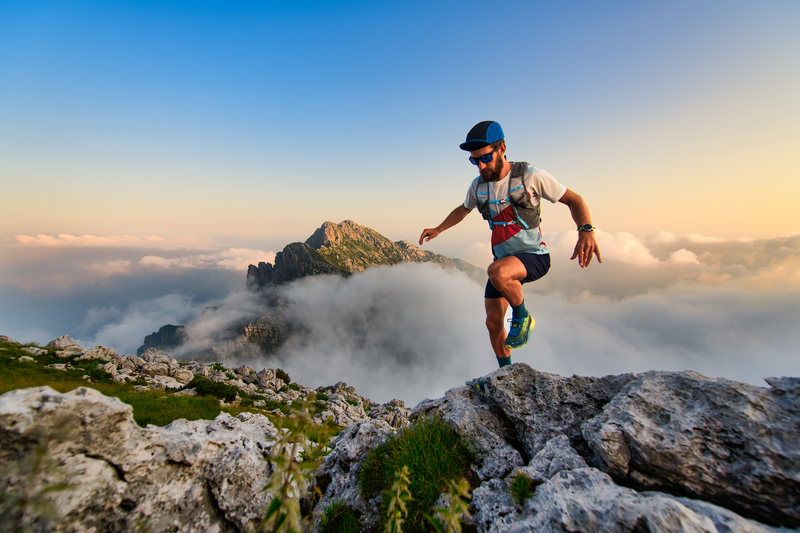
94% of researchers rate our articles as excellent or good
Learn more about the work of our research integrity team to safeguard the quality of each article we publish.
Find out more
BRIEF RESEARCH REPORT article
Front. Aging Neurosci. , 21 November 2023
Sec. Parkinson’s Disease and Aging-related Movement Disorders
Volume 15 - 2023 | https://doi.org/10.3389/fnagi.2023.1279722
Introduction: Fatigue is a common and disabling symptom in Parkinson’s disease (PD), also affecting gait. Detection of fatigue-associated changes of gait using mobile health technologies (MHT) could become increasingly effective.
Methods: Cognitively unimpaired PD patients without fluctuations (UPDRS IV < 1) underwent a standard neurological assessment including the PD-Fatigue scale (PFS-16). PD patients with (PD-F) and without fatigue (PD-N) were matched for age, sex, cognitive function and disease severity. Each participant underwent MHT gait assessment under supervised condition (SC) and unsupervised condition (UC).
Results: Gait parameters of 21 PD-F and 21 PD-N did not significantly differ under SC. Under UC, PD-F showed higher step time, step time variability and asymmetry index compared to PD-N and the PFS-16 correlated with step time.
Conclusion: This is the first MHT-based study with PD patients showing a correlation between fatigue and gait parameters. In addition, the data collected suggest that UC is clearly superior to SC in addressing this question.
Fatigue is a common and disabling symptom in Parkinson’s disease (PD) (Jenkinson et al., 1999; Barone et al., 2009; Gallagher et al., 2010) affecting between 30 and 70 percent of PD patients (Siciliano et al., 2018). This makes fatigue an important target for pharmacological and non-pharmacological interventions (Friedman et al., 2010; Stocchi et al., 2014). The dramatic impact fatigue on quality of life (van Uem et al., 2016) may be mediated by various PD-related symptoms, with movement and mobility deficits playing a central role. For example, it was shown that, in PD, fatigue is associated with the Postural Instability/Gait Difficulty (PIGD) subtype (Hagell and Brundin, 2009; Zhou et al., 2023) and higher total motor sores (Hagell and Brundin, 2009; Zhou et al., 2023). In the general population, fatigue can have a significant impact on mobility and particularly on gait parameters, namely a decrease of walking speed and step length and an increase of step variability (Barbieri et al., 2013; Hamacher et al., 2016). In PD, however, the relationship between fatigue and gait-related parameters has never been explored using digital assessment and thus represent a still debated issue in clinical research (Rochester et al., 2006).
Mobile health technologies (MHT) enable the collection of quantitative and qualitative aspects of gait with high accuracy both in the professional clinical-scientific environment (supervised condition, SC) and in the home of users or patients (unsupervised condition, UC). Several studies suggest that assessment under SC, but not under UC, underestimate PD-related gait deficits (Del Din et al., 2016b; Warmerdam et al., 2020). This might especially true for fluctuating symptoms particularly sensitive to motivation and alertness such as fatigue (Jenkinson et al., 1999; Rochester et al., 2006; Barone et al., 2009; Gallagher et al., 2010).
We therefore investigated in this pilot prospective observational study gait changes in PD patients with and without fatigue matched for age, sex, motor, and cognitive severity. Aim of the study was to evaluate the relationship between gait parameters and fatigue using MHT, with the hypothesis that an home unsupervised assessment might be more informative compared to supervised clinical evaluation in showing this association.
A total of 80 patients with a clinically established PD (Postuma et al., 2015) with at least 1 year of follow-up after diagnosis and beneficial response to dopaminergic treatment from the outpatient Movement disorder Clinic, Neurology Unit at the University of Brescia, Italy from March 2018 to December 2022. The research protocol was approved by the Ethics Committee of the Brescia Hospital, Brescia, Italy (DMA study, NP 1471/22). Written informed consent was obtained from all participants.
Only patients under stable dopaminergic treatment (Postuma et al., 2015) were included. Exclusion criteria were features suggesting atypical parkinsonism (Postuma et al., 2015), a diagnosis of dementia (Emre et al., 2007), impulse control disorder, symptomatic orthostatic hypotension, motor fluctuations (UPDRS-IV > 1), other neurologic disorders or medical conditions potentially affecting gait, need of walking aids, major depressive and bipolar disorder needing pharmacological treatment, schizophrenia, and history of drug and alcohol abuse.
All patients underwent a neurological examination, including the Movement Disorder Society-Unified Parkinson Disease Rating Scale (MDS-UPDRS) part III and IV (Goetz et al., 2008), the Hoehn and Yahr (H&Y) staging (Goetz et al., 2004) and a global cognitive screening using the Montreal Cognitive Assessment test (MOCA) (Nasreddine et al., 2005). Fatigue was assessed using the PD Fatigue Scale (PFS-16) (Brown et al., 2005). Twenty-one patients had ≥ 8 points on the PFS-16 and were considered as the PD group with fatigue (PD-F) (Friedman et al., 2010). Twenty-one PD patients without fatigue (PFS16 < 8, PD-N) matched for age, sex, cognitive function and disease severity were also included.
This selected 42 patients underwent gait assessment under SC and UC (Supplementary Figure 1). Gait assessment under SC was performed with a RehaGait® device on the lower back (Hasomed GmbH, Magdeburg, Germany) (Geritz et al., 2020). Participants walked a 20 m walkway up and down first with self-selected, then with fast speed (Lee et al., 2005). Gait assessment under UC was performed with a Move IV® device on the lower back (Movisens GmbH, Karlsruhe, Germany) over four consecutive days (Härtel et al., 2011). Patients were randomly assigned to start the evaluation with either supervised or unsupervised assessment.
Inertial sensors used in the study for supervised and unsupervised data evaluate the same gait parameters (all included in the analyses) based on lower back algorithms, as extensively described in the ComON study design and in the next session (Geritz et al., 2020).
Raw data were processed using Matlab R2022b (MathWorks, Natick, MA, USA). To analyze gait parameters the raw data of the IMU from the lower back was used for both supervised and unsupervised assessments. To ensure no systematic biases between assessments in terms of heterogeneity, usage and accuracy, raw data of accelerometer and gyroscope from the two assessments were processed with the same validated algorithm (Del Din et al., 2016a; Pham et al., 2017).
As described in the reference (Pham et al., 2017), the raw accelerometer and gyroscope data were processed to first detect the gait events. From the home assessment mean gait parameters per walking bout (WB) was calculated. WBs were determined with more than 3 consecutive steps and then were considered for further analysis. UC gait bouts were split into short (4–19 steps) and long gait bouts (≥ 20 steps) based on the number of steps (Riva et al., 2014; Rennie et al., 2018; Shah et al., 2020) for each day of recording.
Statistical analyses were performed using IBM SPSS statistics version 26. Comparisons of clinical and demographic parameters between PD-F and PD-N were done with Mann-Whitney-U and Fisher’s exact test. Step time, step time variability and step time asymmetry (Paraschiv-Ionescu et al., 2019) were then compared between groups in SC and UC, using Mann Whitney non-parametric analysis and adjusting for age, sex, height, and disease duration. Correlations between PFS-16 values and gait parameters were done using partial correlation analyses and again adjusting for age, sex, height, and disease duration. P < 0.05 was considered statistically significant.
The PD-F group did not significantly differ from the PD-N group in term of age, disease duration, gender distribution, and MDS-UPDRS-III, MoCA, and BMI scores, respectively (Table 1). Under SC, none of the investigated gait parameters did significantly differ between PD-F and PD-N, neither during self-selected, nor during fast pace. Moreover, gait parameters from the SC of the entire PD cohort did not significantly correlate with any demographic or clinical parameter.
Under UC, PD-F showed higher step time (p = 0.02), step time variability (p = 0.005) and step time asymmetry (p = 0.01), compared to PD-N. These differences were more pronounced in the long gait bouts, and less pronounced in the short gait bouts. Details are provided in Table 2. No correlation between digital parameters and PFS-16 scores were found in the whole group. In PD-F, PFS-16 total score correlated with the step time in the longer (r = 0.46, p = 0.05) and shorter bouts (r = 0.56, p = 0.013), whereas no correlation was observed in SC.
Table 2. Differences in gait parameters between PD with and without fatigue in supervised and unsupervised conditions.
This pilot study addressed the complex relationship between fatigue and gait changes in PD patients under supervised and unsupervised home assessment. The findings showed, to our best knowledge for the first time, that subjects with higher fatigue levels present worse gait parameters compared with subjects not reporting fatigue but only when measured in UC using MHT. Conversely, gait parameters as collected in the clinic did not reveal any significant difference between PD patients with and without fatigue. These novel results are of high interest for the research community, as the association between fatigue and “measurable” mobility parameter is still a debated issue in research.
Compared to PD-N, PD-F indeed exhibited in the home environment longer step time and increased step time variability and asymmetry index, which are the typical PD-associated features (Mirelman et al., 2019). Longer step time and step time asymmetry indicate more severe gait alterations in PD-F, whereas increased gait variability has been consistently associated with an increased risk of accelerated progression and an increased risk of falls in PD (Schaafsma et al., 2003).
The study design allowed a matching of PD with similar disease duration and severity, dopaminergic treatment and cognitive function excluding patients with depression or motor fluctuations, in order to minimize the variables potentially influencing both fatigue and motor performances at home and in the clinic (Stocchi et al., 2014; Del Din et al., 2016b; Siciliano et al., 2018; Corrà et al., 2021; Denk et al., 2022; Marin et al., 2022). The differences observed between supervised and unsupervised environment are probably due to different factors, including alertness, motivation, the white-coat and the reverse white-coat effects (respectively worsening and improvement of a clinical parameter measured in clinical setting) and the Hawthorne effect (i.e., change in participants’ behavior due to the awareness that they are being studied) (Warmerdam et al., 2020). In fact, these factors may mask fatigue aspect, and argue for an easier and more consistent role for unsupervised home evaluation for such type of target-variable and assessments.
Of interest, the differences observed in step time and step time variability between the groups in UC were, at least upon visual inspection, more prominent in long walking bouts that are more comparable to supervised analyses (Del Din et al., 2016a). Longer bouts may also reflect a more automatic walking conditions with less alertness and thus higher risk of both fatigue and classical dopamine-related features.
Several further study limitations should be acknowledged. First, the sample size and the pilot nature of this study did not allow specific sex- or fatigue-subcategory analyses (i.e., mental vs. physical), that need to be verified in larger studies. Second, the cross-sectional study design cannot evaluate changes in fatigue and mobility over time. Third, this study excluded patients with major depression, but the link between subtle depressive symptoms psychosocial wellbeing and fatigue should be definitively further explored in larger samples. Fourth, the study excluded a priori PD patients with motor fluctuations to limit the variability of both fatigue and mobility. Fatigue, however, is known to represent an important symptom of L-dopa-induced fluctuation and further studies focused on this specific population are pivotal also to challenge new pharmacological approaches.
Limitations notwithstanding, this study shows that fatigue in PD is associated with specific gait changes which are detectable in home-based environment using MHT technology.
These novel findings can be further investigated in ongoing studies, such as the large IMI consortium IDEA-FAST1 including home-based diaries and longer unsupervised evaluation, which will enable a deeper understanding of the complex relationship between fatigue, mobility and daily activities.
The raw data supporting the conclusions of this article will be made available by the authors, without undue reservation.
The studies involving humans were approved by the Ethics Committee of the Brescia Hospital, Brescia, Italy (DMA study, NP 1471/22). The studies were conducted in accordance with the local legislation and institutional requirements. The participants provided their written informed consent to participate in this study.
APi: Conceptualization, Formal analysis, Methodology, Writing—original draft, Writing—review and editing. AR: Data curation, Formal analysis, Writing—original draft. CZ: Writing—review and editing. CH: Data curation, Formal analysis, Software, Writing—review and editing. AD: Data curation, Formal analysis, Software, Writing—review and editing. RR: Data curation, Formal analysis, Software, Writing—review and editing. WM: Conceptualization, Supervision, Validation, Writing—review and editing. APa: Conceptualization, Supervision, Validation, Writing—review and editing.
The author(s) declare that no financial support was received for the research, authorship, and/or publication of this article.
The authors declare that the research was conducted in the absence of any commercial or financial relationships that could be construed as a potential conflict of interest.
The author(s) declared that they were an editorial board member of Frontiers, at the time of submission. This had no impact on the peer review process and the final decision.
All claims expressed in this article are solely those of the authors and do not necessarily represent those of their affiliated organizations, or those of the publisher, the editors and the reviewers. Any product that may be evaluated in this article, or claim that may be made by its manufacturer, is not guaranteed or endorsed by the publisher.
The Supplementary Material for this article can be found online at: https://www.frontiersin.org/articles/10.3389/fnagi.2023.1279722/full#supplementary-material
Barbieri, F., Santos, P., Lirani-Silva, E., Vitório, R., Gobbi, L., and van Diëen, J. (2013). Systematic review of the effects of fatigue on spatiotemporal gait parameters. J. Back Musculoskelet Rehabil. 26, 125–131. doi: 10.3233/BMR-130371
Barone, P., Antonini, A., Colosimo, C., Marconi, R., Morgante, L., Avarello, T., et al. (2009). The PRIAMO study: A multicenter assessment of nonmotor symptoms and their impact on quality of life in Parkinson’s disease. Mov. Disord. 24, 1641–1649. doi: 10.1002/mds.22643
Brown, R., Dittner, A., Findley, L., and Wessely, S. (2005). The Parkinson fatigue scale. Parkinsonism Relat Disord. 11, 49–55. doi: 10.1016/j.parkreldis.2004.07.007
Corrà, M., Atrsaei, A., Sardoreira, A., Hansen, C., Aminian, K., Correia, M., et al. (2021). Comparison of laboratory and daily-life gait speed assessment during ON and OFF States in Parkinson’s Disease. Sensors 21:3974. doi: 10.3390/s21123974
Del Din, S., Godfrey, A., Galna, B., Lord, S., and Rochester, L. (2016a). Free-living gait characteristics in ageing and Parkinson’s disease: Impact of environment and ambulatory bout length. J. Neuroeng. Rehabil. 13:46. doi: 10.1186/s12984-016-0154-5
Del Din, S., Godfrey, A., Mazzà, C., Lord, S., and Rochester, L. (2016b). Free-living monitoring of Parkinson’s disease: Lessons from the field. Mov. Disord. 31, 1293–1313. doi: 10.1002/mds.26718
Denk, D., Herman, T., Zoetewei, D., Ginis, P., Brozgol, M., Cornejo Thumm, P., et al. (2022). Daily-living freezing of gait as quantified using wearables in people with parkinson disease: Comparison with self-report and provocation tests. Phys. Ther. 102:zac129. doi: 10.1093/ptj/pzac129
Emre, M., Aarsland, D., Brown, R., Burn, D., Duyckaerts, C., Mizuno, Y., et al. (2007). Clinical diagnostic criteria for dementia associated with Parkinson’s disease. Mov. Disord. 22, 1689–707; quiz 1837. doi: 10.1002/mds.21507
Friedman, J., Alves, G., Hagell, P., Marinus, J., Marsh, L., Martinez-Martin, P., et al. (2010). Fatigue rating scales critique and recommendations by the Movement Disorders Society task force on rating scales for Parkinson’s disease. Mov. Disord. 25, 805–822. doi: 10.1002/mds.22989
Gallagher, D., Lees, A., and Schrag, A. (2010). What are the most important nonmotor symptoms in patients with Parkinson’s disease and are we missing them? Mov. Disord. 25, 2493–2500. doi: 10.1002/mds.23394
Geritz, J., Maetzold, S., Steffen, M., Pilotto, A., Corrà, M., Moscovich, M., et al. (2020). Motor, cognitive and mobility deficits in 1000 geriatric patients: Protocol of a quantitative observational study before and after routine clinical geriatric treatment - the ComOn-study. BMC Geriatr. 20:45. doi: 10.1186/s12877-020-1445-z
Goetz, C., Poewe, W., Rascol, O., Sampaio, C., Stebbins, G., Counsell, C., et al. (2004). Movement Disorder Society Task Force report on the Hoehn and Yahr staging scale: Status and recommendations. Mov. Disord. 19, 1020–1028. doi: 10.1002/mds.20213
Goetz, C., Tilley, B., Shaftman, S., Stebbins, G., Fahn, S., Martinez-Martin, P., et al. (2008). Movement Disorder Society-sponsored revision of the Unified Parkinson’s Disease Rating Scale (MDS-UPDRS): Scale presentation and clinimetric testing results. Mov. Disord. 23, 2129–2170. doi: 10.1002/mds.22340
Hagell, P., and Brundin, L. (2009). Towards an understanding of fatigue in Parkinson disease. J. Neurol Neurosurg. Psychiatry 80, 489–492. doi: 10.1136/jnnp.2008.159772
Hamacher, D., Törpel, A., Hamacher, D., and Schega, L. (2016). The effect of physical exhaustion on gait stability in young and older individuals. Gait Posture. 48, 137–139. doi: 10.1016/j.gaitpost.2016.05.007
Härtel, S., Gnam, J. P., Löffler, S., and Bös, K. (2011). Estimation of energy expenditure using accelerometers and activity-based energy models—validation of a new device. Eur. Rev. Aging Phys. Activity 8, 109–114.
Jenkinson, C., Fitzpatrick, R., and Peto, V. (1999). Health-related quality-of-life measurement in patients with Parkinson’s disease. Pharmacoeconomics 15, 157–165. doi: 10.2165/00019053-199915020-00004
Lee, S., Mase, K., and Kogure, K. (2005). Detection of spatio-temporal gait parameters by using wearable motion sensors. Conf. Proc. IEEE Eng. Med. Biol. Soc. 2005, 6836–6839. doi: 10.1109/IEMBS.2005.1616075
Marin, F., Warmerdam, E., Marin, Z., Ben Mansour, K., Maetzler, W., and Hansen, C. (2022). Scoring the sit-to-stand performance of Parkinson’s patients with a single wearable sensor. Sensors 22:8340. doi: 10.3390/s22218340
Mirelman, A., Bonato, P., Camicioli, R., Ellis, T., Giladi, N., Hamilton, J., et al. (2019). Gait impairments in Parkinson’s disease. Lancet Neurol. 18, 697–708. doi: 10.1016/S1474-4422(19)30044-4
Nasreddine, Z., Phillips, N., Bédirian, V., Charbonneau, S., Whitehead, V., Collin, I., et al. (2005). The Montreal Cognitive Assessment, MoCA: A brief screening tool for mild cognitive impairment. J. Am. Geriatr. Soc. 53, 695–699. doi: 10.1111/j.1532-5415.2005.53221.x
Paraschiv-Ionescu, A., Newman, C., Carcreff, L., Gerber, C., Armand, S., and Aminian, K. (2019). Locomotion and cadence detection using a single trunk-fixed accelerometer: Validity for children with cerebral palsy in daily life-like conditions. J. Neuroeng. Rehabil. 16:24. doi: 10.1186/s12984-019-0494-z
Pham, M., Elshehabi, M., Haertner, L., Del Din, S., Srulijes, K., Heger, T., et al. (2017). Validation of a step detection algorithm during straight walking and turning in patients with Parkinson’s disease and older adults using an inertial measurement unit at the lower back. Front. Neurol. 8:457. doi: 10.3389/fneur.2017.00457
Postuma, R., Berg, D., Stern, M., Poewe, W., Olanow, C., Oertel, W., et al. (2015). MDS clinical diagnostic criteria for Parkinson’s disease. Mov. Disord. 30, 1591–1601. doi: 10.1002/mds.26424
Rennie, L., Löfgren, N., Moe-Nilssen, R., Opheim, A., Dietrichs, E., and Franzén, E. (2018). The reliability of gait variability measures for individuals with Parkinson’s disease and healthy older adults - The effect of gait speed. Gait Posture 62, 505–509. doi: 10.1016/j.gaitpost.2018.04.011
Riva, F., Bisi, M., and Stagni, R. (2014). Gait variability and stability measures: Minimum number of strides and within-session reliability. Comput. Biol. Med. 50, 9–13. doi: 10.1016/j.compbiomed.2014.04.001
Rochester, L., Jones, D., Hetherington, V., Nieuwboer, A., Willems, A., Kwakkel, G., et al. (2006). Gait and gait-related activities and fatigue in Parkinson’s disease: What is the relationship? Disabil. Rehabil. 28, 1365–1371. doi: 10.1080/09638280600638034
Schaafsma, J., Giladi, N., Balash, Y., Bartels, A., Gurevich, T., and Hausdorff, J. (2003). Gait dynamics in Parkinson’s disease: Relationship to Parkinsonian features, falls and response to levodopa. J. Neurol. Sci. 212, 47–53. doi: 10.1016/s0022-510x(03)00104-7
Shah, V., McNames, J., Harker, G., Mancini, M., Carlson-Kuhta, P., Nutt, J., et al. (2020). Effect of bout length on gait measures in people with and without Parkinson’s Disease during Daily Life. Sensors 20:5769. doi: 10.3390/s20205769
Siciliano, M., Trojano, L., Santangelo, G., De Micco, R., Tedeschi, G., and Tessitore, A. (2018). Fatigue in Parkinson’s disease: A systematic review and meta-analysis. Mov. Disord. 33, 1712–1723.
Stocchi, F., Abbruzzese, G., Ceravolo, R., Cortelli, P., D’Amelio, M., De Pandis, M., et al. (2014). Prevalence of fatigue in Parkinson disease and its clinical correlates. Neurology 83, 215–220. doi: 10.1212/WNL.0000000000000587
van Uem, J., Marinus, J., Canning, C., van Lummel, R., Dodel, R., Liepelt-Scarfone, I., et al. (2016). Health-Related Quality of Life in patients with Parkinson’s disease–A systematic review based on the ICF model. Neurosci. Biobehav. Rev. 61, 26–34. doi: 10.1016/j.neubiorev.2015.11.014
Warmerdam, E., Hausdorff, J., Atrsaei, A., Zhou, Y., Mirelman, A., Aminian, K., et al. (2020). Long-term unsupervised mobility assessment in movement disorders. Lancet Neurol. 19, 462–470. doi: 10.1016/S1474-4422(19)30397-7
Keywords: gait analysis, fatigue, Parkinson’s disease, supervised and unsupervised assessment, mobile health technology
Citation: Pilotto A, Rizzardi A, Zatti C, Hansen C, Donini A, Romijnders R, Maetzler W and Padovani A (2023) Unsupervised but not supervised gait parameters are related to fatigue in Parkinson’s disease: a pilot study. Front. Aging Neurosci. 15:1279722. doi: 10.3389/fnagi.2023.1279722
Received: 18 August 2023; Accepted: 30 October 2023;
Published: 21 November 2023.
Edited by:
Alessandra Nicoletti, University of Catania, ItalyReviewed by:
Carlo Alberto Artusi, University of Turin, ItalyCopyright © 2023 Pilotto, Rizzardi, Zatti, Hansen, Donini, Romijnders, Maetzler and Padovani. This is an open-access article distributed under the terms of the Creative Commons Attribution License (CC BY). The use, distribution or reproduction in other forums is permitted, provided the original author(s) and the copyright owner(s) are credited and that the original publication in this journal is cited, in accordance with accepted academic practice. No use, distribution or reproduction is permitted which does not comply with these terms.
*Correspondence: Andrea Pilotto, cGlsb3R0b2FuZHJlYWVAZ21haWwuY29t
Disclaimer: All claims expressed in this article are solely those of the authors and do not necessarily represent those of their affiliated organizations, or those of the publisher, the editors and the reviewers. Any product that may be evaluated in this article or claim that may be made by its manufacturer is not guaranteed or endorsed by the publisher.
Research integrity at Frontiers
Learn more about the work of our research integrity team to safeguard the quality of each article we publish.