C., Thompson, M., Bachman, C., Owens, M. M., and Murphy, M., And Palmer, R. (2020). Associations of cigarette smoking with Gray and white matter in the Uk biobank. Neuropsychopharmacology 45, 1215–1222. doi: 10.1038/S41386-020-0630-2
Grinberg, L. T., Nitrini, R., Suemoto, C. K., Lucena Ferretti-Rebustini, R. E., Leite, R. E., Farfel, J. M., et al. (2013). Prevalence of dementia subtypes in a developing country: a Clinicopathological study. Clinics 68, 1140–1145. doi: 10.6061/Clinics/2013(08)13
Hedden, T., Mormino, E. C., Amariglio, R. E., Younger, A. P., Schultz, A. P., Becker, J. A., et al. (2012). Cognitive profile of amyloid burden and white matter Hyperintensities in cognitively Normal older adults. J. Neurosci. 32, 16233–16242. doi: 10.1523/Jneurosci.2462-12.2012
Hu, H. Y., Ou, Y. N., Shen, X. N., Qu, Y., Ma, Y. H., Wang, Z. T., et al. (2021). White matter Hyperintensities and risks of cognitive impairment and dementia: a systematic review and Meta-analysis of 36 prospective studies. Neurosci. Biobehav. Rev. 120, 16–27. doi: 10.1016/J.Neubiorev.2020.11.007
Iturria-Medina, Y., Sotero, R. C., Toussaint, P. J., Mateos-Pérez, J. M., and Evans, A. C. (2016). Early role of vascular dysregulation on late-onset Alzheimer's Disease based on multifactorial data-driven analysis. Nat. Commun. 7:11934. doi: 10.1038/Ncomms11934
Jack, C. R., Bennett, D. A., Blennow, K., Carrillo, M. C., Dunn, B., Haeberlein, S. B., et al. (2018). Nia-aa research framework: toward a biological definition of Alzheimer's Disease. Alzheimers Dement. 14, 535–562. doi: 10.1016/J.Jalz.2018.02.018
Keller, J. A., Sigurdsson, S., Klaassen, K., Hirschler, L., Van Buchem, M. A., Launer, L. J., et al. (2023). White matter Hyperintensity shape is associated with Long-term dementia risk. Alzheimers Dement. 19, 5632–5641. doi: 10.1002/Alz.13345
Kester, M. I., Goos, J. D., Teunissen, C. E., Benedictus, M. R., Bouwman, F. H., Wattjes, M. P., et al. (2014). Associations between cerebral small-vessel Disease and Alzheimer Disease pathology as measured by cerebrospinal fluid biomarkers. JAMA Neurol. 71, 855–862. doi: 10.1001/Jamaneurol.2014.754
Klohs, J. (2019). An integrated view on vascular dysfunction in Alzheimer's Disease. Neurodegener Dis 19, 109–127. doi: 10.1159/000505625
Kurz, A., and Riemenschneider, M., And Wallin, A. (2003). Potential biological markers for cerebrovascular Disease. Int. Psychogeriatr. 15, 89–97. doi: 10.1017/S1041610203009025
Landau, S. M., Harvey, D., Madison, C. M., Koeppe, R. A., Reiman, E. M., Foster, N. L., et al. (2011). Associations between cognitive, functional, and Fdg-pet measures of decline in ad and mci. Neurobiol. Aging 32, 1207–1218. doi: 10.1016/J.Neurobiolaging.2009.07.002
Lee, S., Viqar, F., Zimmerman, M. E., Narkhede, A., Tosto, G., Benzinger, T. L., et al. (2016). White matter Hyperintensities are a Core feature of Alzheimer's Disease: evidence from the dominantly inherited Alzheimer network. Ann. Neurol. 79, 929–939. doi: 10.1002/Ana.24647
Li, T. R., And Liu, F. Q. (2022). Β-amyloid promotes platelet activation and activated platelets act as bridge between risk factors and Alzheimer's Disease. Mech. Ageing Dev. 207,:111725. doi: 10.1016/J.Mad.2022.111725
Li, T. R., Lyu, D. Y., and Liu, F. Q., And Alzheimer’s Disease Neuroimaging Initiative (2022a). Cerebrospinal fluid Strem2 in Alzheimer's Disease is associated with both amyloid and tau pathologies but not with cognitive status. J. Alzheimers Dis. 90, 1123–1138. doi: 10.3233/Jad-220598
Li, T. R., Yang, Q., and Hu, X., And Han, Y. (2022b). Biomarkers and tools for predicting Alzheimer's Disease in the preclinical stage. Curr. Neuropharmacol. 20, 713–737. doi: 10.2174/1570159x19666210524153901
Liu, Y., Braidy, N., Poljak, A., and Chan, D., And Sachdev, P. (2018). Cerebral small vessel Disease and the risk of Alzheimer's Disease: a systematic review. Ageing Res. Rev. 47, 41–48. doi: 10.1016/J.Arr.2018.06.002
Long, J. M., And Holtzman, D. M. (2019). Alzheimer Disease: An update on pathobiology and treatment strategies. Cell 179, 312–339. doi: 10.1016/J.Cell.2019.09.001
Loos, C., Makin, S., Staals, J., Dennis, M. S., and Van Oostenbrugge, R. J., And Wardlaw, J. M. (2018). Long-term morphological changes of symptomatic lacunar infarcts and surrounding white matter on structural magnetic resonance imaging. Stroke 49, 1183–1188. doi: 10.1161/Strokeaha.117.020495
Love, S., And Miners, J. S. (2016). Cerebrovascular Disease in ageing and Alzheimer's Disease. Acta Neuropathol. 131, 645–658. doi: 10.1007/S00401-015-1522-0
Luo, J., Ma, Y., Agboola, F. J., Grant, E., Morris, J. C., Mcdade, E., et al. (2023). Longitudinal relationships of white matter Hyperintensities and Alzheimer Disease biomarkers across the adult life span. Neurology 101:E164-164e177. doi: 10.1212/Wnl.0000000000207378
Marchant, N. L., Reed, B. R., Sanossian, N., Madison, C. M., Kriger, S., Dhada, R., et al. (2013). The aging brain and cognition: contribution of vascular injury and Aβ to mild cognitive dysfunction. JAMA Neurol. 70, 488–495. doi: 10.1001/2013.Jamaneurol.405
Marnane, M., Al-Jawadi, O. O., Mortazavi, S., Pogorzelec, K. J., Wang, B. W., Feldman, H. H., et al. (2016). Periventricular Hyperintensities are associated with elevated cerebral amyloid. Neurology 86, 535–543. doi: 10.1212/Wnl.0000000000002352
Mattsson, N., Cullen, N. C., Andreasson, U., and Zetterberg, H., And Blennow, K. (2019). Association between longitudinal plasma Neurofilament light and neurodegeneration in patients with Alzheimer Disease. JAMA Neurol. 76, 791–799. doi: 10.1001/Jamaneurol.2019.0765
Mcaleese, K. E., Firbank, M., Dey, M., Colloby, S. J., Walker, L., Johnson, M., et al. (2015). Cortical tau load is associated with white matter Hyperintensities. Acta Neuropathol. Commun. 3:60. doi: 10.1186/S40478-015-0240-0
Mckhann, G., Drachman, D., Folstein, M., Katzman, R., and Price, D., And Stadlan, E. M. (1984). Clinical diagnosis of Alzheimer's Disease: report of the Nincds-Adrda work group under the auspices of department of health and human services task force on Alzheimer's Disease. Neurology 34, 939–944. doi: 10.1212/Wnl.34.7.939
Moroni, F., Ammirati, E., and Hainsworth, A. H., And Camici, P. G. (2020). Association of white matter Hyperintensities and cardiovascular Disease: the importance of microcirculatory Disease. Circ. Cardiovasc. Imaging 13,:E010460. doi: 10.1161/Circimaging.120.010460
Ortner, M., Lanz, K., Goldhardt, O., Müller-Sarnowski, F., Diehl-Schmid, J., Förstl, H., et al. (2023). Elecsys cerebrospinal fluid immunoassays accurately detect Alzheimer's Disease regardless of concomitant small vessel Disease. J. Alzheimers Dis. 93, 1537–1549. doi: 10.3233/Jad-221187
Osborn, K. E., Liu, D., Samuels, L. R., Moore, E. E., Cambronero, F. E., Acosta, L., et al. (2018). Cerebrospinal fluid Β-Amyloid42 and Neurofilament light relate to white matter Hyperintensities. Neurobiol. Aging 68, 18–25. doi: 10.1016/J.Neurobiolaging.2018.03.028
Park, J. H., Seo, S. W., Kim, C., Kim, S. H., Kim, G. H., Kim, S. T., et al. (2014). Effects of cerebrovascular Disease and amyloid Beta burden on cognition in subjects with subcortical vascular cognitive impairment. Neurobiol. Aging 35, 254–260. doi: 10.1016/J.Neurobiolaging.2013.06.026
Prins, N. D., And Scheltens, P. (2015). White matter Hyperintensities, cognitive impairment and dementia: An update. Nat. Rev. Neurol. 11, 157–165. doi: 10.1038/Nrneurol.2015.10
Roseborough, A., Ramirez, J., and Black, S. E., And Edwards, J. D. (2017). Associations between amyloid Β and white matter Hyperintensities: a systematic review. Alzheimers Dement. 13, 1154–1167. doi: 10.1016/J.Jalz.2017.01.026
Rost, N. S., Brodtmann, A., Pase, M. P., Van Veluw, S. J., Biffi, A., Duering, M., et al. (2022). Post-stroke cognitive impairment and dementia. Circ. Res. 130, 1252–1271. doi: 10.1161/Circresaha.122.319951
Royse, S. K., Cohen, A. D., and Snitz, B. E., And Rosano, C. (2021). Differences in Alzheimer's Disease and related dementias pathology among African American and Hispanic women: a qualitative literature review of biomarker studies. Front. Syst. Neurosci. 15,:685957. doi: 10.3389/Fnsys.2021.685957
Schneider, J. A., Arvanitakis, Z., and Bang, W., And Bennett, D. A. (2007). Mixed brain pathologies account for Most dementia cases in community-dwelling older persons. Neurology 69, 2197–2204. doi: 10.1212/01.Wnl.0000271090.28148.24
Silva, N., Bracko, O., Nelson, A. R., De Oliveira, F. F., Robison, L. S., Shaaban, C. E., et al. (2022). Vascular cognitive impairment and dementia: An early career researcher perspective. Alzheimers Dement. 14:E12310. doi: 10.1002/Dad2.12310
Snowdon, D. A., Greiner, L. H., Mortimer, J. A., Riley, K. P., and Greiner, P. A., And Markesbery, W. R. (1997). Brain infarction and the clinical expression of Alzheimer Disease. The Nun study. JAMA 277, 813–817. doi: 10.1001/jama.1997.03540340047031
Toledo, J. B., Arnold, S. E., Raible, K., Brettschneider, J., Xie, S. X., Grossman, M., et al. (2013). Contribution of cerebrovascular Disease in autopsy confirmed neurodegenerative Disease cases in the National Alzheimer's coordinating Centre. Brain 136, 2697–2706. doi: 10.1093/Brain/Awt188
Troncoso, J. C., Zonderman, A. B., Resnick, S. M., Crain, B., and Pletnikova, O., And O'brien, R. J. (2008). Effect of infarcts on dementia in the Baltimore longitudinal study of aging. Ann. Neurol. 64, 168–176. doi: 10.1002/Ana.21413
Twait, E. L., Min, B., Beran, M., and Vonk, J., And Geerlings, M. I. (2023). The cross-sectional association between amyloid burden and white matter Hyperintensities in older adults without cognitive impairment: a systematic review and Meta-analysis. Ageing Res. Rev. 88,:101952. doi: 10.1016/J.Arr.2023.101952
Van Den Berg, E., Geerlings, M. I., Biessels, G. J., and Nederkoorn, P. J., And Kloppenborg, R. P. (2018). White matter Hyperintensities and cognition in mild cognitive impairment and Alzheimer's Disease: a domain-specific Meta-analysis. J. Alzheimers Dis. 63, 515–527. doi: 10.3233/Jad-170573
van Waalwijk van Doorn, L., Ghafoorian, M., van, E., Claassen, J., Arighi, A., Bozzali, M., et al. (2021). White matter Hyperintensities are no major confounder for Alzheimer's Disease cerebrospinal fluid biomarkers. J. Alzheimers Dis. 79, 163–175. doi: 10.3233/Jad-200496
Van Westen, D., Lindqvist, D., Blennow, K., Minthon, L., Nägga, K., Stomrud, E., et al. (2016). Cerebral white matter lesions - associations with Aβ isoforms and amyloid pet. Sci. Rep. 6:20709. doi: 10.1038/Srep20709
Verhaaren, B. F., Vernooij, M. W., De Boer, R., Hofman, A., Niessen, W. J., Van Der Lugt, A., et al. (2013). High blood pressure and cerebral white matter lesion progression in the general population. Hypertension 61, 1354–1359. doi: 10.1161/Hypertensionaha.111.00430
Walsh, P., Sudre, C. H., Fiford, C. M., Ryan, N. S., Lashley, T., Frost, C., et al. (2020). Csf amyloid is a consistent predictor of white matter Hyperintensities across the Disease course from aging to Alzheimer's Disease. Neurobiol. Aging 91, 5–14. doi: 10.1016/J.Neurobiolaging.2020.03.008
Weaver, N. A., Doeven, T., Barkhof, F., Biesbroek, J. M., Groeneveld, O. N., Kuijf, H. J., et al. (2019). Cerebral amyloid burden is associated with white matter Hyperintensity location in specific posterior white matter regions. Neurobiol. Aging 84, 225–234. doi: 10.1016/J.Neurobiolaging.2019.08.001
Wei, K., Tran, T., Chu, K., Borzage, M. T., Braskie, M. N., Harrington, M. G., et al. (2019). White matter Hypointensities and Hyperintensities have equivalent correlations with age and Csf Β-amyloid in the nondemented elderly. Brain Behav. 9:E01457. doi: 10.1002/Brb3.1457
Ying, C., Kang, P., Binkley, M. M., Ford, A. L., Chen, Y., Hassenstab, J., et al. (2023). Neuroinflammation and amyloid deposition in the progression of mixed Alzheimer and vascular dementia. Neuroimage Clin. 38:103373. doi: 10.1016/J.Nicl.2023.103373
Yoshita, M., Fletcher, E., Harvey, D., Ortega, M., Martinez, O., Mungas, D. M., et al. (2006). Extent and distribution of white matter Hyperintensities in Normal aging, mci, and ad. Neurology 67, 2192–2198. doi: 10.1212/01.Wnl.0000249119.95747.1f
Zhang, H., Lyu, D., and Jia, J., And Alzheimer’s Disease Neuroimaging Initiative (2022). The trajectory of cerebrospinal fluid growth-associated protein 43 in the Alzheimer's Disease continuum: a longitudinal study. J. Alzheimers Dis. 85, 1441–1452. doi: 10.3233/Jad-215456
Zheng, Q., And Wang, X. (2024). Alzheimer's disease: Insights into pathology, molecular mechanisms, and therapy. Protein Cell. doi: 10.1093/Procel/Pwae026
Keywords: Alzheimer’s disease, WMH, cerebral small vessel disease, Aβ, cognition, neurodegeneration
Citation: Li T-R, Li B-L, Xu X-R, Zhong J, Wang T-S, Liu F-Q and the Alzheimer’s Disease Neuroimaging Initiative (2024) Association of white matter hyperintensities with cognitive decline and neurodegeneration. Front. Aging Neurosci. 16:1412735. doi: 10.3389/fnagi.2024.1412735
Received: 05 April 2024; Accepted: 28 August 2024;
Published: 12 September 2024.
Edited by:
Kassandra Kisler, University of Southern California, United StatesReviewed by:
C. Elizabeth Shaaban, University of Pittsburgh, United StatesCopyright © 2024 Li, Li, Xu, Zhong, Wang, Liu and the Alzheimer’s Disease Neuroimaging Initiative. This is an open-access article distributed under the terms of the Creative Commons Attribution License (CC BY). The use, distribution or reproduction in other forums is permitted, provided the original author(s) and the copyright owner(s) are credited and that the original publication in this journal is cited, in accordance with accepted academic practice. No use, distribution or reproduction is permitted which does not comply with these terms.
*Correspondence: Tao-Ran Li, bGl0YW9yYW5AbmptdS5lZHUuY24=; Feng-Qi Liu, bGl1ZmVuZ3FpQG5qbXUuZWR1LmNu
†ORCID: Tao-Ran Li, https://orcid.org/0000-0002-0451-2850
Feng-Qi Liu, https://orcid.org/0000-0002-7504-2243
Disclaimer: All claims expressed in this article are solely those of the authors and do not necessarily represent those of their affiliated organizations, or those of the publisher, the editors and the reviewers. Any product that may be evaluated in this article or claim that may be made by its manufacturer is not guaranteed or endorsed by the publisher.
Research integrity at Frontiers
Learn more about the work of our research integrity team to safeguard the quality of each article we publish.