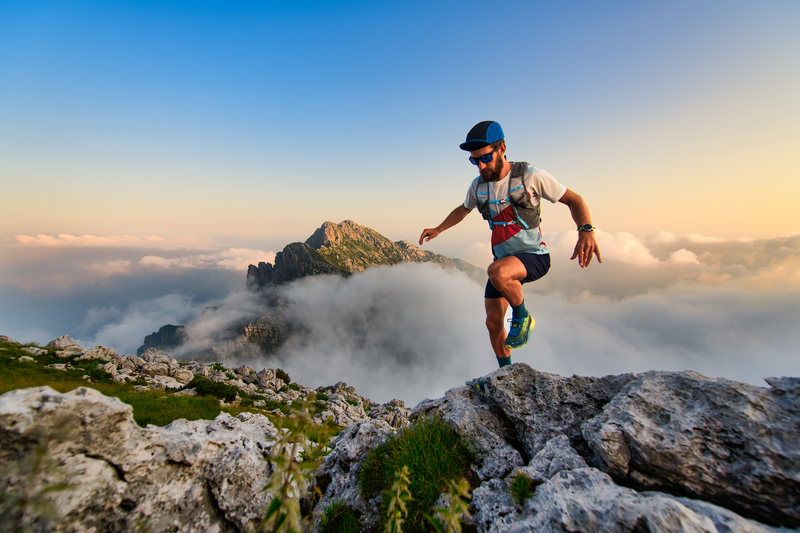
94% of researchers rate our articles as excellent or good
Learn more about the work of our research integrity team to safeguard the quality of each article we publish.
Find out more
ORIGINAL RESEARCH article
Front. Aging Neurosci. , 05 June 2024
Sec. Neurocognitive Aging and Behavior
Volume 16 - 2024 | https://doi.org/10.3389/fnagi.2024.1346807
This article is part of the Research Topic Interventions for Cognitive Decline in Pathological Aging: A Community-Driven Approach View all 7 articles
Background: Sleep-related disorders have been associated with cognitive decline and neurodegeneration. American Indians are at increased risk for dementia. Here, we aim to characterize, for the first time, the associations between sleep characteristics and subsequent cognitive performance in a sample of aging American Indians.
Methods: We performed analyses on data collected in two ancillary studies from the Strong Heart Study, which occurred approximately 10 years apart with an overlapping sample of 160 American Indians (mean age at follow-up 73.1, standard deviation 5.6; 69.3% female and 80% with high school completion). Sleep measures were derived by polysomnography and self-reported questionnaires, including sleep timing and duration, sleep latency, sleep stages, indices of sleep-disordered breathing, and self-report assessments of poor sleep and daytime sleepiness. Cognitive assessment included measures of general cognition, processing speed, episodic verbal learning, short and long-delay recall, recognition, and phonemic fluency. We performed correlation analyses between sleep and cognitive measures. For correlated variables, we conducted separate linear regressions. We analyzed the degree to which cognitive impairment, defined as more than 1.5 standard deviations below the average Modified Mini Mental State Test score, is predicted by sleep characteristics. All regression analyses were adjusted for age, sex, years of education, body mass index, study site, depressive symptoms score, difference in age from baseline to follow-up, alcohol use, and presence of APOE e4 allele.
Results: We found that objective sleep characteristics measured by polysomnography, but not subjective sleep characteristics, were associated with cognitive performance approximately 10 years later. Longer sleep latency was associated with worse phonemic fluency (β = −0.069, p = 0.019) and increased likelihood of being classified in the cognitive impairment group later in life (odds ratio 1.037, p = 0.004). Longer duration with oxygen saturation < 90% was associated with better immediate verbal memory, and higher oxygen saturation with worse total learning, short and long-delay recall, and processing speed.
Conclusion: In a sample of American Indians, sleep characteristics in midlife were correlated with cognitive performance a decade later. Sleep disorders may be modifiable risk factors for cognitive impairment and dementia later in life, and suitable candidates for interventions aimed at preventing neurodegenerative disease development and progression.
Sleep quality is a complex construct that can be measured both objectively and subjectively, and includes quantitative aspects of sleep, such as duration and latency, but also qualitative aspects, such as how restored someone feels after sleeping (Buysse et al., 1989; Nelson et al., 2022). Sleep–wake cycle disruptions are frequent during the course of dementia and the prodromic phases of neurodegeneration (Lyketsos et al., 2002; Moran et al., 2005; Zhao et al., 2016). The relationship between sleep and dementia is bidirectional; while physiological and neuropathological aspects of dementia reduce the quality of sleep, poor sleep quality may also increase the risk of neurodegenerative diseases and dementia (McCoy and Strecker, 2011; Lim et al., 2014; Borges et al., 2019). Sleep and nighttime behavior disturbances are among the most important symptoms of dementia, leading to a need for long-term care, institutionalization, and caregiver burden in various populations (Hope et al., 1998; Moran et al., 2005; Fonseca et al., 2021), with attendant costs to individuals and society.
Poor sleep quality and short sleep duration during midlife have been associated with increased amyloid-beta deposition and the acceleration of regional brain hypometabolism (Choe et al., 2019), respectively. A study in young adults also showed that certain sleep disturbance patterns (such as elevated slow waves and daytime sleepiness) were associated with genetic liability for Alzheimer’s Disease (AD) before the appearance of other AD biomarkers (Muto et al., 2020). Disturbed sleep may also impact negatively on sleep-dependent consolidation of memory and on cerebral spinal fluid mediated clearance of metabolic residues from the brain, which are linked processes (Fultz et al., 2019) also known to be correlated with amyloidosis and AD (Bateman et al., 2007).
Sleep plays an important role in cognitive performance (Walker, 2009; Tsapanou et al., 2020), and the importance of sleep for the codification and consolidation of memory as well as for the retrieval of new declarative memories (Walker, 2009) is well established. Without adequate sleep, hippocampal function is disrupted and, consequently, so is the ability to record new experiences (Eichenbaum, 2004). Good sleep quality has been shown to correlate with better cognitive function in children (El-Sheikh et al., 2019) and has an impact on cognitive function during the waking period in adolescence, contributing to learning (Tarokh et al., 2016). In 141 middle-aged participants on the Sleep Heart Health Study, more severe oxygen saturation was associated with worse motor speed and processing speed performance after 9–40 months (Quan et al., 2006). A 25-year follow-up study including 7,959 participants demonstrated that persistent short sleep duration (<6 h) at ages 50, 60 and 70 was associated with a 30% increase in the risk of dementia compared with 6–8 h of sleep duration (Sabia et al., 2021). Correlations between sleep disorders and cognitive measures in healthy individuals (Walker, 2009; Tsapanou et al., 2016) and those with AD (Bonanni et al., 2005; Shin et al., 2014) have been found, with poorer nighttime sleep quality being associated with worse performance on praxis, immediate recall, and global cognition in AD patients (Shin et al., 2014). Furthermore, daytime sleepiness has been associated with cognitive performance deficits in both cross-sectional and longitudinal studies (Foley et al., 2001; Ohayon and Vecchierini, 2002; Bonanni et al., 2005; Merlino et al., 2010; Elwood et al., 2011; Tsapanou et al., 2016; Muto et al., 2020), as well as with incident dementia in many longitudinal studies (Foley et al., 2001; Elwood et al., 2011; Schlosser Covell et al., 2012). Some studies have also found sleep-disordered breathing to be associated with generally impaired cognitive function (Cohen-Zion et al., 2001; Spira et al., 2008), particularly impaired executive function (Ju et al., 2012) and memory (Aloia et al., 2003; Ju et al., 2012).
There is a growing body of evidence showing that there are racial/ethnic disparities related to sleep quality (Kalliny and McKenzie, 2017; Pandi-Perumal et al., 2017; Prasad et al., 2018; Denney et al., 2023). Sleep quality is influenced by various social, cultural, and genetic factors (Kalliny and McKenzie, 2017; Prasad et al., 2018). It is believed that the racial differences found in sleep disorders are due to several environmental stressors in personal health, such as socioeconomic inequalities, acculturation, and racism (Ruiter et al., 2011; Egan et al., 2017; Prasad et al., 2018; Liu et al., 2021). Sleep-related disorders, such as obstructive sleep apnea, and excessive daytime sleepiness have been linked to poor health outcomes, including cardiovascular disease (Ruiter et al., 2010; Drager et al., 2017; Egan et al., 2017). Also, there is evidence that both sleep disorders (Ruiter et al., 2010; Chen et al., 2015) and cardiovascular diseases (Egan et al., 2017; Zuma et al., 2021) are influenced by race/ethnicity. Furthermore, sleep-related disorders as well as vascular factors that can be associated with sleep deficiencies, such as arterial hypertension, diabetes, and hypercholesterolemia, are known risk factors for cognitive impairment and AD and related dementias. American Indians have a high prevalence of cerebral vascular disease (Hutchinson and Shin, 2014; Suchy-Dicey et al., 2017), stroke (Harris et al., 2015) and type 2 diabetes (Centers for Disease Control and Prevention, 2003; Cholerton et al., 2019), along with the presence of other health disparities (Hutchinson and Shin, 2014), but the impact of sleep-related disorders and their relationships with dementia are understudied in this population.
A recent study of self-reported sleep duration and mortality risk in different racial and ethnic groups found elevated risks of all-cause mortality for those who reported sleeping less than 5 h or more than 9 h per day, with variation by racial and ethnic identification (Denney et al., 2023). This study found that relative to White individuals, a significantly higher proportion of American Indians reported sleeping less than 5 h or more than 9 h. However, the authors acknowledged that the study was underpowered to estimate the association between sleep duration and mortality for this specific population.
To our knowledge, there are no studies investigating sleep-related disorders and their cognitive consequences focused specifically on American Indians. Data are needed in this population to identify possible opportunities for primary and secondary prevention of cognitive decline. The aim of this study is to characterize, for the first-time, sleep characteristics and their relationships with cognitive performance and classification of cognitive impairment approximately 10 years later in a sample of American Indians. Based on data showing that prevalent health and racial/ethnic disparities may contribute to sleep disorders (Kalliny and McKenzie, 2017; Pandi-Perumal et al., 2017; Prasad et al., 2018), that sleep disorders are frequently linked to cognitive decline (Foley et al., 2001; Merlino et al., 2010; Tsapanou et al., 2016), and that older American Indians are at greater risk for cognitive decline and dementia (Centers for Disease Control and Prevention, 2003; Hutchinson and Shin, 2014; Suchy-Dicey et al., 2017), we hypothesize that poor sleep quality will be associated with worse cognitive performance 10 years later.
The current study involves secondary analyses of data collected in two multisite ancillary studies from the Strong Heart Study: the Sleep Heart Health Study (Quan et al., 1997) (SHHS), which includes polysomnography and self-reported sleep quality data, and the Cerebrovascular Disease and its Consequences in American Indians (CDCAI) study (Suchy-Dicey et al., 2016), which includes neuropsychological assessments. Written informed consent was obtained from all participants for both studies. Study procedures were approved by the appropriate Institutional Research Boards, Tribal Research Review Boards, or Tribal Councils.
Sleep data were collected in the SHHS, which enrolled participants from several observational studies of cardiovascular and respiratory disease including the Strong Heart Study (SHS). The SHHS took place from 1995 until 2009; its design and methods have been fully described elsewhere (Quan et al., 1997). Sleep data were collected during a home visit. This included a polysomnogram, measures of sleep-disordered breathing, and self-report assessments of poor sleep quality, snoring, insomnia, and daytime sleepiness from the SHHS Sleep Habits Questionnaire (Quan et al., 1997).
The CDCAI study is the largest cohort of aging American Indians that included neuropsychological data collection. The study took place from 2010 until 2013 and included 818 participants from the SHS recruited from three study sites – the Northwest Plains, Southern Plains, and Southwest region – representing 11 of the 13 original SHS communities. The design and methods of the CDCAI study have been fully described elsewhere (Suchy-Dicey et al., 2016). See Figure 1 for a diagram of all three studies involved.
Of the 818 American Indians who completed neuropsychological testing in the CDCAI study (mean age of 73.0 years during the years 2010–2013, standard deviation 5.9 years; 555 females, 67.9%), we identified 160 who also had sleep data from the SHHS (mean age of 62.6 years during the years 1995–1998, standard deviation 5.4 years; 111 females, 69.3%). The participant inclusion diagram is shown in Figure 1.
Sleep characteristics assessed in the SHHS were identified by (1) polysomnography using the validated portable PS-2 polysomnography system (Compumedics, Abottsville, AU) (Redline et al., 1998), including electroencephalogram (derivations C3/A1 and C4/A2), left and right electrooculogram, submental chin electromyogram, and electrocardiogram (modified Lead I), as well as measurements of abdominal and thoracic excursions, oxyhemoglobin saturation, airflow, body position, and ambient light; (2) sleep-disordered breathing evaluated using apnea-hypopnea and respiratory disturbance indices; and (3) self-reported indicators of poor sleep quality (delayed sleep onset, short sleep duration, snoring, and insomnia) and daytime sleepiness collected with the SHHS Sleep Habits Questionnaire (Quan et al., 1997). Polysomnography data were available for 139 participants, the remaining participants (n = 21) declined to undergo polysomnography. Scoring of sleep architecture was performed using Rechtschaffen and Kales criteria (Rechtschaffen and Kales, 1968).
Sleep variables used for this study were: sleep onset time, total sleep time, sleep efficiency, sleep latency, rapid eye movement (REM) sleep latency, percentage sleep time in REM sleep, percentage sleep time in stage 1 sleep, percentage sleep time in stage 2 sleep, percentage sleep time in slow wave sleep (stages 3 and 4 were combined in slow wave sleep), obstructive apnea hypopnea index (irrespective of any associated oxygen desaturation as well as associated with oxygen desaturation ≥4%), arousal index, average oxygen saturation, lowest oxygen saturation during non-REM (NREM) sleep, lowest oxygen saturation during REM sleep, number of oxygen desaturations, respiratory disturbance index (RDI) (associated oxygen desaturation ≥3%), central apnea index, lowest overall oxygen saturation, oxygen saturation classification (defined as: normal or minimal >90%, mild desaturation 80–90%, and severe desaturation <80%), and percent of sleep time with oxygen saturation < 90%. Self-reported delayed sleep onset, as well as Epworth Sleepiness Scale (ESS) score and daytime sleepiness classification (ESS > 10) were also included. Detailed information and metrics have been fully described elsewhere (Quan et al., 1997, 2007).
The neuropsychological assessment in the CDCAI study included (1) the Modified Mini Mental State Test (3MSE) (Teng and Chui, 1987), a measure of general cognition based on the Mini Mental State Test (Folstein et al., 1975); (2) the Wechsler Adult Intelligence Scale (WAIS-IV) digit symbol coding test (Wechsler, 2008), a measure of processing speed; (3) the California Verbal Learning Test, Second Edition, Short Form (CVLT-SF) (Delis et al., 2000), which measures episodic verbal learning (total learning), recall (short-delay and long-delay free recall), and recognition discriminability; and (4) the Controlled Oral Word Association (COWA) test (Ruff et al., 1996), which provides a measure of verbal phonemic fluency. Measurements were grouped in cognitive domains as follows: (1) Memory, CVLT-SF subtests for total learning, short-delay free recall, long-delay free recall, and recognition discriminability; (2) Processing speed, WAIS-IV digit symbol coding test (number correctly coded in 120 s); (3) Executive Function, COWA test; and (4) Global cognition, 3MSE test. All cognitive variables are continuous raw scores, with higher scores corresponding to better performance.
Subjects were categorized as having cognitive impairment if their individual z-scores for the 3MSE test, based on the mean and standard deviation of the sample during the 10-year follow-up (N = 160), were less than −1.5 (i.e., more than 1.5 standard deviations below the group average 3MSE score). While there is no established cutoff point for this population, this classification considers the individual in comparison with the group of the same race/ethnicity, and a criterion of 1.5 standard deviations from the mean is a common approach to define mild cognitive impairment (Petersen et al., 2001, 2014).
During the CDCAI study, participants completed self-administered questionnaires for sociodemographic data, including sex (self-reported as male or female), education (years), self-reported alcohol consumption (current or former drinker), and height and weight from which body mass index (BMI) was calculated. The Centers for Epidemiologic Studies – Depression (CESD) scale assessed symptoms of depression (range 0–60, higher scores indicate greater depressive symptoms) (Radloff, 1977). To account for the time interval between the studies, we also included a variable for participants’ age difference from baseline to follow-up (age at CDCAI – age at SHHS). Blood was collected for genetic assessment of the presence of the apolipoprotein E e4 (APOE e4) allele, a risk factor for dementia (Gharbi-Meliani et al., 2021), determined with isoelectric focusing.
Descriptive statistics were calculated and presented as absolute and relative frequencies or as means and standard deviations for the sample. The Kolmogorov–Smirnov test was used to test for normality, and nonparametric analysis was used when needed. Relationships among sleep variables were assessed by means of correlations.
To determine whether sleep characteristics were associated with cognitive performance and cognitive impairment assessed approximately 10 years later, we first calculated correlations between each of the sleep measures from the SHHS and each of the cognitive outcomes from the CDCAI study using Spearman rho as data did not follow a normal distribution. For variables that were significantly correlated, linear regression analyses were conducted with each cognitive outcome as the dependent variable and each sleep measures as the independent variable, adjusting for age, sex, years of education, BMI, study site, CESD depressive symptoms score, participants’ age difference from baseline to follow-up, alcohol use, and APOE e4 genotype.
The degree to which cognitive impairment was predicted by sleep characteristics was examined with odds ratios determined with logistic regression, adjusting for age, sex, years of education, BMI, site, CESD depressive symptoms score, participant’s age difference from baseline to follow up, alcohol use, and APOE e4 genotype. Sleep variables were included in this logistic regression model if: (1) there was an association of the variable with cognitive outcomes in the bivariate analyses; (2) any collinearity was resolved; and (3) there was an empirical or theoretical rationale for including the variable in the model. To reduce the dimensionality of the comparisons, this analysis was performed as a stepwise regression analysis.
Examination of correlations between predictor variables revealed pairs of highly correlated sleep variables. We found collinearity for oxygen desaturation index, respiratory disturbance index, obstructive apnea hypopnea index, lowest overall oxygen saturation level, and lowest % of oxygen saturation during REM and NREM sleep. When collinearity was encountered, we selected only those variables that were correlated with cognitive outcomes in the bivariate analysis. Any remaining collinearity was resolved by choosing the variables with the greatest clinical utility (e.g., percent of sleep time with oxygen saturation < 90% instead of average oxygen saturation).
The type I error threshold for statistical significance was set at 0.05. This study involves a discovery-based approach in an exploratory analysis of sleep and cognitive data reported for the first time for American Indians. Therefore, to control type I error given multiple comparisons, but still be able to identify as many significant associations as possible, we used false discovery rate corrections to p-values, which is a less conservative approach than Bonferroni corrections. Statistical analyses were carried out using the Statistical Package for the Social Sciences (SPSS), version 28 for Windows.
Table 1 shows the demographic characteristics of the participants as well as results from the neuropsychological assessment battery and CESD depression scale. Demographic data of this subgroup are comparable to data from the entire CDCAI cohort, as are the results of the cognitive tests. An exception was the mean 3MSE, a test of general cognition with a greater range of scores (1–100). The mean 3MSE score for this group (mean = 93.9/ standard deviation = 11.8) was higher than the mean for the entire CDCAI cohort (mean = 88.5/ standard deviation = 10.1). Of the 160 participants of this group, 111 (69.4%) were female, with a mean age of 62.6 years at the first timepoint (SHHS) and 73.1 years at the second timepoint (CDCAI). In the sample, 50% of the participants were from the Northern Plains, 37.5% were from the Southern Plains, and 12.5% were from the Southwest region. The mean interval between studies measured by participants’ age difference from baseline to follow-up was 10.4 years, ranging from 7 to 16 years. Participants had from 6 to 20 years of education, with 80% of the sample having completed high school or more. Twenty three percent of the sample were carriers of the APOE e4 allele.
Table 2 presents the sleep variables. Mean sleep duration was 371.3 min (6.19 h), with a standard deviation of 73.5 min. Of the total sample, 18% slept less than 5 h, 41.7% slept for less than 6 h, and 74.8% slept for less than 7 h, as measured by polysomnography. 33 participants had a sleep latency of more than 30 min (23.7% of 139). Forty percent of the sample had moderate to severe respiratory disturbance as determined by a respiratory disturbance index ≥15 events per hour. Moreover, 55.6% were classified with mild oxygen desaturation (80–90% saturation) and 24.3% were classified with severe oxygen desaturation, as measured during polysomnography.
Based on self-reported information on the Epworth Sleepiness Scale, 20% of the sample was classified as having excessive daytime sleepiness, with 48.1% out of the 160 participants reporting daytime sleepiness at least twice a month or more, and 6.2% reporting daytime sleepiness almost every day. Furthermore, out of the 160 study participants, 41.2% reported having delayed sleep onset at least twice a month, while 2.5% reported delayed sleep onset almost every day.
Table 3 shows the correlations between sleep characteristics and cognitive performance evaluated approximately 10 years later, adjusted for multiple comparisons using false discovery rate.
Verbal list learning performance was positively correlated with sleep efficiency and respiratory disturbance index, and negatively correlated with percentage of sleep time in stage 1, average oxygen saturation, and lowest percent of oxygen saturation during REM sleep. Short-delay free recall was positively correlated with percent of sleep time with oxygen saturation below 90% and negatively correlated with average oxygen saturation (i.e., better oxygen saturation predicted impaired short-delay free recall). Long-delay free recall was positively correlated with percent of sleep time in REM and percent of sleep time with oxygen saturation below 90%, and negatively correlated with average oxygen saturation and lowest % of oxygen saturation during REM sleep (i.e., better oxygen saturation overall and during REM sleep predicted impaired long-delay free recall).
Total COWA score was positively correlated with sleep duration and sleep efficiency, and negatively correlated with sleep latency.
WAIS-IV digit symbol coding test performance was negatively correlated with percent of sleep time in stage 1 and average oxygen saturation.
3MSE score was positively correlated with sleep efficiency and negatively correlated with average oxygen saturation. Recognition discriminability measured by the CVLT-SF was not correlated with any of the sleep variables.
For the sleep and cognition variable pairs that were significantly correlated, separate linear regression analyses were conducted using each cognitive outcome as the dependent variable and the associated sleep variable as the independent variable (predictor), adjusting for the covariates. Table 4 shows the variable pairs that were statistically significant in these regression analyses.
Table 4. Significant associations between sleep characteristics and cognitive performance in pairwise linear regression models adjusted for age, BMI, sex, study site, years of education, CESD depressive symptoms score, age difference from baseline to follow-up, alcohol use, and presence of APOE e4 allele.
More time in REM sleep predicted better performance on CVLT-SF total learning. Higher oxygen saturation predicted worse performance on CVLT-SF total learning, short-delay free recall, and long-delay free recall.
Longer sleep latency predicted worse performance on the COWA test.
More time in REM sleep predicted worse performance on the WAIS-IV digit symbol coding test.
Table 5 shows the results of stepwise logistic regression for clinically relevant cognitive impairment, defined as >1.5 standard deviations below the average 3MSE score, with multiple sleep variables as predictors and adjusted for the covariates. All sleep variables with any significant correlations with cognition (Table 3) entered the model (i.e., sleep latency, sleep duration, sleep efficiency, percentage of time in stage 1, % sleep in REM, lowest % of oxygen saturation during REM, respiratory index 3%, % sleep time with oxygen <90%), except for average oxygen saturation due to collinearity with percent sleep time with oxygen saturation < 90%. Following completion of the stepwise regression process, the logistic regression model was significant (X216 = 56.20, p < 0.001), explaining 57.8% of the variance (Nagelkerke R2) and correctly classifying 88.5% of cases. Sleep latency was the only sleep variable that was retained through the stepwise regression process. Longer sleep latency (expressed in minutes) was associated with greater likelihood of being classified in the cognitive impairment group, with odds ratio 1.035 (95% confidence interval: 1.010–1.061; p = 0.005).
Table 5. Associations between sleep characteristics and the odds of cognitive impairment, adjusted for age, sex, BMI, site, years of education, CESD depressive symptoms score, age difference from baseline to follow-up, alcohol use, and presence of the APOE e4 allele (N = 130).
Age, sex, years of education, and study site were significant as covariates. Older individuals (with age expressed in years) were more likely to be classified in the cognitive impairment group, with odds ratio 1.189 (95% confidence interval: 1.033–1.368; p = 0.016). Females were less likely to exhibit cognitive impairment compared with males, with odds ratio 0.089 (95% confidence interval: 0.010–0.759; p = 0.027). Fewer years of education predicted greater likelihood of cognitive impairment, with odds ratio 0.564 (95% confidence interval: 0.384–0.827; p = 0.003). Cognitive impairment was more probable in the Southern Planes, with odds ratio 19.334 (95% confidence interval: 2.378–157.181; p = 0.006); and in the Southwest region, with odds ratio 37.615 (95% confidence interval: 2.165–653.632; p = 0.013), as compared to the Northern Plains.
This study characterized, for the first-time, associations between sleep characteristics and cognitive performance in a sample of aging American Indians. We found that sleep characteristics derived from polysomnography predicted cognitive performance assessed 10 years later. Aspects of sleep were correlated with cognitive performance in nearly all domains investigated by the neuropsychological tests – episodic verbal learning, short-delay and long-delay free recall, processing speed, verbal phonemic fluency, and 3MSE general cognition (Table 4). Furthermore, after adjustment for age, BMI, sex, study site, years of education, CESD depressive symptoms score, and presence of the APOE e4 allele, longer sleep latency was associated with increased likelihood of cognitive impairment later in life.
Sleep-related disorders have previously been associated with cognitive decline (Suh et al., 2018; Mei et al., 2023), dementia (Robbins et al., 2021a,b), and neurodegeneration (Mullins et al., 2020). Our findings support our initial hypothesis that sleep characteristics are correlated with subsequent cognitive performance in American Indians, such that future cognitive deficits may be predictable from polysomnographically recorded sleep. Longer sleep latency in particular was associated with worse cognitive outcomes, which is consistent with findings in the general population (Suh et al., 2018; Robbins et al., 2021a). Notably, in a nationally representative longitudinal study with 2,812 Medicare beneficiaries in the United States, more than 30 min of sleep latency as well as very short sleep duration (≤5 h) were associated with incident dementia after 5 years (Robbins et al., 2021a). And in a study comprising 2,238 individuals with normal cognition and 655 individuals with mild cognitive impairment in Korea, more than 30 min of sleep latency was associated with increased risk of cognitive decline at 4-year follow-up (Suh et al., 2018). In our study, 23.7% of the sample had more than 30 min of sleep latency. Longer sleep latency was associated with poorer cognitive scores on the COWA test of verbal phonemic fluency – and, importantly, with increased likelihood of cognitive impairment, as defined by more than 1.5 standard deviations below the mean on the 3MSE.
We also found that higher oxygen saturation at baseline was associated with worse total learning, short-delay free recall, and long-delay free recall performance, as measured by the CVLT-SF as well as the WAIS-IV digit symbol coding test at follow-up. These findings were unexpected and inconsistent with what many general population studies have found (Cohen-Zion et al., 2001; Aloia et al., 2003; Spira et al., 2008; Yamout et al., 2012). In our study, surprisingly, associations for all variables related to oxygen saturation and cognitive performance indicated that greater severity of oxygen desaturation was associated with better cognitive performance. We have some hypotheses for these findings. It is possible that participation in the SHHS stimulated awareness of sleep disruptions, particularly in participants meeting criteria for sleep treatment. Thus, those with worse rates of oxygen saturation at baseline may have sought sleep treatment during the approximately 10-year interval between the SHHS and the CDCAI study and, with treatment, improved their cognitive performance. It is also possible that those who had low average oxygen saturation during the SHHS study did not survive to follow up or were too debilitated to participate in the CDCAI study, causing a bias in the results and leaving those who had good average oxygen saturation in the CDCAI study to be relatively more cognitively impaired. However, it is also important to note that the associations between oxygen saturation and cognition in our sample are not statistically robust and may not generalize to other samples. Moreover, since the literature on sleep and cognition in indigenous population is scarce, we used a discovery-based approach with a non-conservative correction for multiple comparisons (FDR), and therefore, we need to consider potential type 1 error. Future studies with larger samples of American Indians that are focused on cognitive disruptions related to sleep are needed to clarify this.
Our study found an influence of study site on associations of sleep with cognitive impairment, even when considering differences in time interval among participants. This raises questions regarding geographical location and its environmental impact or the possibility of inconsistency among sites. All sites included in the study for both the SHHS and CDCAI studies underwent the same training and certification to administer the cognitive tests and perform home polysomnography. The quality of polysomnograms was monitored through central scoring and quality assurance procedures, with no differences observed among study sites (Redline et al., 1998). Nevertheless, future studies should ensure methodological rigidity and consistency of the collected measurements to investigate whether the geographic region of different tribes and communities may have a substantive impact on the associations between sleep quality and cognitive performance.
Our findings highlight the importance of considering subjective and objective measures of sleep in research on sleep and cognition. Different ethnic and racial populations may subjectively experience sleep quality in different ways. In the present study, quantitative measures using polysomnography were better at capturing cognitive performance 10 years later in this population. Given that many determinants of sleep health are modifiable and that sleep is an important population-wide health indicator, public interventions in sleep health can have an impact on mitigating health disparities (Hale et al., 2020). Sleep disorders may be a modifiable risk factor for physical and mental health concerns later in life, including dementia (van Straten et al., 2018; Blackman et al., 2021). As a population facing significant health disparities (Hutchinson and Shin, 2014; Manson and Buchwald, 2021), American Indians have been shown to be at risk for vascular disease (Suchy-Dicey et al., 2017) and cognitive decline (de Souza-Talarico et al., 2016; Cholerton et al., 2019), but studies investigating sleep in this population have been lacking. The current study helps to fill this gap. Documenting sleep characteristics in aging American Indians and determining their relationship with cognitive outcomes provides direction for testing more specific hypotheses in this population, such as whether there is a predictive relationship between mid-life sleep latency and subsequent neurodegeneration, whether sleep treatments would be beneficial for long-term cognitive performance, and whether any deleterious effects of racial and ethnic sleep health disparities are involved.
There is a dearth of studies investigating whether sleep patterns in indigenous populations are different than those in other populations. A study involving 31,724 participants (7% Native Hawaiian and Pacific Inlanders, 14% Black individuals, and 79% White individuals) reported that suboptimal sleep duration (less than 6 h per day) occurred more frequently in native Hawaiian and Pacific Inlanders compared to White individuals and Black individuals and was linked to hypertension and diabetes, even after controlling for other variables (Matthews et al., 2018). In our study 41.7% of participants slept less than 6 h per day, and 74.8% slept less than 7 h per day, which is a substantially greater proportion than what has been reported in non-indigenous populations (Kocevska et al., 2021; Sabia et al., 2021). Our sample had a mean sleep duration of 6.19 h (with a SD of 1.23). A meta-analysis with the general population showed that mean sleep efficiency for those 41–65 and > 65 years of age ranged from 88 to 90%, depending on the age group (Kocevska et al., 2021), while in our study, mean sleep efficiency was 77.4% (Table 2). Differences in sample characteristics prevent us from comparing directly between studies. Nonetheless, our data encourage further effort to understand sleep disparities in American Indians. Although there is scarce literature on sleep in American Indians, a systematic review among Indigenous populations in high-income countries indicated that there is a higher incidence of obstructive sleep apnea, and that sleep apnea severity is greater in indigenous compared to non-indigenous populations. A higher prevalence of obesity, medical comorbidity, and health disparities appears to underlie this difference (Woods et al., 2015). A recent study including American Indians, Alaska Natives, Hispanics, and non-Hispanic White older adults with ADRD in Washington State highlighted that difficulty accessing healthcare may explain some of the health disparities (Amiri et al., 2024). The relationship between sleep disorders, cognition and race is complex and likely involves multiple genetic, behavioral and environmental factors. The relationship between sleep and the aging process of older adults from native communities is still unknown. Nonetheless, it is likely that interventions to improve sleep would be beneficial irrespective of race and ethnicity (Egan et al., 2017).
Limitations of the study include a modest sample size and potential bias in our sample. Specifically, the mean 3MSE score for our sample was higher than the mean for the entire CDCAI cohort (Mascarenhas Fonseca et al., 2023), suggesting that there may have been some selection bias, with underrepresentation of individuals with more pronounced cognitive impairment in our sample. Furthermore, we do not have data on whether participants sought sleep treatment during the interval between studies, or regarding reasons for non-participation in the CDCAI study. As such, sample bias needs to be considered when interpreting our findings.
A restricted number of neuropsychological tests was used in the CDCAI study, and there was no baseline cognitive assessment. Sleep and sleep loss effects on cognition are multi-dimensional (Oonk et al., 2008), and important aspects of cognitive performance may have been missed. Moreover, whereas there is broad consensus on how to measure and describe the quantity of sleep in a standardized and reproduceable way (Terzano et al., 2002; Fiorillo et al., 2019; Malhotra et al., 2021), there is debate about the best variables to use when describing sleep quality and the severity of sleep-disordered breathing (Redline et al., 2000; Tucker et al., 2007; Davis et al., 2011). Thus, our selection of sleep and cognition variables may have influenced the results. The lack of sleep assessments at the time of the neuropsychological assessments limits our understanding of whether any changes in sleep during the interval between the SHHS and CDCAI studies (whether from aging, disease, or sleep treatments) may have influenced our findings. And a generalized interpretation of the results was hampered by a lack of clinical diagnoses of sleep disorders and of dementia or prodromal dementia. Furthermore, the PSG recording montage used in SHHS did not include a nasal pressure sensor, which decreases the sensitivity of detecting hypopneic events. However, the impact thereof is not differential and would bias any findings related to sleep disordered breathing to the null rather than the converse. Further, the relatively small sample size hindered the investigation of specific analyses such as a stratified approach for APOE e4 carriers or sex.
Importantly, even though we found that sleep characteristics measured in mid-life predicted cognitive performance and cognitive impairment approximately 10 years later, that does not necessarily mean that the sleep characteristics in mid-life were causally involved. There is a bidirectional relationship between sleep and cognition, and there may be a common effect in play that links them here. Other factors not included in this study could be driving the observed associations. There is an extensive body of literature indicating that poor sleep may have a profound impact on brain functioning with advancing age (Lim et al., 2014; Borges et al., 2019), and it is likely that this played a role in the present findings as well, but our study design did not allow us to demonstrate that definitively.
This is the first study to analyze relationships between sleep and cognitive measures in an aging sample of American Indians. We reported novel findings regarding the associations between sleep characteristics, derived from polysomnography and questionnaires, and cognitive performance assessed approximately 10 years later. We found that polysomnography-derived sleep characteristics, but not self-reported sleep measures, collected in mid-life were associated with deficits in cognitive performance in this population later in life. In particular, longer sleep latency was associated with cognitive impairment approximately 10 years later. If further research indicates that sleep latency may serve as a biomarker of cognitive decline with advanced aging, it could provide a straightforward and important tool for early intervention. As American Indians have increased risk for AD and other age-related disorders (Centers for Disease Control and Prevention, 2006; Hutchinson and Shin, 2014; Suchy-Dicey et al., 2017), understanding how poor sleep affects American Indians health across the lifespan is paramount. Sleep and sleep disorders are possible modifiable risk factors for cognitive impairment and dementia, and therefore, are suitable candidates for interventions aimed at preventing neurodegenerative disease development and progression.
The data supporting the findings of this study are the intellectual property of the sovereign tribes and communities from whom they were collected and are not directly shareable without the express permission and consent of those entities. Researchers interested in using the data may follow standard Strong Heart Study procedures, per tribal data use agreements. Further inquiries can be directed to the corresponding author.
Ethical review and approval were granted for the Strong Heart Study and its ancillary studies. Written informed consent was obtained from all participants. Study procedures for this specific analysis of deidentified data were approved by the appropriate Institutional Research Boards, Tribal Research Review Boards, or Tribal Councils.
LF: Writing – review & editing, Writing – original draft, Software, Methodology, Investigation, Formal analysis, Conceptualization. MF: Writing – review & editing. NC: Writing – review & editing. NM: Writing – review & editing. DB: Writing – review & editing. HVD: Writing – review & editing. SQ: Writing – review & editing, Supervision. AS-D: Writing – review & editing, Supervision.
The author(s) declare financial support was received for the research, authorship, and/or publication of this article. The Strong Heart Study has been funded in whole or in part with federal funds from the National Institutes of Health, under contract numbers 75N92019D00027, 75N92019D00028, 75N92019D00029, and 75N92019D00030; cooperative agreements U01HL41642, U01HL41652, U01HL41654, U01HL65520, and U01HL65521; and research grants R01HL109315, R01HL109301, R01HL109284, R01HL109282, and R01HL109319. The SHHS study has been funded in part from the National Heart, Lung, and Blood Institute under grant numbers U01HL53938 (University of Arizona) and U01HL63429 (Missouri Breaks Research Institute). The CDCAI study has been funded in whole or in part with federal funds from the National Institutes of Health (R01HL093086, P50AG005136, U54MD000507, and K01AG057821). LF is currently supported by an Alzheimer’s Association grant (AARFD-21-851373) and by the National Institute on Aging of the National Institutes of Health (Award Number K99AG082864). HVD’s contribution to this work was supported by Army Research Office grant W911NF-21-S-0008. The content is solely the responsibility of the authors and does not necessarily represent the official views of the National Institutes of Health, the Department of Defense, or the Alzheimer’s Association.
SQ serves and receives compensation as a consultant for Teledoc, Bryte Foundation, Jazz Pharmaceuticals, and Whispersom, is a member of the Data Safety and Monitoring Board for clinical trials sponsored by Apnimed, and is the Field Chief Editor for Frontiers in Sleep. HVD serves and receives compensation as a consultant for Jazz Pharmaceuticals.
The remaining authors declare that the research was conducted in the absence of any commercial or financial relationships that could be construed as a potential conflict of interest.
All claims expressed in this article are solely those of the authors and do not necessarily represent those of their affiliated organizations, or those of the publisher, the editors and the reviewers. Any product that may be evaluated in this article, or claim that may be made by its manufacturer, is not guaranteed or endorsed by the publisher.
Aloia, M. S., Ilniczky, N., Di Dio, P., Perlis, M. L., Greenblatt, D. W., and Giles, D. E. (2003). Neuropsychological changes and treatment compliance in older adults with sleep apnea. J. Psychosom. Res. 54, 71–76. doi: 10.1016/s0022-3999(02)00548-2
Amiri, S., Keffeler, J. I., Crain, D. R., Denney, J. T., and Buchwald, D. (2024). Racial, ethnic, and rural disparities in distance to physicians among decedents with Alzheimer's disease and related dementias in Washington State. Alzheimers Dement. doi: 10.1002/alz.13756 (Epub ahead of print).
Bateman, R. J., Wen, G., Morris, J. C., and Holtzman, D. M. (2007). Fluctuations of CSF amyloid-beta levels: implications for a diagnostic and therapeutic biomarker. Neurology 68, 666–669. doi: 10.1212/01.wnl.0000256043.50901.e3
Blackman, J., Swirski, M., Clynes, J., Harding, S., Leng, Y., and Coulthard, E. (2021). Pharmacological and non-pharmacological interventions to enhance sleep in mild cognitive impairment and mild Alzheimer's disease: a systematic review. J. Sleep Res. 30:e13229. doi: 10.1111/jsr.13229
Bonanni, E., Maestri, M., Tognoni, G., Fabbrini, M., Nucciarone, B., Manca, M. L., et al. (2005). Daytime sleepiness in mild and moderate Alzheimer's disease and its relationship with cognitive impairment. J. Sleep Res. 14, 311–317. doi: 10.1111/j.1365-2869.2005.00462.x
Borges, C. R., Poyares, D., Piovezan, R., Nitrini, R., and Brucki, S. (2019). Alzheimer's disease and sleep disturbances: a review. Arq. Neuropsiquiatr. 77, 815–824. doi: 10.1590/0004-282X20190149
Buysse, D. J., Reynolds, C. F., Monk, T. H., Berman, S. R., and Kupfer, D. J. (1989). The Pittsburgh sleep quality index: a new instrument for psychiatric practice and research. Psychiatry Res. 28, 193–213. doi: 10.1016/0165-1781(89)90047-4
Centers for Disease Control and Prevention (CDC) (2003). Diabetes prevalence among American Indians and Alaska natives and the overall population--United States, 1994-2002. MMWR Morb. Mortal Wkly. Rep. 52, 702–704.
Centers for Disease Control and Prevention (CDC) (2006). Diagnosed diabetes among American Indians and Alaska Natives aged <35 years--United States, 1994-2004. MMWR Morb. Mortal Wkly. Rep. 55, 1201–1203.
Chen, X., Wang, R., Zee, P., Lutsey, P. L., Javaheri, S., Alcántara, C., et al. (2015). Racial/ethnic differences in sleep disturbances: the multi-ethnic study of atherosclerosis (MESA). Sleep 38, 877–888. doi: 10.5665/sleep.4732
Choe, Y. M., Byun, M. S., Yi, D., Lee, J. H., Jeon, S. Y., Sohn, B. K., et al. (2019). Sleep experiences during different lifetime periods and in vivo Alzheimer pathologies. Alzheimers Res. Ther. 11:79. doi: 10.1186/s13195-019-0536-6
Cholerton, B., Omidpanah, A., Verney, S. P., Nelson, L. A., Baker, L. D., Suchy-Dicey, A., et al. (2019). Type 2 diabetes and later cognitive function in older American Indians: the strong heart study. Int. J. Geriatr. Psychiatry 34, 1050–1057. doi: 10.1002/gps.5108
Cohen-Zion, M., Stepnowsky, C., Marler, S. T., Kripke, D. F., and Ancoli-Israel, S. (2001). Changes in cognitive function associated with sleep disordered breathing in older people. J. Am. Geriatr. Soc. 49, 1622–1627. doi: 10.1046/j.1532-5415.2001.t01-1-49270.x
Davis, C. J., Clinton, J. M., Jewett, K. A., Zielinski, M. R., and Krueger, J. M. (2011). Delta wave power: an independent sleep phenotype or epiphenomenon? J. Clin. Sleep Med. 7, S16–S18. doi: 10.5664/JCSM.1346
de Souza-Talarico, J. N., de Carvalho, A. P., Brucki, S. M., Nitrini, R., and Ferretti-Rebustini, R. E. (2016). Dementia and cognitive impairment prevalence and associated factors in indigenous populations: a systematic review. Alzheimer Dis. Assoc. Disord. 30, 281–287. doi: 10.1097/WAD.0000000000000140
Delis, D. C., Kramer, J. H., Kaplan, E., and Ober, B. A. C. V. L. T. (2000). California verbal learning test. 2nd Edn. San Antonio, TX: Psychological Corporation.
Denney, J. T., Zamora-Kapoor, A., Hansen, D. A., and Whitney, P. (2023). Race/ethnicity, sleep duration, and mortality risk in the United States. SSM Popul. Health 21:101350. doi: 10.1016/j.ssmph.2023.101350
Drager, L. F., McEvoy, R. D., Barbe, F., Lorenzi-Filho, G., and Redline, S.INCOSACT Initiative (International Collaboration of Sleep Apnea Cardiovascular Trialists) (2017). Sleep apnea and cardiovascular disease: lessons from recent trials and need for team science. Circulation 136, 1840–1850. doi: 10.1161/CIRCULATIONAHA.117.029400
Egan, K. J., Knutson, K. L., Pereira, A. C., and von Schantz, M. (2017). The role of race and ethnicity in sleep, circadian rhythms and cardiovascular health. Sleep Med. Rev. 33, 70–78. doi: 10.1016/j.smrv.2016.05.004
Eichenbaum, H. (2004). Hippocampus: cognitive processes and neural representations that underlie declarative memory. Neuron 44, 109–120. doi: 10.1016/j.neuron.2004.08.028
El-Sheikh, M., Philbrook, L. E., Kelly, R. J., Hinnant, J. B., and Buckhalt, J. A. (2019). What does a good night's sleep mean? Nonlinear relations between sleep and children's cognitive functioning and mental health. Sleep 42:zsz078. doi: 10.1093/sleep/zsz078
Elwood, P. C., Bayer, A. J., Fish, M., Pickering, J., Mitchell, C., and Gallacher, J. E. (2011). Sleep disturbance and daytime sleepiness predict vascular dementia. J. Epidemiol. Community Health 65, 820–824. doi: 10.1136/jech.2009.100503
Fiorillo, L., Puiatti, A., Papandrea, M., Ratti, P. L., Favaro, P., Roth, C., et al. (2019). Automated sleep scoring: a review of the latest approaches. Sleep Med. Rev. 48:101204. doi: 10.1016/j.smrv.2019.07.007
Foley, D., Monjan, A., Masaki, K., Ross, W., Havlik, R., White, L., et al. (2001). Daytime sleepiness is associated with 3-year incident dementia and cognitive decline in older Japanese-American men. J. Am. Geriatr. Soc. 49, 1628–1632. doi: 10.1046/j.1532-5415.2001.t01-1-49271.x
Folstein, M. F., Folstein, S. E., and McHugh, P. R. (1975). "Mini-mental state". A practical method for grading the cognitive state of patients for the clinician. J. Psychiatr. Res. 12, 189–198. doi: 10.1016/0022-3956(75)90026-6
Fonseca, L. M., Mattar, G. P., Haddad, G. G., Burduli, E., McPherson, S. M., Guilhoto, L. M. F. F., et al. (2021). Neuropsychiatric symptoms of Alzheimer’s disease in down syndrome and its impact on caregiver distress. J. Alzheimers Dis. 81, 137–154. doi: 10.3233/JAD-201009
Fultz, N. E., Bonmassar, G., Setsompop, K., Stickgold, R. A., Rosen, B. R., Polimeni, J. R., et al. (2019). Coupled electrophysiological, hemodynamic, and cerebrospinal fluid oscillations in human sleep. Science 366, 628–631. doi: 10.1126/science.aax5440
Gharbi-Meliani, A., Dugravot, A., Sabia, S., Regy, M., Fayosse, A., Schnitzler, A., et al. (2021). The association of APOE ε4 with cognitive function over the adult life course and incidence of dementia: 20 years follow-up of the Whitehall II study. Alzheimers Res. Ther. 13:5. doi: 10.1186/s13195-020-00740-0
Hale, L., Troxel, W., and Buysse, D. J. (2020). Sleep health: an opportunity for public health to address health equity. Annu. Rev. Public Health 41, 81–99. doi: 10.1146/annurev-publhealth-040119-094412
Harris, R., Nelson, L. A., Muller, C., and Buchwald, D. (2015). Stroke in American Indians and Alaska natives: a systematic review. Am. J. Public Health 105, e16–e26. doi: 10.2105/AJPH.2015.302698
Hope, T., Keene, J., Gedling, K., Fairburn, C. G., and Jacoby, R. (1998). Predictors of institutionalization for people with dementia living at home with a carer. Int. J. Geriatr. Psychiatry 13, 682–690. doi: 10.1002/(sici)1099-1166(1998100)13:10<682::aid-gps847>3.0.co;2-y
Hutchinson, R. N., and Shin, S. (2014). Systematic review of health disparities for cardiovascular diseases and associated factors among American Indian and Alaska native populations. PLoS One 9:e80973. doi: 10.1371/journal.pone.0080973
Ju, G., Yoon, I. Y., Lee, S. D., Kim, T. H., Choe, J. Y., and Kim, K. W. (2012). Effects of sleep apnea syndrome on delayed memory and executive function in elderly adults. J. Am. Geriatr. Soc. 60, 1099–1103. doi: 10.1111/j.1532-5415.2012.03961.x
Kalliny, M., and McKenzie, J. G. (2017). Occupational health and sleep issues in underserved populations. Prim. Care 44, e73–e97. doi: 10.1016/j.pop.2016.09.010
Kocevska, D., Lysen, T. S., Dotinga, A., Koopman-Verhoeff, M. E., Luijk, M. P. C. M., Antypa, N., et al. (2021). Sleep characteristics across the lifespan in 1.1 million people from the Netherlands, United Kingdom and United States: a systematic review and meta-analysis. Nat. Hum. Behav. 5, 113–122. doi: 10.1038/s41562-020-00965-x
Lim, M. M., Gerstner, J. R., and Holtzman, D. M. (2014). The sleep-wake cycle and Alzheimer's disease: what do we know? Neurodegener. Dis. Manag. 4, 351–362. doi: 10.2217/nmt.14.33
Liu, J., Ghastine, L., Um, P., Rovit, E., and Wu, T. (2021). Environmental exposures and sleep outcomes: a review of evidence, potential mechanisms, and implications. Environ. Res. 196:110406. doi: 10.1016/j.envres.2020.110406
Lyketsos, C. G., Lopez, O., Jones, B., Fitzpatrick, A. L., Breitner, J., and DeKosky, S. (2002). Prevalence of neuropsychiatric symptoms in dementia and mild cognitive impairment: results from the cardiovascular health study. JAMA 288, 1475–1483. doi: 10.1001/jama.288.12.1475
Malhotra, A., Ayappa, I., Ayas, N., Collop, N., Kirsch, D., Mcardle, N., et al. (2021). Metrics of sleep apnea severity: beyond the apnea-hypopnea index. Sleep 44:zsab030. doi: 10.1093/sleep/zsab030
Manson, S. M., and Buchwald, D. S. (2021). Aging and health of American Indians and Alaska natives: contributions from the native investigator development program. J. Aging Health 33, 3S–9S. doi: 10.1177/08982643211014399
Mascarenhas Fonseca, L., Sage Chaytor, N., Olufadi, Y., Buchwald, D., Galvin, J. E., Schmitter-Edgecombe, M., et al. (2023). Intraindividual cognitive variability and magnetic resonance imaging in aging American Indians: data from the strong heart study. J. Alzheimers Dis. 91, 1395–1407. doi: 10.3233/JAD-220825
Matthews, E. E., Li, C., Long, C. R., Narcisse, M. R., Martin, B. C., and McElfish, P. A. (2018). Sleep deficiency among native Hawaiian/Pacific Islander, Black, and White Americans and the association with cardiometabolic diseases: analysis of the National Health Interview Survey Data. Sleep Health 4, 273–283. doi: 10.1016/j.sleh.2018.01.004
McCoy, J. G., and Strecker, R. E. (2011). The cognitive cost of sleep lost. Neurobiol. Learn. Mem. 96, 564–582. doi: 10.1016/j.nlm.2011.07.004
Mei, X., Zhao, Z., Qiu, Z., Wang, J., Yu, H., and Zheng, C. (2023). Association of sleep disorders with clinical symptoms and age in Chinese older adult patients with and without cognitive decline. Front. Aging Neurosci. 15:1189837. doi: 10.3389/fnagi.2023.1189837
Merlino, G., Piani, A., Gigli, G. L., Cancelli, I., Rinaldi, A., Baroselli, A., et al. (2010). Daytime sleepiness is associated with dementia and cognitive decline in older Italian adults: a population-based study. Sleep Med. 11, 372–377. doi: 10.1016/j.sleep.2009.07.018
Moran, M., Lynch, C. A., Walsh, C., Coen, R., Coakley, D., and Lawlor, B. A. (2005). Sleep disturbance in mild to moderate Alzheimer's disease. Sleep Med. 6, 347–352. doi: 10.1016/j.sleep.2004.12.005
Mullins, A. E., Kam, K., Parekh, A., Bubu, O. M., Osorio, R. S., and Varga, A. W. (2020). Obstructive sleep apnea and its treatment in aging: effects on Alzheimer's disease biomarkers, cognition, brain structure and neurophysiology. Neurobiol. Dis. 145:105054. doi: 10.1016/j.nbd.2020.105054
Muto, V., Koshmanova, E., Ghaemmaghami, P., Jaspar, M., Meyer, C., Elansary, M., et al. (2020). Alzheimer's disease genetic risk and sleep phenotypes: association with more slow-waves and daytime sleepiness. Sleep 44:zsaa137. doi: 10.1093/sleep/zsaa137
Nelson, K. L., Davis, J. E., and Corbett, C. F. (2022). Sleep quality: an evolutionary concept analysis. Nurs. Forum 57, 144–151. doi: 10.1111/nuf.12659
Ohayon, M. M., and Vecchierini, M. F. (2002). Daytime sleepiness and cognitive impairment in the elderly population. Arch. Intern. Med. 162, 201–208. doi: 10.1001/archinte.162.2.201
Oonk, M., Tucker, A., Belenky, G., and Van Dongen, H. (2008). Excessive sleepiness: determinants, outcomes, and context. Int. J. Sleep Wakefulness. 1, 141–147,
Pandi-Perumal, S. R., Abumuamar, A. M., Spence, D. W., Chattu, V. K., Moscovitch, A., and BaHammam, A. S. (2017). Racial/ethnic and social inequities in sleep medicine: the tip of the iceberg? J. Natl. Med. Assoc. 109, 279–286. doi: 10.1016/j.jnma.2017.04.005
Petersen, R. C., Caracciolo, B., Brayne, C., Gauthier, S., Jelic, V., and Fratiglioni, L. (2014). Mild cognitive impairment: a concept in evolution. J. Intern. Med. 275, 214–228. doi: 10.1111/joim.12190
Petersen, R. C., Doody, R., Kurz, A., Mohs, R. C., Morris, J. C., Rabins, P. V., et al. (2001). Current concepts in mild cognitive impairment. Arch. Neurol. 58, 1985–1992. doi: 10.1001/archneur.58.12.1985
Prasad, B., Saxena, R., Goel, N., and Patel, S. R. (2018). Genetic ancestry for sleep research: leveraging health inequalities to identify causal genetic variants. Chest 153, 1478–1496. doi: 10.1016/j.chest.2018.03.024
Quan, S. F., Howard, B. V., Iber, C., Kiley, J. P., Nieto, F. J., O'Connor, G. T., et al. (1997). The sleep heart health study: design, rationale, and methods. Sleep 20, 1077–1085
Quan, S. F., O’Connor, G. T., Quan, J. S., Redline, S., Resnick, H. E., Shahar, E., et al. (2007). Association of physical activity with sleep-disordered breathing. Sleep Breath. 11, 149–157. doi: 10.1007/s11325-006-0095-5
Quan, S. F., Wright, R., Baldwin, C. M., Kaemingk, K. L., Goodwin, J. L., Kuo, T. F., et al. (2006). Obstructive sleep apnea-hypopnea and neurocognitive functioning in the sleep heart health study. Sleep Med. 7, 498–507. doi: 10.1016/j.sleep.2006.02.005
Radloff, L. S. (1977). The CES-D scale: a self-report depression scale for research in the general population. Appl. Psychol. Meas. 1, 385–401. doi: 10.1177/014662167700100306
Rechtschaffen, A., and Kales, A. (1968). A manual of standardized terminology, techniques and scoring system for sleep stages of human subjects. Washington, DC: Public Health Service, US Government Printing Office.
Redline, S., Kapur, V. K., Sanders, M. H., Quan, S. F., Gottlieb, D. J., Rapoport, D. M., et al. (2000). Effects of varying approaches for identifying respiratory disturbances on sleep apnea assessment. Am. J. Respir. Crit. Care Med. 161, 369–374. doi: 10.1164/ajrccm.161.2.9904031
Redline, S., Sanders, M. H., Lind, B. K., Quan, S. F., Iber, C., Gottlieb, D. J., et al. (1998). Methods for obtaining and analyzing unattended polysomnography data for a multicenter study. Sleep heart Health Research Group. Sleep 21, 759–767
Robbins, R., Quan, S. F., Weaver, M. D., Bormes, G., Barger, L. K., and Czeisler, C. A. (2021a). Examining sleep deficiency and disturbance and their risk for incident dementia and all-cause mortality in older adults across 5 years in the United States. Aging 13, 3254–3268. doi: 10.18632/aging.202591
Robbins, R., Weaver, M. D., Barger, L. K., Wang, W., Quan, S. F., and Czeisler, C. A. (2021b). Sleep difficulties, incident dementia and all-cause mortality among older adults across 8 years: findings from the National Health and aging trends study. J. Sleep Res. 30:e13395. doi: 10.1111/jsr.13395
Ruff, R. M., Light, R. H., Parker, S. B., and Levin, H. S. (1996). Benton controlled oral word association test: reliability and updated norms. Arch. Clin. Neuropsychol. 11, 329–338. doi: 10.1093/arclin/11.4.329
Ruiter, M. E., DeCoster, J., Jacobs, L., and Lichstein, K. L. (2010). Sleep disorders in African Americans and Caucasian Americans: a meta-analysis. Behav. Sleep Med. 8, 246–259. doi: 10.1080/15402002.2010.509251
Ruiter, M. E., Decoster, J., Jacobs, L., and Lichstein, K. L. (2011). Normal sleep in African-Americans and Caucasian-Americans: a meta-analysis. Sleep Med. 12, 209–214. doi: 10.1016/j.sleep.2010.12.010
Sabia, S., Fayosse, A., Dumurgier, J., van Hees, V. T., Paquet, C., Sommerlad, A., et al. (2021). Association of sleep duration in middle and old age with incidence of dementia. Nat. Commun. 12:2289. doi: 10.1038/s41467-021-22354-2
Schlosser Covell, G. E., Dhawan, P. S., Lee Iannotti, J. K., Hoffman-Snyder, C. R., Wellik, K. E., Caselli, R. J., et al. (2012). Disrupted daytime activity and altered sleep-wake patterns may predict transition to mild cognitive impairment or dementia: a critically appraised topic. Neurologist 18, 426–429. doi: 10.1097/NRL.0b013e318272f7ef
Shin, H. Y., Han, H. J., Shin, D. J., Park, H. M., Lee, Y. B., and Park, K. H. (2014). Sleep problems associated with behavioral and psychological symptoms as well as cognitive functions in Alzheimer's disease. J. Clin. Neurol. 10, 203–209. doi: 10.3988/jcn.2014.10.3.203
Spira, A. P., Blackwell, T., Stone, K. L., Redline, S., Cauley, J. A., Ancoli-Israel, S., et al. (2008). Sleep-disordered breathing and cognition in older women. J. Am. Geriatr. Soc. 56, 45–50. doi: 10.1111/j.1532-5415.2007.01506.x
Suchy-Dicey, A. M., Shibata, D., Best, L. G., Verney, S. P., Longstreth, W. T. Jr., Lee, E. T., et al. (2016). Cranial magnetic resonance imaging in elderly American Indians: design, methods, and implementation of the cerebrovascular disease and its consequences in American Indians study. Neuroepidemiology 47, 67–75. doi: 10.1159/000443277
Suchy-Dicey, A. M., Shibata, D. K., Madhyastha, T. M., Grabowski, T. J., Longstreth, W. T., and Buchwald, D. S. (2017). Findings of vascular brain injury and structural loss from cranial magnetic resonance imaging in elderly American Indians: the strong heart study. Neuroepidemiology 48, 39–47. doi: 10.1159/000459624
Suh, S. W., Han, J. W., Lee, J. R., Byun, S., Kwon, S. J., Oh, S. H., et al. (2018). Sleep and cognitive decline: a prospective nondemented elderly cohort study. Ann. Neurol. 83, 472–482. doi: 10.1002/ana.25166
Tarokh, L., Saletin, J. M., and Carskadon, M. A. (2016). Sleep in adolescence: physiology, cognition and mental health. Neurosci. Biobehav. Rev. 70, 182–188. doi: 10.1016/j.neubiorev.2016.08.008
Teng, E. L., and Chui, H. C. (1987). The modified mini-mental state (3MS) examination. J. Clin. Psychiatry 48, 314–318
Terzano, M. G., Parrino, L., Smerieri, A., Chervin, R., Chokroverty, S., Guilleminault, C., et al. (2002). Atlas, rules, and recording techniques for the scoring of cyclic alternating pattern (CAP) in human sleep. Sleep Med. 3, 187–199. doi: 10.1016/s1389-9457(02)00003-5
Tsapanou, A., Gu, Y., O'Shea, D., Eich, T., Tang, M. X., Schupf, N., et al. (2016). Daytime somnolence as an early sign of cognitive decline in a community-based study of older people. Int. J. Geriatr. Psychiatry 31, 247–255. doi: 10.1002/gps.4318
Tsapanou, A., Scarmeas, N., and Stern, Y. (2020). Sleep and the aging brain. A multifaceted approach. Sleep Sci 13, 152–156. doi: 10.5935/1984-0063.20190128
Tucker, A. M., Dinges, D. F., and Van Dongen, H. P. (2007). Trait interindividual differences in the sleep physiology of healthy young adults. J. Sleep Res. 16, 170–180. doi: 10.1111/j.1365-2869.2007.00594.x
van Straten, A., van der Zweerde, T., Kleiboer, A., Cuijpers, P., Morin, C. M., and Lancee, J. (2018). Cognitive and behavioral therapies in the treatment of insomnia: a meta-analysis. Sleep Med. Rev. 38, 3–16. doi: 10.1016/j.smrv.2017.02.001
Walker, M. P. (2009). The role of sleep in cognition and emotion. Ann. N. Y. Acad. Sci. 1156, 168–197. doi: 10.1111/j.1749-6632.2009.04416.x
Woods, C. E., Usher, K., and Maguire, G. P. (2015). Obstructive sleep apnoea in adult indigenous populations in high-income countries: an integrative review. Sleep Breath. 19, 45–53. doi: 10.1007/s11325-014-1032-7
Yamout, K., Goldstein, F. C., Lah, J. J., Levey, A. I., and Bliwise, D. L. (2012). Neurocognitive correlates of nocturnal oxygen desaturation in a memory clinic population. J. Clin. Exp. Neuropsychol. 34, 325–332. doi: 10.1080/13803395.2011.642849
Zhao, Q. F., Tan, L., Wang, H. F., Jiang, T., Tan, M. S., Tan, L., et al. (2016). The prevalence of neuropsychiatric symptoms in Alzheimer's disease: systematic review and meta-analysis. J. Affect. Disord. 190, 264–271. doi: 10.1016/j.jad.2015.09.069
Keywords: Native Americans, health disparities, sleep quality, cognitive impairment, polysomnography
Citation: Fonseca LM, Finlay MG, Chaytor NS, Morimoto NG, Buchwald D, Van Dongen HPA, Quan SF and Suchy-Dicey A (2024) Mid-life sleep is associated with cognitive performance later in life in aging American Indians: data from the Strong Heart Study. Front. Aging Neurosci. 16:1346807. doi: 10.3389/fnagi.2024.1346807
Received: 29 November 2023; Accepted: 23 April 2024;
Published: 05 June 2024.
Edited by:
Mirnova E. Ceide, Montefiore Health System, United StatesReviewed by:
Carey E. Gleason, University of Wisconsin-Madison, United StatesCopyright © 2024 Fonseca, Finlay, Chaytor, Morimoto, Buchwald, Van Dongen, Quan and Suchy-Dicey. This is an open-access article distributed under the terms of the Creative Commons Attribution License (CC BY). The use, distribution or reproduction in other forums is permitted, provided the original author(s) and the copyright owner(s) are credited and that the original publication in this journal is cited, in accordance with accepted academic practice. No use, distribution or reproduction is permitted which does not comply with these terms.
*Correspondence: Luciana Mascarenhas Fonseca, bHVjaWFuYS5mb25zZWNhQHdzdS5lZHU=
Disclaimer: All claims expressed in this article are solely those of the authors and do not necessarily represent those of their affiliated organizations, or those of the publisher, the editors and the reviewers. Any product that may be evaluated in this article or claim that may be made by its manufacturer is not guaranteed or endorsed by the publisher.
Research integrity at Frontiers
Learn more about the work of our research integrity team to safeguard the quality of each article we publish.