- 1The Russell H. Morgan Department of Radiology and Radiological Science, Johns Hopkins University School of Medicine, Baltimore, MD, United States
- 2Department of Integrated Health Sciences, Nagoya University Graduate School of Medicine, Nagoya, Japan
- 3Department of Radiology, National Center for Geriatrics and Gerontology, Ōbu, Aichi, Japan
- 4Department of Neurology, Nagoya City University Graduate School of Medical Sciences, Nagoya, Japan
The blood–brain barrier (BBB) plays important roles in the maintenance of brain homeostasis. Its main role includes three kinds of functions: (1) to protect the central nervous system from blood-borne toxins and pathogens; (2) to regulate the exchange of substances between the brain parenchyma and capillaries; and (3) to clear metabolic waste and other neurotoxic compounds from the central nervous system into meningeal lymphatics and systemic circulation. Physiologically, the BBB belongs to the glymphatic system and the intramural periarterial drainage pathway, both of which are involved in clearing interstitial solutes such as β-amyloid proteins. Thus, the BBB is believed to contribute to preventing the onset and progression for Alzheimer’s disease. Measurements of BBB function are essential toward a better understanding of Alzheimer’s pathophysiology to establish novel imaging biomarkers and open new avenues of interventions for Alzheimer’s disease and related dementias. The visualization techniques for capillary, cerebrospinal, and interstitial fluid dynamics around the neurovascular unit in living human brains have been enthusiastically developed. The purpose of this review is to summarize recent BBB imaging developments using advanced magnetic resonance imaging technologies in relation to Alzheimer’s disease and related dementias. First, we give an overview of the relationship between Alzheimer’s pathophysiology and BBB dysfunction. Second, we provide a brief description about the principles of non-contrast agent-based and contrast agent-based BBB imaging methodologies. Third, we summarize previous studies that have reported the findings of each BBB imaging method in individuals with the Alzheimer’s disease continuum. Fourth, we introduce a wide range of Alzheimer’s pathophysiology in relation to BBB imaging technologies to advance our understanding of the fluid dynamics around the BBB in both clinical and preclinical settings. Finally, we discuss the challenges of BBB imaging techniques and suggest future directions toward clinically useful imaging biomarkers for Alzheimer’s disease and related dementias.
1. Introduction
The blood–brain barrier (BBB) maintains brain homeostasis through sophisticated anatomical and physiological systems. The BBB is composed of endothelial cells of the capillary wall, pericytes embedded in the endothelial basement membrane, and astrocytic end-feet that surround the parenchymal basement membrane, known overall as the glia limitans (Engelhardt and Sorokin, 2009). Tight junction proteins seal the endothelial cells together and restrict the intrusion of bloodstream substances into the central nervous system (Ballabh et al., 2004). The BBB is also a highly selective semipermeable border, which allows the passive diffusion of some hydrophobic molecules such as oxygen, carbon dioxide, and hormones, as well as facilitates the selective and active transport of water, ions, organic anions, and hydrophilic macromolecules such as glucose and amino acids that are vital nutrients to neurons (Obermeier et al., 2013). Any neuron is less than 10–20 μm away from a capillary (Tsai et al., 2009), which implies that every neuron has its own capillary. This demonstrates the critical relationship between the vascular and neuronal compartments, called the neurovascular unit (NVU; Montagne et al., 2016; Chagnot et al., 2021).
For imaging analysis of the BBB, the most commonly used approaches are to track the uptake of intravenously injected tracers as they leak from the bloodstream into the brain through the BBB. In rodents, two-photon microscopy can monitor the leakage of fluorescent dyes across the BBB, but the field of view is small, and scanning depth is limited (Burgess et al., 2014; Dickie et al., 2020). Photoacoustic imaging enables visualization of dyes or probes with specific absorption characteristics at greater depths than fluorescence-based imaging systems, but is still unable to provide adequate penetration for human brains and is difficult to quantify (Beard, 2011). For human subjects, positron emission tomography (PET) can quantitatively measure the activity of BBB-specific transporters (Piert et al., 1996; Syvänen and Eriksson, 2013), but has lower spatial resolution among the conventional imaging modalities and requires blood sampling during the scan. In addition, repeat scanning in at-risk healthy populations for longitudinal aging and individuals with cognitive decline is difficult to justify due to the cumulative dose of ionizing radiation even if it has little effect on the participants’ health (Nasrallah et al., 2013).
Hence, non-invasive methods with which to detect BBB function for in vivo human subjects are needed to reveal the impact of BBB dysfunction on the pathogenesis and progression of Alzheimer’s disease (AD) pathological conditions. We emphasize that in vivo human experiments are essential to assess the BBB physiology because the fluid dynamics around the BBB would cease in ex vivo human brain tissues. In terms of its non-invasiveness and convenience in a clinical setting, magnetic resonance imaging (MRI) fits well with clinical research to investigate to what extent the BBB functions would be altered along with aging and Alzheimer’s pathological processes. Therefore, we focused on the magnetic resonance-based BBB imaging methodologies in this review. First, we give an overview of the relationship between Alzheimer’s pathophysiology and BBB dysfunction. Second, we provide a brief description about the principles of non-contrast agent-based and contrast agent-based BBB imaging methodologies. Third, we summarize previous studies that have reported the findings of each BBB imaging method in individuals with the AD continuum. Fourth, we introduce a wide range of AD pathophysiology in relation to BBB imaging technologies to advance our understanding of the fluid dynamics around the BBB in both clinical and preclinical settings. Finally, we discuss the challenges of BBB imaging techniques and suggest future directions toward clinically useful imaging biomarkers for AD and related dementias.
2. Alzheimr’s pathopyhsiology in relation to blood–brain barrier dysfunction
There is increasing evidence that supports the involvement of BBB dysfunction in the early stages of Alzheimer’s disease (AD; Montagne et al., 2015; van de Haar et al., 2016a,b; Montagne et al., 2017, 2020; Nation et al., 2019; Sweeney et al., 2019) and related dementias, such as cerebral small vessel disease (CSVD; Zhang et al., 2017; Shao et al., 2019; Wardlaw et al., 2019; Uchida et al., 2020). The pathological hallmarks of AD include the deposition of extracellular β-amyloid (Aβ) aggregates in the brain parenchyma as senile plaques and within the cerebral vessel walls and leptomeninges as cerebral amyloid angiopathy, along with intracellular hyperphosphorylated tau aggregates as neurofibrillary tangles, and neuronal cell loss as neurodegeneration (Jack et al., 2018). Recent clinicopathological and radiological data suggest that there are close relationships between BBB dysfunction and these established Alzheimer’s biomarkers (Cockerill et al., 2018; Michalicova et al., 2020; Wang et al., 2021; Ishida et al., 2022). Excessive accumulation of toxic forms of Aβ and tau proteins is assumed to result from an imbalance between its production and clearance (Tarasoff-Conway et al., 2015). Physiologically, the BBB belongs to the glymphatic system (Iliff et al., 2012, 2013; Nedergaard and Goldman, 2020) and the intramural periarterial drainage pathway (Carare et al., 2008; Weller et al., 2010; Hawkes et al., 2011; Morris et al., 2014), both of which are involved in clearing interstitial solutes such as Aβ (Cockerill et al., 2018; Wang et al., 2021) and tau proteins (Michalicova et al., 2020; Ishida et al., 2022). Further, the ε4 allele of APOE gene is the strongest and most validated genetic risk factor for sporadic AD (Yamazaki et al., 2019). Emerging evidence suggests that APOE ɛ4 directly impairs the BBB: astrocyte-secreted ApoE4 induces the degeneration of brain capillary pericytes that maintain BBB integrity (Bell et al., 2012), and individuals carrying APOE ɛ4 are closely linked to the onset and progression of AD pathogenesis, independent of pathological Alzheimer’s biomarkers (Montagne et al., 2020; Uchida et al., 2022a).
3. Relationship between neurovascular unit and blood–brain barrier imaging
3.1. Normal neurovascular unit physiology in relation to blood–brain barrier imaging
Due to the existence of capillary endothelial cells with tight junctions, trans-endothelial permeability to plasma proteins and inorganic solutes is limited (Nitta et al., 2003; Wardlaw et al., 2003). In addition, the regulation of brain water transport is essential to brain homeostasis and its dysfunction is associated with several neurological conditions. Trans-endothelium water exchanges are through both passive (i.e., diffusion) and active (i.e., co-transport by ion pumps, carrier proteins, and transcytosis) pathways between the capillary and interstitial fluids (Oresković and Klarica, 2010). The key BBB-related water exchange pathway operates through a set of perivascular trans-membrane proteins, which are called aquaporin-4 (AQP4) channels. AQP4 channels were well-regulated and localized to perivascular astrocytic end-feet, known as AQP4 polarization, and form a central pathway for the glymphatic system, facilitating water transport across the basement membrane (Ibata et al., 2011; Papadopoulos and Verkman, 2013; Ohene et al., 2019). The BBB water exchange flow through AQP4 channels plays a role in the drainage of brain metabolites and other neurotoxic compounds from the central nervous system into meningeal lymphatics and systemic circulation as a part of the glymphatic system (Iliff et al., 2012; Figure 1A).
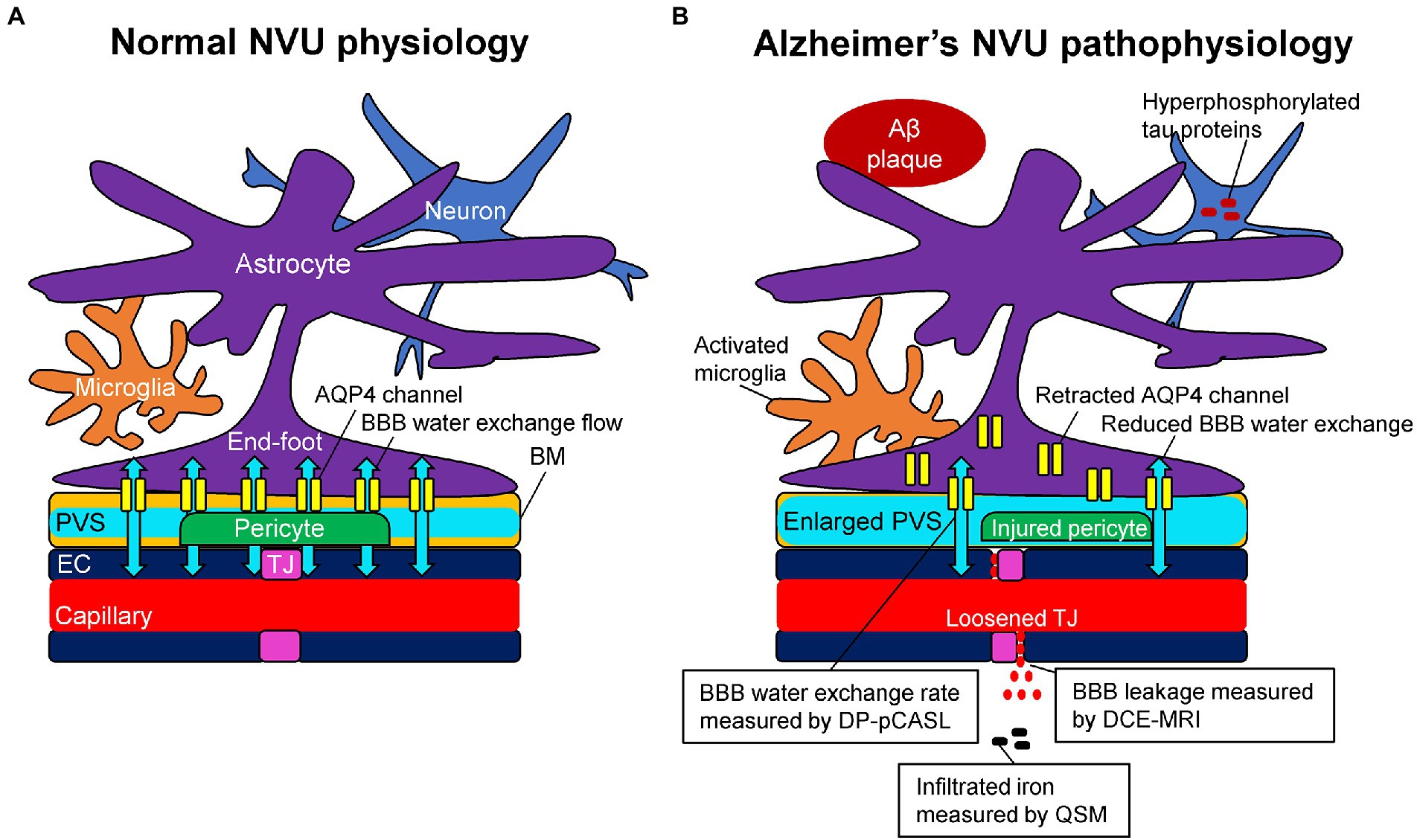
Figure 1. Schematic representation of the neurovascular unit (NVU) in normal physiology (A) and Alzheimer’s pathophysiology (B). (A) The capillary lumen is formed by endothelial cells (EC), pericytes embedded in the basement membrane (BM), and astrocytic end-feet. Tight junction (TJ) proteins seal the ECs together and restrict the passage of solutes into the brain. The BM are separated into two layers, which are attached to the ECs and astrocytic feet, forming the perivascular space (PVS). Aquaporin-4 (AQP4) water channels expressed in the astrocytic end-feet membranes facilitate bidirectional water flow between the capillary, PVS, and interstitial tissues, resulting in the glymphatic flow. (B) In Alzheimer’s pathophysiology, the damaged TJ proteins loosen the seal of the ECs and provide a route for passive diffusion of toxic substances into the extravascular space, which can further damage or downregulate the NVU system. As a consequence of the injured pericyte that normally anchors the AQP4 water channels to astrocytic end-feet, the retraction of AQP4 from astrocytic end-feet membranes occurs with a reduction of bidirectional water flow, resulting in an enlarged PVS. Based on these pathophysiological changes, dynamic contrast-enhanced magnetic resonance imaging (DCE-MRI) can measure the blood–brain barrier (BBB) leakage, pseudo-continuous diffusion-prepared arterial spin labeling (DP-pCASL) can estimate the BBB water exchange rate, and quantitative susceptibility mapping (QSM) can detect the brain iron concentration, respectively.
3.2. Alzheimer’s neurovascular unit pathophysiology in relation to blood–brain barrier imaging
Alzheimer’s pathological changes include NVU pathophysiology, which can be detected by BBB imaging (Figure 1B). There are mainly two key mathematical MRI models for BBB imaging, which will be discussed in this review: those that utilize contrast agents to enhance relaxation rate differences between the intravascular and extravascular compartments (Joseph and Novel, 2020); and those that utilize the dynamic properties of arterial spin labeling (ASL) to first isolate signals from intravascular spins and then estimate the water exchange rate on the evolving signals around the BBB (Dickie et al., 2020). The former model is called dynamic contrast-enhanced MRI (DCE-MRI), which requires the injection of gadolinium-based contrast agents into the vein and has been widely used to measure BBB permeability (Heye and Culling, 2014). Subtle BBB leakage, triggered by loosened endothelial tight junctions (Laurent et al., 2006; Wang et al., 2011) and injured pericytes (Montagne et al., 2015), can be detected using DCE-MRI. The latter model is called ASL-based BBB imaging, which utilizes water as an endogenous tracer alternative to contrast agents. Among ASL-based BBB imaging, a diffusion-prepared pseudo-continuous arterial spin labeling (DP-pCASL) technique has been developed to measure the water exchange rate across the BBB (St Lawrence et al., 2012; Lin et al., 2019; Shao et al., 2019). Aberrant AQP4 expression is linked with decreased efficiency of the BBB water exchange rate (Ohene et al., 2019) and results in excessive Aβ brain deposition (Figure 2; Uchida et al., 2022a). In addition to these direct mathematical BBB models, measurement of several toxic substances from blood can be regarded as an indirect biomarker for BBB dysfunction. Blood-derived substances include fibrinogen, thrombin, hemoglobin, iron-containing hemosiderin, free iron, plasmin, environmental toxins and metals, and possibly, microbial pathogens, which can have toxic neuronal effects and lead to oxidative stress and activation of the proinflammatory microglial response, resulting in the pathological changes seen in AD (Zlokovic, 2011). Quantitative susceptibility mapping (QSM) has been available as an auxiliary biomarker that reflects disease severity in AD to measure brain tissue iron concentration, which is partly due to the BBB leakage caused by the damaged NVU (Cogswell et al., 2021; Uchida et al., 2022b; Figure 3).
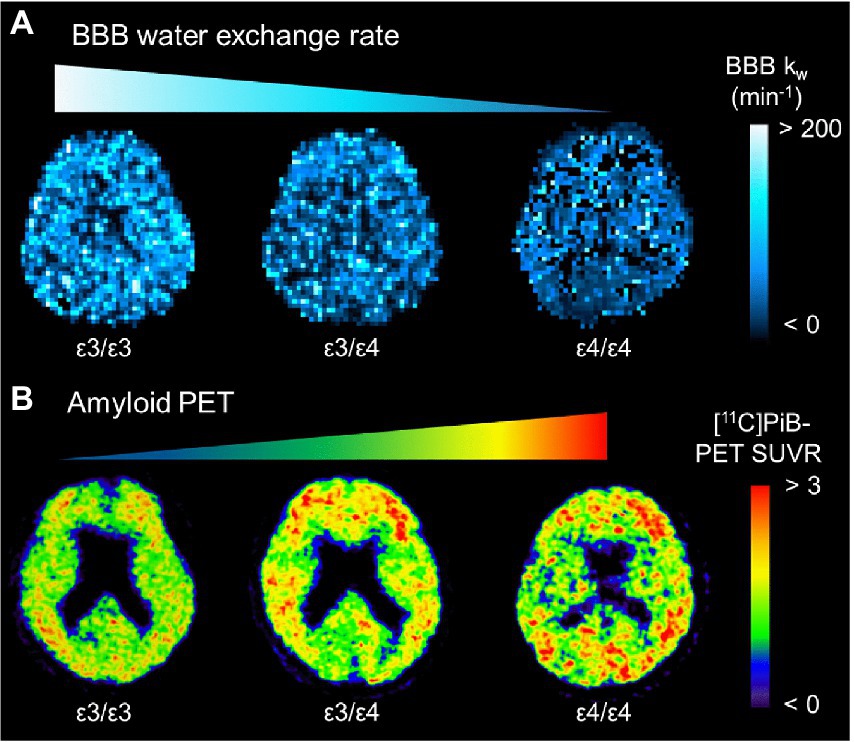
Figure 2. Representative BBB kw map (A) and [11C]PiB-PET SUVR (B) from an APOE ɛ4 noncarrier (ε3/ε3), a heterozygote (ε3/ε4), and a homozygote (ε4/ε4). The kw map from the homozygote (ε4/ε4) displays the lowest kw values, which are associated with the highest SUVRs of [11C]PiB-PET. APOE: apolipoprotein E; BBB, blood–brain barrier; PiB, Pittsburgh compound B; SUVR, standard uptake value ratio [adapted with permission from Uchida et al. (2022a)].
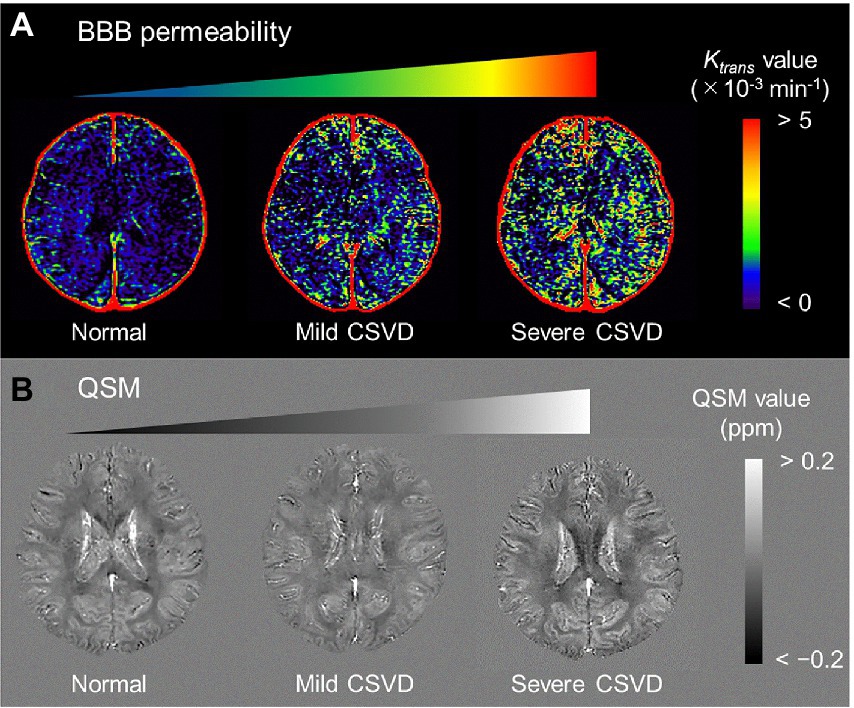
Figure 3. Representative BBB permeability Ktrans map (A) and QSM (B) from normal, mild CSVD, and severe CSVD. The Ktrans map from the severe CSVD displays the highest Ktrans values, which are associated with the highest susceptibility values. BBB, blood–brain barrier; CSVD, cerebral small vascular disease; QSM, quantitative susceptibility mapping [adapted with permission from Uchida et al., 2020].
4. Modeling for blood–brain barrier imaging
4.1. Modeling for blood–brain barrier water exchange rate (kw)
The BBB water exchange rate, kw, can be calculated based on the capillary permeability surface-area product of water (PSw) per unit-mass tissue according to the Renkin-Crone equation (Renkin, 1959; Crone, 1963):
where Ew is the water extraction ratio between the capillary and brain tissue compartments and CBF is the cerebral blood flow. To estimate Ew, a long post-labeling delay (PLD) is required for complete extraction of labeled water in the brain tissue space (Lin et al., 2018; Shao et al., 2018; Uchida et al., 2022a). A single-pass approximation (SPA) model for ASL signals has been proposed to estimate the exchange rate of labeled water from the capillary into the brain tissue space, which does not account for labeled water signal contributions from the brain tissue into the capillary space during the image acquisition (St Lawrence et al., 2012). The BBB water exchange rate, kw, is defined as PSw divided by the distribution volume of water tracer in the capillary space. In a pCASL sequence with prepared diffusion sensitizing gradients of low strength, known as DP-pCASL, signals from the intravascular spins can be nulled, leaving only signals from the extravascular spins. By applying a bi-exponential diffusion signal model to utilize the difference in signal decay between the capillary and brain tissue compartments, the proportion of signals in each compartment can be determined as a function of PLD (St Lawrence et al., 2012; Shao et al., 2019):
where ΔM(t) is the ASL signal at any b values, t is the PLD, A1 and A2 are intravascular and extravascular labeled water fractions, A1(t) + A2(t) = 1, and D1 and D2 are the corresponding apparent diffusion coefficients, respectively. Then, we can estimate the capillary fraction, A1(t), at only two b values, which are zero and a large b value (bDW) sufficient to suppress the vascular signal, but with minimum effect on the tissue signal:
The BBB water exchange rate, kw, can be extracted based on the look-up table approach between the capillary fraction, A1(t), and kw values (Figure 4), incorporating the arterial transit time (ATT), the T1 of arterial blood and gray matter, the labeling efficiency, and the brain–blood partition coefficient as additional inputs for the SPA model (Uchida et al., 2022a). The representative kw maps are shown in Figure 2A.
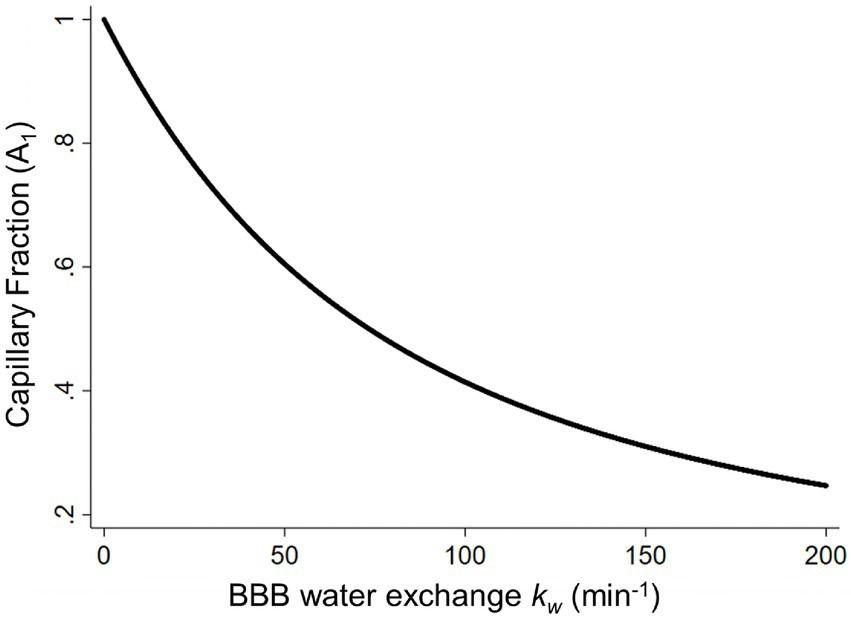
Figure 4. The capillary fraction of labeled water (A1) plotted as a function of the BBB water-exchange rate kw (min−1). Simulated data was generated using the arterial transit time, the T1 of arterial blood (1.66 s) and gray matter (1.82 s), the labeling efficiency (85%), the brain–blood partition coefficient (0.9 ml/g), and the b values (0 and 50 s/mm2) at a long post labeling delay (1.80 s) as inputs for the look-up table algorithm. BBB, blood–brain barrier [adapted with permission from Uchida et al. (2022a)].
4.2. Modeling for blood–brain barrier permeability (Ktrans)
The BBB permeability, Ktrans, is the rate at which contrast agent is delivered to the extravascular space per volume of tissue and contrast agent concentration in the blood plasma. Various pharmacokinetic models have been applied to analyze DCE-MRI data, ranging from relatively simple visual assessment of gadolinium enhancement curves to more complex fitting to pharmacokinetic models, mainly based on the Tofts and Patlak models (Heye and Culling, 2014). The conventional Tofts model assumes a bidirectional flux of tracer between the intra-and extravascular compartments with the volume transfer constant, Ktrans, and negligible blood volume (Tofts and Kermode, 1991). This model was extended by introducing the non-negligible blood plasma compartment as a well-mixed and highly perfused compartment (Tofts et al., 1999). The conventional Tofts model and the extended Tofts model are used for the aggressive alterations of the Ktrans values, such as are found in brain tumors. Meanwhile, the Patlak model assumes a unidirectional flux from the intravascular compartment into the extravascular compartment to estimate subtle BBB leakage of contrast agent to the extravascular space (Patlak et al., 1983). This two-compartment unidirectional transport model ignores the flux from the extravascular space to the intravascular space and provides Ktrans values as the most sensitive modeling with which to detect subtle BBB permeability, which could be applied to the Alzheimer’s NVU pathophysiology (Barnes et al., 2016). In the Patlak model, target parameters are the fractional plasma volume (Vp) and the BBB permeability Ktrans values:
where Ctissue(t) is the contrast agent concentration of the tissue and Cp(t) is determined in the arterial input function (AIF). To obtain the dynamic tracer concentration of plasma as AIF, the dynamic tracer concentration in Cb(t) is converted into dynamic plasma concentration, Cp(t):
where Hct is the hematocrit in the arterial blood plasma. The representative Ktrans maps are shown in Figure 3A.
4.3. Comparison between arterial spin labeling-based blood–brain barrier imaging and dynamic contrast-enhanced MRI
A number of clinicoradiological studies using the BBB water exchange rate measured by ASL-based BBB imaging, BBB permeability measured by DCE-MRI, or both have been conducted in subjects with the AD continuum. A direct comparative analysis of these methodologies found only few correlations between the BBB water exchange rate kw and BBB permeability Ktrans (Shao et al., 2020), suggesting that the mechanisms that regulate water exchange rate across the BBB and the BBB permeability of contrast agents are different (Table 1). In DCE-MRI, the Ktrans values can measure the paracellular leakage of contrast agent through the damaged endothelium and tight junctions. Meanwhile, the kw values can represent the trans-endothelium water exchange rate as both passive diffusion and active transport through ion pumps and AQP4 channels. DCE-MRI becomes more increasingly permeable to large molecules with aging (Montagne et al., 2015), particularly in patients with AD and CSVD (Farrall and Wardlaw, 2009; Zhang et al., 2017; Montagne et al., 2019). In contrast, the water exchange rate across the BBB shows a reverse trend and declines with aging (Li et al., 2005; Anderson et al., 2020). The following sections provide an overview of the relationships between each BBB imaging method and established Alzheimer’s biomarkers, as well as cognitive performance from previous reports.
5. Findings of blood–brain barrier imaging in Alzheimer’s disease subjects
5.1. Overview of dynamic contrast-enhanced MRI in subjects with the Alzheimer’s disease continuum
Dynamic contrast-enhanced MRI has been proven valuable in the assessment of many brain pathologies that cause BBB breakdown, such as tumors (Harrer et al., 2004; Provenzale et al., 2006; Singh et al., 2007; Mills et al., 2009; Bagher-Ebadian et al., 2012; Li et al., 2012; Zhang et al., 2012; Larsson et al., 2013), multiple sclerosis (Gaitán et al., 2011; Jelescu et al., 2011; Shinohara et al., 2011; Ingrisch et al., 2012; Cramer et al., 2014), and ischemic strokes (Kassner et al., 2009; Vidarsson et al., 2009; Thornhill et al., 2010; Topakian et al., 2010; Taheri et al., 2011). While these diseases show significant BBB breakdown, there has been growing interest in the application of DCE-MRI to pathologies associated with more subtle and chronic BBB disruption, such as CSVD (Wardlaw et al., 2008, 2009; Uchida et al., 2020), diabetes (Starr et al., 2003), and AD (Starr et al., 2009; Montagne et al., 2017). As a matter of fact, widely varying estimates of the BBB permeability Ktrans values were reported in each study, for each image acquisition parameter and postprocessing technique. For instance, the selection of pharmacokinetic model directly reflects Ktrans values: if the acquisition duration is short and the rate of BBB leakage estimates are slow, the Patlak model that does not allow back-flow into the capillary will be suitable, while the conventional Tofts and the extended Tofts models can result in increased uncertainty in the fitted parameters (Heye and Culling, 2014). Since contrast agents have relatively large molecular weights (Gd-DTPA 550 Da), BBB permeability necessarily reaches beyond a physiological level before extravasation occurs (Shao et al., 2020). Hence, DCE-MRI with the Patlak model analysis has been increasingly used to quantify low-level BBB permeability in patients with AD pathological changes. An overview of DCE-MRI studies for subjects with the AD continuum is summarized in Table 2 (Starr et al., 2009; Anderson et al., 2011; Montagne et al., 2015; van de Haar et al., 2016a,b, 2017; Montagne et al., 2019; Nation et al., 2019; Dickie et al., 2020; Freeze et al., 2020; Montagne et al., 2020; Chagnot et al., 2021; Li et al., 2021; Choi et al., 2022). As mentioned above, there are considerable differences in Ktrans values among the studies. Note that measuring BBB permeability with DCE-MRI would be confounded by several factors that should be considered when acquiring or interpreting such data. Especially in studies of AD that involve subtle BBB permeability, the modifications implemented to improve the accuracy of Ktrans values must be considered (Manning et al., 2021). As another matter of note, the invasiveness of the injection of gadolinium-based contrast agents and the contraindication for patients with renal insufficiency who might possibly develop nephrogenic systemic fibrosis should be noted. To err on the safe side, the DCE-MRI methodology must be guided by the risk–benefit ratio (Montagne et al., 2016).
5.2. Overview of arterial spin labeling-based blood–brain barrier imaging in subjects with the Alzheimer’s disease continuum
In ASL-based BBB imaging, water is an endogenous tracer alternative to contrast agents, and has a much smaller molecular weight (≈ 18 Da). Therefore, assessing the BBB water exchange rate using the ASL-based BBB imaging can provide a more sensitive assessment of BBB dysfunction at the earliest stages of AD. A recent review has comprehensively summarized the mechanisms of water exchange rate across the BBB, acquisition methods, and mathematical models (Dickie et al., 2020). ASL is a non-invasive technique with which to measure cerebral blood flow, and kinetic models have been proposed to map the transvascular water exchange rate based on the T2 (Ohene et al., 2019) or diffusion coefficient (Shao et al., 2019) differences between the intra-and extravascular compartments. Regional water exchange rate across the BBB can be quantified based on the kinetic modeling of ASL signals in the two compartments. Clinical studies have shown that an altered BBB water exchange rate is associated with aging (Li et al., 2005; Anderson et al., 2020), multiple sclerosis (Rooney et al., 2015), and obstructive sleep apnea (Palomares et al., 2015). In a cohort of early-stage AD subjects who were categorized into apolipoprotein E genotyping, an apolipoprotein E ɛ4 dose was associated with a decreased BBB water exchange rate, resulting in brain Aβ accumulations and cognitive disturbances (Uchida et al., 2022a). An overview of ASL-based BBB imaging studies for subjects with the AD continuum is summarized in Table 3 (Shao et al., 2019; Joseph and Novel, 2020; Shao et al., 2020; Gold et al., 2021; Ford et al., 2022; Uchida et al., 2022a).
6. Other Alzheimer’s disease pathophysiology in relation to blood–brain barrier imaging
6.1. Aging
Aging is the most common cause of AD pathogenesis, and BBB dysfunction is a hallmark of aging and aging-related disorders, including AD and CSVD (Ford et al., 2022). Converging evidence suggests that BBB dysfunction plays a central role in the aging brain (Weiss et al., 2009; Banks et al., 2021). BBB permeability Ktrans values were increased with normal aging (Montagne et al., 2015) and associated with age-related cognitive dysfunction (Bowman et al., 2018; Nation et al., 2019), which was the most prominent in the hippocampus (Montagne et al., 2015; Ivanidze et al., 2019). These Ktrans values measured by the DCE-MRI analysis primarily reflect the paracellular leakage of the relatively large gadolinium contrast agents through injured endothelial tight junctions (Laurent et al., 2006; Wang et al., 2011). Meanwhile, ASL-based BBB imaging can capture the transcellular transport of the much smaller water molecules through AQP4 channels on astrocytic end-feet. Decreased AQP4 polarization was associated with aging and Aβ brain deposition in animal models (Yang et al., 2011; Kress et al., 2014; Ishida et al., 2020) and in human brain tissues (Zeppenfeld et al., 2017). In accordance with these basic experiments, a recent paper found that BBB water exchange rate kw values, measured by DP-pCASL with Quantitative Permeability Mapping analysis in vivo, demonstrated a negative correlation with aging, with adjustments for gender and the number of vascular risk factors as covariates (Ford et al., 2022).
6.2. Vascular risk factors
A close relationship between AD pathogenesis and vascular risk factors, such as hypertension, diabetes, hyperlipidemia, smoking, and lack of exercise, is supported by cumulative evidence (Viswanathan et al., 2009). These vascular risk factors lead to BBB dysfunctions that are associated with AD and vascular cognitive impairments (Yang et al., 2022). BBB imaging is useful for estimating to what extent the BBB function would be damaged at the individual level (Thrippleton et al., 2019), and is part of the design of a therapeutic trial to control vascular risk factors for the prevention of cognitive decline (Chagnot et al., 2021). BBB permeability Ktrans values, using DCE-MRI in patients with diabetes, were increased in white matter, which is reflective of cerebral microangiopathy, before CSVD features, such as lacunes, microbleeds, and white matter hyperintensities, could be visualized on conventional MRI (Chen et al., 2021). BBB water exchange rate kw values using DP-pCASL in patients with vascular risk factors were positively correlated with white matter hyperintensity severity and negatively with executive/episodic memory scores (Shao et al., 2019).
6.3. White matter hyperintensity
White matter hyperintensities are common findings in the elderly population and generally considered ischemic lesions related to CSVD (Yamanaka et al., 2019). CSVD covers a wide array of pathologies involving the dysfunction of the cerebral small vessels. Clinical manifestations include recurrent stroke, cognitive impairment, and gait disturbance. CSVD is a common cause of dementia, with characteristic broadened white matter hyperintensities. While the pathogenesis of white matter hyperintensity remains unclear, BBB leakage is one of the most accepted hypotheses due to its strong association with white matter hyperintensity (Wardlaw et al., 2016; Li et al., 2017; Kerkhofs et al., 2021). Notably, normal-appearing white matter tissues surrounding white matter hyperintensities presented increased BBB permeability, suggesting that an abnormality on BBB imaging could precede further extensions of these white matter lesions (Shao et al., 2020). Because DCE-MRI-based differences in BBB leakage due to white matter lesions is very subtle (Zhang et al., 2017), the Patlak model is recommended to elucidate these differences (Thrippleton et al., 2019). Several reviews have summarized the mechanism of BBB disruption, offered recommendations for BBB imaging analyses, and interpretations of BBB abnormalities, particularly in the white matter, in patients with CSVD (Heye and Culling, 2014; Thrippleton et al., 2019; Dickie et al., 2020; Chagnot et al., 2021).
6.4. Cerebrospinal fluid
Cerebrospinal fluid, predominantly produced in the ventricles and circulating throughout the brain, fills the perivascular space and interacts closely with BBB function (Nakada and Kwee, 2019). The production, circulation, and clearance of cerebrospinal fluid have crucial pathophysiological implications for brain diseases. It also plays a role in the clearance of Aβ and tau proteins to protect from AD pathogenesis (Selkoe and Hardy, 2016). To support this protective function, reduced cerebrospinal fluid production and clearance caused exacerbated AD pathologies (Silverberg et al., 2001; Tarasoff-Conway et al., 2015). Several neuroimaging modalities have been developed to measure cerebrospinal fluid dynamics (Mehta et al., 2022). MR cisternography and MR myelography were conventionally used for the diagnosis of anatomical cerebrospinal fluid disorders (Mokri, 2014). Extending beyond the leakage, phase-contrast cine MRI is the most widely used imaging modality for cerebrospinal fluid dynamics, which is a non-invasive technique without the need for contrast administration or catheterization (Barkhof et al., 1994). Decreased cerebrospinal fluid flow using phase-contrast cine MRI analysis was associated with cognitive deficits in elderly individuals (Attier-Zmudka et al., 2019).
6.5. Oxygen extraction fraction
In addition to the interstitial and cerebrospinal fluid dynamics, pre-and post-capillary vessels in the brain are also key factors in AD pathogenesis. Close monitoring of arterial and venous blood oxygenation serves as a novel biomarker for the study of cerebral hemodynamics (Lu and Ge, 2008), which can aid in understanding the NVU pathophysiology. Oxygen extraction fraction is a physiologic marker that reflects the percentage of oxygen extracted from the blood supply of the brain, which is directly associated with brain oxygen metabolism (Buxton et al., 2004). The oxygen extraction fraction shows a pronounced increase with aging in cognitively healthy individuals (Peng et al., 2014). Meanwhile, oxygen extraction fraction in cognitively impaired individuals has shown various results due to the etiology-based diagnosis of cognitive impairment. For instance, AD pathophysiology led to diminished neural activities, and thereby, decreased oxygen extraction fraction in patients with AD (Butterfield and Halliwell, 2019). Conversely, CSVD pathophysiology caused a reduction in blood supply and resulted in an elevated oxygen extraction fraction (Jann et al., 2021). Thus, these findings were useful in the differential diagnosis for AD and vascular cognitive impairment (Jiang et al., 2020). Longitudinal changes in an elevated oxygen extraction fraction in older adults were associated with the progression of vascular risk factors and white matter hyperintensity volumes, independent of the AD pathologies (Lin et al., 2022).
6.6. Brain iron
The homeostasis and physiological role of brain iron in AD and CSVD has been debated for decades (Tao et al., 2014). QSM has been used to detect the abnormal iron deposition in each specific region as a clinical application and quantify the iron concentration for clinicoradiological research (Acosta-Cabronero et al., 2013; Ayton et al., 2017; Kim et al., 2017; Tiepolt et al., 2018; Gong et al., 2019; Uchida et al., 2020, 2022a,b; Cogswell et al., 2021). Although abnormally high levels of iron are thought to induce free radicals, resulting in neuronal loss and cognitive dysfunction, whether iron deposition is a cause or a result of Alzheimer’s pathogenesis remains elusive. The former hypothesis that brain iron would play a role in the cause of AD is supported by combined QSM and BBB imaging studies, which revealed iron leakage owing to BBB disruption in CSVD using DCE-MRI (Mikati et al., 2014; Tariq et al., 2018; Uchida et al., 2020), and subtle BBB dysfunction in early stages of the AD continuum with the ɛ4 allele of the APOE gene using DP-pCASL (Uchida et al., 2022a). Meanwhile, cerebral microbleeds are frequently observed as an incidental finding, or in the context of an associated Alzheimer’s pathologic finding, such as cerebral amyloid angiopathy (Haller et al., 2018). Consequently, brain iron perturbations detected by QSM could be valuable monitoring tools during AD pathological processes (Fazlollahi et al., 2020; Rotta et al., 2021).
7. Challenges, expectations, and future directions
7.1. Challenges
Challenges and pitfalls exist in the measurement of BBB function to capture AD pathophysiology using magnetic resonance-based BBB imaging technologies. In the DCE-MRI analysis, paracellular BBB leakage of low-molecular-weight gadolinium contrast agents is tracked dynamically as these agents pass from the intravascular to the extravascular space (Heye and Culling, 2014). This approach can detect only major damage to endothelial tight junctions such that gadolinium leaks out of the BBB. Therefore, it cannot detect abnormalities in early AD where the BBB damage has not yet reached the threshold of gadolinium leakage through the endothelium. In addition, it is difficult to find specific transporter alterations, such as AQP4, because the contrast agents are not specifically designed to trace BBB functions (Dickie et al., 2020). In ASL-based BBB imaging, it is difficult to measure anatomical details because of its low spatial resolution and signal-to-noise ratio when acquiring the ASL images (Dickie et al., 2020). Low spatial resolution also causes partial volume errors, whereas the AIF definition on the superior sagittal sinus with a coronal section in the DCE-MRI analysis minimizes these errors (Chagnot et al., 2021). Further technical limitations of these BBB imaging analyses should be highlighted: Gibbs ringing; signal drift; patient motion; AIF definition errors; and kinetic model inaccuracy can confound measurements due to the low amplitude of signal changes (Thrippleton et al., 2019; Chagnot et al., 2021).
7.2. Expectations
While it has long been recognized that water does not diffuse freely across the BBB (Eichling et al., 1974; Bolwig and Lassen, 1975; Herscovitch et al., 1987; Takagi et al., 1987), the idea that water could be used as an internal tracer for measuring BBB function has only recently been proposed (Dickie et al., 2020). In addition to potential safety benefits with no concerns for accumulation of gadolinium in the brain, the use of water to probe BBB function in AD pathophysiology has the following expectations: 1) due to the small size of a water molecule, subtle BBB alterations are likely to be detectable at an earlier stage during the AD pathogenesis; 2) water is physiologically transported across the BBB by both passive and active pathways through co-transporters and uniporters (Zeuthen, 2010) between the capillary, cerebrospinal, and interstitial fluids, potentially providing a wide range of BBB pathophysiology; 3) water has its own transport protein (AQP4) within the NVU system, which closely correlates with the glymphatic flow involved in clearing interstitial solutes (Iliff et al., 2012).
7.3. Future directions
A number of MRI techniques with which to measure the BBB water exchange rate have been developed in addition to DP-pCASL. Recently, a motion-compensated diffusion-weighted pCASL was proposed to acquire intravascular/extravascular perfusion signals from multiple PLDs. Using three-compartment SPA modeling, signal-to-noise ratio increased three-fold and spatial resolution achieved 3.5mm3 isotropic (Shao et al., 2023). Additionally, a multi-echo ASL method was developed to improve the ability to distinguish intravascular-and extravascular-labeled water (Wells et al., 2009). The principal of this method to quantify the BBB water exchange rate depends entirely on intrinsic R2 (= 1/T2) differences between the multi-compartmental origin of labeled water (Wells et al., 2013). If a reliable estimation of the BBB water exchange rate using the multi-echo ASL analysis can be achieved, spatial resolution and the signal-to-noise ratio of the kw map will be improved (Gregori et al., 2013). Furthermore, the water extraction-with-phase-contrast-arterial-spin-tagging (WEPCAST; Lin et al., 2018) and magnetization transfer-weighted ASL methods (Silva et al., 1997) take unique approaches to the measurement of the BBB water exchange rate. The former measures PSw by quantifying the transmitted fraction of labeled water that passes into the superior sagittal sinus during a single pass (Lin et al., 2018). The latter can separate intravascular and extravascular signals based on their different magnetization transfer effects because macromolecular spins interact more strongly with extravascular water (Silva et al., 1997). Since there has been, as yet, no gold standard model with which to measure the BBB water exchange rate, a direct comparison of the kw values among these methodologies will be needed.
In addition to the cross-sectional design of much of the BBB imaging research, longitudinal clinical and radiological studies for individuals who are suspected to be on the AD continuum should be performed. Such studies could reveal the onset of BBB dysfunction, the relationship between BBB imaging alterations and the Alzheimer’s pathology and cognitive decline, and the potential opportunity for timely therapeutic and preventive interventions. ASL-based BBB imaging, which makes use of water as an internal tracer to estimate the BBB function, fits well with these longitudinal clinical and radiological studies so that individuals with the AD continuum can undergo repeated follow-up examinations without concern about adverse effects.
8. Conclusion
Magnetic resonance-based BBB imaging, such as DCE-MRI and DP-pCASL, has already contributed significantly to a better understanding of AD pathogenesis in relation to NVU pathophysiology. Despite being more broadly used, we also highlighted the technical limitations of each BBB imaging model. Nevertheless, we conclude that these magnetic resonance-based BBB imaging methodologies are unique and useful applications that reflect the pathophysiological properties between the interstitial, cerebrospinal, and capillary fluids in the central nervous system and have the potential to measure the efficacy of future BBB-targeted therapeutics in clinical settings for AD and related dementias.
Author contributions
YU: conceptualization, investigation, data curation, writing—original draft, and funding acquisition. HK: conceptualization, data curation, and writing—review and editing. KS: data curation and writing—review and editing. KO: supervision and writing— review and editing. NM: conceptualization, supervision, and writing— review and editing. All authors contributed to the article and approved the submitted version.
Funding
This work was supported by Grants-in-aid of the 24th General Assembly of the Japanese Association of Medical Sciences, Reiwa 3 Grants-in-aid for Young Scientists of the Kowa Life Science Foundation, Grants-in-aid from the 2021 Japan Brain Foundation, and KAKENHI Grant-In-Aid for Scientific Research C (22 K07520).
Conflict of interest
KO is a consultant for “AnatomyWorks” and “Corporate-M.” This arrangement is being managed by the Johns Hopkins University in accordance with its conflict-of-interest policies.
The remaining authors declare that the research was conducted in the absence of any commercial or financial relationships that could be construed as a potential conflict of interest.
Publisher’s note
All claims expressed in this article are solely those of the authors and do not necessarily represent those of their affiliated organizations, or those of the publisher, the editors and the reviewers. Any product that may be evaluated in this article, or claim that may be made by its manufacturer, is not guaranteed or endorsed by the publisher.
References
Acosta-Cabronero, J., Williams, G. B., Cardenas-Blanco, A., Arnold, R. J., Lupson, V., and Nestor, P. J. (2013). In vivo quantitative susceptibility mapping (QSM) in Alzheimer's disease. PLoS One 8:e81093. doi: 10.1371/journal.pone.0081093
Anderson, V. C., Lenar, D. P., Quinn, J. F., and Rooney, W. D. (2011). The blood-brain barrier and microvascular water exchange in Alzheimer's disease. Cardiovasc. Psychiatry Neurol. 2011:615829. doi: 10.1155/2011/615829
Anderson, V. C., Tagge, I. J., Li, X., Quinn, J. F., Kaye, J. A., Bourdette, D. N., et al. (2020). Observation of reduced homeostatic metabolic activity and/or coupling in white matter aging. J. Neuroimaging 30, 658–665. doi: 10.1111/jon.12744
Attier-Zmudka, J., Sérot, J. M., Valluy, J., Saffarini, M., Macaret, A. S., Diouf, M., et al. (2019). Decreased cerebrospinal fluid flow is associated with cognitive deficit in elderly patients. Front. Aging Neurosci. 11:87. doi: 10.3389/fnagi.2019.00087
Ayton, S., Fazlollahi, A., Bourgeat, P., Raniga, P., Ng, A., Lim, Y. Y., et al. (2017). Cerebral quantitative susceptibility mapping predicts amyloid-beta-related cognitive decline. Brain 140, 2112–2119. doi: 10.1093/brain/awx137
Bagher-Ebadian, H., Jain, R., Nejad-Davarani, S. P., Mikkelsen, T., Lu, M., Jiang, Q., et al. (2012). Model selection for DCE-T1 studies in glioblastoma. Magn. Reson. Med. 68, 241–251. doi: 10.1002/mrm.23211
Ballabh, P., Braun, A., and Nedergaard, M. (2004). The blood-brain barrier: an overview: structure, regulation, and clinical implications. Neurobiol. Dis. 16, 1–13. doi: 10.1016/j.nbd.2003.12.016
Banks, W. A., Reed, M. J., Logsdon, A. F., Rhea, E. M., and Erickson, M. A. (2021). Healthy aging and the blood-brain barrier. Nat Aging 1, 243–254. doi: 10.1038/s43587-021-00043-5
Barkhof, F., Kouwenhoven, M., Scheltens, P., Sprenger, M., Algra, P., and Valk, J. (1994). Phase-contrast cine MR imaging of normal aqueductal CSF flow. Effect of aging and relation to CSF void on modulus MR. Acta Radiol. 35, 123–130. doi: 10.1177/028418519403500204
Barnes, S. R., Ng, T. S., Montagne, A., Law, M., Zlokovic, B. V., and Jacobs, R. E. (2016). Optimal acquisition and modeling parameters for accurate assessment of low Ktrans blood-brain barrier permeability using dynamic contrast-enhanced MRI. Magn. Reson. Med. 75, 1967–1977. doi: 10.1002/mrm.25793
Beard, P. (2011). Biomedical photoacoustic imaging. Interface Focus 1, 602–631. doi: 10.1098/rsfs.2011.0028
Bell, R. D., Winkler, E. A., Singh, I., Sagare, A. P., Deane, R., Wu, Z., et al. (2012). Apolipoprotein E controls cerebrovascular integrity via cyclophilin a. Nature 485, 512–516. doi: 10.1038/nature11087
Bolwig, T. G., and Lassen, N. A. (1975). The diffusion permeability to water of the rat blood-brain barrier. Acta Physiol. Scand. 93, 415–422. doi: 10.1111/j.1748-1716.1975.tb05831.x
Bowman, G. L., Dayon, L., Kirkland, R., Wojcik, J., Peyratout, G., Severin, I. C., et al. (2018). Blood-brain barrier breakdown, neuroinflammation, and cognitive decline in older adults. Alzheimers Dement. 14, 1640–1650. doi: 10.1016/j.jalz.2018.06.2857
Burgess, A., Nhan, T., Moffatt, C., Klibanov, A. L., and Hynynen, K. (2014). Analysis of focused ultrasound-induced blood-brain barrier permeability in a mouse model of Alzheimer's disease using two-photon microscopy. J. Control. Release 192, 243–248. doi: 10.1016/j.jconrel.2014.07.051
Butterfield, D. A., and Halliwell, B. (2019). Oxidative stress, dysfunctional glucose metabolism and Alzheimer disease. Nat. Rev. Neurosci. 20, 148–160. doi: 10.1038/s41583-019-0132-6
Buxton, R. B., Uludağ, K., Dubowitz, D. J., and Liu, T. T. (2004). Modeling the hemodynamic response to brain activation. NeuroImage 23, S220–S233. doi: 10.1016/j.neuroimage.2004.07.013
Carare, R. O., Bernardes-Silva, M., Newman, T. A., Page, A. M., Nicoll, J. A., Perry, V. H., et al. (2008). Solutes, but not cells, drain from the brain parenchyma along basement membranes of capillaries and arteries: significance for cerebral amyloid angiopathy and neuroimmunology. Neuropathol. Appl. Neurobiol. 34, 131–144. doi: 10.1111/j.1365-2990.2007.00926.x
Chagnot, A., Barnes, S. R., and Montagne, A. (2021). Magnetic resonance imaging of blood-Brain barrier permeability in dementia. Neuroscience 474, 14–29. doi: 10.1016/j.neuroscience.2021.08.003
Chen, Y. C., Lu, B. Z., Shu, Y. C., and Sun, Y. T. (2021). Spatiotemporal dynamics of cerebral vascular permeability in type 2 diabetes-related cerebral Microangiopathy. Front. Endocrinol. 12:805637. doi: 10.3389/fendo.2021.805637
Choi, J. D., Moon, Y., Kim, H. J., Yim, Y., Lee, S., and Moon, W. J. (2022). Choroid plexus volume and permeability at Brain MRI within the Alzheimer disease clinical spectrum. Radiology 304, 635–645. doi: 10.1148/radiol.212400
Cockerill, I., Oliver, J. A., Xu, H., Fu, B. M., and Zhu, D. (2018). Blood-Brain barrier integrity and clearance of amyloid-β from the BBB. Adv. Exp. Med. Biol. 1097, 261–278. doi: 10.1007/978-3-319-96445-4_14
Cogswell, P. M., Wiste, H. J., Senjem, M. L., Gunter, J. L., Weigand, S. D., Schwarz, C. G., et al. (2021). Associations of quantitative susceptibility mapping with Alzheimer's disease clinical and imaging markers. NeuroImage 224:117433. doi: 10.1016/j.neuroimage.2020.117433
Cramer, S. P., Simonsen, H., Frederiksen, J. L., Rostrup, E., and Larsson, H. B. (2014). Abnormal blood-brain barrier permeability in normal appearing white matter in multiple sclerosis investigated by MRI. NeuroImage Clin. 4, 182–189. doi: 10.1016/j.nicl.2013.12.001
Crone, C. (1963). The permeability of capillaries in various organs as determined by use of the 'indicator diffusion' method. Acta Physiol. Scand. 58, 292–305. doi: 10.1111/j.1748-1716.1963.tb02652.x
Dickie, B. R., Parker, G. J. M., and Parkes, L. M. (2020). Measuring water exchange across the blood-brain barrier using MRI. Prog. Nucl. Magn. Reson. Spectrosc. 116, 19–39. doi: 10.1016/j.pnmrs.2019.09.002
Eichling, J. O., Raichle, M. E., Grubb, R. L. Jr., and Ter-Pogossian, M. M. (1974). Evidence of the limitations of water as a freely diffusible tracer in brain of the rhesus monkey. Circ. Res. 35, 358–364. doi: 10.1161/01.res.35.3.358
Engelhardt, B., and Sorokin, L. (2009). The blood-brain and the blood-cerebrospinal fluid barriers: function and dysfunction. Semin. Immunopathol. 31, 497–511. doi: 10.1007/s00281-009-0177-0
Farrall, A. J., and Wardlaw, J. M. (2009). Blood-brain barrier: ageing and microvascular disease--systematic review and meta-analysis. Neurobiol. Aging 30, 337–352. doi: 10.1016/j.neurobiolaging.2007.07.015
Fazlollahi, A., Raniga, P., Bourgeat, P., Yates, P., Bush, A. I., Salvado, O., et al. (2020). Restricted effect of cerebral microbleeds on regional magnetic susceptibility. J. Alzheimers Dis. 76, 571–577. doi: 10.3233/jad-200076
Ford, J. N., Zhang, Q., Sweeney, E. M., Merkler, A. E., de Leon, M. J., Gupta, A., et al. (2022). Quantitative water permeability mapping of blood-Brain-barrier dysfunction in aging. Front. Aging Neurosci. 14:867452. doi: 10.3389/fnagi.2022.867452
Freeze, W. M., Jacobs, H. I. L., de Jong, J. J., Verheggen, I. C. M., Gronenschild, E., Palm, W. M., et al. (2020). White matter hyperintensities mediate the association between blood-brain barrier leakage and information processing speed. Neurobiol. Aging 85, 113–122. doi: 10.1016/j.neurobiolaging.2019.09.017
Gaitán, M. I., Shea, C. D., Evangelou, I. E., Stone, R. D., Fenton, K. M., Bielekova, B., et al. (2011). Evolution of the blood-brain barrier in newly forming multiple sclerosis lesions. Ann. Neurol. 70, 22–29. doi: 10.1002/ana.22472
Gold, B. T., Shao, X., Sudduth, T. L., Jicha, G. A., Wilcock, D. M., Seago, E. R., et al. (2021). Water exchange rate across the blood-brain barrier is associated with CSF amyloid-β 42 in healthy older adults. Alzheimers Dement. 17, 2020–2029. doi: 10.1002/alz.12357
Gong, N. J., Dibb, R., Bulk, M., van der Weerd, L., and Liu, C. (2019). Imaging beta amyloid aggregation and iron accumulation in Alzheimer's disease using quantitative susceptibility mapping MRI. NeuroImage 191, 176–185. doi: 10.1016/j.neuroimage.2019.02.019
Gregori, J., Schuff, N., Kern, R., and Günther, M. (2013). T2-based arterial spin labeling measurements of blood to tissue water transfer in human brain. J. Magn. Reson. Imaging 37, 332–342. doi: 10.1002/jmri.23822
Haller, S., Vernooij, M. W., Kuijer, J. P. A., Larsson, E. M., Jäger, H. R., and Barkhof, F. (2018). Cerebral microbleeds: imaging and clinical significance. Radiology 287, 11–28. doi: 10.1148/radiol.2018170803
Harrer, J. U., Parker, G. J., Haroon, H. A., Buckley, D. L., Embelton, K., Roberts, C., et al. (2004). Comparative study of methods for determining vascular permeability and blood volume in human gliomas. J. Magn. Reson. Imaging 20, 748–757. doi: 10.1002/jmri.20182
Hawkes, C. A., Härtig, W., Kacza, J., Schliebs, R., Weller, R. O., Nicoll, J. A., et al. (2011). Perivascular drainage of solutes is impaired in the ageing mouse brain and in the presence of cerebral amyloid angiopathy. Acta Neuropathol. 121, 431–443. doi: 10.1007/s00401-011-0801-7
Herscovitch, P., Raichle, M. E., Kilbourn, M. R., and Welch, M. J. (1987). Positron emission tomographic measurement of cerebral blood flow and permeability-surface area product of water using [15O] water and [11C]butanol. J. Cereb. Blood Flow Metab. 7, 527–542. doi: 10.1038/jcbfm.1987.102
Heye, A. K., and Culling, R. D. (2014). Valdes Hernandez Mdel C, Thrippleton MJ, Wardlaw JM. Assessment of blood-brain barrier disruption using dynamic contrast-enhanced MRI. A systematic review. NeuroImage Clin. 6, 262–274. doi: 10.1016/j.nicl.2014.09.002
Ibata, K., Takimoto, S., Morisaku, T., Miyawaki, A., and Yasui, M. (2011). Analysis of aquaporin-mediated diffusional water permeability by coherent anti-stokes Raman scattering microscopy. Biophys. J. 101, 2277–2283. doi: 10.1016/j.bpj.2011.08.045
Iliff, J. J., Lee, H., Yu, M., Feng, T., Logan, J., Nedergaard, M., et al. (2013). Brain-wide pathway for waste clearance captured by contrast-enhanced MRI. J. Clin. Invest. 123, 1299–1309. doi: 10.1172/jci67677
Iliff, J. J., Wang, M., Liao, Y., Plogg, B. A., Peng, W., Gundersen, G. A., et al. (2012). A paravascular pathway facilitates CSF flow through the brain parenchyma and the clearance of interstitial solutes, including amyloid β. Sci. Transl. Med. 4:147ra11. doi: 10.1126/scitranslmed.3003748
Ingrisch, M., Sourbron, S., Morhard, D., Ertl-Wagner, B., Kümpfel, T., Hohlfeld, R., et al. (2012). Quantification of perfusion and permeability in multiple sclerosis: dynamic contrast-enhanced MRI in 3D at 3T. Investig. Radiol. 47, 252–258. doi: 10.1097/RLI.0b013e31823bfc97
Ishida, K., Misawa, K., Nishimura, H., Hirata, T., Yamamoto, M., and Ota, N. (2020). 5-Caffeoylquinic acid ameliorates cognitive decline and reduces Aβ deposition by modulating Aβ clearance pathways in APP/PS2 transgenic mice. Nutrients 12:494. doi: 10.3390/nu12020494
Ishida, K., Yamada, K., Nishiyama, R., Hashimoto, T., Nishida, I., Abe, Y., et al. (2022). Glymphatic system clears extracellular tau and protects from tau aggregation and neurodegeneration. J. Exp. Med. 219:1275. doi: 10.1084/jem.20211275
Ivanidze, J., Mackay, M., Hoang, A., Chi, J. M., Cheng, K., Aranow, C., et al. (2019). Dynamic contrast-enhanced MRI reveals unique blood-Brain barrier permeability characteristics in the hippocampus in the Normal Brain. AJNR Am. J. Neuroradiol. 40, 408–411. doi: 10.3174/ajnr.A5962
Jack, C. R. Jr., Bennett, D. A., Blennow, K., Carrillo, M. C., Dunn, B., Haeberlein, S. B., et al. (2018). NIA-AA research framework: toward a biological definition of Alzheimer's disease. Alzheimers Dement. 14, 535–562. doi: 10.1016/j.jalz.2018.02.018
Jann, K., Shao, X., Ma, S. J., Cen, S. Y., D'Orazio, L., Barisano, G., et al. (2021). Evaluation of cerebral blood flow measured by 3D PCASL as biomarker of vascular cognitive impairment and dementia (VCID) in a cohort of elderly Latinx subjects at risk of small vessel disease. Front. Neurosci. 15:627627. doi: 10.3389/fnins.2021.627627
Jelescu, I. O., Leppert, I. R., Narayanan, S., Araújo, D., Arnold, D. L., and Pike, G. B. (2011). Dual-temporal resolution dynamic contrast-enhanced MRI protocol for blood-brain barrier permeability measurement in enhancing multiple sclerosis lesions. J. Magn. Reson. Imaging 33, 1291–1300. doi: 10.1002/jmri.22565
Jiang, D., Lin, Z., Liu, P., Sur, S., Xu, C., Hazel, K., et al. (2020). Brain oxygen extraction is differentially altered by Alzheimer's and vascular diseases. J. Magn. Reson. Imaging 52, 1829–1837. doi: 10.1002/jmri.27264
Joseph, C. R., and Novel, M. R. I. (2020). Techniques identifying vascular leak and paravascular flow reduction in early Alzheimer disease. Biomedicine 8:228. doi: 10.3390/biomedicines8070228
Kassner, A., Roberts, T. P., Moran, B., Silver, F. L., and Mikulis, D. J. (2009). Recombinant tissue plasminogen activator increases blood-brain barrier disruption in acute ischemic stroke: an MR imaging permeability study. AJNR Am. J. Neuroradiol. 30, 1864–1869. doi: 10.3174/ajnr.A1774
Kerkhofs, D., Wong, S. M., Zhang, E., Staals, J., Jansen, J. F. A., van Oostenbrugge, R. J., et al. (2021). Baseline blood-brain barrier leakage and longitudinal microstructural tissue damage in the periphery of white matter hyperintensities. Neurology 96, e2192–e2200. doi: 10.1212/wnl.0000000000011783
Kim, H. G., Park, S., Rhee, H. Y., Lee, K. M., Ryu, C. W., Rhee, S. J., et al. (2017). Quantitative susceptibility mapping to evaluate the early stage of Alzheimer's disease. NeuroImage Clin. 16, 429–438. doi: 10.1016/j.nicl.2017.08.019
Kress, B. T., Iliff, J. J., Xia, M., Wang, M., Wei, H. S., Zeppenfeld, D., et al. (2014). Impairment of paravascular clearance pathways in the aging brain. Ann. Neurol. 76, 845–861. doi: 10.1002/ana.24271
Larsson, C., Kleppestø, M., Rasmussen, I. Jr., Salo, R., Vardal, J., Brandal, P., et al. (2013). Sampling requirements in DCE-MRI based analysis of high grade gliomas: simulations and clinical results. J. Magn. Reson. Imaging 37, 818–829. doi: 10.1002/jmri.23866
Laurent, S., Elst, L. V., and Muller, R. N. (2006). Comparative study of the physicochemical properties of six clinical low molecular weight gadolinium contrast agents. Contrast Media Mol. Imaging 1, 128–137. doi: 10.1002/cmmi.100
Li, K. L., Buonaccorsi, G., Thompson, G., Cain, J. R., Watkins, A., Russell, D., et al. (2012). An improved coverage and spatial resolution--using dual injection dynamic contrast-enhanced (ICE-DICE) MRI: a novel dynamic contrast-enhanced technique for cerebral tumors. Magn. Reson. Med. 68, 452–462. doi: 10.1002/mrm.23252
Li, Y., Li, M., Zhang, X., Shi, Q., Yang, S., Fan, H., et al. (2017). Higher blood-brain barrier permeability is associated with higher white matter hyperintensities burden. J. Neurol. 264, 1474–1481. doi: 10.1007/s00415-017-8550-8
Li, M., Li, Y., Zuo, L., Hu, W., and Jiang, T. (2021). Increase of blood-brain barrier leakage is related to cognitive decline in vascular mild cognitive impairment. BMC Neurol. 21:159. doi: 10.1186/s12883-021-02189-6
Li, K. L., Zhu, X., Hylton, N., Jahng, G. H., Weiner, M. W., and Schuff, N. (2005). Four-phase single-capillary stepwise model for kinetics in arterial spin labeling MRI. Magn. Reson. Med. 53, 511–518. doi: 10.1002/mrm.20390
Lin, Z., Li, Y., Su, P., Mao, D., Wei, Z., Pillai, J. J., et al. (2018). Non-contrast MR imaging of blood-brain barrier permeability to water. Magn. Reson. Med. 80, 1507–1520. doi: 10.1002/mrm.27141
Lin, Z., Lim, C., Jiang, D., Soldan, A., Pettigrew, C., Oishi, K., et al. (2022). Longitudinal changes in brain oxygen extraction fraction (OEF) in older adults: relationship to markers of vascular and Alzheimer's pathology. Alzheimers Dement. doi: 10.1002/alz.12727. [Epub ahead of print].
Lin, Z., Sur, S., Soldan, A., Pettigrew, C., Miller, M., Oishi, K., et al. (2019). Brain oxygen extraction by using MRI in older individuals: relationship to apolipoprotein E genotype and amyloid burden. Radiology 292, 140–148. doi: 10.1148/radiol.2019182726
Lu, H., and Ge, Y. (2008). Quantitative evaluation of oxygenation in venous vessels using T2-relaxation-under-spin-tagging MRI. Magn. Reson. Med. 60, 357–363. doi: 10.1002/mrm.21627
Manning, C., Stringer, M., Dickie, B., Clancy, U., Valdés Hernandez, M. C., Wiseman, S. J., et al. (2021). Sources of systematic error in DCE-MRI estimation of low-level blood-brain barrier leakage. Magn. Reson. Med. 86, 1888–1903. doi: 10.1002/mrm.28833
Mehta, N. H., Suss, R. A., Dyke, J. P., Theise, N. D., Chiang, G. C., Strauss, S., et al. (2022). Quantifying cerebrospinal fluid dynamics: a review of human neuroimaging contributions to CSF physiology and neurodegenerative disease. Neurobiol. Dis. 170:105776. doi: 10.1016/j.nbd.2022.105776
Michalicova, A., Majerova, P., and Kovac, A. (2020). Tau protein and its role in blood-Brain barrier dysfunction. Front. Mol. Neurosci. 13:570045. doi: 10.3389/fnmol.2020.570045
Mikati, A. G., Tan, H., Shenkar, R., Li, L., Zhang, L., Guo, X., et al. (2014). Dynamic permeability and quantitative susceptibility: related imaging biomarkers in cerebral cavernous malformations. Stroke 45, 598–601. doi: 10.1161/strokeaha.113.003548
Mills, S. J., Soh, C., O'Connor, J. P., Rose, C. J., Buonaccorsi, G. A., Cheung, S., et al. (2009). Tumour enhancing fraction (EnF) in glioma: relationship to tumour grade. Eur. Radiol. 19, 1489–1498. doi: 10.1007/s00330-008-1288-z
Mokri, B. (2014). Spontaneous CSF leaks: low CSF volume syndromes. Neurol. Clin. 32, 397–422. doi: 10.1016/j.ncl.2013.11.002
Montagne, A., Barnes, S. R., Sweeney, M. D., Halliday, M. R., Sagare, A. P., Zhao, Z., et al. (2015). Blood-brain barrier breakdown in the aging human hippocampus. Neuron 85, 296–302. doi: 10.1016/j.neuron.2014.12.032
Montagne, A., Huuskonen, M. T., Rajagopal, G., Sweeney, M. D., Nation, D. A., Sepehrband, F., et al. (2019). Undetectable gadolinium brain retention in individuals with an age-dependent blood-brain barrier breakdown in the hippocampus and mild cognitive impairment. Alzheimers Dement. 15, 1568–1575. doi: 10.1016/j.jalz.2019.07.012
Montagne, A., Nation, D. A., Pa, J., Sweeney, M. D., Toga, A. W., and Zlokovic, B. V. (2016). Brain imaging of neurovascular dysfunction in Alzheimer's disease. Acta Neuropathol. 131, 687–707. doi: 10.1007/s00401-016-1570-0
Montagne, A., Nation, D. A., Sagare, A. P., Barisano, G., Sweeney, M. D., Chakhoyan, A., et al. (2020). APOE4 leads to blood-brain barrier dysfunction predicting cognitive decline. Nature 581, 71–76. doi: 10.1038/s41586-020-2247-3
Montagne, A., Toga, A. W., and Zlokovic, B. V. (2016). Blood-Brain barrier permeability and gadolinium: benefits and potential pitfalls in research. JAMA Neurol. 73, 13–14. doi: 10.1001/jamaneurol.2015.2960
Montagne, A., Zhao, Z., and Zlokovic, B. V. (2017). Alzheimer's disease: a matter of blood-brain barrier dysfunction? J. Exp. Med. 214, 3151–3169. doi: 10.1084/jem.20171406
Morris, A. W., Carare, R. O., Schreiber, S., and Hawkes, C. A. (2014). The cerebrovascular basement membrane: role in the clearance of β-amyloid and cerebral amyloid Angiopathy. Front. Aging Neurosci. 6:251. doi: 10.3389/fnagi.2014.00251
Nakada, T., and Kwee, I. L. (2019). Fluid dynamics inside the Brain barrier: current concept of interstitial flow, glymphatic flow, and cerebrospinal fluid circulation in the Brain. Neuroscientist 25, 155–166. doi: 10.1177/1073858418775027
Nasrallah, F. A., Pagès, G., Kuchel, P. W., Golay, X., and Chuang, K. H. (2013). Imaging brain deoxyglucose uptake and metabolism by glucoCEST MRI. J. Cereb. Blood Flow Metab. 33, 1270–1278. doi: 10.1038/jcbfm.2013.79
Nation, D. A., Sweeney, M. D., Montagne, A., Sagare, A. P., D'Orazio, L. M., Pachicano, M., et al. (2019). Blood-brain barrier breakdown is an early biomarker of human cognitive dysfunction. Nat. Med. 25, 270–276. doi: 10.1038/s41591-018-0297-y
Nedergaard, M., and Goldman, S. A. (2020). Glymphatic failure as a final common pathway to dementia. Science 370, 50–56. doi: 10.1126/science.abb8739
Nitta, T., Hata, M., Gotoh, S., Seo, Y., Sasaki, H., Hashimoto, N., et al. (2003). Size-selective loosening of the blood-brain barrier in claudin-5-deficient mice. J. Cell Biol. 161, 653–660. doi: 10.1083/jcb.200302070
Obermeier, B., Daneman, R., and Ransohoff, R. M. (2013). Development, maintenance and disruption of the blood-brain barrier. Nat. Med. 19, 1584–1596. doi: 10.1038/nm.3407
Ohene, Y., Harrison, I. F., Nahavandi, P., Ismail, O., Bird, E. V., Ottersen, O. P., et al. (2019). Non-invasive MRI of brain clearance pathways using multiple echo time arterial spin labelling: an aquaporin-4 study. NeuroImage 188, 515–523. doi: 10.1016/j.neuroimage.2018.12.026
Oresković, D., and Klarica, M. (2010). The formation of cerebrospinal fluid: nearly a hundred years of interpretations and misinterpretations. Brain Res. Rev. 64, 241–262. doi: 10.1016/j.brainresrev.2010.04.006
Palomares, J. A., Tummala, S., Wang, D. J., Park, B., Woo, M. A., Kang, D. W., et al. (2015). Water exchange across the blood-Brain barrier in obstructive sleep apnea: an MRI diffusion-weighted pseudo-continuous arterial spin labeling study. J. Neuroimaging 25, 900–905. doi: 10.1111/jon.12288
Papadopoulos, M. C., and Verkman, A. S. (2013). Aquaporin water channels in the nervous system. Nat. Rev. Neurosci. 14, 265–277. doi: 10.1038/nrn3468
Patlak, C. S., Blasberg, R. G., and Fenstermacher, J. D. (1983). Graphical evaluation of blood-to-brain transfer constants from multiple-time uptake data. J. Cereb. Blood Flow Metab. 3, 1–7. doi: 10.1038/jcbfm.1983.1
Peng, S. L., Dumas, J. A., Park, D. C., Liu, P., Filbey, F. M., McAdams, C. J., et al. (2014). Age-related increase of resting metabolic rate in the human brain. NeuroImage 98, 176–183. doi: 10.1016/j.neuroimage.2014.04.078
Piert, M., Koeppe, R. A., Giordani, B., Berent, S., and Kuhl, D. E. (1996). Diminished glucose transport and phosphorylation in Alzheimer's disease determined by dynamic FDG-PET. J. Nucl. Med. 37, 201–208.
Provenzale, J. M., York, G., Moya, M. G., Parks, L., Choma, M., Kealey, S., et al. (2006). Correlation of relative permeability and relative cerebral blood volume in high-grade cerebral neoplasms. AJR Am. J. Roentgenol. 187, 1036–1042. doi: 10.2214/ajr.04.0676
Renkin, E. M. (1959). Transport of potassium-42 from blood to tissue in isolated mammalian skeletal muscles. Am. J. Phys. 197, 1205–1210. doi: 10.1152/ajplegacy.1959.197.6.1205
Rooney, W. D., Li, X., Sammi, M. K., Bourdette, D. N., Neuwelt, E. A., and Springer, C. S. Jr. (2015). Mapping human brain capillary water lifetime: high-resolution metabolic neuroimaging. NMR Biomed. 28, 607–623. doi: 10.1002/nbm.3294
Rotta, J., Perosa, V., Yakupov, R., Kuijf, H. J., Schreiber, F., Dobisch, L., et al. (2021). Detection of cerebral microbleeds with venous connection at 7-tesla MRI. Neurology 96, e2048–e2057. doi: 10.1212/wnl.0000000000011790
Selkoe, D. J., and Hardy, J. (2016). The amyloid hypothesis of Alzheimer's disease at 25 years. EMBO Mol. Med. 8, 595–608. doi: 10.15252/emmm.201606210
Shao, X., Jann, K., Ma, S. J., Yan, L., Montagne, A., Ringman, J. M., et al. (2020). Comparison between blood-Brain barrier water exchange rate and permeability to gadolinium-based contrast agent in an elderly cohort. Front. Neurosci. 14:571480. doi: 10.3389/fnins.2020.571480
Shao, X., Ma, S. J., Casey, M., D'Orazio, L., Ringman, J. M., and Wang, D. J. J. (2019). Mapping water exchange across the blood-brain barrier using 3D diffusion-prepared arterial spin labeled perfusion MRI. Magn. Reson. Med. 81, 3065–3079. doi: 10.1002/mrm.27632
Shao, X., Wang, Y., Moeller, S., and Wang, D. J. J. (2018). A constrained slice-dependent background suppression scheme for simultaneous multi-slice pseudo-continuous arterial spin labeling. Magn. Reson. Med. 79, 394–400. doi: 10.1002/mrm.26643
Shao, X., Zhao, C., and Shou, Q. (2023). St Lawrence KS, Wang DJJ. Quantification of blood-brain barrier water exchange and permeability with multi-delay diffusion-weighted pseudo-continuous arterial spin labeling. Magn. Reson. Med. doi: 10.1002/mrm.29581. [Epub ahead of print].
Shinohara, R. T., Crainiceanu, C. M., Caffo, B. S., Gaitán, M. I., and Reich, D. S. (2011). Population-wide principal component-based quantification of blood-brain-barrier dynamics in multiple sclerosis. NeuroImage 57, 1430–1446. doi: 10.1016/j.neuroimage.2011.05.038
Silva, A. C., Zhang, W., Williams, D. S., and Koretsky, A. P. (1997). Estimation of water extraction fractions in rat brain using magnetic resonance measurement of perfusion with arterial spin labeling. Magn. Reson. Med. 37, 58–68. doi: 10.1002/mrm.1910370110
Silverberg, G. D., Heit, G., Huhn, S., Jaffe, R. A., Chang, S. D., Bronte-Stewart, H., et al. (2001). The cerebrospinal fluid production rate is reduced in dementia of the Alzheimer's type. Neurology 57, 1763–1766. doi: 10.1212/wnl.57.10.1763
Singh, A., Haris, M., Rathore, D., Purwar, A., Sarma, M., Bayu, G., et al. (2007). Quantification of physiological and hemodynamic indices using T(1) dynamic contrast-enhanced MRI in intracranial mass lesions. J. Magn. Reson. Imaging 26, 871–880. doi: 10.1002/jmri.21080
St Lawrence, K. S., Owen, D., and Wang, D. J. (2012). A two-stage approach for measuring vascular water exchange and arterial transit time by diffusion-weighted perfusion MRI. Magn. Reson. Med. 67, 1275–1284. doi: 10.1002/mrm.23104
Starr, J. M., Farrall, A. J., Armitage, P., McGurn, B., and Wardlaw, J. (2009). Blood-brain barrier permeability in Alzheimer's disease: a case-control MRI study. Psychiatry Res. 171, 232–241. doi: 10.1016/j.pscychresns.2008.04.003
Starr, J. M., Wardlaw, J., Ferguson, K., MacLullich, A., Deary, I. J., and Marshall, I. (2003). Increased blood-brain barrier permeability in type II diabetes demonstrated by gadolinium magnetic resonance imaging. J. Neurol. Neurosurg. Psychiatry 74, 70–76. doi: 10.1136/jnnp.74.1.70
Sweeney, M. D., Montagne, A., Sagare, A. P., Nation, D. A., Schneider, L. S., Chui, H. C., et al. (2019). Vascular dysfunction-the disregarded partner of Alzheimer's disease. Alzheimers Dement. 15, 158–167. doi: 10.1016/j.jalz.2018.07.222
Syvänen, S., and Eriksson, J. (2013). Advances in PET imaging of P-glycoprotein function at the blood-brain barrier. ACS Chem. Neurosci. 4, 225–237. doi: 10.1021/cn3001729
Taheri, S., Gasparovic, C., Huisa, B. N., Adair, J. C., Edmonds, E., Prestopnik, J., et al. (2011). Blood-brain barrier permeability abnormalities in vascular cognitive impairment. Stroke 42, 2158–2163. doi: 10.1161/strokeaha.110.611731
Takagi, S., Ehara, K., and Finn, R. D. (1987). Water extraction fraction and permeability-surface product after intravenous injection in rats. Stroke 18, 177–183. doi: 10.1161/01.str.18.1.177
Tao, Y., Wang, Y., Rogers, J. T., and Wang, F. (2014). Perturbed iron distribution in Alzheimer's disease serum, cerebrospinal fluid, and selected brain regions: a systematic review and meta-analysis. J. Alzheimers Dis. 42, 679–690. doi: 10.3233/jad-140396
Tarasoff-Conway, J. M., Carare, R. O., Osorio, R. S., Glodzik, L., Butler, T., Fieremans, E., et al. (2015). Clearance systems in the brain-implications for Alzheimer disease. Nat. Rev. Neurol. 11, 457–470. doi: 10.1038/nrneurol.2015.119
Tariq, S., d'Esterre, C. D., Sajobi, T. T., Smith, E. E., Longman, R. S., Frayne, R., et al. (2018). A longitudinal magnetic resonance imaging study of neurodegenerative and small vessel disease, and clinical cognitive trajectories in non demented patients with transient ischemic attack: the PREVENT study. BMC Geriatr. 18:163. doi: 10.1186/s12877-018-0858-4
Thornhill, R. E., Chen, S., Rammo, W., Mikulis, D. J., and Kassner, A. (2010). Contrast-enhanced MR imaging in acute ischemic stroke: T2* measures of blood-brain barrier permeability and their relationship to T1 estimates and hemorrhagic transformation. AJNR Am. J. Neuroradiol. 31, 1015–1022. doi: 10.3174/ajnr.A2003
Thrippleton, M. J., Backes, W. H., Sourbron, S., Ingrisch, M., van Osch, M. J. P., Dichgans, M., et al. (2019). Quantifying blood-brain barrier leakage in small vessel disease: review and consensus recommendations. Alzheimers Dement. 15, 840–858. doi: 10.1016/j.jalz.2019.01.013
Tiepolt, S., Schafer, A., Rullmann, M., Roggenhofer, E., Netherlands Brain, B., Gertz, H. J., et al. (2018). Quantitative susceptibility mapping of amyloid-beta aggregates in Alzheimer's disease with 7T MR. J. Alzheimers Dis. 64, 393–404. doi: 10.3233/JAD-180118
Tofts, P. S., Brix, G., Buckley, D. L., Evelhoch, J. L., Henderson, E., Knopp, M. V., et al. (1999). Estimating kinetic parameters from dynamic contrast-enhanced T(1)-weighted MRI of a diffusable tracer: standardized quantities and symbols. J. Magn. Reson. Imaging 10, 223–232. doi: 10.1002/(sici)1522-2586(199909)10:3<223::aid-jmri2>3.0.co;2-s
Tofts, P. S., and Kermode, A. G. (1991). Measurement of the blood-brain barrier permeability and leakage space using dynamic MR imaging 1: Fundamental concepts. Magn. Reson. Med. 17, 357–367. doi: 10.1002/mrm.1910170208
Topakian, R., Barrick, T. R., Howe, F. A., and Markus, H. S. (2010). Blood-brain barrier permeability is increased in normal-appearing white matter in patients with lacunar stroke and leucoaraiosis. J. Neurol. Neurosurg. Psychiatry 81, 192–197. doi: 10.1136/jnnp.2009.172072
Tsai, P. S., Kaufhold, J. P., Blinder, P., Friedman, B., Drew, P. J., Karten, H. J., et al. (2009). Correlations of neuronal and microvascular densities in murine cortex revealed by direct counting and co-localization of nuclei and vessels. J. Neurosci. 29, 14553–14570. doi: 10.1523/jneurosci.3287-09.2009
Uchida, Y., Kan, H., Sakurai, K., Arai, N., Inui, S., Kobayashi, S., et al. (2020). Iron leakage owing to blood-brain barrier disruption in small vessel disease CADASIL. Neurology 95, e1188–e1198. doi: 10.1212/WNL.0000000000010148
Uchida, Y., Kan, H., Sakurai, K., Horimoto, Y., Hayashi, E., Iida, A., et al. (2022a). APOE ɛ4 dose associates with increased brain iron and β-amyloid via blood-brain barrier dysfunction. J. Neurol. Neurosurg. Psychiatry 93, 772–778. doi: 10.1136/jnnp-2021-328519
Uchida, Y., Kan, H., Sakurai, K., Oishi, K., and Matsukawa, N. (2022b). Quantitative susceptibility mapping as an imaging biomarker for Alzheimer's disease: the expectations and limitations. Front. Neurosci. 16:938092. doi: 10.3389/fnins.2022.938092
van de Haar, H. J., Burgmans, S., Jansen, J. F., van Osch, M. J., van Buchem, M. A., Muller, M., et al. (2016a). Blood-Brain barrier leakage in patients with early Alzheimer disease. Radiology 281, 527–535. doi: 10.1148/radiol.2016152244
van de Haar, H. J., Jansen, J. F. A., Jeukens, C., Burgmans, S., van Buchem, M. A., Muller, M., et al. (2017). Subtle blood-brain barrier leakage rate and spatial extent: considerations for dynamic contrast-enhanced MRI. Med. Phys. 44, 4112–4125. doi: 10.1002/mp.12328
van de Haar, H. J., Jansen, J. F. A., van Osch, M. J. P., van Buchem, M. A., Muller, M., Wong, S. M., et al. (2016b). Neurovascular unit impairment in early Alzheimer's disease measured with magnetic resonance imaging. Neurobiol. Aging 45, 190–196. doi: 10.1016/j.neurobiolaging.2016.06.006
Vidarsson, L., Thornhill, R. E., Liu, F., Mikulis, D. J., and Kassner, A. (2009). Quantitative permeability magnetic resonance imaging in acute ischemic stroke: how long do we need to scan? Magn. Reson. Imaging 27, 1216–1222. doi: 10.1016/j.mri.2009.01.019
Viswanathan, A., Rocca, W. A., and Tzourio, C. (2009). Vascular risk factors and dementia: how to move forward? Neurology 72, 368–374. doi: 10.1212/01.wnl.0000341271.90478.8e
Wang, D., Chen, F., Han, Z., Yin, Z., Ge, X., and Lei, P. (2021). Relationship between amyloid-β deposition and blood-Brain barrier dysfunction in Alzheimer's disease. Front. Cell. Neurosci. 15:695479. doi: 10.3389/fncel.2021.695479
Wang, Y., Liu, J., Zhang, Z., Wang, X., and Zhang, C. (2011). Structure and permeability changes of the blood-brain barrier in APP/PS1 mice: an Alzheimer’s disease animal model. Neurochem. J. 5, 220–222. doi: 10.1134/s1819712411030135
Wardlaw, J. M., Doubal, F., Armitage, P., Chappell, F., Carpenter, T., Muñoz Maniega, S., et al. (2009). Lacunar stroke is associated with diffuse blood-brain barrier dysfunction. Ann. Neurol. 65, 194–202. doi: 10.1002/ana.21549
Wardlaw, J. M., Farrall, A., Armitage, P. A., Carpenter, T., Chappell, F., Doubal, F., et al. (2008). Changes in background blood-brain barrier integrity between lacunar and cortical ischemic stroke subtypes. Stroke 39, 1327–1332. doi: 10.1161/strokeaha.107.500124
Wardlaw, J. M., Makin, S. J., Valdés Hernández, M. C., Armitage, P. A., Heye, A. K., Chappell, F. M., et al. (2016). Blood-brain barrier failure as a core mechanism in cerebral small vessel disease and dementia: evidence from a cohort study. Alzheimers Dement. 13, 634–643. doi: 10.1016/j.jalz.2016.09.006
Wardlaw, J. M., Sandercock, P. A., Dennis, M. S., and Starr, J. (2003). Is breakdown of the blood-brain barrier responsible for lacunar stroke, leukoaraiosis, and dementia? Stroke 34, 806–812. doi: 10.1161/01.Str.0000058480.77236.B3
Wardlaw, J. M., Smith, C., and Dichgans, M. (2019). Small vessel disease: mechanisms and clinical implications. Lancet Neurol. 18, 684–696. doi: 10.1016/s1474-4422(19)30079-1
Weiss, N., Miller, F., Cazaubon, S., and Couraud, P. O. (2009). The blood-brain barrier in brain homeostasis and neurological diseases. Biochim. Biophys. Acta 1788, 842–857. doi: 10.1016/j.bbamem.2008.10.022
Weller, R. O., Galea, I., Carare, R. O., and Minagar, A. (2010). Pathophysiology of the lymphatic drainage of the central nervous system: implications for pathogenesis and therapy of multiple sclerosis. Pathophysiology 17, 295–306. doi: 10.1016/j.pathophys.2009.10.007
Wells, J. A., Lythgoe, M. F., Choy, M., Gadian, D. G., Ordidge, R. J., and Thomas, D. L. (2009). Characterizing the origin of the arterial spin labelling signal in MRI using a multiecho acquisition approach. J. Cereb. Blood Flow Metab. 29, 1836–1845. doi: 10.1038/jcbfm.2009.99
Wells, J. A., Siow, B., Lythgoe, M. F., and Thomas, D. L. (2013). Measuring biexponential transverse relaxation of the ASL signal at 9.4 T to estimate arterial oxygen saturation and the time of exchange of labeled blood water into cortical brain tissue. J. Cereb. Blood Flow Metab. 33, 215–224. doi: 10.1038/jcbfm.2012.156
Yamanaka, T., Uchida, Y., Sakurai, K., Kato, D., Mizuno, M., Sato, T., et al. (2019). Anatomical links between white matter Hyperintensity and medial temporal atrophy reveal impairment of executive functions. Aging Dis. 10, 711–718. doi: 10.14336/ad.2018.0929
Yamazaki, Y., Zhao, N., Caulfield, T. R., Liu, C. C., and Bu, G. (2019). Apolipoprotein E and Alzheimer disease: pathobiology and targeting strategies. Nat. Rev. Neurol. 15, 501–518. doi: 10.1038/s41582-019-0228-7
Yang, J., Lunde, L. K., Nuntagij, P., Oguchi, T., Camassa, L. M., Nilsson, L. N., et al. (2011). Loss of astrocyte polarization in the tg-ArcSwe mouse model of Alzheimer's disease. J. Alzheimers Dis. 27, 711–722. doi: 10.3233/jad-2011-110725
Yang, Q., Wei, X., Deng, B., Chang, Z., Jin, D., Huang, Y., et al. (2022). Cerebral small vessel disease alters neurovascular unit regulation of microcirculation integrity involved in vascular cognitive impairment. Neurobiol. Dis. 170:105750. doi: 10.1016/j.nbd.2022.105750
Zeppenfeld, D. M., Simon, M., Haswell, J. D., D'Abreo, D., Murchison, C., Quinn, J. F., et al. (2017). Association of Perivascular Localization of Aquaporin-4 with cognition and Alzheimer disease in aging brains. JAMA Neurol. 74, 91–99. doi: 10.1001/jamaneurol.2016.4370
Zeuthen, T. (2010). Water-transporting proteins. J. Membr. Biol. 234, 57–73. doi: 10.1007/s00232-009-9216-y
Zhang, C. E., Wong, S. M., van de Haar, H. J., Staals, J., Jansen, J. F., Jeukens, C. R., et al. (2017). Blood-brain barrier leakage is more widespread in patients with cerebral small vessel disease. Neurology 88, 426–432. doi: 10.1212/wnl.0000000000003556
Zhang, N., Zhang, L., Qiu, B., Meng, L., Wang, X., and Hou, B. L. (2012). Correlation of volume transfer coefficient Ktrans with histopathologic grades of gliomas. J. Magn. Reson. Imaging 36, 355–363. doi: 10.1002/jmri.23675
Keywords: Alzheimer’s disease, biomarker, blood–brain barrier, MRI, neurovascular unit
Citation: Uchida Y, Kan H, Sakurai K, Oishi K and Matsukawa N (2023) Contributions of blood–brain barrier imaging to neurovascular unit pathophysiology of Alzheimer’s disease and related dementias. Front. Aging Neurosci. 15:1111448. doi: 10.3389/fnagi.2023.1111448
Edited by:
Giulio Maria Pasinetti, Icahn School of Medicine at Mount Sinai, United StatesReviewed by:
Gloria Chiang, NewYork-Presbyterian, United StatesLiangdong Zhou, Cornell University, United States
Copyright © 2023 Uchida, Kan, Sakurai, Oishi and Matsukawa. This is an open-access article distributed under the terms of the Creative Commons Attribution License (CC BY). The use, distribution or reproduction in other forums is permitted, provided the original author(s) and the copyright owner(s) are credited and that the original publication in this journal is cited, in accordance with accepted academic practice. No use, distribution or reproduction is permitted which does not comply with these terms.
*Correspondence: Yuto Uchida, dWNoaWRheXV0bzA3MjBAeWFob28uY28uanA=; Noriyuki Matsukawa,
bm9yaW1AbWVkLm5hZ295YS1jdS5hYy5qcA==