- 1Department of Clinical and Health Psychology, College of Public Health and Health Professions, University of Florida, Gainesville, FL, United States
- 2Center for Cognitive Aging and Memory, McKnight Brain Institute, University of Florida, Gainesville, FL, United States
- 3Advanced Magnetic Resonance Imaging and Spectroscopy Facility, McKnight Brain Institute, University of Florida, Gainesville, FL, United States
- 4Department of Aging and Geriatric Research, College of Medicine, University of Florida, Gainesville, FL, United States
- 5College of Medicine, Institute on Aging, University of Florida, Gainesville, FL, United States
- 6Department of Neurology, College of Medicine, Norman Fixel Institute for Neurological Diseases, University of Florida, Gainesville, FL, United States
Aging is associated with declines in mitochondrial efficiency and energy production which directly impacts the availability of adenosine triphosphate (ATP), which contains high energy phosphates critical for a variety of cellular functions. Previous phosphorous magnetic resonance spectroscopy (31P MRS) studies demonstrate cerebral ATP declines with age. The purpose of this study was to explore the functional relationships of frontal and posterior ATP levels with cognition in healthy aging. Here, we measured frontal and posterior ATP levels using 31P MRS at 3 Tesla (3 T) and assessed cognition using the Montreal Cognitive Assessment (MoCA) in 30 healthy older adults. We found that greater frontal, but not posterior, ATP levels were significantly associated with better MoCA performance. This relationship remained significant after controlling for age, sex, years of education, and brain atrophy. In conclusion, our findings indicate that cognition is related to ATP in the frontal cortex. These preliminary findings may have important implications in the search for non-invasive markers of in vivo mitochondrial function and the impact of ATP availability on cognition. Future studies are needed to confirm the functional significance of regional ATP and cognition across the lifespan.
Introduction
With the number of adults over the age of 65 in the United States expected to double by 2050 (Ortman et al., 2014), cognitive decline and transition to dementia is one of the most prominent public health concerns. Even in the absence of clear neurodegenerative disease, cognition declines in later life which can impact the ability to carry out day-to-day tasks (Burke and Barnes, 2006). Importantly, accumulating evidence indicates changes in brain structure and function are associated with declines in cognition and functional independence (Cabeza, 2002; Raz et al., 2005). Further, cellular changes are a common feature of aging, such as reduced mitochondrial efficiency and energy production (Culmsee and Landshamer, 2006; Grimm et al., 2016; Dienel, 2019). Yet, the relations among reduced mitochondrial function, cognition, and aging remains unclear. Therefore, it is critical to understand how age-related changes in the brain contribute to cognitive aging to identify potential intervention targets to sustain or enhance cognition and functional independence in older adults.
It is well-documented that crystalized cognitive functions (e.g., general knowledge and vocabulary) remain stable, and may even improve, with advancing age (Salthouse, 2010). Conversely, fluid cognitive functions, such as the ability to reason abstractly and solve problems, are sensitive to age-related changes. Such deficits are linked to age-related changes in speed of processing, attention/working memory, and learning efficiency. These changes are particularly prominent in executive functions (Salthouse et al., 2003; Salthouse, 2010). Executive functions refer to a group of higher-order cognitive processes such as updating, inhibition, and set shifting that are critical for carrying out goal-directed behaviors and adapting to novel situations (Miyake et al., 2000; Lezak et al., 2004). Executive functions are heavily dependent on the functional integrity of the prefrontal cortices (Cummings, 1993). Convergently, decreases in brain volume, cortical thickness, and gray matter in the frontal lobes are associated with advancing age, and occur earlier and more severely than any other region of the brain (Raz, 2000; Drag and Bieliauskas, 2010). These structural changes are associated with significant changes in functional activity. Functional magnetic resonance imaging (fMRI) studies show age-related changes in frontal activation patterns (Gazzaley et al., 2004; Reuter-Lorenz et al., 2000). In addition, aging is associated with increased connectivity within frontal regions (Davis et al., 2012), but reduced connectivity with more posterior regions (Dennis et al., 2008). Further, fMRI and PET studies show age-related changes in blood flow across frontal regions are associated with declines on tasks of abstraction, inhibition, switching (Goh et al., 2013), and semantic organization (Nyberg et al., 2010). In addition, declines in mitochondrial function occurs as adults age, which may in part account for age-related structural and functional brain changes (Culmsee and Landshamer, 2006; Leuner et al., 2007; Forester et al., 2010; Grimm et al., 2016).
The Montreal Cognitive Assessment (MoCA) is a brief measure of global cognitive function that is widely used in clinical settings to screen for mild cognitive impairment and dementia (Nasreddine et al., 2005). The MoCA assesses a broad array of cognitive domains including attention/working memory, set-shifting, naming, letter fluency, recent learning and memory, and visuospatial skills. Given that a number of these domains fall under the umbrella of executive functions, the MoCA is considered sensitive to fronto-executive dysfunction. In fact, this tool shows adequate sensitivity to executive dysfunction associated with normal aging, mild cognitive impairment, and other forms of cognitive decline (Hoops et al., 2009; Gluhm et al., 2013). Further, neuroimaging studies demonstrate set-shifting (Zakzanis et al., 2005; Jacobson et al., 2011), letter fluency (Phelps et al., 1997), and attention/working memory (Gerton et al., 2004; Sun et al., 2005) performances on the MoCA are largely associated with brain activity across frontal areas of the brain, though, to a lesser extent, posterior regions are also implicated.
Adenosine triphosphate (ATP) is a high energy phosphate compound found in living cells and supports a variety of cellular functions. In the brain, the majority of ATP is formed within the mitochondria through oxidative phosphorylation. In turn, ATP plays a vital role in neuronal activity and bioenergetics (Bratic and Trifunovic, 2010). Phosphorous magnetic resonance spectroscopy (31P MRS) is a non-invasive technique that allows for the in vivo investigation of high energy phosphates, such as ATP (Buchli et al., 1994; Ross and Sachdev, 2004; Cady, 2012). Considering the relationship with neuronal activity (Raichle and Mintun, 2006; Bélanger et al., 2011), ATP has been studied in the aging brain. Prior work demonstrates ATP declines with age (Forester et al., 2010; Schmitz et al., 2018). Critically, both whole-brain and frontal ATP have been associated with poor performance on fronto-executive tasks (Volz et al., 1998; Harper et al., 2016). Although promising, the relative functional significance of frontal and posterior ATP on cognition remains unclear.
The purpose of the current preliminary study was to investigate the relationship between frontal and posterior ATP levels with cognitive function in healthy older adults using a widely administered index of cognitive function, the MoCA. The primary 31P MRS voxel of interest was placed in the frontal lobes. An additional voxel was placed in the posterior cortex (i.e., parietal lobe) to serve as a control. These selections were made because frontal regions are more strongly related to cognition as assessed by the MoCA than posterior regions (Julayanont and Nasreddine, 2017). The overall hypothesis was that changes in ATP within specific brain regions will be functionally associated with cognition. Specifically, since older adults evidence changes (1) on frontally mediated tasks of executive functions as well as (2) in frontal brain structure-function, and (3) the MoCA carries a high ‘executive’ load, it was predicted that ATP derived from frontal regions, but not posterior regions, would be associated with cognitive performance.
Materials and methods
Participants
Data were collected at baseline from participants recruited for the Efficient Brain Study, a Phase II cross-over clinical trial investigating the impact of Fermented Papaya Product (FPP) on brain mitochondrial function, neuroinflammation, and cognitive function in older adults (NCT02771366). The sample included 30 healthy older adults ranging from 65 to 89 years old (see Table 1) recruited at the University of Florida. Inclusion criteria for this study included cognitively normal older adults (i.e., MoCA Total Score ≥ 22; Carson et al., 2018). Study participants could not have a history of head injury or brain damage, severe psychiatric disease or psychological disorder, no formal diagnosis or evidence of dementia, or neurological brain disease. This study was approved by the Western Institutional Review Board (WIRB), and all participants provided written, informed consent. At the baseline visit, participants completed a battery of cognitive assessments, medical history and mood questionnaires, and multimodal MRI/MRS scan. In this study, we used the MoCA and 31P MRS data for our analyses.
Montreal Cognitive Assessment (MoCA)
The Montreal Cognitive Assessment (MoCA), a widely used clinical tool to screen for non-normative cognitive decline, was used as an index of global cognition in the current study (Nasreddine et al., 2005). This tool assesses several cognitive domains including attention, concentration, executive functions, memory, language, visuospatial skills, abstraction, calculation, and orientation. Total scores range from 0 to 30, with higher scores reflecting better performance. One point was added to the scores of participants who had 12 years of education or less.
MRS acquisition and analysis
Neuroimaging data were collected on a Phillips 3 T MRI at the University of Florida McKnight Brain Institute. The scanning session was split into two parts. During the first part, participants were scanned using a dual-tuned (1H/31P) whole head quadrature coil (Rapid Biomedical, Germany). A T1 weighted structural image was acquired (1 mm3 isotropic voxel size, 240 × 240 mm acquisition matrix, 160 sagittal slices, Turbo Field Echo) for placement of the two 31P MRS voxels. The 31P MRS voxels were 156 mL in volume (60 mm a-p direction × 65 mm r-l direction × 40 mm f-h direction). For localized 31P MRS acquisition an image-selected in vivo spectroscopy (ISIS) sequence was used with adiabatic localization pulses. Parameters for the two voxels were identical and as follows: TR = 4 s; TE = 0.10 ms; 4,096 spectral points; spectral bandwidth = 4500 Hz; 8 phase cycles; and with −100 Hz offset which with 128 averages resulted in 8 min 40 s scan time for each voxel. The voxels were placed at midline to sample from an equal portion of left and right hemisphere and angled to provide sampling of the largest amount of brain tissue while avoiding skull. See Figure 1 for representative voxel placements.
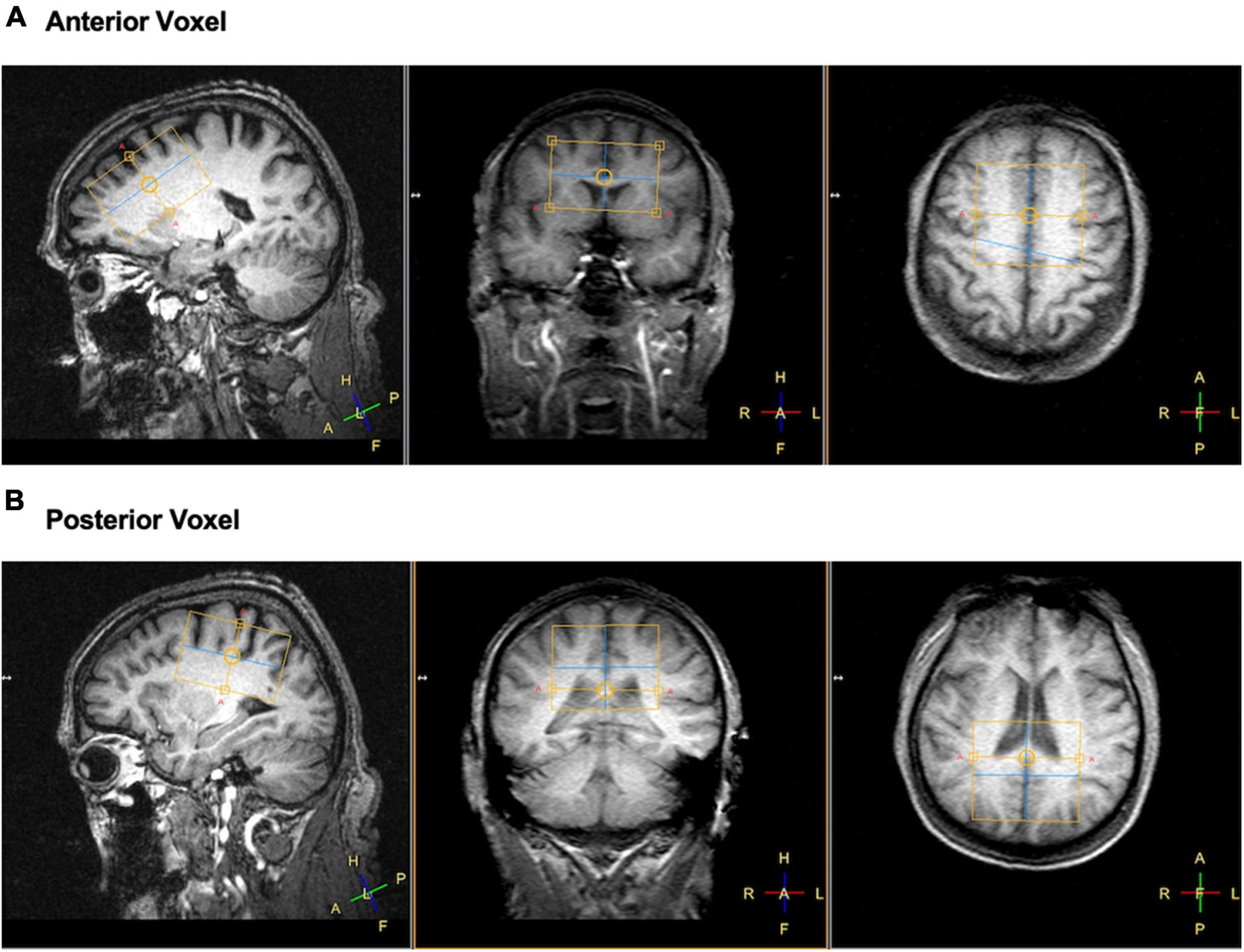
Figure 1. Example of voxel placements for panel (A) frontal and (B) posterior regions of the brain in left sagittal, coronal, and axial view. Yellow boxes represent the location of the voxel collected.
Following 31P MRS acquisition, the head coil was switched to a Phillips 32-channel head coil and the participant was repositioned. A second T1 3D MPRAGE weighted structural image was acquired with 1 mm3 isotropic voxel size, 240 × 240 mm acquisition matrix, 170 sagittal slices. Parameters for the two voxels were identical and as follows: TE = 3.3 ms, TR = 7.2 ms, TI = 903.51062 ms.
The AMARES (Advanced Method for Accurate, Robust, and Efficient Spectral Fitting; Vanhamme et al., 1997) time domain spectra fitting algorithm in the jMRUI software package was used to analyze the 31P spectra (Stefan et al., 2009). All 31P MRS spectra were zero filled with 4,096 points and a 13 Hz Lorentzian line broadening (apodization) was applied before auto-phase shifting to enhance SNR. PCr was adjusted to 0 ppm and constraints for the chemical shifts of the other signals. Seven resonance peaks were manually identified and quantified using previously published estimates (Santos-Díaz and Noseworthy, 2020): gamma, alpha, and beta nucleotide triphosphates (NTP), phosphocreatine (PCr), phosphomonoesters (PME), phosphodiesters (PDE), and inorganic phosphate (Pi). See Figure 2 for a representative output. In general, NTPs provide energy and phosphate group for phosphorylation, and are commonly regarded as ATP in the 31P MRS literature (Forester et al., 2010; Ren et al., 2015; Das et al., 2020; Sassani et al., 2020). Thus, in line with prior 31P studies, ATP was estimated by calculating a ratio of summed NTP peaks (e.g., alpha-, beta-, and gamma; ATP) to pooled total phosphorous (TP) within a specific region (i.e., frontal and posterior ATP TP ratios).
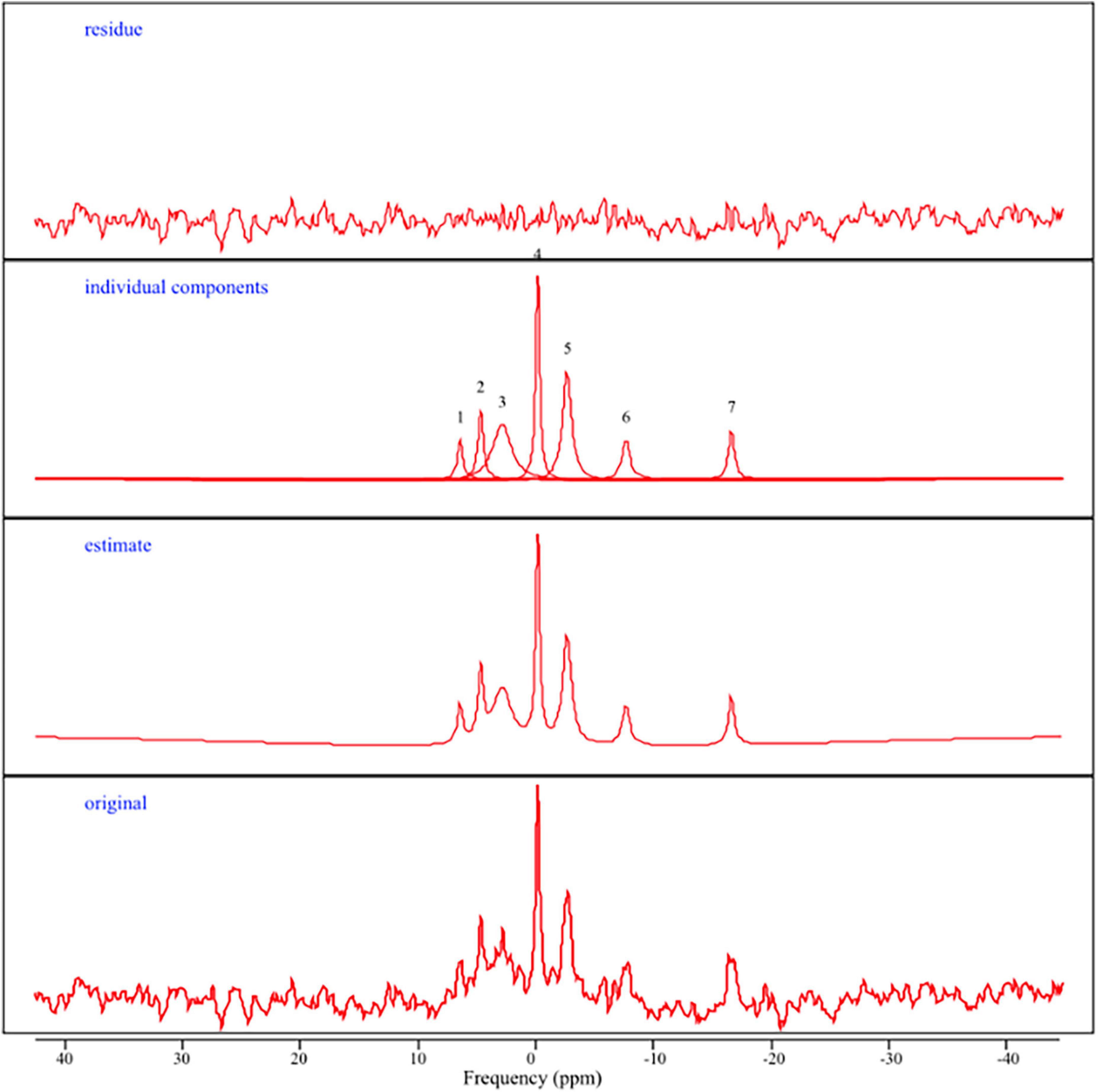
Figure 2. Representative 31P MRS spectra. From top to bottom: (residue) the difference between the estimated curve and the original curve; (individual components) representation of 31P metabolite peaks including 1 = PME, 2 = Pi, 3 = PDE, 4 = PCr, 5 = gamma ATP, 6 = alpha ATP, 7 = beta ATP; (estimate) spectrum generated after jMRUI/AMARES processing; (original) 31P spectrum obtained in the frequency domain in parts per million (ppm).
To control for differing levels of brain atrophy, the acquisition voxels were segmented into gray matter, white matter, and cerebral spinal fluid (CSF) using the software SPM as implemented in the software Gannet. Since we acquired two T1 structural scans we used the higher quality 32-channel acquisition for segmentation purposes (after transforming the 32-channel acquisition into the space of the 31P acquisition using FSL FLIRT; Zhang et al., 2001; Smith et al., 2004). All regression models were run using CSF fraction or gray and white matter as a covariate. All models significant with the CSF fraction approach were also significant for the gray and white matter approach. Therefore, CSF fraction was used as a covariate in subsequent analyses because it makes fewer tissue-specific assumptions and is consistent with previously published approaches in 31P MRS (Rijpma et al., 2018; Schmitz et al., 2018).
Data analysis
First, a multiple linear regression was performed to examine the relationship between demographic variables (age, education, and sex) and MoCA scores (cognitive performance). Next, separate multiple regressions were performed to examine the relationship between age, CSF fraction, and ATP TP ratios (frontal, posterior). Finally, separate multiple linear regressions were performed to examine the relationship between ATP TP ratios (frontal, posterior) and MoCA scores (overall cognitive performance), controlling for age, education, sex, and CSF fraction. These analyses were repeated in exploratory analyses with supplemental 31P metabolites including Pi, PCr, PME, and PDE. All statistical analyses were conducted using the Statistical Package for the Social Sciences (SPSS) Version 25.
Results
MoCA scores as a function of demographic variables
The relationship between MoCA scores and demographic variables were investigated using multiple linear regression. The results of this regression indicated the demographic variables did not account for a significant proportion of variance in MoCA performance (R2 = 0.015, F(3, 29) = 1.15, p = 0.349). No significant predictors emerged.
31P Metabolites as a function of age
The relationship between frontal ATP TP ratios and age was examined using multiple linear regression, while controlling for CSF fraction. The overall model was significant (R2 = 0.14, F(2, 29) = 3.37, p = 0.049). At the trending level, CSF fraction was positively related to age (β = 0.42, p = 0.058), while frontal ATP TP ratios were not (β = 0.05, p = 0.825). Exploratory analyses indicated that there was not a relationship between age and supplemental 31P metabolites in the frontal voxel (all p’s > 0.150).
A similar multiple linear regression analysis examined the relationship between posterior ATP TP ratios and age, while controlling for CSF fraction. The overall model was significant (R2 = 0.22, F(2, 29) = 5.16, p = 0.013). CSF fraction was positively related to age (β = 0.47, p = 0.009). Posterior ATP ratios were not related to age (β = 0.20, p = 0.238). Exploratory analyses indicated that there was not a relationship between age and supplemental 31P metabolites in the posterior voxel (all p’s > 0.327).
MoCA scores as a function of 31P metabolites
The relationship between frontal ATP TP ratios and MoCA scores were examined using multiple linear regression, while controlling for sex, age, education, and CSF fraction. The results of this regression indicated ATP in this region accounted for a significant amount of the variance in MoCA scores (R2 = 0.41, F(5, 24) = 3.38, p = 0.020; See Figure 3A). Higher frontal ATP TP ratios were related to better cognitive performance on the MoCA (β = 0.49, p = 0.021), while controlling for CSF fraction (β = −0.68, p = 0.003). Conversely, age (β = 0.05, p = 0.81), sex (β = 0.22, p = 0.21), and education (β = 0.14, p = 0.41) were not related to MoCA scores. In contrast, exploratory analyses indicated that there was not a relationship between MoCA scores and supplemental 31P metabolites in the frontal voxel (all p’s > 0.338).
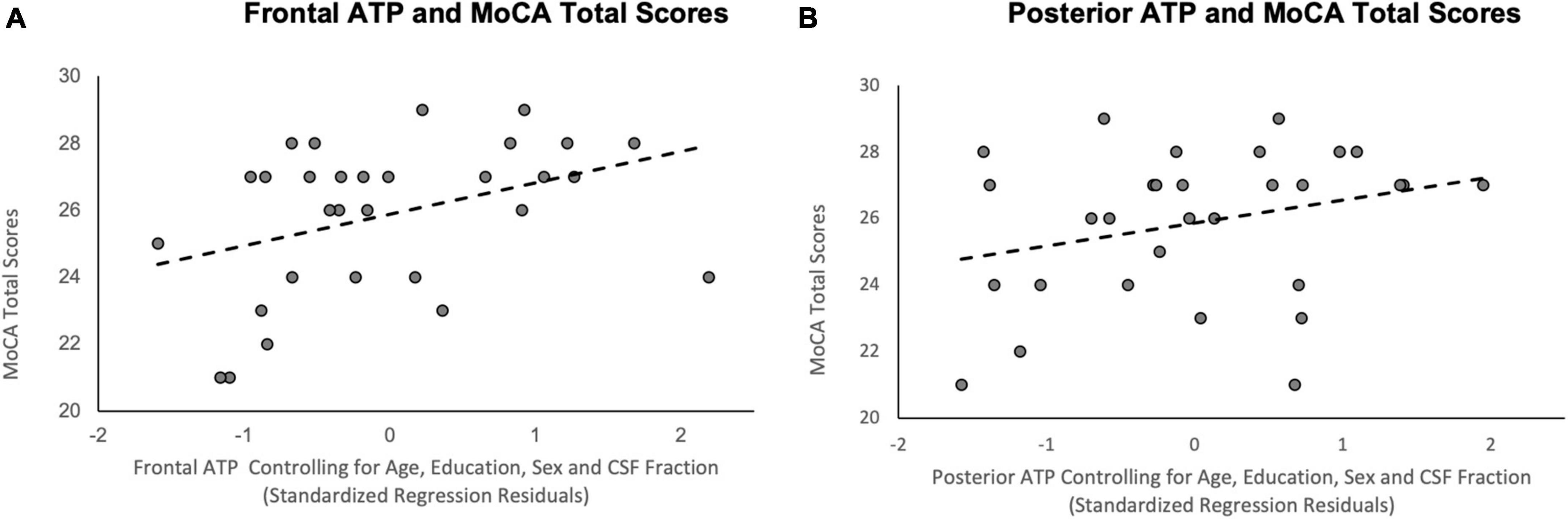
Figure 3. Scatterplots of the relationship between ATP TP ratios and MoCA total scores. (A) Frontal ATP TP ratios; (B) Posterior ATP TP ratios; R2 reflects variance explained from the partial correlation between regional ATP TP ratios and MoCA total scores; X-axis = standardized residual of ATP TP ratios after controlling for age, sex, education, and CSF fraction; Y-axis = MoCA total scores.
A similar multiple linear regression analysis examined the relationship between posterior ATP TP ratios and MoCA scores. The results of this regression demonstrated that the five predictors accounted for a significant amount of variance in MoCA scores (R2 = 0.38, F(5, 24) = 2.91, p = 0.034; See Figure 3B). Among the variables, CSF fraction (β = −0.50, p = 0.023) and, to a lesser extent, sex (β = 0.38, p = 0.049), were significantly related to MoCA scores. Posterior ATP TP ratios did not account for a significant amount of variance in MoCA performance (β = 0.32, p = 0.91), nor did age (β = 0.14, p = 0.54) or years of education (β = 0.29, p = 0.14). Exploratory analyses showed a similar non-significant relationship between MoCA scores and supplemental 31P metabolites in the posterior voxel (all p’s > 0.194).
Discussion
Aging is associated with declines in cognition and mitochondrial function. However, the relationship between mitochondrial function and cognition remains unclear. The purpose of this preliminary study was to explore the functional relationships of frontal and posterior ATP on cognition in a sample of healthy older adults. We had two preliminary findings. First, we did not find a significant relationship between 31P metabolites and age, which is not consistent with previous studies (Ross and Sachdev, 2004; Forester et al., 2010; Schmitz et al., 2018). A potential explanation is range restriction due to a small sample size suppressed a possible effect. Future studies are needed to determine whether the relationship between 31P metabolites and age is robust in more demographically heterogenous and larger samples.
Our second major finding was that we found frontal, but not posterior, ATP was associated with MoCA total scores. Specifically, higher availability of frontal ATP was associated with better performance on the MoCA. This relationship persisted after controlling for demographic characteristics (i.e., age, sex, and education) and age-related atrophy (i.e., CSF voxel). However, other frontal 31P metabolites were not related to MoCA performance. These findings are partially consistent with previous 31P MRS research, which has demonstrated associations between ATP (i.e., frontal or whole brain) and cognition (i.e., executive functions) (Volz et al., 1998; Harper et al., 2016). We expanded on this previous research in several ways. In terms of methodology, we examined ATP in two regions of the brain within the same sample. Most importantly, we demonstrated the differential relationship between regional ATP and cognition in older adults. This pattern of results is consistent with the “last in, first out” hypothesis, which suggests brain regions last to develop show increased vulnerability to the aging process (Raz, 2001). Specifically, there are preferential volumetric and functional changes in the frontal lobes relative to other regions of the brain in aging (Gazzaley et al., 2004; Dennis et al., 2008; Davis et al., 2012; Reuter-Lorenz et al., 2000), which correspond to declines in executive functions (Drag and Bieliauskas, 2010). Speculating beyond the results of this study, 31P MRS markers of ATP within a focal brain region may map onto the functional role of that region in mediating cognition. An alternative explanation for our findings is that other factors impacted this observed relationship, despite our efforts to control for potential covariates. For example, aging is accompanied by changes in metabolic and/or cardiovascular health, (e.g., glycemic control, hypertension), which have been shown to have negative effects on the brain and cognition (Suji and Sivakami, 2004; Tzourio et al., 2014). Together, this may raise the question as to whether metabolic and/or cardiovascular health may attenuate or exacerbate the relationship between ATP and cognition. Future work may benefit from examining the relationship between metabolic and cardiovascular risk on ATP, and its resulting impact on cognition.
In the broader context, plasticity of mitochondria is compromised in aging, which directly disrupts homeostatic regulation of the fusion-fission cycle that allows mitochondrial functional and genetic complementation, and the proper distribution of newly synthesized mitochondria during cell division. This is further compounded by altered mitochondrial biogenesis and degradation (Berman et al., 2008). In turn, there is an accumulation of damaged or dysfunctional mitochondria in cells, which are then rendered unable to execute vital cellular functions, such as production of ATP (Bratic and Trifunovic, 2010). Our findings demonstrate that ATP is associated with behavior, and specifically cognition, in a sample of healthy older adults. Clinically relevant, dysfunctional or damaged mitochondria are implicated in pathological aging processes, including Alzheimer’s disease (AD) (Bertoni-Freddari et al., 2004). As with normal aging, the use of 31P MRS to investigate brain-behavior relationships and progression of clinical symptoms has been vastly underutilized (Ross and Sachdev, 2004). Additional investigation is warranted to determine the clinical utility of regional ATP as marker of preclinical AD risk in healthy older adults. Such an approach may elucidate potential mitochondrial mechanisms contributing to normal and pathological aging processes.
There are limitations to this study. First, our sample included a small population (n = 30) that was mostly white and well-educated participants who were cognitively normal. While our findings make a relevant contribution to our understanding of the aging brain, they should also be viewed as preliminary due to limits in overall sample size and the findings should be confirmed in a larger sample with a less homogeneous demographic population. Second, our results are limited to cognition as indexed by a global cognitive screener. Even so, the MoCA is a widely used clinical tool that has adequate psychometric properties and sensitivity to cognitive changes in several clinical populations as well as aging. Therefore, learning whether there is a relationship between 31P metabolites and MoCA total scores is highly clinically relevant. Future studies are needed to confirm the functional significance of regional ATP and cognition. Third, the current study used a cross-sectional design. Thus, the longitudinal course of these observed relationships is unknown. Future research should explore the stability of these relationships and its relationship to the progression of cognitive decline over time.
In conclusion, this study adds to the growing body of literature examining the associations between 31P metabolites and cognition in older adults. Importantly, we provide evidence that frontal, but not posterior, ATP is associated with global cognition, even after controlling for well-known predictors of cognition including age, education, sex, and brain atrophy. These findings may have important implications in the search for non-invasive markers of in vivo mitochondrial function and the impact of ATP on behavior across the lifespan.
Data availability statement
The raw data supporting the conclusions of this article will be made available by the authors, without undue reservation.
Ethics statement
The studies involving human participants were reviewed and approved by the Western Institutional Review Board. The patients/participants provided their written informed consent to participate in this study.
Author contributions
All authors made substantial contributions to the development of the study, analysis and/or interpretation of data, drafting of the manuscript, and/or providing critical review of the manuscript, and approved the final version.
Funding
This work was supported by the Osato Research (Japan) and the National Institutes of Health (T32NS082168 and F31AG071264), the University of Florida Center for Cognitive Aging and Memory Clinical Translational Research, and the McKnight Brain Research Foundation.
Conflict of interest
The authors declare that the research was conducted in the absence of any commercial or financial relationships that could be construed as a potential conflict of interest.
Publisher’s note
All claims expressed in this article are solely those of the authors and do not necessarily represent those of their affiliated organizations, or those of the publisher, the editors and the reviewers. Any product that may be evaluated in this article, or claim that may be made by its manufacturer, is not guaranteed or endorsed by the publisher.
References
Bélanger, M., Allaman, I., and Magistretti, P. J. (2011). Brain energy metabolism: Focus on astrocyte-neuron metabolic cooperation. Cell Metab. 14, 724–738. doi: 10.1016/j.cmet.2011.08.016
Berman, S. B., Pineda, F. J., and Hardwick, J. M. (2008). Mitochondrial fission and fusion dynamics: The long and short of it. Cell Death Differ. 15, 1147–1152. doi: 10.1038/cdd.2008.57
Bertoni-Freddari, C., Fattoretti, P., Giorgetti, B., Solazzi, M., Balietti, M., and Meier-Ruge, W. (2004). Role of mitochondrial deterioration in physiological and pathological brain aging. Gerontology 50, 187–192. doi: 10.1159/000076779
Bratic, I., and Trifunovic, A. (2010). Mitochondrial energy metabolism and ageing. Biochim. Biophys. Acta Bioenerg. 1797, 961–967.
Buchli, R., Duc, C. O., Martin, E., and Boesiger, P. (1994). Assessment of absolute metabolite concentrations in human tissue by 31P MRS in vivo. Part I: Cerebrum, cerebellum, cerebral gray and white matter. Magn. Reson. Med. 32, 447–452. doi: 10.1002/mrm.1910320404
Burke, S. N., and Barnes, C. A. (2006). Neural plasticity in the ageing brain. Nat. Rev. Neurosci. 7, 30–40. doi: 10.1038/nrn1809
Cabeza, R. (2002). Hemispheric asymmetry reduction in older adults: The HAROLD model. Psychol. Aging 17, 85–100. doi: 10.1037/0882-7974.17.1.85
Cady, E. B. (2012). “In vivo cerebral 31P magnetic resonance spectroscopy,” in Neural metabolism in vivo advances in neurobiology, Vol. 4, eds Y. Choi and R. Greutter (Boston, MA: Springer). doi: 10.1007/978-1-4614-1788-0_6
Carson, N., Leach, L., and Murphy, K. J. (2018). A re-examination of Montreal Cognitive Assessment (MoCA) cutoff scores. Int. J. Geriatr. Psychiatry 33, 379–388. doi: 10.1002/gps.4756
Culmsee, C., and Landshamer, S. (2006). Molecular insights into mechanisms of the cell death program: Role in the progression of neurodegenerative disorders. Curr. Alzheimer Res. 3, 269–283.
Cummings, J. L. (1993). Frontal-subcortical circuits and human behavior. Arch. Neurol. 50, 873–880. doi: 10.1001/archneur.1993.00540080076020
Das, N., Ren, J., Spence, J. S., Rackley, A., and Chapman, S. B. (2020). Relationship of parieto-occipital brain energy phosphate metabolism and cognition using 31P MRS at 7-Tesla in amnestic mild cognitive impairment. Front. Aging Neurosci. 12:222. doi: 10.3389/fnagi.2020.00222
Davis, S. W., Kragel, J. E., Madden, D. J., and Cabeza, R. (2012). The architecture of cross-hemispheric communication in the aging brain: Linking behavior to functional and structural connectivity. Cereb. Cortex 22, 232–242. doi: 10.1093/cercor/bhr123
Dennis, N. A., Hayes, S. M., Prince, S. E., Madden, D. J., Huettel, S. A., and Cabeza, R. (2008). Effects of aging on the neural correlates of successful item and source memory encoding. J. Exp. Psychol. Learn. Mem. Cogn. 34:791. doi: 10.1037/0278-7393.34.4.791
Dienel, G. A. (2019). Brain glucose metabolism: Integration of energetics with function. Physiol. Rev. 99, 949–1045.
Drag, L. L., and Bieliauskas, L. A. (2010). Contemporary review 2009: Cognitive aging. J. Geriatr. Psychiatry Neurol. 23, 75–93. doi: 10.1177/0891988709358590
Forester, B. P., Berlow, Y. A., Harper, D. G., Jensen, J. E., Lange, N., Froimowitz, M. P., et al. (2010). Age-related changes in brain energetics and phospholipid metabolism. NMR Biomed. 23, 242–250. doi: 10.1002/nbm.1444
Gazzaley, A., Rissman, J., and D’esposito, M. (2004). Functional connectivity during working memory maintenance. Cogn. Affect. Behav. Neurosci. 4, 580–599.
Gerton, B. K., Brown, T. T., Meyer-Lindenberg, A., Kohn, P., Holt, J. L., Olsen, R. K., et al. (2004). Shared and distinct neurophysiological components of the digits forward and backward tasks as revealed by functional neuroimaging. Neuropsychologia 42, 1781–1787. doi: 10.1016/j.neuropsychologia.2004.04.023
Gluhm, S., Goldstein, J., Brown, D., Van Liew, C., Gilbert, P. E., and Corey-Bloom, J. (2013). Usefulness of the Montreal Cognitive Assessment (MoCA) in Huntington’s disease. Mov. Disord. 28, 1744–1747.
Goh, J. O., Beason-Held, L. L., An, Y., Kraut, M. A., and Resnick, S. M. (2013). Frontal function and executive processing in older adults: Process and region specific age-related longitudinal functional changes. Neuroimage 69, 43–50. doi: 10.1016/j.neuroimage.2012.12.026
Grimm, A., Friedland, K., and Eckert, A. (2016). Mitochondrial dysfunction: The missing link between aging and sporadic Alzheimer’s disease. Biogerontology 17, 281–296.
Harper, D. G., Joe, E. B., Jensen, J. E., Ravichandran, C., and Forester, B. P. (2016). Brain levels of high-energy phosphate metabolites and executive function in geriatric depression. Int. J. Geriatr. Psychiatry 31, 1241–1249. doi: 10.1002/gps.4439
Hoops, S., Nazem, S., Siderowf, A. D., Duda, J. E., Xie, S. X., Stern, M. B., et al. (2009). Validity of the MoCA and MMSE in the detection of MCI and dementia in Parkinson disease. Neurology 73, 1738–1745.
Jacobson, S. C., Blanchard, M., Connolly, C. C., Cannon, M., and Garavan, H. (2011). An fMRI investigation of a novel analogue to the Trail-Making Test. Brain Cogn. 77, 60–70. doi: 10.1016/j.bandc.2011.06.001
Julayanont, P., and Nasreddine, Z. S. (2017). “Montreal Cognitive Assessment (MoCA): Concept and clinical review,” in Cognitive screening instruments: A practical approach, ed. A. J. Larner (Cham: Springer), 139–195.
Leuner, K., Hauptmann, S., Abdel-Kader, R., Scherping, I., Keil, U., Strosznajder, J. B., et al. (2007). Mitochondrial dysfunction: The first domino in brain aging and Alzheimer’s disease? Antioxid. Redox Signal. 9, 1659–1676. doi: 10.1089/ars.2007.1763
Lezak, M. D., Howieson, D. B., Loring, D. W., and Fischer, J. S. (2004). Neuropsychological assessment. Oxford: Oxford University Press.
Miyake, A., Friedman, N. P., Emerson, M. J., Witzki, A. H., Howerter, A., and Wager, T. D. (2000). The unity and diversity of executive functions and their contributions to complex “frontal lobe” tasks: A latent variable analysis. Cogn. Psychol. 41, 49–100. doi: 10.1006/cogp.1999.0734
Nasreddine, Z. S., Phillips, N. A., Bédirian, V., Charbonneau, S., Whitehead, V., Collin, I., et al. (2005). The Montreal Cognitive Assessment, MoCA: A brief screening tool for mild cognitive impairment. J. Am. Geriatr. Soc. 53, 695–699. doi: 10.1111/j.1532-5415.2005.53221.x
Nyberg, L., Salami, A., Andersson, M., Eriksson, J., Kalpouzos, G., Kauppi, K., et al. (2010). Longitudinal evidence for diminished frontal cortex function in aging. Proc. Natl. Acad. Sci. U.S.A. 107, 22682–22686.
Ortman, J. M., Velkoff, V. A., and Hogan, H. (2014). An aging nation: The older population in the United States. Report Number P25-1140. Washington, DC: U.S. Census Bureau.
Phelps, E. A., Hyder, F., Blamire, A. M., and Shulman, R. G. (1997). FMRI of the prefrontal cortex during overt verbal fluency. Neuroreport 8, 561–565. doi: 10.1097/00001756-199701200-00036
Raichle, M. E., and Mintun, M. A. (2006). Brain work and brain imaging. Annu. Rev. Neurosci. 29, 449–476. doi: 10.1146/annurev.neuro.29.051605.112819
Raz, N. (2000). “Aging of the brain and its impact on cognitive performance: Integration of structural and functional findings,” in The handbook of aging and cognition, eds F. I. M. Craik and T. A. Salthouse (Mahwah, NJ: Lawrence Erlbaum Associates Publishers), 1–90.
Raz, N. (2001). “Ageing and the brain,” in Encyclopedia of life sciences, (London: Nature Publishing Group).
Raz, N., Lindenberger, U., Rodrigue, K. M., Kennedy, K. M., Head, D., Williamson, A., et al. (2005). Regional brain changes in aging healthy adults: General trends, individual differences and modifiers. Cereb. Cortex 15, 1676–1689. doi: 10.1093/cercor/bhi044
Ren, J., Sherry, A. D., and Malloy, C. R. (2015). 31P-MRS of healthy human brain: ATP synthesis, metabolite concentrations, pH, and T1 relaxation times. NMR Biomed. 28, 1455–1462. doi: 10.1002/nbm.3384
Reuter-Lorenz, P. A., Jonides, J., Smith, E. E., Hartley, A., Miller, A., Marshuetz, C., et al. (2000). Age differences in the frontal lateralization of verbal and spatial working memory revealed by PET. J. Cogn. Neurosci. 12, 174–187. doi: 10.1162/089892900561814
Rijpma, A., van der Graaf, M., Meulenbroek, O., Rikkert, M. G. O., and Heerschap, A. (2018). Altered brain high-energy phosphate metabolism in mild Alzheimer’s disease: A 3-dimensional 31P MR spectroscopic imaging study. Neuroimage Clin. 18, 254–261. doi: 10.1016/j.nicl.2018.01.031
Ross, A. J., and Sachdev, P. S. (2004). Magnetic resonance spectroscopy in cognitive research. Brain Res. Rev. 44, 83–102. doi: 10.1016/j.brainresrev.2003.11.001
Salthouse, T. A., Atkinson, T. M., and Berish, D. E. (2003). Executive functioning as a potential mediator of age-related cognitive decline in normal adults. J. Exp. Psychol. Gen. 132:566.
Santos-Díaz, A., and Noseworthy, M. D. (2020). Phosphorus magnetic resonance spectroscopy and imaging (31P-MRS/MRSI) as a window to brain and muscle metabolism: A review of the methods. Biomed. Signal Process. Control 60: 101967.
Sassani, M., Alix, J. J., McDermott, C. J., Baster, K., Hoggard, N., Wild, J. M., et al. (2020). Magnetic resonance spectroscopy reveals mitochondrial dysfunction in amyotrophic lateral sclerosis. Brain 143, 3603–3618.
Schmitz, B., Wang, X., Barker, P. B., Pilatus, U., Bronzlik, P., Dadak, M., et al. (2018). Effects of aging on the human brain: A proton and phosphorus MR spectroscopy study at 3T. J. Neuroimaging 28, 416–421. doi: 10.1111/jon.12514
Smith, S. M., Jenkinson, M., Woolrich, M. W., Beckmann, C. F., Behrens, T. E., Johansen-Berg, H., et al. (2004). Advances in functional and structural MR image analysis and implementation as FSL. Neuroimage 23, S208–S219. doi: 10.1016/j.neuroimage.2004.07.051
Stefan, D. D. C. F., Di Cesare, F., Andrasescu, A., Popa, E., Lazariev, A., Vescovo, E., et al. (2009). Quantitation of magnetic resonance spectroscopy signals: The jMRUI software package. Meas Sci. Technol. 20:104035.
Sun, X., Zhang, X., Chen, X., Zhang, P., Bao, M., Zhang, D., et al. (2005). Age-dependent brain activation during forward and backward digit recall revealed by fMRI. Neuroimage 26, 36–47. doi: 10.1016/j.neuroimage.2005.01.022
Tzourio, C., Laurent, S., and Debette, S. (2014). Is hypertension associated with an accelerated aging of the brain? Hypertension 63, 894–903.
Vanhamme, L., van den Boogaart, A., and Van Huffel, S. (1997). Improved method for accurate and efficient quantification of MRS data with use of prior knowledge. J. Magn. Reson. 129, 35–43. doi: 10.1006/jmre.1997.1244
Volz, H. P., Rzanny, R., Riehemann, S., May, S., Hegewald, H., Preussler, B., et al. (1998). 31 P magnetic resonance spectroscopy in the frontal lobe of major depressed patients. Eur. Arch. Psychiatry Clin. Neurosci. 248, 289–295.
Zakzanis, K. K., Mraz, R., and Graham, S. J. (2005). An fMRI study of the trail making test. Neuropsychologia 43, 1878–1886.
Keywords: phosphorous magnetic resonance spectroscopy, adenosine triphosphate, cognition, aging, brain energy metabolism
Citation: Lopez FV, O’Shea A, Rosenberg JT, Leeuwenburgh C, Anton S, Bowers D and Woods AJ (2023) Frontal adenosine triphosphate markers from 31P MRS are associated with cognitive performance in healthy older adults: preliminary findings. Front. Aging Neurosci. 15:1180994. doi: 10.3389/fnagi.2023.1180994
Received: 06 March 2023; Accepted: 17 July 2023;
Published: 08 August 2023.
Edited by:
P. Hemachandra Reddy, Texas Tech University Health Sciences Center, United StatesReviewed by:
Eva-Maria Ratai, Massachusetts General Hospital and Harvard Medical School, United StatesLijing Xin, Swiss Federal Institute of Technology Lausanne, Switzerland
Copyright © 2023 Lopez, O’Shea, Rosenberg, Leeuwenburgh, Anton, Bowers and Woods. This is an open-access article distributed under the terms of the Creative Commons Attribution License (CC BY). The use, distribution or reproduction in other forums is permitted, provided the original author(s) and the copyright owner(s) are credited and that the original publication in this journal is cited, in accordance with accepted academic practice. No use, distribution or reproduction is permitted which does not comply with these terms.
*Correspondence: Adam J. Woods, YWp3b29kc0BwaGhwLnVmbC5lZHU=