- 1Department of MRI, The First Affiliated Hospital of Zhengzhou University, Zhengzhou, Henan, China
- 2Engineering Technology Research Center for Detection and Application of Brain Function of Henan Province, Zhengzhou, Henan, China
- 3Engineering Research Center of Medical Imaging Intelligent Diagnosis and Treatment of Henan Province, Zhengzhou, Henan, China
- 4Department of Radiology, Tianjin Key Laboratory of Functional Imaging, Tianjin Medical University General Hospital, Tianjin, China
- 5Department of Radiology, Tianjin Huanhu Hospital, Tianjin, China
- 6Department of Interventional Radiology, The First Affiliated Hospital of Zhengzhou University, Zhengzhou, China
- 7MR Research China, GE Healthcare, Beijing, China
Introduction: The objective of this study was to characterize the alteration patterns of dynamic spatiotemporal activity in chronic subcortical stroke patients with varying motor outcomes, while investigating the imaging indicators relevant to the assessment of potential cognitive deficits in these patients.
Methods: A total of 136 patients and 88 normal controls were included in the analysis of static and dynamic intrinsic brain activity, determined by amplitude of low-frequency fluctuations.
Results: The findings unveiled that subcortical stroke patients exhibited significantly aberrant temporal dynamics of intrinsic brain activity, involving regions within multiple brain networks. These spatiotemporal patterns were found to be contingent upon the side of the lesion. In addition, these aberrant metrics demonstrated potential in discerning cognitive deficits in stroke patients with memory impairment, with the dynamic indices exerting more influence than the static ones. The observe findings may indicate that subcortical stroke can trigger imbalances in the segregation and integration of spatiotemporal patterns across the entire brain with multi-domain networks, especially in patients with poor motor outcomes.
Conclusion: It suggests that the temporal dynamics indices of intrinsic brain activity could serve as potential imaging indicators for assessing cognitive impairment in patients with chronic subcortical stroke, which may be associated with the motor outcomes.
Introduction
Motor and cognitive impairments are prevalent after stroke (Zhao et al., 2018; Shekhar et al., 2021; Chen et al., 2022), even in patients with mild strokes (Einstad et al., 2021). Compared to motor function injuries, cognitive function injuries have the characteristics of being concealed and delayed, resulting in patients with cognitive impairment not seeking medical treatment promptly. Especially the occurrence of mild cognitive impairment in chronic stage is frequently ignored by patients families, and may gradually develop into irreversible severe cognitive decline. Additionally, patients with motor function impairments may also suffer from concurrent cognitive impairments, specifically memory and executive function (Van Der Werf et al., 2003; Delavaran et al., 2017). This may be attributed to the fact that the brain is a complex network consisting of multiple subnetworks serving different functions (Wang et al., 2014). A structural lesion may disrupt the optimal balance between integration and segregation in the entire brain, thereby significantly contributing to the widespread abnormalities in network coherence associated with behavioral deficits.
Memory is considered a fundamental in human cognitive activities. It plays a crucial role in various cognitive processes, including learning, problem-solving, reasoning, intellectual performance, other significant aspects of human cognition (Mecklinger, 2010). Memory allows us to learn from past events, make decisions, and navigate through our daily lives (Mecklinger, 2010). The absence of memory would significantly hinder our capacity to acquire new knowledge, identify individuals, retrieve crucial information, or even maintain self-awareness. The previous studies have revealed that the decline in memory has a significant impact on the long-term cognitive functions in patients after a stroke (Van Der Werf et al., 2003; Al-Qazzaz et al., 2014; Gorelick and Nyenhuis, 2015; de Lima Ferreira et al., 2019). In this study, we mainly explored the characteristics of memory impairment and the change patterns of brain activity in stroke patients with different motor outcomes for identification and prevention of concurrent impair with behavior decline.
Research has demonstrated the significance of regional spontaneous neural characteristics in comprehending neuropathological and neurophysiological conditions (Tsai et al., 2014). The analysis of amplitude of low-frequency fluctuations (ALFF) is a reliable and reproducible resting-state functional magnetic resonance imaging (rs-fMRI) technique utilized for measurement of regional spontaneous neuronal activity (Zang et al., 2007). The ALFF has been widely used to locate abnormal functional activities associated with various brain disorders by calculating the amplitude of low-frequency oscillation of each voxel (Zang et al., 2007; Cao et al., 2018; Li et al., 2019; Cui et al., 2020; Wu et al., 2023). Prior study have illustrated that local properties (i.e., ALFF) play a crucial role in comprehending the neural pathology associated with stroke (Quan et al., 2022). The identification of localized activity through ALFF in a distinct cortical region may hold particular significance in reflecting specific aspects of functional processes within the network (Rubinov and Sporns, 2010; Wang P. et al., 2019). However, the traditional rs-fMRI technique studies are usually focused on static metrics (e.g., static ALFF, sALFF), which may overlook the valuable information within the temporal features of neural fluctuations. The activity of the brain is inherently dynamic, which is reflected in its temporal variability. Dynamic ALFF (dALFF) is derived from time-variant intrinsic brain activity, effectively highlights aberrant functional activity in psychological and cognitive disorders. The dALFF metric takes into account not only the average amplitude of these low-frequency fluctuations but also their temporal variability. By examining the dynamic changes in the ALFF, we can gain insights into the dynamic characteristics of brain activity and its relationship with various cognitive processes and neurological disorders. Previous studies have demonstrated that patients with stroke exhibit dynamic alterations in spontaneous neural activity across multiple brain networks (Guo et al., 2019; Ma et al., 2021; Wang et al., 2022), indicating the potential utility of dynamic local metrics as predictive tools for functional outcomes in stroke patients. This dynamic attribute emphasizes the materiality of an intact neuroanatomical connectome in facilitating effective information exchange among interconnected regions.
Additionally, the impact of stroke on cognition was closely associated with the lesion-side (Biesbroek et al., 2017; Zhao et al., 2018). It was found that there is heterogeneity in both brain structure and function of stroke patients with different lesion-sides (Diao et al., 2017; Wang C. et al., 2019). This may be attributed to the fact that despite exhibiting nearly identical structural characteristics in the left and right hemispheres, they exhibit significant functional disparities. Specifically, the localization of specific behavioral functions in one hemisphere is a salient feature of the human brain known as its dominant hemisphere. The left hemisphere is typically dominant in the majority of adults due to right-handedness. Consequently, variations in stroke-side of the dominant or non-dominant hemispheres may give rise to diverse characteristics and degrees of impairment in behavioral functionality, accompanied by heterogeneous damage and reorganization processes affecting both brain structure and function. However, it still lacks a comprehensive understanding of the spatiotemporal patterns in intrinsic brain activity related to memory impairment among patients with varying motor outcomes on different lesion sides.
In this study, we utilize static and dynamic metrics (i.e., sALFF, dALFF) to characterize the spatiotemporal patterns of alteration in chronic subcortical stroke patients with different motor outcomes. In addition, to investigate the characterize of significant heterogeneity in memory indicators and internal brain activity patterns in patients with different lesion-sides compared to healthy control group. The relationship between these indicators and memory impairment was also investigated, identifying reliable imaging markers for evaluation of potential memory dysfunction in these patients with diverse motor outcomes.
Materials and methods
Participants
The experimental protocol received approval from the medical research ethics committees of the First Affiliated Hospital of Zhengzhou University, Tianjin Medical University General Hospital, and Tianjin Huanhu Hospital. Additionally, it received approval and pre-registration from the Chinese Clinical Trial Registry (ChiCTR1900027064). Every participant provided written informed consent.
The inclusion criteria for stroke patients were as follows: (a) first-onset ischemic stroke with motor deficits in both upper and lower extremities at the onset of stroke; (b) a single lesion involving the motor pathway, such as at the level of the internal capsule or pontine, in stroke patients; and (c) right-handedness prior to stroke onset. Exclusion criteria included: (a) recurrent stroke based on clinical history and MRI evaluation; (b) any other brain abnormalities detected on MR images; (c) white matter hyperintensities greater than 1 were assessed using the modified Fazekas score (Fazekas et al., 1987); and (d) a history of any other neurological or psychiatric disorders.
The sample size estimation was conducted using G* Power software (version 3.1). Parameters were set as follows: two-tailed statistical test for the difference between two independent means, effect size d = 0.8, alpha error probability = 0.05, power = 0.95, and allocation ratio = 1. The calculated sample size of each group was 42, and a total of 136 eligible patients consented to participate in this study. Among them, there were 71 patients with left-hemisphere stroke (50 at the level of the internal capsule and 21 at the level of the pontine) and 65 patients with right-hemisphere stroke (46 at the level of the internal capsule and 19 at the level of the pontine). No randomization was performed to allocate subjects and no blinding were performed, as only two groups of subjects (patient and normal control) was recruited in this study. Additionally, the enrolled subjects were chronic ischemic stroke and did not undergo specific treatment protocols. 88 individuals without any medical history were recruited as normal controls (NC). A lesion incidence map of stroke patients is presented in Figure 1. In detail, the localization of the stroke was determined by two experienced neuroradiologists using three-dimensional T1-weighted images (3D-T1WI). Initially, we standardized the 3D-T1WI to the Montreal Neurological Institute (MNI) space as a reference. Subsequently, we manually delineated the lesions slice by slice on the normalized 3D-T1WI utilize MRIcron software.1 This process resulted in generating a lesion mask for each participant. Finally, the lesion masks of stroke patients were superimposed onto the MNI template to create a lesion incidence map of stroke patients.
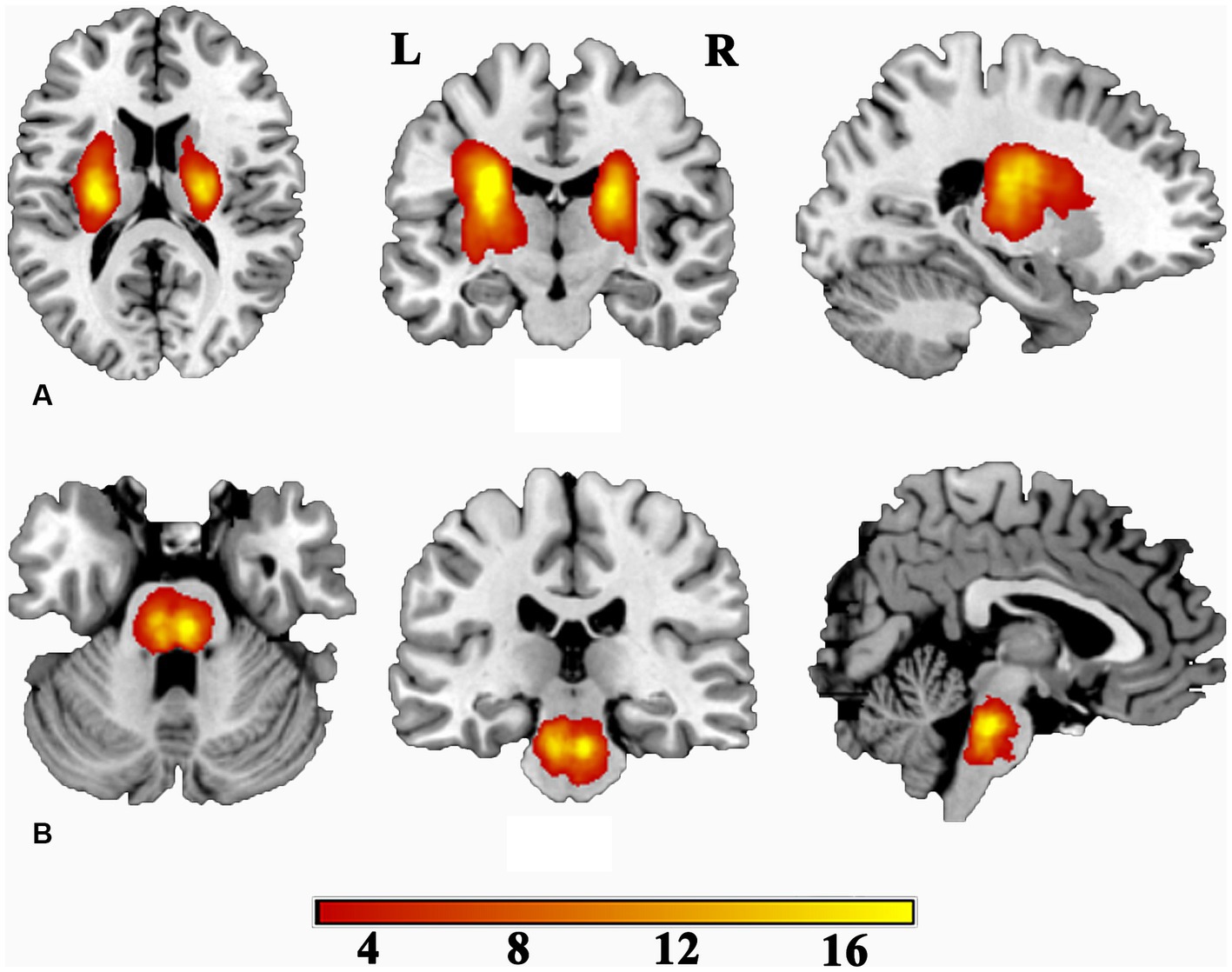
Figure 1. Lesion incidence map for patients with subcortical stroke. (A) For patients with stroke at the level of the internal capsule. (B) For patients with stroke at the level of the pontine. L, left; R, right. Color indicates the lesion incidence frequency.
MRI data acquisition
MRI data were acquired from participants using 3.0-Tesla MR scanners at three hospitals, namely The First Affiliated Hospital of Zhengzhou University and Tianjin Medical University General Hospital (both equipped with a Discovery MR750 scanners from General Electric, Milwaukee, WI), and Tianjin Huanhu Hospital (equipped with a Magnetom Trio Tim MR scanner from Siemens, Erlangen, Germany). All participants were instructed to keep their eyes closed and remain still during the scanning procedure. Multi-parametric MRI including diffusion-weighted images (DWI), T1- and T2-weighted image (T1WI and T2WI), and T2 fluid-attenuated inversion recovery (T2-FLAIR) images were acquired to facilitate identification of the stroke lesion, recurrent stroke, white matter hyperintensity, and other brain abnormalities.
The images of subjects acquired from both Discovery MR750 scanners were obtained using identical parameters. For the MR750 scanners, rs-fMRI data were acquired using a gradient echo single-shot echo-planar imaging sequence with the following parameters: time of repetition (TR)/time of echo (TE) = 2,000/41 ms; field of view (FOV) = 220 mm × 220 mm; matrix size = 64 × 64; flip angle = 90°; slice thickness = 4 mm with a gap of 0.5 mm between slices; total number of slices = 32; and time points acquired per subject was set to be 180. Sagittal 3D-T1WI were utilized to acquire anatomical images, with TR/TE = 8.2/3.2 ms; FOV = 256 mm × 256 mm; matrix = 256 × 256; slice thickness = 1.0 mm, no gap; and a total of 188 slices resulting in isotropic voxel measuring at a resolution of 1-mm3. For the Trion Tim scanner, rs-fMRI data were acquired using an echo-planar imaging sequence with TR/TE = 2,000/30 ms, flip angle = 90°, FOV = 220 mm × 100 mm, matrix size = 64 × 64, thickness = 3 mm, gap = 1 mm, slices = 36, and volumes = 180. The 3D-T1WI was acquired using the magnetization prepared rapid acquisition gradient echo (MPRAGE) sequence with the following imaging parameters: TR/TE = 2,000/2.26 ms, flip angle = 9°, FOV = 256 mm × 232 mm, matrix = 256 × 232, thickness = 1 mm, and slices = 192, resulting in a 1-mm3 isotropic voxel.
Neurological assessments
The National Institutes of Health Stroke Scale (NIHSS) was employed for the evaluation of global neurological deficits. Fugl-Meyer assessment of the whole extremity (WE_FM) was conducted to assess motor deficits in the chronic subcortical stroke patients, encompassing both upper- and lower-extremity motor function evaluation. According to the WE_FM scores, the patients were categorized into two subgroups: partial recovery (PR, WE_FM < 100) and complete recovery (CR, WE_FM = 100) (Zhang et al., 2014; Liu et al., 2023). The Rey Auditory Verbal Learning Test (RAVLT) was used to evaluate memory function (Ferreira Correia and Campagna Osorio, 2014), encompassing both verbal short-term memory (VSTM) and verbal long-term memory (VLTM).
fMRI data processing
All pre-processing was performed using the Data Processing & Analysis of Brain Imaging (DPABI) toolbox (Yan et al., 2016),2 with the following steps: (1) the first 10 time points from each subjects were discarded to ensure steady-state magnetization; (2) the remaining time points were corrected to consistent image timing across slices; (3) a realignment procedure for head-motion correction was performed to exclude a part of participant data exhibiting head motion >2.0 mm or translation >2.0° rotation. Additionally, scrubbing was implemented with a frame-wise displacement threshold of 0.5 in order to reduce motion-related artifacts; (4) the functional images were subsequently normalized to the standard Montreal Neurological Institute (MNI) templates using Diffeomorphic Anatomical Registration Through Exponentiated Lie algebra (DARTEL), followed by resampling to a voxel size of 3 mm × 3 mm × 3 mm; (5) detrending the data; (6) conducting linear regression analysis to regress out the effects of several covariates; and (7) applying temporal band-pass filtering within frequency range of 0.01–0.08 Hz.
sALFF calculation
The sALFF was calculated using DPABI software in MATLAB. Specifically, the BOLD time series of a given voxel were first transformed into the frequency domain via a fast Fourier transform. The square root of each frequency in the power spectrum was then calculated and averaged across 0.01–0.08 Hz to obtain the sALFF value. Prior to statistical analyses, these values were z-transformed. All analyses were conducted at the whole-brain level.
dALFF variance calculation
The ALFF was proposed to characterize the resting-state functional activity of each voxel in the brain. The time series was initially transformed into the frequency domain, and subsequently, the ALFF was computed by measuring power within the low-frequency range of 0.01–0.08 Hz. A sliding window approach was employed to compute the dALFF using the Dynamic Brain Connectome (DynamicBC) toolbox (Liao et al., 2014).3 A window size of 30 TRs (60 s) and a window overlap of 90% were selected. To ensure the robustness of our findings, we conducted repeated analyses of dALFF using additional window lengths of 22 TRs and 50 TRs. For each window, an ALFF map was computed and the variance across all windows was used to quantify dynamic dALFF. The resulting dALFF maps were transformed into z-scores for statistical analysis.
Statistical analysis
The statistical analyses were performed using SPM 124 and GraphPad Prism.5 Data normality was assessed using the Shapiro–Wilk statistic, while differences in age between patients and the NC group were detected using a two-sample t-test with Welch’s correction. The non-parametric Mann–Whitney test was employed to discern disparities in educational attainment between the patient and NC cohorts. Fisher’s exact test was used to examine sex differences between the groups. A one-sample t-test was conducted to assess intra-group patterns of sALFF and dALFF maps. A general linear model (GLM) was employed to compare the difference in sALFF and dALFF maps between stroke patients and NC group, with age, sex, years of education, and neuroimage scanning sites as covariates. The height level family wise error (FWE) method was utilized for multiple comparison correction (p < 0.05, cluster size >20 voxels). The non-parametric Kruskal–Wallis test with Dunn’s multiple comparisons was employed to compare the dALFF values among the PR, CR, and NC groups (p < 0.05). Additionally, the dALFF values from the areas exhibiting significant inter-differences in patients were extracted for the analysis based on a region of interest (ROI). The Cohen’s d statistic (Parker and Hagan-Burke, 2007) was employed to quantify the effect size (ES) of inter-group differences. One-way ANOVA with Tukey’s multiple comparisons test was utilized to detect differences in RAVLT scores among the PR, CR, and NC groups (p < 0.05).
Spearman correlation analyses were performed to explore the relationship between dALFF variability in these regions of inter-group differences and memory scale scores with a Benjamini-Hochberg-Yekutieli (BY) FDR method (Benjamini and Yekutieli, 2001) for multiple comparison correction (p < 0.05). Finally, receiver operating characteristic (ROC) curve analysis (Mandrekar, 2010; Chen et al., 2018) was performed to investigate the potential value of dALFF variability indicators as an imaging biomarker for distinguishing stroke patients from healthy individuals. The Youden’s index J (J = sensitivity + specificity – 1) was utilized to identify the optimal cut-off that balances sensitivity and specificity.
Results
Clinical characteristics
The demographic, clinical, and behavioral characteristics are presented in Table 1. There were no significant inter-group differences in age between left-stroke patients and normal controls (p = 0.7209) or between right-stroke patients and normal controls (p = 0.4213). There were significant inter-group differences in sex between left-stroke patients and normal controls (p = 0.0195), whereas no significant inter-group differences in sex were observed between the right-stroke group and normal controls (p = 0.4078). There were significant inter-group differences in the years of education between the left-stroke group and normal controls (p = 0.0005) as well as between the right-stroke group and normal controls (p = 0.0021).
The RAVLT scores are displayed in Figure 2. The left-stroke patients with both PR (PVSTM < 0.0001, PVLTM = 0.0010) and CR (PVSTM = 0.0008, PVLTM = 0.0330) involvement exhibited poorer performance in the VSTM and VLTM tests compared to normal controls, while no significant difference was observed between the PR and CR groups (PVSTM = 0.0991, PVLTM = 0.4869). The right-stroke patients in the PR group exhibited significantly poorer performance on both the VSTM test (PVSTM < 0.0001) and the VLTM test (PVLTM < 0.0001) compared to normal controls, while there were no significant inter-group differences between the CR group and normal controls (PVSTM = 0.2111, PVLTM = 0.8076). Additionally, the right-stroke patients in the PR group performed significantly worse in both the VSTM test (PVSTM = 0.0103) and the VLTM test (PVLTM = 0.0002) compared to their counterparts in the CR group.
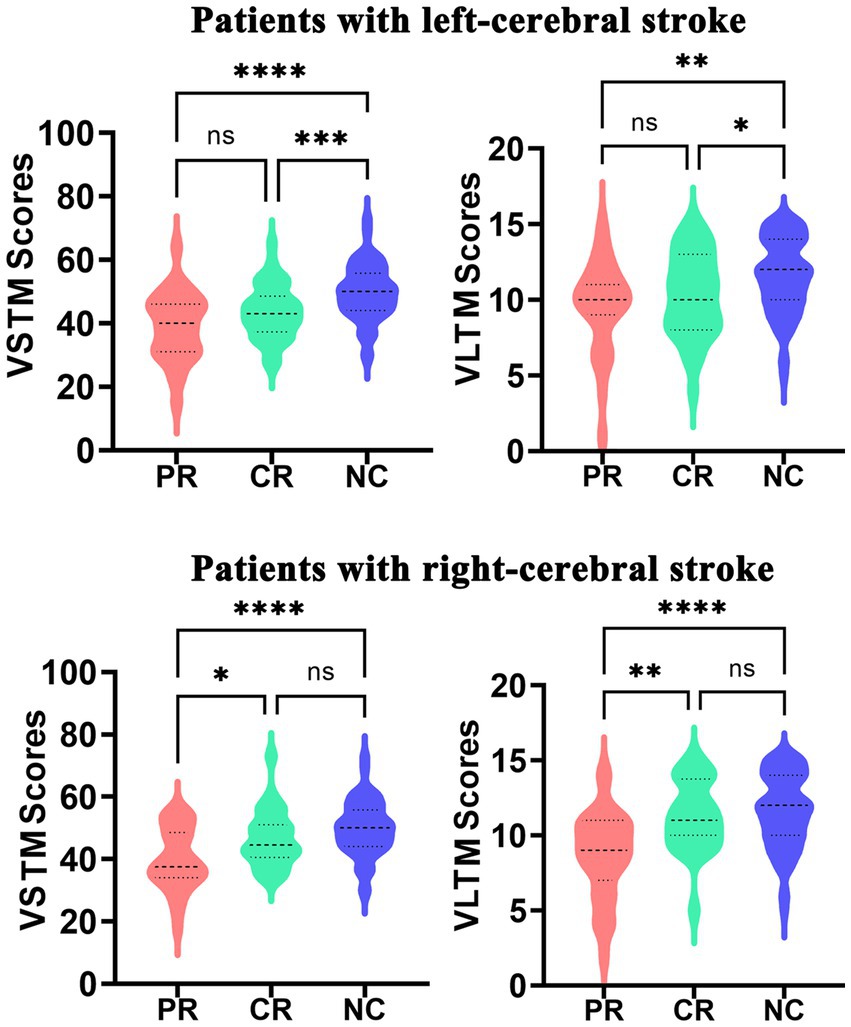
Figure 2. The significant inter-group differences in VLTM and VSTM among the PR, CR, and NC groups. CR, complete recovery; PR, partial recovery; NC, normal control; ns, none significant; VLTM, verbal long-term memory; VSTM, verbal short-term memory.
sALFF changes
Significant differences in sALFF were observed between the right-stroke and NC groups, while no significant differences were detected between the left-stroke and NC groups after multiple comparison correction (FWE, p < 0.05, cluster size >50). In detail, there were no significant differences observed between the left subcortical stroke patients and the NC group after multiple comparison correction (FWE, p < 0.05). However, in order to observe the trend of ALFF in the left-stroke patients more accurately, we performed a comparison without multiple comparison correction (uncorrected, p < 0.001, cluster size >50). As a result, it was found that the left-stroke patients had increased ALFF in the bilateral cuneus and bilateral precuneus regions, while decreased ALFF was observed in left inferior orbitofrontal cortex (Figure 3A). Compared to the normal control group, chronic stroke patients with right-hemisphere lesions exhibited significantly increased sALFF in left superior occipital gyrus and significantly decreased sALFF in right fusiform (Figure 3C).
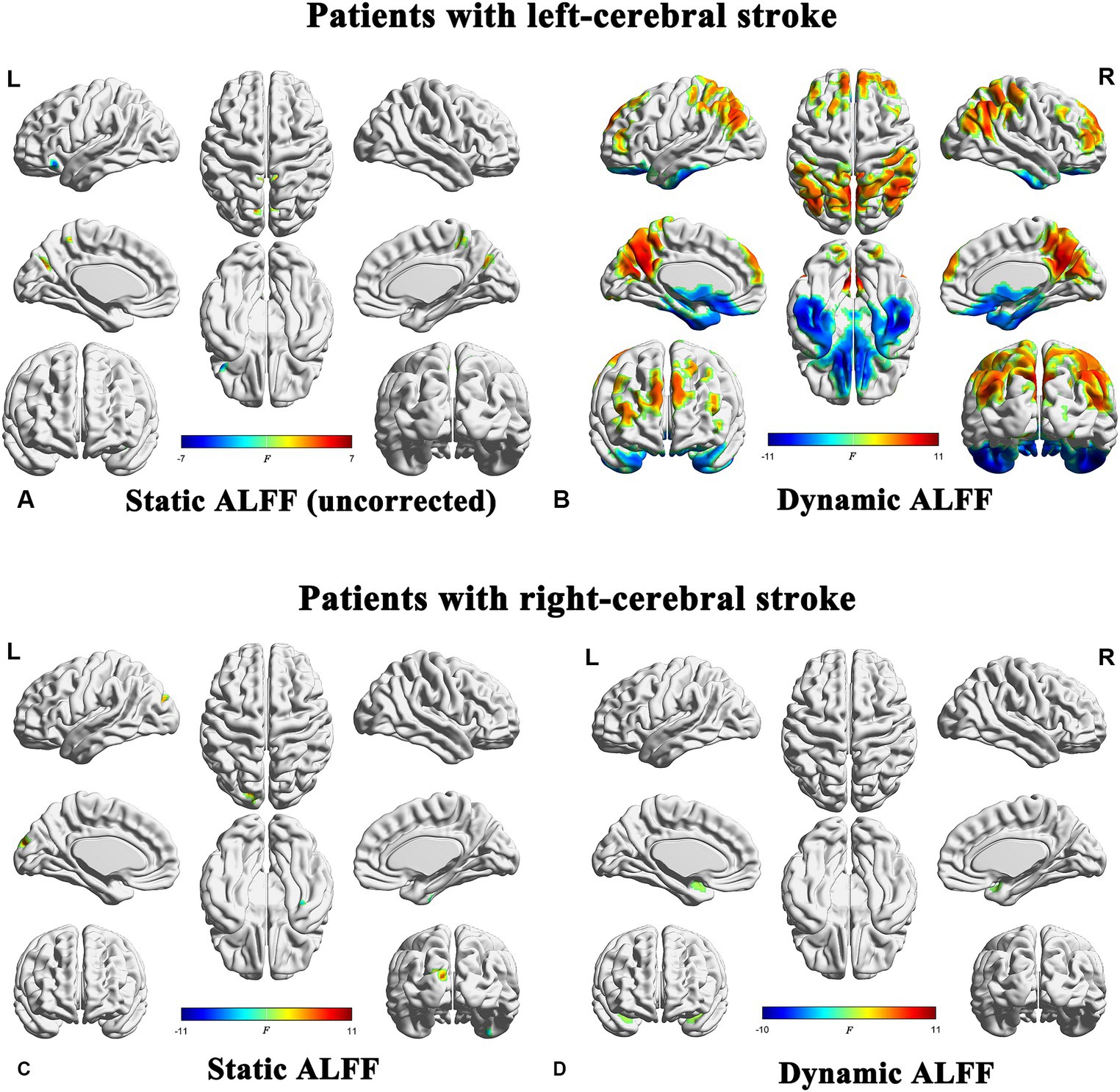
Figure 3. Brain regions showing significant differences in both sALFF and dALFF analyses between stroke patients and normal controls (FWE correction, p < 0.05). ALFF, amplitude of low-frequency fluctuations; L, left; R, right.
dALFF changes
The statistical significance of the differences in dALFF at a window length of 30 TRs between the stroke groups (both the left- and right-cerebral stroke) and the NC group was demonstrated in Figures 3B,D, 4B,E, while the significant inter-group differences among PR, CR, and NC were displayed in Figure 5 and Table 2. The validation results for both the left- and right-cerebral stroke, obtained using window lengths of 22 (Figures 4A,D) and 50 (Figures 4C,F) TRs respectively, are displayed in Figure 4.
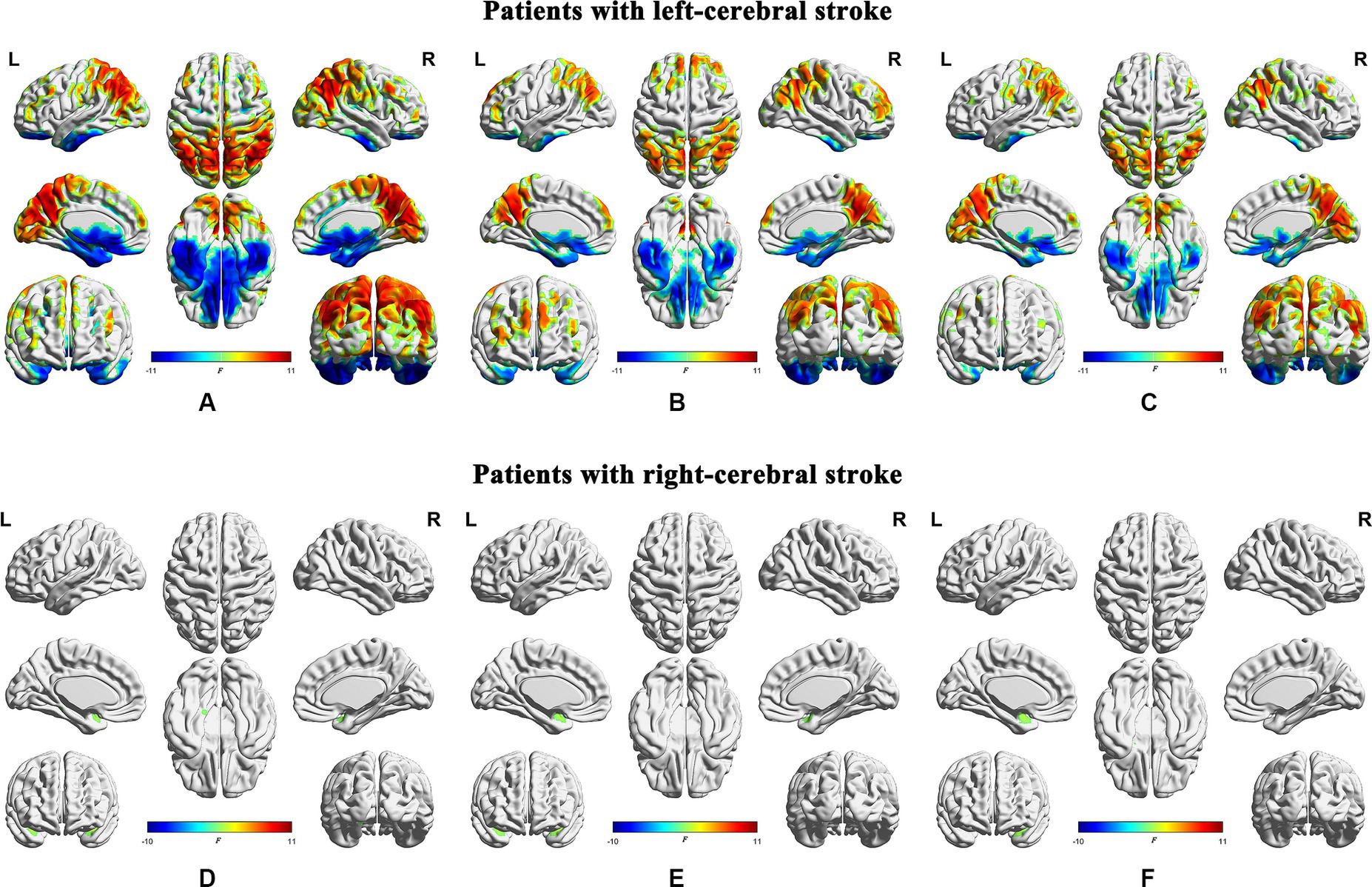
Figure 4. Validation results obtained with different window lengths. Compared with normal controls, patients with left-cerebral stroke showed significant dALFF variances, as illustrated in panels (A) (22 TRs), (B) (30 TRs), and (C) (50 TRs). By contrast, patients with right-cerebral stroke showed significant dALFF variances, as illustrated in panels (D) (22 TRs), (E) (30 TRs), and (F) (50 TRs) (FWE correction, p < 0.05). ALFF, amplitude of low-frequency fluctuations; L, left; R, right.
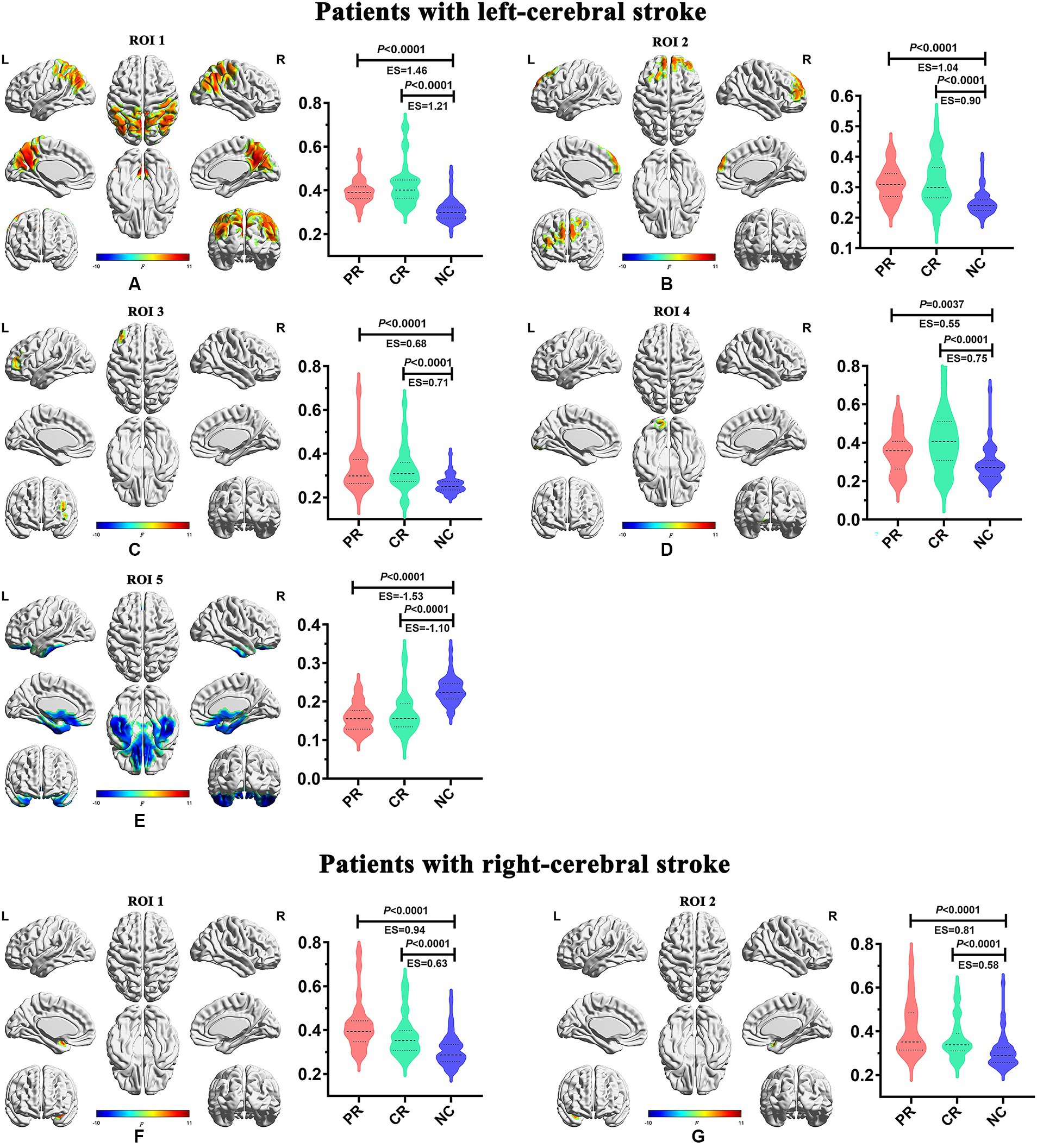
Figure 5. Inter-group differences of dynamic temporal variability among the PR, CR, and NC groups. CR, complete recovery; ES, effect size; L, left; NC, normal control; PR, partial recovery; R, right; ROI, region of interest.
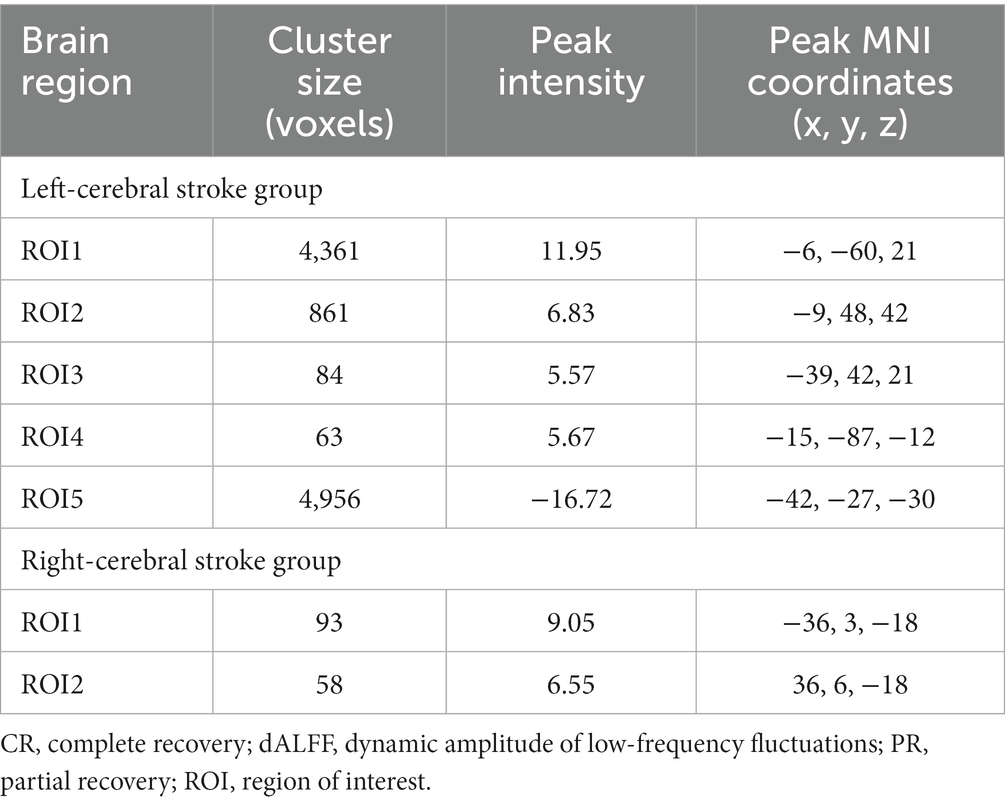
Table 2. Brain regions with significant inter-group differences in dALFF among PR, CR, and NC groups.
In the main results (with a window length of 30 TRs), the left-stroke patients displayed significantly increased dALFF in comparison to the NC group, primarily in regions associated with dorsal attention network (DAN) and sensorimotor network (SMN) (ROI1, Figure 5A), involving bilateral precuneus, bilateral angular gyrus, bilateral inferior parietal gyrus, bilateral cuneus, bilateral superior parietal gyrus, right supramarginal gyrus, bilateral superior and middle occipital gyrus, bilateral middle temporal gyrus, right superior temporal gyrus, bilateral middle and posterior cingulum gyrus, bilateral postcentral gyrus, right precentral gyrus, and bilateral paracentral lobule; as well as frontal network (FN), involving bilateral superior and middle frontal gyrus (ROI2, Figure 5B), left middle and inferior frontal gyrus (ROI3, Figure 5C), and left lingual gyrus (ROI4, Figure 5D), part of the visual network (VN). These patients also exhibited decreased dALFF, primarily in the regions of frontotemporal network (FTN) and basal ganglia network (BGN) (ROI5, Figure 5E), encompassing bilateral superior, middle and inferior orbitofrontal cortex, bilateral rectus gyrus, bilateral inferior temporal gyrus, bilateral superior and middle temporal poles, bilateral fusiform gyrus, bilateral parahippocampus, bilateral hippocampus, bilateral pallidum, putamen and caudate.
In comparison with the NC group, the right-stroke patients showed significantly increased dALFF in regions of left temporal-insula network (TIN; ROI1, Figure 5F), encompassing the left superior temporal pole, superior temporal gyrus, and left insula; as well as right TIN (ROI2, Figure 5G), including the right superior temporal pole, superior temporal gyrus, and right insula.
Correlation analysis
In left-cerebral stroke patients, a significant positive correlation was observed between the increased dALFF of ROI1 and VSTM (Figure 6A) in PR group (p = 0.014, r = 0.443), yet no correlation was found in either the CR (p = 0.107, r = 0.259) or NC (p = 0.988, r = 0.002) group. As well as, the increased dALFF of ROI1 had a significant positive correlated with VLTM (Figure 6B) both in PR (p = 0.002, r = 0.544) and CR (p = 0.036, r = 0.303) group, while no significant correlation demonstrated in NC group (p = 0.668, r = −0.047). Additionally, the decreased dALFF of ROI5 displayed a significant negative correlation with VSTM (Figure 6C) both in PR (p = 0.031, r = −0.394) and CR (p = 0.040, r = −0.327) group, but no correlation was found in NC group (p = 0.743, r = 0.036).
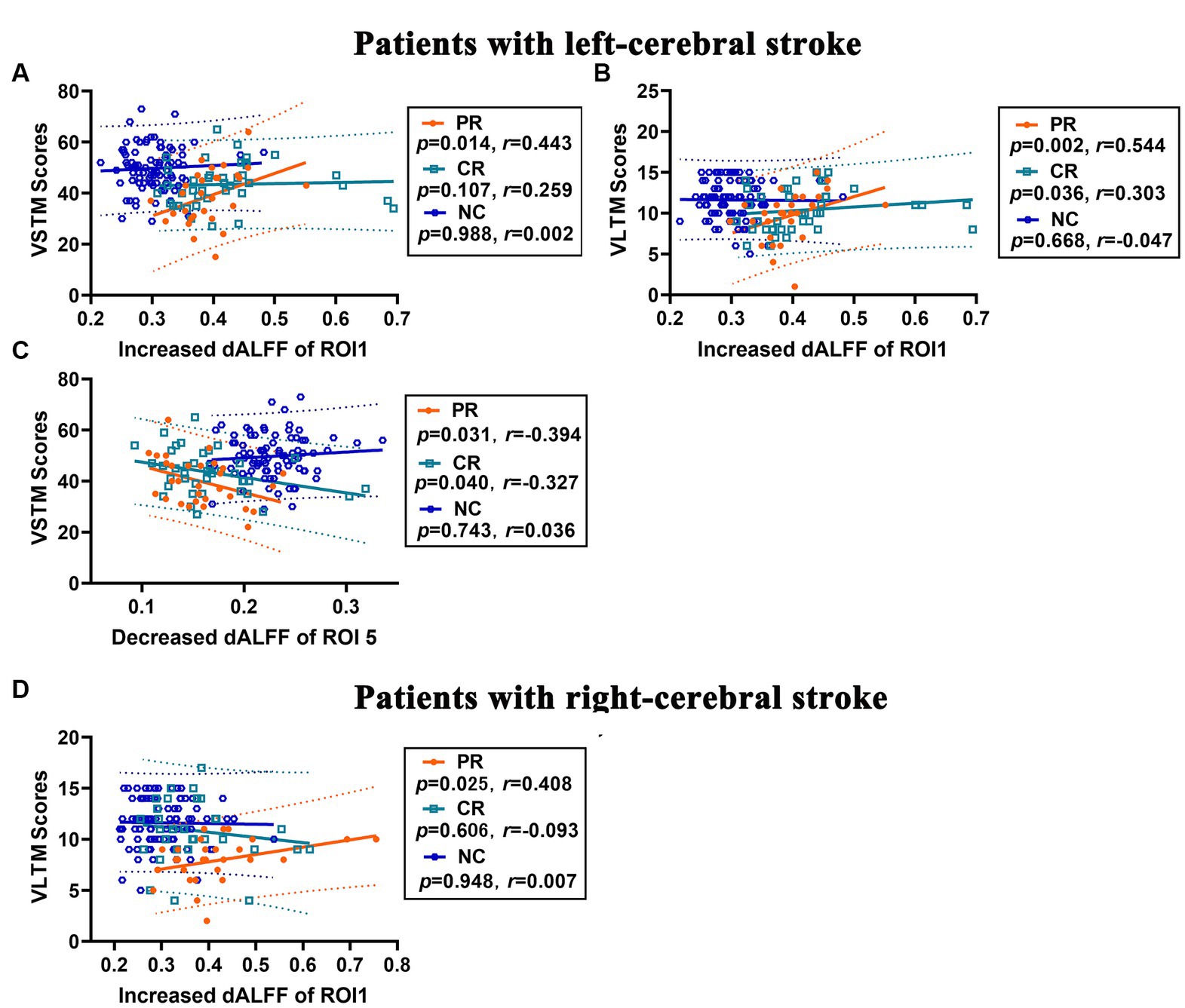
Figure 6. Correlation analysis between changes in dALFF and memory scores in stroke patients. (A–C) Correlations between increased dALFF of ROI1 and VSTM score (A) and VLTM score (B), and between decreased dALFF of ROI5 and VSTM score (C) in patients with left-cerebral stroke. (D) Correlations between increased dALFF of ROI1 and VLTM score in patients with right-cerebral stroke. dALFF, dynamic amplitude of low-frequency fluctuations; ROI, region of interest; VLTM, verbal long-term memory; VSTM, verbal short-term memory.
In the right-cerebral stroke patients, a significant positive correlation was observed between the increased dALFF values of ROI1 and VLTM scores (Figure 6D) in PR group (p = 0.025, r = 0.408), while no significant correlation was found in CR (p = 0.606, r = −0.093) and NC (p = 0.948, r = 0.007) group.
ROC curve analysis results
The left-stroke group exhibited an area under curve (AUC) value of 0.944 and 0.943 in ROI1 with increased dALFF variability for PR and CR patients, respectively (Figure 7A), while the AUC values were 0.930 and 0.891 in ROI5 with decreased dALFF for PR and CR patients, respectively (Figure 7B). In the right-stroke group, increased dALFF of ROI1 in both PR and CR patients demonstrated AUC values of 0.870 and 0.755, respectively (Figure 7C).
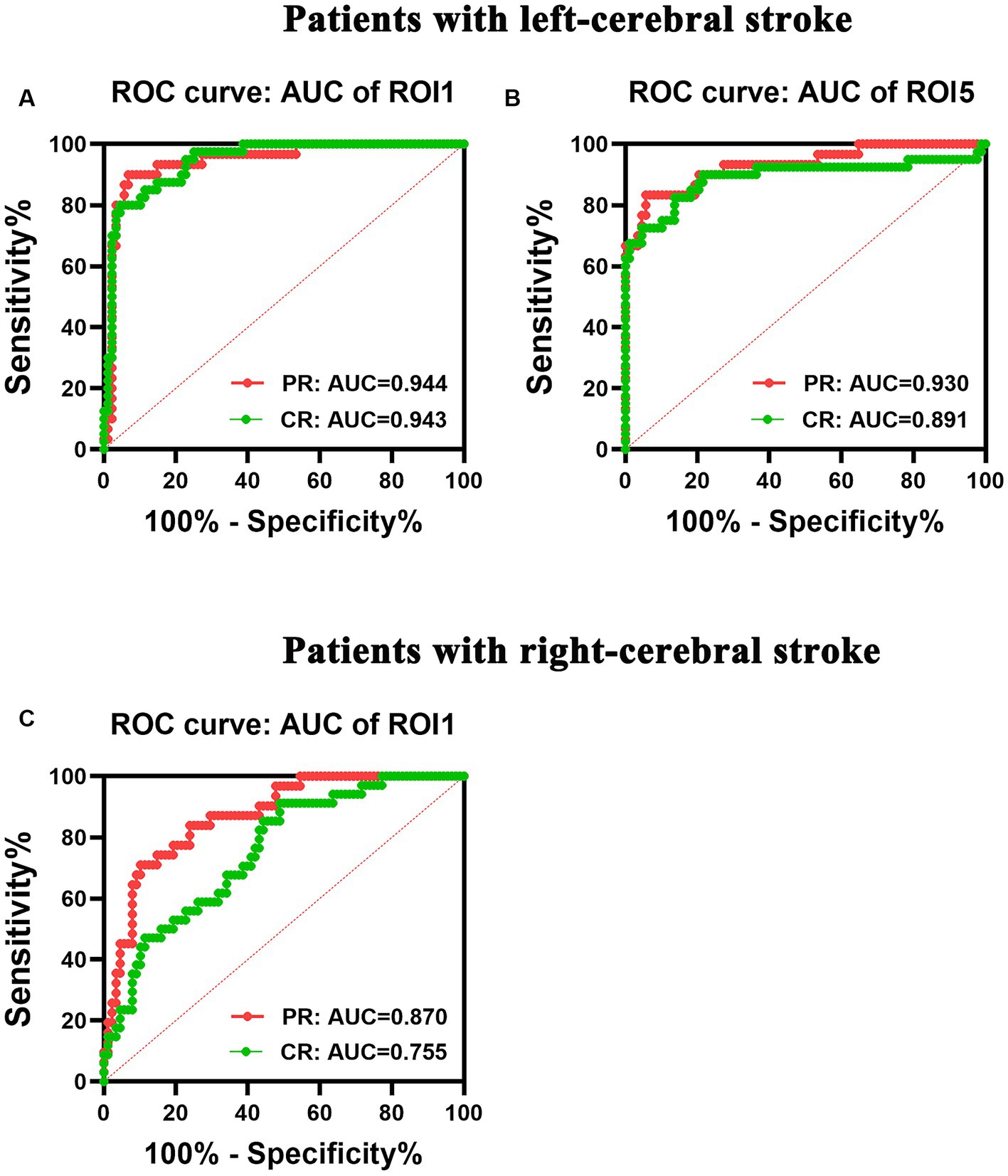
Figure 7. ROC analysis of the performance of changes in dALFF in patients with left- (A,B) and right-cerebral strokes (C). AUC, area under curve; CR, complete recovery; dALFF, dynamic amplitude of low-frequency fluctuations; PR, partial recovery; ROC, receiver operating characteristic; ROI, region of interest.
Discussion
In this study, we investigated the spatiotemporal patterns of intrinsic brain activity in subcortical stroke patients with different degrees of motor recovery, elucidating the correspondence between these metrics change and memory impairments. We observed that chronic subcortical stroke patients with motor pathways damage exhibited both short- and long-term memory impairments, particularly those who did not fully recover their motor function. This implies that the functional deficits induced by stroke in subcortical motor pathways affect not only the motor system but also the cognitive system. This is in line with the previous research indicating a reciprocal relationship between motor and cognitive impairments and repairs (Kannan et al., 2019; Einstad et al., 2021).
Our study revealed significant short-term and long-term memory impairments in both left- and right-cerebral stroke patients, with the memory impairment increasing as motor recovery worsened. Notably, particularly in left-cerebral subcortical stroke patients, substantial memory function impairment persists even in cases of good motor recovery. This finding aligns with a previous study conducted on mild strokes (Einstad et al., 2021) which revealed that around one-third of patients exhibited cognitive or motor deficits, along with concomitant impairment manifested in some survivors. Additionally, the author discovered a significant correlation between motor performance and deficits in memory. Generally, the capacity of short-term memory reflects the brain’s aptitude for rapidly assimilating and processing novel information and experiences, while long-term memory is associated with the ability to effectively encode and retrieve information over extended periods (Mecklinger, 2010). The memory functions are crucial for the daily functioning success of those stroke patients (Al-Qazzaz et al., 2014).
Compared to the normal controls, significant differences were observed in the characteristics of both static and dynamic spontaneous intrinsic brain activity throughout the entire brain in these patients who experienced partial recovery or complete recovery. It implies that chronic subcortical stroke patients, even those who have achieved good motor function recovery, still exhibit abnormal intrinsic brain activity at the global level. This may underlie their heightened vulnerability to cognitive dysfunction compared to age-matched controls. Additionally, this study found that the dynamic temporal variability exhibited greater sensitive than the static metrics in characterizing brain activity changes in patients with chronic subcortical stroke, particularly those with left-cerebral stroke. In detail, the static and temporal dynamic analyses showed similar alteration patterns of intrinsic brain activity in the right-stroke group. Nevertheless, no significant differences were observed in the static analysis in the left-stroke group when compared with the normal controls, whereas the patients displayed significant dynamic temporal variability involving the regions of multiple brain networks (FWE correction, p < 0.05). Notably, the tendency of change observed in the left-stroke patients was consistent with that revealed by the dynamic analysis when multiple comparison correction was not applied in the static analysis (uncorrected, p < 0.001). It suggest that temporal dynamic metrics are more effective in capturing uncontrolled yet recurring spontaneous intrinsic brain activity patterns than static indexes. It may be attributed to the static analysis assumes average quantities and does not account for the dynamic nature of brain activity in the resting state (Chang and Glover, 2010). However, the brain’s activity is inherently dynamic. This temporal dynamic variability is closely related to neural networks functionality and can reveal fluctuations in network interactions (Allen et al., 2014) and patterns of recurrent brain activity that are beyond the scope of static analyses (Liegeois et al., 2017; Qin et al., 2021). The findings of this study indicate that the dynamic neural properties metrics have greater potential than the static characteristics for diagnosing and predicting cerebral neurological function states in the stroke patients.
Notably, the dynamic temporal variability of neural circuits had a more significant impact in the left-stroke group than in the right-stroke group when compared to normal control group. This may be responsible for the greater memory dysfunction observed in patients with left subcortical strokes, even after adequate motor function recovery. Specifically, the left-stroke patients displayed significantly increased dynamic temporal variability of intrinsic brain activity across multiple brain networks, mainly in the regions of the dorsal attention network, sensorimotor network, frontal network, and visual network. In addition, they displayed significantly decreased dynamic temporal variability mainly in the regions of frontotemporal network and basal ganglia network. In contrast, the right-stroke patients only exhibited significantly increased dynamic temporal variability of intrinsic brain activity in the regions of bilateral temporal-insula networks. The activated intrinsic brain activity is associated with heightened neuronal excitability and metabolism, whereas reduced intrinsic brain activity signify inhibition of spontaneous neuronal activity. In the present study, the stroke patients displayed abnormalities in local temporal dynamic variability within the regions of primary perceptual networks (i.e., VN, SMN, BGN) and higher cognitive (i.e., FN, DAN, FPN, TIN) networks. These observations suggest that a subcortical stroke can trigger imbalances between the segregation and integration of spatiotemporal patterns of neural activity within the whole brain network. This finding is consistent with prior neuroimaging studies based on function and structure connection, which demonstrated that dysfunction induced by the subcortical stroke involves multiple-domain functional networks (Wang et al., 2014, 2021, 2022; Wang C. et al., 2019). The regions of advanced cognitive control network (i.e., FN) are responsible for externally directed higher-order cognitive function and diverse demanding tasks, such as guiding fine motor function (Liang et al., 2016; Wen et al., 2018). This may support the existence of a reciprocal relationship between motor and cognitive impairments and their respective repair mechanisms.
This study further revealed a significant correlation between the altered dynamic temporal variability and short- and long-term memory scores in both left- and right-stroke patients with varying degrees of motor recovery. This implies that the temporal dynamic variances indicators hold the potential to detect memory dysfunctions in chronic subcortical stroke patients, especially in the group of patients with poor motor outcomes. Additionally, the ROC curve analysis found that the altered regions of dALFF, which had exhibited significant correlations with memory, demonstrated notable AUC values in both left- and right-stroke patients, particularly those with partial recovery of motor function. It may suggest that the temporal dynamic variance indicator has significant potential in distinguishing chronic stroke patients with memory deficits from normal controls.
Additionally, our findings indicate significant heterogeneity in the left- and right- hemispheres stroke when compared to normal controls, both in terms of temporal dynamic variability and memory function. The precise neural mechanisms of the lesion-side effect on these indicators following a subcortical stroke remain unclear; yet, there may be several potential explanations. The right-handed subcortical stroke patients with lesions in the left hemisphere showed more extensive temporal dynamic variances than those in right-sided patients when compared with normal controls. These findings suggest that left-sided stroke patients have a greater imbalance of spatiotemporal patterns of intrinsic brain activity in the whole brain than right-sided patients, which is partly consistent with previous studies reporting more extensive changes in volumetric structural covariance and functional networks in the left-sided patients than in those with right-sided strokes (Wang C. et al., 2019; Wang et al., 2022). This may be attributed to the fact that the right-handedness stroke patients with lesions in left-dominant hemisphere demonstrate lower proficiency in performing daily tasks and exhibit more severe dysfunction than lesions in the right-non-dominant. Moreover, it is generally observed that the extent of brain injury correlates with the level of brain reorganization. In the study, left-stroke patients with good outcomes still exhibited considerable abnormalities in both their long-term and short-term memories, whereas right-stroke patients with equivalent motor recovery did not exhibit any notable deviations from normal controls in terms of memory function. Hence, a potential neural mechanism that can explain the heterogeneity of intrinsic brain activity resulting from the lesion-side may be the difference in severity of brain damage between the dominant and non-dominant hemispheres. Another potential explanation could be associated with the functional asymmetry between the left and right-cerebral hemispheres (Chiu and Damasio, 1980; Good et al., 2001; Corballis, 2014). The left hemisphere exerts a more significant role in executive functions for healthy right-handed individuals than the right hemisphere (Verstynen et al., 2005). Therefore, left-sided subcortical stroke patients with right-handedness may exhibit a higher potential for cortical reorganization, and more extensive disequilibrium in temporal dynamic variability than the right-sided strokes. Additionally, there existed a subtle disparity in the spatial distribution of lesions between lesion-side groups, which may also be associated with the impact of lesion-side on dynamic spatiotemporal patterns of intrinsic brain activity. These findings highlight the importance of considering the effect of the lesion-side and conducting individualized research on post-stroke brain dysfunction in future research endeavors. Certainly, it is essential to conduct more comprehensive research to explore the precise neural mechanisms of the lesion-side effect on the brain activity following subcortical strokes.
The present study is subject to certain limitations. Firstly, there was a wide variation in the time interval between stroke onset and MRI scan among participants, ranging from 6 to 70 months. This heterogeneity may have impacted the findings pertaining to specific post-stroke stages and could potentially limit the generalizability of our results. Future studies will focus on a specific post-stroke stage. Secondly, this study solely utilizes the ALFF as a metric to gauge the intrinsic brain activity. Future analyses will incorporate dynamic functional connection/network metrics with multiple indices to investigate the characteristics of behavioral dysfunctional in subcortical stroke patients. Thirdly, this was a cross-sectional study, and future research should consider conducting a longitudinal study to track the spatiotemporal patterns of intrinsic brain activity in these patients for early prediction. Last but not least, significant inter-group differences were observed in sex in left-stroke patients and the years of education in both left- and right-stroke patients compared to normal controls. Although we have adjusted for these factors in our analysis, they may still affect the specificity of our findings. Future studies will strive to augment the sample size to address potential confounding variables.
Conclusion
The study revealed that chronic subcortical stroke patients with motor pathway damage exhibited both short- and long-term memory dysfunctions, particularly those with partial motor recovery. Additionally, these patients displayed atypical spatiotemporal patterns of intrinsic brain activity across the regions of multiple-domain brain networks, especially in terms of the dynamic temporal variability. The implication is that a subcortical stroke can disrupt the equilibrium between the segregation and integration of intrinsic neural activity within the entire brain network. Furthermore, this study indicated that the indicators of temporal dynamic variability could serve as valuable imaging biomarkers for evaluating potential cognitive dysfunction in chronic subcortical stroke patients with diverse motor outcomes.
Data availability statement
The raw data supporting the conclusions of this article will be made available by the corresponding author, without undue reservation.
Ethics statement
The studies involving humans were approved by the Institutional Review Boards of the First Affiliated Hospital of Zhengzhou University, Tianjin Medical University General Hospital, and Tianjin Huanhu Hospital. The studies were conducted in accordance with the local legislation and institutional requirements. The participants provided their written informed consent to participate in this study. Written informed consent was obtained from the individual(s) for the publication of any potentially identifiable images or data included in this article.
Author contributions
CW: Conceptualization, Data curation, Formal analysis, Funding acquisition, Investigation, Methodology, Project administration, Resources, Supervision, Validation, Visualization, Writing – original draft, Writing – review & editing. JL: Data curation, Funding acquisition, Investigation, Writing – review & editing. JG: Conceptualization, Data curation, Writing – review & editing. SH: Methodology, Writing – review & editing. PM: Data curation, Investigation, Writing – review & editing. YWe: Data curation, Validation, Writing – review & editing. YWa: Methodology, Writing – review & editing. XW: Data curation, Investigation, Writing – review & editing. ZL: Project administration, Resources, Supervision, Writing – review & editing. KX: Formal analysis, Investigation, Writing – review & editing. KW: Formal analysis, Methodology, Writing – review & editing. JC: Funding acquisition, Project administration, Writing – review & editing.
Funding
The author(s) declare financial support was received for the research, authorship, and/or publication of this article. This study was supported by the Natural Science Foundation of China (82271974 and 81871327), the Young Teacher Cultivation Foundation of Zhengzhou University (JC21854044), the Tianjin Key Technology R&D Program (17ZXMFSY00090), and the Young Talents Promotion Program of Henan Province (2021HYTP012).
Acknowledgments
The authors thank all the volunteers, patients, and their caregivers for generously supporting this study. The authors thank the neurology team at the First Affiliated Hospital of Zhengzhou University, Tianjin Medical University General Hospital, and Tianjin Huanhu Hospital for patient recruitments and collection of clinical data.
Conflict of interest
KW was employed by MR Research China, GE Healthcare.
The remaining authors declare that the research was conducted in the absence of any commercial or financial relationships that could be construed as a potential conflict of interest.
Publisher’s note
All claims expressed in this article are solely those of the authors and do not necessarily represent those of their affiliated organizations, or those of the publisher, the editors and the reviewers. Any product that may be evaluated in this article, or claim that may be made by its manufacturer, is not guaranteed or endorsed by the publisher.
Footnotes
2. ^http://www.rfmri.org/dpabi
3. ^v4.0, www.restfmri.net/forum/DynamicBC.
4. ^http://www.fil.ion.ucl.ac.uk/spm/
5. ^Version 9.3.0, http://www.graphpad.com.
References
Allen, E. A., Damaraju, E., Plis, S. M., Erhardt, E. B., Eichele, T., and Calhoun, V. D. (2014). Tracking whole-brain connectivity dynamics in the resting state. Cereb. Cortex 24, 663–676. doi: 10.1093/cercor/bhs352
Al-Qazzaz, N. K., Ali, S. H., Ahmad, S. A., Islam, S., and Mohamad, K. (2014). Cognitive impairment and memory dysfunction after a stroke diagnosis: a post-stroke memory assessment. Neuropsychiatr. Dis. Treat. 10, 1677–1691. doi: 10.2147/NDT.S67184
Benjamini, Y., and Yekutieli, D. (2001). The control of the false discovery rate in multiple testing under dependency. Ann. Stat. 29, 1165–1188. doi: 10.1214/aos/1013699998
Biesbroek, J. M., Weaver, N. A., and Biessels, G. J. (2017). Lesion location and cognitive impact of cerebral small vessel disease. Clin. Sci. (Lond.) 131, 715–728. doi: 10.1042/CS20160452
Cao, W., Sun, X., Dong, D., Yao, S., and Huang, B. (2018). Sex differences in spontaneous brain activity in adolescents with conduct disorder. Front. Psychol. 9:1598. doi: 10.3389/fpsyg.2018.01598
Chang, C., and Glover, G. H. (2010). Time-frequency dynamics of resting-state brain connectivity measured with fMRI. NeuroImage 50, 81–98. doi: 10.1016/j.neuroimage.2009.12.011
Chen, X., Li, M., Chen, N., Lai, H., Huang, Z., Tu, Y., et al. (2022). Structural covariance of the Ipsilesional primary motor cortex in subcortical stroke patients with motor deficits. Neural Plast. 2022, 1460326–1460312. doi: 10.1155/2022/1460326
Chen, J., Sun, D., Shi, Y., Jin, W., Wang, Y., Xi, Q., et al. (2018). Dynamic alterations in spontaneous neural activity in multiple brain networks in subacute stroke patients: a resting-state fMRI study. Front. Neurosci. 12:994. doi: 10.3389/fnins.2018.00994
Chiu, H. C., and Damasio, A. R. (1980). Human cerebral asymmetries evaluated by computed tomography. J. Neurol. Neurosurg. Psychiatry 43, 873–878. doi: 10.1136/jnnp.43.10.873
Corballis, M. C. (2014). Left brain, right brain: facts and fantasies. PLoS Biol. 12:e1001767. doi: 10.1371/journal.pbio.1001767
Cui, Q., Sheng, W., Chen, Y., Pang, Y., Lu, F., Tang, Q., et al. (2020). Dynamic changes of amplitude of low-frequency fluctuations in patients with generalized anxiety disorder. Hum. Brain Mapp. 41, 1667–1676. doi: 10.1002/hbm.24902
de Lima Ferreira, G., de Oliveira Lopes, M. V., Montoril, M. H., Diniz, C. M., and Santana, R. F. (2019). Clinical validation of the nursing diagnosis of impaired memory in patients with a stroke. Jpn. J. Nurs. Sci. 16, 136–144. doi: 10.1111/jjns.12220
Delavaran, H., Jonsson, A. C., Lovkvist, H., Iwarsson, S., Elmstahl, S., Norrving, B., et al. (2017). Cognitive function in stroke survivors: a 10-year follow-up study. Acta Neurol. Scand. 136, 187–194. doi: 10.1111/ane.12709
Diao, Q., Liu, J., Wang, C., Cao, C., Guo, J., Han, T., et al. (2017). Gray matter volume changes in chronic subcortical stroke: a cross-sectional study. Neuroimage Clin. 14, 679–684. doi: 10.1016/j.nicl.2017.01.031
Einstad, M. S., Saltvedt, I., Lydersen, S., Ursin, M. H., Munthe-Kaas, R., Ihle-Hansen, H., et al. (2021). Associations between post-stroke motor and cognitive function: a cross-sectional study. BMC Geriatr. 21:103. doi: 10.1186/s12877-021-02055-7
Fazekas, F., Chawluk, J. B., Alavi, A., Hurtig, H. I., and Zimmerman, R. A. (1987). MR signal abnormalities at 1.5 T in Alzheimer’s dementia and normal aging. AJR Am. J. Roentgenol. 149, 351–356. doi: 10.2214/ajr.149.2.351
Ferreira Correia, A., and Campagna Osorio, I. (2014). The Rey auditory verbal learning test: normative data developed for the Venezuelan population. Arch. Clin. Neuropsychol. 29, 206–215. doi: 10.1093/arclin/act070
Good, C. D., Johnsrude, I., Ashburner, J., Henson, R. N., Friston, K. J., and Frackowiak, R. S. (2001). Cerebral asymmetry and the effects of sex and handedness on brain structure: a voxel-based morphometric analysis of 465 normal adult human brains. NeuroImage 14, 685–700. doi: 10.1006/nimg.2001.0857
Gorelick, P. B., and Nyenhuis, D. (2015). Stroke and cognitive decline. JAMA 314, 29–30. doi: 10.1001/jama.2015.7149
Guo, J., Biswal, B. B., Han, S., Li, J., Yang, S., Yang, M., et al. (2019). Altered dynamics of brain segregation and integration in poststroke aphasia. Hum. Brain Mapp. 40, 3398–3409. doi: 10.1002/hbm.24605
Kannan, L., Vora, J., Bhatt, T., and Hughes, S. L. (2019). Cognitive-motor exergaming for reducing fall risk in people with chronic stroke: a randomized controlled trial. NeuroRehabilitation 44, 493–510. doi: 10.3233/NRE-182683
Li, J., Duan, X., Cui, Q., Chen, H., and Liao, W. (2019). More than just statics: temporal dynamics of intrinsic brain activity predicts the suicidal ideation in depressed patients. Psychol. Med. 49, 852–860. doi: 10.1017/S0033291718001502
Liang, X., Zou, Q., He, Y., and Yang, Y. (2016). Topologically reorganized connectivity architecture of default-mode, executive-control, and salience networks across working memory task loads. Cereb. Cortex 26, 1501–1511. doi: 10.1093/cercor/bhu316
Liao, W., Wu, G. R., Xu, Q., Ji, G. J., Zhang, Z., Zang, Y. F., et al. (2014). DynamicBC: a MATLAB toolbox for dynamic brain connectome analysis. Brain Connect. 4, 780–790. doi: 10.1089/brain.2014.0253
Liegeois, R., Laumann, T. O., Snyder, A. Z., Zhou, J., and Yeo, B. T. T. (2017). Interpreting temporal fluctuations in resting-state functional connectivity MRI. NeuroImage 163, 437–455. doi: 10.1016/j.neuroimage.2017.09.012
Liu, J., Wang, C., Qin, W., Ding, H., Peng, Y., Guo, J., et al. (2023). Cortical structural changes after subcortical stroke: patterns and correlates. Hum. Brain Mapp. 44, 727–743. doi: 10.1002/hbm.26095
Ma, H., Huang, G., Li, M., Han, Y., Sun, J., Zhan, L., et al. (2021). The predictive value of dynamic intrinsic local metrics in transient ischemic attack. Front. Aging Neurosci. 13:808094. doi: 10.3389/fnagi.2021.808094
Mandrekar, J. N. (2010). Receiver operating characteristic curve in diagnostic test assessment. J. Thorac. Oncol. 5, 1315–1316. doi: 10.1097/JTO.0b013e3181ec173d
Mecklinger, A. (2010). The control of long-term memory: brain systems and cognitive processes. Neurosci. Biobehav. Rev. 34, 1055–1065. doi: 10.1016/j.neubiorev.2009.11.020
Parker, R. I., and Hagan-Burke, S. (2007). Useful effect size interpretations for single case research. Behav. Ther. 38, 95–105. doi: 10.1016/j.beth.2006.05.002
Qin, Y., Liu, X., Guo, X., Liu, M., Li, H., and Xu, S. (2021). Low-frequency repetitive transcranial magnetic stimulation restores dynamic functional connectivity in subcortical stroke. Front. Neurol. 12:771034. doi: 10.3389/fneur.2021.771034
Quan, X., Hu, S., Meng, C., Cheng, L., Lu, Y., Xia, Y., et al. (2022). Frequency-specific changes of amplitude of low-frequency fluctuations in patients with acute basal ganglia ischemic stroke. Neural Plast. 2022, 4106131–4106110. doi: 10.1155/2022/4106131
Rubinov, M., and Sporns, O. (2010). Complex network measures of brain connectivity: uses and interpretations. NeuroImage 52, 1059–1069. doi: 10.1016/j.neuroimage.2009.10.003
Shekhar, S., Liu, Y., Wang, S., Zhang, H., Fang, X., Zhang, J., et al. (2021). Novel mechanistic insights and potential therapeutic impact of TRPC6 in neurovascular coupling and ischemic stroke. Int. J. Mol. Sci. 22:2074. doi: 10.3390/ijms22042074
Tsai, Y. H., Yuan, R., Huang, Y. C., Weng, H. H., Yeh, M. Y., Lin, C. P., et al. (2014). Altered resting-state FMRI signals in acute stroke patients with ischemic penumbra. PLoS One 9:e105117. doi: 10.1371/journal.pone.0105117
Van Der Werf, Y. D., Scheltens, P., Lindeboom, J., Witter, M. P., Uylings, H. B., and Jolles, J. (2003). Deficits of memory, executive functioning and attention following infarction in the thalamus; a study of 22 cases with localised lesions. Neuropsychologia 41, 1330–1344. doi: 10.1016/S0028-3932(03)00059-9
Verstynen, T., Diedrichsen, J., Albert, N., Aparicio, P., and Ivry, R. B. (2005). Ipsilateral motor cortex activity during unimanual hand movements relates to task complexity. J. Neurophysiol. 93, 1209–1222. doi: 10.1152/jn.00720.2004
Wang, P., Li, R., Liu, B., Wang, C., Huang, Z., Dai, R., et al. (2019). Altered static and temporal dynamic amplitude of low-frequency fluctuations in the background network during working memory states in mild cognitive impairment. Front. Aging Neurosci. 11:152. doi: 10.3389/fnagi.2019.00152
Wang, C., Miao, P., Liu, J., Li, Z., Wei, Y., Wang, Y., et al. (2021). Validation of cerebral blood flow connectivity as imaging prognostic biomarker on subcortical stroke. J. Neurochem. 159, 172–184. doi: 10.1111/jnc.15359
Wang, C., Qin, W., Zhang, J., Tian, T., Li, Y., Meng, L., et al. (2014). Altered functional organization within and between resting-state networks in chronic subcortical infarction. J. Cereb. Blood Flow Metab. 34, 597–605. doi: 10.1038/jcbfm.2013.238
Wang, Y., Wang, C., Wei, Y., Miao, P., Liu, J., Wu, L., et al. (2022). Abnormal functional connectivities patterns of multidomain cognitive impairments in pontine stroke patients. Hum. Brain Mapp. 43, 4676–4688. doi: 10.1002/hbm.25982
Wang, C., Zhao, L., Luo, Y., Liu, J., Miao, P., Wei, S., et al. (2019). Structural covariance in subcortical stroke patients measured by automated MRI-based volumetry. Neuroimage Clin. 22:101682. doi: 10.1016/j.nicl.2019.101682
Wen, T., Mitchell, D. J., and Duncan, J. (2018). Response of the multiple-demand network during simple stimulus discriminations. NeuroImage 177, 79–87. doi: 10.1016/j.neuroimage.2018.05.019
Wu, X., Wang, L., Jiang, H., Fu, Y., Wang, T., Ma, Z., et al. (2023). Frequency-dependent and time-variant alterations of neural activity in post-stroke depression: a resting-state fMRI study. Neuroimage Clin. 38:103445. doi: 10.1016/j.nicl.2023.103445
Yan, C. G., Wang, X. D., Zuo, X. N., and Zang, Y. F. (2016). DPABI: Data Processing & Analysis for (resting-state) brain imaging. Neuroinformatics 14, 339–351. doi: 10.1007/s12021-016-9299-4
Zang, Y. F., He, Y., Zhu, C. Z., Cao, Q. J., Sui, M. Q., Liang, M., et al. (2007). Altered baseline brain activity in children with ADHD revealed by resting-state functional MRI. Brain Dev. 29, 83–91. doi: 10.1016/j.braindev.2006.07.002
Zhang, J., Meng, L., Qin, W., Liu, N., Shi, F. D., and Yu, C. (2014). Structural damage and functional reorganization in ipsilesional m1 in well-recovered patients with subcortical stroke. Stroke 45, 788–793. doi: 10.1161/STROKEAHA.113.003425
Zhao, L., Biesbroek, J. M., Shi, L., Liu, W., Kuijf, H. J., Chu, W. W., et al. (2018). Strategic infarct location for post-stroke cognitive impairment: a multivariate lesion-symptom mapping study. J. Cereb. Blood Flow Metab. 38, 1299–1311. doi: 10.1177/0271678X17728162
Glossary
Keywords: cognitive, dALFF, fMRI, intrinsic brain activity, sALFF, stroke, temporal dynamic
Citation: Wang C, Liu J, Guo J, Han S, Miao P, Wei Y, Wang Y, Wang X, Li Z, Xue K, Wang K and Cheng J (2023) Dynamic brain activity states of memory impairment in stroke patients with varying motor outcomes. Front. Aging Neurosci. 15:1294009. doi: 10.3389/fnagi.2023.1294009
Edited by:
Peng Li, Southern Medical University, ChinaReviewed by:
Tingwei Mi, Monell Chemical Senses Center, United StatesXu Zhang, University of Texas Health Science Center at Houston, United States
Jingyuan Dai, The Ohio State University, United States
Copyright © 2023 Wang, Liu, Guo, Han, Miao, Wei, Wang, Wang, Li, Xue, Wang and Cheng. This is an open-access article distributed under the terms of the Creative Commons Attribution License (CC BY). The use, distribution or reproduction in other forums is permitted, provided the original author(s) and the copyright owner(s) are credited and that the original publication in this journal is cited, in accordance with accepted academic practice. No use, distribution or reproduction is permitted which does not comply with these terms.
*Correspondence: Caihong Wang, fccwangch@zzu.edu.cn