- 1Department of Psychiatry, Renmin Hospital of Wuhan University, Wuhan, China
- 2Wuxi Mental Health Center, Nanjing Medical University, Wuxi, China
- 3Affiliated Shuyang Hospital, Nanjing University of Chinese Medicine, Suqian, China
- 4Department of Psychiatry, The First Affiliated Hospital of Xinxiang Medical University, Xinxiang, China
- 5Peking-Tsinghua Center for Life Sciences and PKU-IDG/McGovern Institute for Brain Research, Peking University, Beijing, China
- 6Medical Research Center, Binzhou Medical University Hospital, Binzhou, China
- 7Department of Neuroscience, City University of Hong Kong, Kowloon, Hong Kong SAR, China
- 8NHC Key Laboratory of Mental Health, Peking University Sixth Hospital, Peking University Institute of Mental Health, Peking University, Beijing, China
Objective: Mild cognitive impairment (MCI) is a heterogeneous syndrome characterized by cognitive impairment on neurocognitive tests but accompanied by relatively intact daily activities. Due to high variation and no objective methods for diagnosing and treating MCI, guidance on neuroimaging is needed. The study has explored the neuroimaging biomarkers using the support vector machine (SVM) method to predict MCI.
Methods: In total, 53 patients with MCI and 68 healthy controls were involved in scanning resting-state functional magnetic resonance imaging (rs-fMRI). Neurocognitive testing and Structured Clinical Interview, such as Alzheimer's Disease Assessment Scale-Cognitive Subscale (ADAS-Cog) test, Activity of Daily Living (ADL) Scale, Hachinski Ischemic Score (HIS), Clinical Dementia Rating (CDR), Montreal Cognitive Assessment (MoCA), and Hamilton Rating Scale for Depression (HRSD), were utilized to assess participants' cognitive state. Neuroimaging data were analyzed with the regional homogeneity (ReHo) and SVM methods.
Results: Compared with healthy comparisons (HCs), ReHo of patients with MCI was decreased in the right caudate. In addition, the SVM classification achieved an overall accuracy of 68.6%, sensitivity of 62.26%, and specificity of 58.82%.
Conclusion: The results suggest that abnormal neural activity in the right cerebrum may play a vital role in the pathophysiological process of MCI. Moreover, the ReHo in the right caudate may serve as a neuroimaging biomarker for MCI, which can provide objective guidance on diagnosing and managing MCI in the future.
Introduction
Mild cognitive impairment (MCI) is a heterogeneous syndrome defined as deficits on neurocognitive testing but without significant damage to activities of daily living (ADLs) (Winblad et al., 2004). Objective evidence cognitive or functional decline, labeled “subjective cognitive decline,” does not always accompany subjective awareness of cognitive impairment (Tao et al., 2021). MCI reflects that cognitive function deteriorates in neuropsychological testing, but ADL is intact relatively (Petersen, 2006).
One characteristic of MCI is heterogeneous, which means that about 5–15% of the patients are at the risk of getting dementia every year. At the same time, about half of the patients keep stable at 5 years, and symptoms sort out over time in the minority (Dunne et al., 2021). The collaboration of Cohort Studies of Memory in an International Consortium (COSMIC), which spread standard diagnostic criteria to estimate the prevalence of MCI more reliably across different regions, confirmed the prevalence of MCI in adults over 60 years up to 6% across 11 studies (Sachdev et al., 2015).
The guideline date updated by the American Academy of Neurology estimated a prevalence of 6.7% in 65–69-year-olds and 25% for 80–84-year-olds (Livingston et al., 2017). Jia et al. estimated that overall MCI prevalence was 15.5% in China, representing 38.77 million people (Jia et al., 2020). Consistently, 10 million new instances of dementia are enlisted, and it is assessed that 13,500,000 individuals are associated with the risk of dementia by 2050 (WHO., 2022). It presents an opportunity for reducing vascular risk and altering behavioral patterns when a diagnosis of MCI is made. Given diagnostic and therapeutic practice variation, things could change if a more definitive diagnosis is available. Therefore, standard national guidance is eagerly needed for using neuroimaging in MCI (Gillis et al., 2019; McWhirter et al., 2020). There is a need for precise prognosis methods to ensure that the diagnosis of MCI is a possibility for people to prevent the risk of Alzheimer's disease (AD). To improve the specificity and sensitivity of MCI diagnosis, we need cognitive testing and neuroimaging with more evidence.
Resting-state functional magnetic resonance imaging (rs-fMRI) is a non-invasive imaging tool to detect brain activity (Biswal et al., 1995; Smitha et al., 2017). As shown in previous research, rs-fMRI has been confirmed as a reliable instrument to explore the brain's mechanism, reflecting the signature of the brain's neural network (van den Heuvel and Hulshoff Pol, 2010; Pan et al., 2021). Regional homogeneity (ReHo) is a test-retest method to detect the characteristic of brain connectome (Biswal, 2012; Cheng et al., 2019; Geng et al., 2019; Hou et al., 2021). The support vector machine (SVM) is among the most popular methods in the machine learning (ML) that has exceeded practical neuroimage analysis in the past 20 years (Orru et al., 2012; Pisner and Schnyer, 2020). SVM has been applied in different fields and addresses various classification problems because of its relative simplicity and flexibility (Gori et al., 2015; Cheng et al., 2021; Shan et al., 2021; Gao et al., 2022). There have been hundreds of studies using ML to accurately classify patients with heterogeneous mental and neurodegenerative disorders, which make improvements in the early diagnosis.
In brain disorders research, SVMs are deployed by multivoxel pattern analysis (MVPA) and solve plenty of clinical problems because of their relative simplicity. Even if data are high dimensional, there is a lower risk of overfitting in SVMs (Zhang et al., 2020). Recently, SVMs have been applied in precision medicine. SVM analysis can predict the diagnosis and prognosis, particularly for patients with brain diseases, such as Alzheimer's (Franzmeier et al., 2020; Xie et al., 2022), schizophrenia's (Chand et al., 2022; Lei et al., 2022), and depression's (Al-Hakeim et al., 2019; Bone et al., 2021). The results would make improvement in the diagnostic accuracy.
More research participation is required to increase the investment in neuroimaging biomarkers to achieve this goal. To determine whether there are distinct or specific alterations in MCI that can be used to differentiate MCI from healthy controls, we examined specific or distinctive alterations in MCI. The study hypothesized that patients with MCI have abnormal ReHo in the brain region and the abnormal region serves as a biomarker to aid in diagnosing and prediction of MCI.
Methods
Participants and procedures
In total, 140 subjects were consecutively recruited from Wuxi Mental Health Center in China between June 2018 and May 2022.
In total, 70 patients with MCI and 70 age-, education-, and gender-matched healthy subjects, which were right-handed, were recruited. The patients were diagnosed by two psychiatrists with criteria in the Structured Clinical Interview of the fifth version of the Diagnostic and Statistical Manual of Mental Disorders (DSM-V) independently. All participants were assessed by Alzheimer's Disease Assessment Scale-Cognitive Subscale (ADAS-Cog) test (Kueper et al., 2018), ADL Scale, Hachinski Ischemic Score (HIS), Hamilton Rating Scale for Depression-17 (HRSD), Clinical Dementia Rating (CDR), and Montreal Cognitive Assessment (MoCA). The patients who met the criteria (CDR ≥ 0.5, MoCA <26) were included. Exclusion criteria were as follows: history of brain damage, other neurological disorders, other mental disorders, such as acute illness, substance abuse, schizophrenia, dementia, bipolar disorder, and other severe limb or head tremor. All healthy comparisons (HCs) were recruited from the community as volunteers without a history of neurologic disorders. In control comparisons, neuropsychology evaluations were also performed the same as patients with MCI. The study was approved by the Medical Ethics Committee of Wuxi Mental Health Center. Each participant had written informed consent and submitted it before enrollment.
Materials
ADAS-Cog test
The ADAS-Cog helps to evaluate cognition and differentiation between normal and impaired cognitive functioning. The administrator accumulates points for the errors in the test task for a total score. The score ranges from 0 to 70 and a score of 70 epitomizes the most severe impairment (Cano et al., 2010).
The ADL scale
The ADL is used to describe fundamental skills in taking care of oneself independently (Edemekong et al., 2022). ADL is used as an indicator of a person's functional status and to assess older adults' functional status (Árnadóttir, 2016).
Hachinski ischemic score
The vascular burden is considered a risk factor for cognitive dysfunction. HIS is a clinical tool used to identify vascular dementia (Johnson et al., 2014). The scores were related to cognitive functioning, such as global cognition, executive functioning, immediate memory, and attention. HIS can significantly predict the diagnosis of MCI (Paul et al., 2003).
Hamilton rating scale for depression
A 17-item HRSD was used to assess the symptom of depression (Hamilton, 1967).
Clinical dementia rating
Clinical Dementia Rating is a global rating device calculated on six behavioral and cognitive fields, such as memory, orientation, problem solving, judgment, personal care, and home and hobbies performance. The CDR is based on a scale ranging from 0 to 3: no dementia (CDR = 0), questionable dementia (CDR = 0.5), MCI (CDR = 1), moderate cognitive impairment (CDR = 2), and severe cognitive impairment (CDR = 3) (Khan, 2016).
Montreal cognitive assessment
Montreal Cognitive Assessment score ranges from 0 to 30 points, and a cut score of 26 represents excellent specificity and sensitivity separating MCI from healthy subjects (De Reuck, 2020).
Image acquisition
All the rs-MRI data were obtained by an Achieva 3.0T Scanner (Philips, Amsterdam, the Netherlands) at the Wuxi Mental Health Center on the first day. Participants were instructed to remain still, close their eyes, and avoid falling asleep during the scan. Echo-planar imaging of resting-state functional images was performed using the following parameters: repetition time/echo time (TR/TE) 2,000/30 ms, 31 slices, 90° flip angles, 22 cm × 22 cm field of view (FOV), 5 mm slice thickness, and 1 mm pitch.
Data preprocessing
Imaging data were preprocessed with Data Processing Assistant for Resting-State (DPARSF) software in MATLAB (Yan et al., 2016). It was necessary to exclude the first five time points to minimize the error caused by the initial signal instability and participants' adaptation times. Following this, time-slice correction and head movement correction were completed. Maximum displacements in x-, y-, or z-axes and maximum rotations in angular direction were no more than 2 mm and 2°, respectively. Image data were corrected for spatial normalization to the standard Montreal Neurological Institute (MNI) space. Samples were resampled to 3 × 3 × 3 mm. Filter was used along with linear detrending (0.01–0.08 Hz) and linearly detrended. A series of covariates were removed that included the signal from a region centered in the white matter, and six head-motion parameters were calculated from rigid bodies (Gao et al., 2022).
ReHo analyses
Using the Kendall coefficient of concordance (KCC) between the time series of a given voxel and the 26 nearest voxels, we generated the ReHo map for each participant. The non-brain tissues were then removed using a whole-brain mask. As part of the standardization process, we divided each ReHo map by its global mean KCC within the whole-brain mask. Spatial smoothing of the ReHo maps was performed with a Gaussian kernel of 4.5 mm full-width at half-maximum (FWHM).
Statistical analysis
With the help of two-sample t-tests, age, education, and whole-brain voxel-based maps were compared. The gender was compared by the chi-square test. By controlling topological family-wise errors (FWEs) computed using Gaussian Random Field theory, all significant clusters were corrected at the cluster level. The threshold was p < 0.01 for cluster-forming voxel-level heights, and the threshold was p < 0.05 for cluster-wise FWEs. Gray matter (GM) masks were generated group wise, i.e., voxels.
Classification analysis
For each participant, we calculated the Pearson correlation between the time series of all pairs of brain voxels. In MATLAB, LIBSVM was used to run the SVM method. With ReHo values extracted from different brain regions, SVM was applied to test the ability to distinguish patients from healthy controls.
Results
Demographics
From June 2018 to May 2022, 140 participants were enrolled. Among all participants, 17 patients and 2 HCs were excluded because of the head motion parameters. The remaining 53 patients with MCI and 68 age-, education-, and sex-matched control volunteers were recruited. The subjects were divided into 2 groups: patients with MCI (n = 53) and HCs (n = 68). Demographic information and clinical assessment scores are shown in Table 1. There were no significant differences in age, gender, and year of education between the two groups (p > 0.05). Compared with the HCs, MCI patients has poorer scores in all cognition tests, including ADAS-Cog test (p < 0.05), HIS (p < 0.05), CDR (p < 0.05), and MoCA (p < 0.05). There was no significance in the ADL Scale and HRSD between groups (p > 0.05, Table 1).
ReHO: Patients vs. HCs
Compared with HCs, patients with MCI displayed a significantly decreased ReHo in the right caudate (Table 2 and Figure 1).
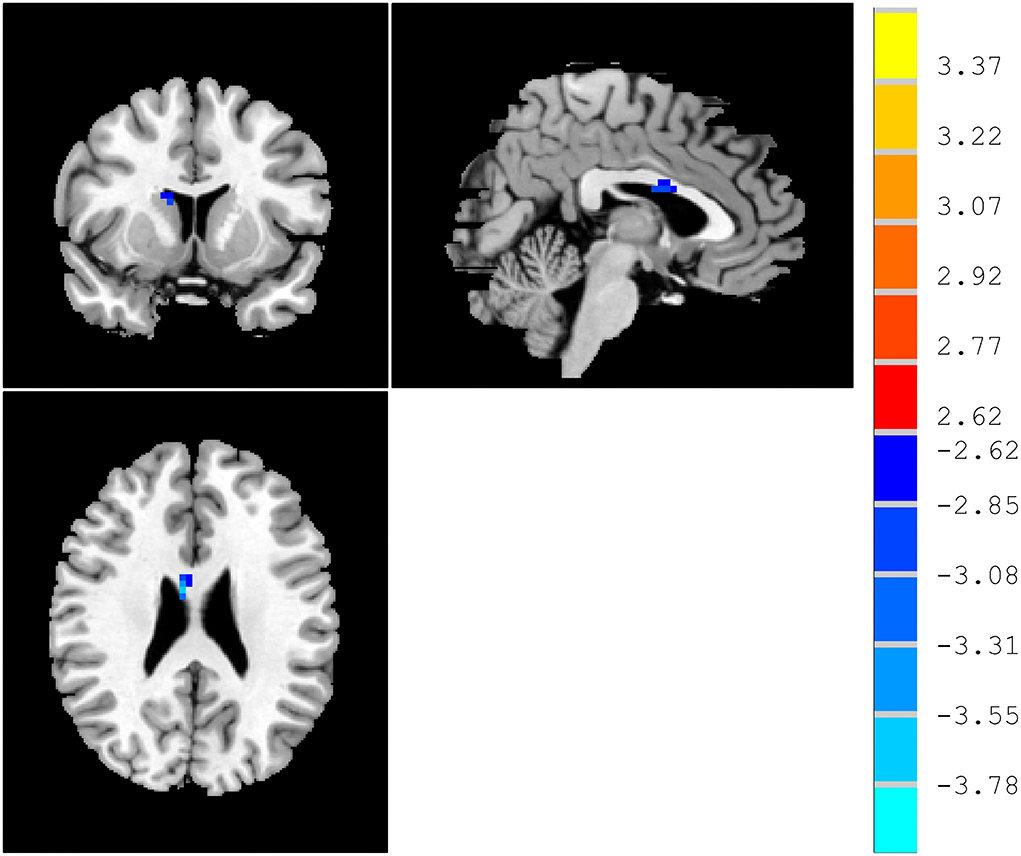
Figure 1. ReHo differences between patients with MCI and HCs. Red and blue denote higher and lower ReHo, respectively, and the color bars represent the t-values from the t-test of the group analysis. ReHo, regional homogeneity; MCI, mild cognitive impairment; HCs, healthy comparisons.
The results of the SVM
A combination of decreased ReHo in right caudate was a potential biomarker to diagnose MCI by SVM. The SVM classification achieved an overall accuracy (68.6%), sensitivity (62.26%), and specificity (58.82%) (Figure 2).
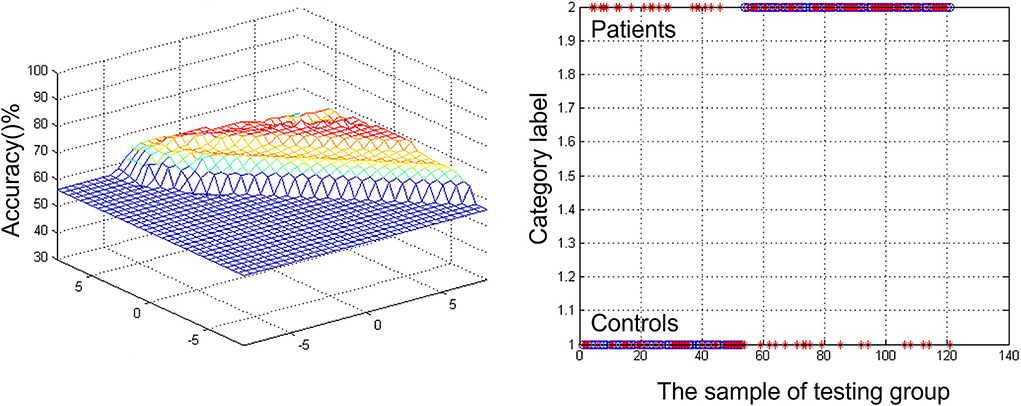
Figure 2. Visualizing classifications based on support vector machine (SVM) by the decreased regional homogeneity (ReHo) values in the right caudate to discriminate patients with mild cognitive impairment (MCI) from healthy comparisons. (Left) SVM parameters' result of 3D view. (Right) Classified map of the ReHo values in the right caudate.
Discussion
Mild cognitive impairment could be a prodromal stage of dementia. Over the past decade, neuroimaging has gained increasing attention as a predictor of mental disorders (Grueso and Viejo-Sobera, 2021). The association between MCI and right caudate was assessed in fMRI comprising 53 MCI patients with a range of demographic characteristics, and HCs were matched to patients. As shown in the results, when compared with the HCs, patients with MCI had poorer scores in all cognition tests, such as ADAS-Cog test (p < 0.05), HIS (p < 0.05), CDR (p < 0.05), and MoCA (p < 0.05). There was no significance in the ADL Scale and HRSD between the two groups (p > 0.05). Patients with MCI at times will ultimately advance to dementia, yet the cognitive symptoms have not ultimately shown.
Compared with HCs, the ReHo of patients with MCI in the right caudate is lower than HCs. The caudate nucleus plays a critical role in various higher neurological functions. It is a region that is not only in executive functioning but also related to learning, memory, motivation, and emotion (Fisher et al., 2006; Grahn et al., 2008). One can consider the head of the caudate nucleus as the cognitive and emotional portion (Seger and Cincotta, 2005; Graff-Radford et al., 2017). It is possible that the caudate was restricted from overactivity due to its significant involvement in the working memory task. Ekman et al. found in patients with Parkinson's disease and MCI, blood-oxygen-level-dependent (BOLD) signals are reduced in the right caudate and frontal cortex, as well as impaired presynaptic function in the caudate (Ekman et al., 2012). Working memory is updated by the caudate and anterior cingulate cortex and their functional changes are associated with Parkinson's disease with MCI. Specifically, Qiu et al. found that MCI smokers showed decreased functional connectivity of right caudate to left inferior parietal lobule (Qiu et al., 2022). Compared to controls, caudate volumes were lower in MCI (4.43% right) (Madsen et al., 2010).
Nevertheless, in the early stages, although biomarkers might be found in the result of fMRI, it stays trying for the location of MCI to dementia progression in clinical practice (Zhang et al., 2012). For this reason, finding the contrast between those patients with MCI and healthy subjects is significant. To tackle this issue, researchers established neuroimaging datasets from patients with MCI, HCs, and different variables, such as demographic, genetic, and cognitive measurements (Pellegrini et al., 2018). SVM can characterize non-linear choice limits in high-layered variable space by tackling a quadratic improvement issue (Grueso and Viejo-Sobera, 2021). This technique can provide a valuable insight into a disease that occurs with neural patterns, such as autism (Gori et al., 2015), attention deficit hyperactivity disorder (Park et al., 2016), and schizophrenia (Cheng et al., 2015; Pina-Camacho et al., 2015; Arbabshirani et al., 2017). In the study, the decreased ReHo of right caudate was a potential biomarker to diagnose MCI by SVM. The SVM results showed a diagnostic accuracy of 68.6% (83/121). The ReHo was decreased in the right caudate, with a sensitivity of 62.26% (33/53) and a specificity of 58.82% (40/68). The level of prediction may seem modest, but it must be viewed in the context of other risk factors that patients may have for progression over the next 20–30 years. Since these patients usually coexist, it is crucial to understand how they interact. The results suggest that future therapy studies should directly verify the ability to deliver compounds to the caudate nucleus as the cognitive and emotional portion.
In summary, this study is the first to demonstrate the relation between the right cerebrum and MCI using ML based on fMRI imaging data. The right cerebrum may play an important role in early intervention in patients with MCI. It would be interesting to combine ReHo value and MCI to fully investigate its diagnostic accuracy.
They can serve as an objective and reliable complementary tool to improve diagnosis accuracy and ultimately predict the MCI. In addition, by classifying biomarkers, it is possible to revise clinical diagnosis in cases of uncertain diagnosis. The results add to a superior comprehension of the mechanism in the cognitive decline of patients with MCI.
Limitation
With regard to the research methods, some limitations need to be acknowledged. First, cross-sectional design limits our ability to interpret causal relationships between patients with MCI and HCs. This study needs to be confirmed in the future to increase the likelihood of generalizing its results to MCI patients with different clinical characteristics. Second, several factors confound our study, such as illness duration, medication, lifestyle, and diet. In the future, we will explore potential variations. Third, there was no information available about whether changes occurred in the caudate before the development of MCI. Understanding the cause and effect may be possible through long-term follow-up observations. Fourth, we do not make the use of the medial temporal lobe atrophy (MTA) score (Scheltens' scale) in distinguishing patients with MCI and AD from those without impairment.
Conclusion
In conclusion, the results suggest that abnormal neural activity in the right cerebrum plays a vital role in the process of MCI. Moreover, the ReHo in right caudate may serve as a neuroimaging biomarker for MCI, which can provide objective guidance on diagnosing and managing MCI in the future.
Data availability statement
The raw data supporting the conclusions of this article will be made available by the authors, without undue reservation.
Ethics statement
The studies involving human participants were reviewed and approved by Medical Ethics Committee of Wuxi Mental Health Center. The patients/participants provided their written informed consent to participate in this study. Written informed consent was obtained from the individual(s) for the publication of any potentially identifiable images or data included in this article.
Author contributions
GW, YZ, and FS: conception and design. YG: statistical analysis. YZ: drafting of the manuscript. XZ, JH, and SW: conduction. XC and ML: critical revision of the manuscript. All authors read and approved the final paper.
Conflict of interest
The authors declare that the research was conducted in the absence of any commercial or financial relationships that could be construed as a potential conflict of interest.
Publisher's note
All claims expressed in this article are solely those of the authors and do not necessarily represent those of their affiliated organizations, or those of the publisher, the editors and the reviewers. Any product that may be evaluated in this article, or claim that may be made by its manufacturer, is not guaranteed or endorsed by the publisher.
References
Al-Hakeim, H. K., Al-Fadhel, S. Z., Al-Dujaili, A. H., Carvalho, A., Sriswasdi, S., Maes, M., et al. (2019). Development of a novel neuro-immune and opioid-associated fingerprint with a cross-validated ability to identify and authenticate unknown patients with major depression: far beyond differentiation, discrimination, and classification. Mol. Neurobiol. 56, 7822–7835. doi: 10.1007/s12035-019-01647-0
Arbabshirani, M. R., Plis, S., Sui, J., and Calhoun, V. D. (2017). Single subject prediction of brain disorders in neuroimaging: Promises and pitfalls. Neuroimage 145, 137–165. doi: 10.1016/j.neuroimage.2016.02.079
Árnadóttir, G. (2016). “Chapter 26 - Impact of neurobehavioral deficits on activities of daily living,” in: G. Gillen, (Ed.), Stroke Rehabilitation (Fourth Edition), Mosby. p. 573–611. doi: 10.1016/B978-0-323-17281-3.00026-5
Biswal, B., Yetkin, F. Z., Haughton, V. M., and Hyde, J. S. (1995). Functional connectivity in the motor cortex of resting human brain using echo-planar MRI. Magn. Reson. Med. 34, 537–541. doi: 10.1002/mrm.1910340409
Biswal, B. B. (2012). Resting state fMRI: a personal history. Neuroimage 62, 938–944. doi: 10.1016/j.neuroimage.2012.01.090
Bone, C., Simmonds-Buckley, M., Thwaites, R., Sandford, D., Merzhvynska, M., Rubel, J., et al. (2021). Dynamic prediction of psychological treatment outcomes: development and validation of a prediction model using routinely collected symptom data. Lancet Digit Health 3, e231–e240. doi: 10.1016/S2589-7500(21)00018-2
Cano, S. J., Posner, H. B., Moline, M. L., Hurt, S. W., Swartz, J., Hsu, T., et al. (2010). The ADAS-cog in Alzheimer's disease clinical trials: psychometric evaluation of the sum and its parts. J. Neurol. Neurosurg. Psychiat. 81, 1363–1368. doi: 10.1136/jnnp.2009.204008
Chand, G. B., Singhal, P., Dwyer, D. B., Wen, J., Erus, G., Doshi, J., et al. (2022). Schizophrenia imaging signatures and their associations with cognition, psychopathology, and genetics in the general population. Am. J. Psychiatry. doi: 10.1176/appi.ajp.21070686. [Epub ahead of print].
Cheng, H., Newman, S., Goni, J., Kent, J. S., Howell, J., Bolbecker, A., et al. (2015). Nodal centrality of functional network in the differentiation of schizophrenia. Schizophr. Res. 168, 345–352. doi: 10.1016/j.schres.2015.08.011
Cheng, J., Liu, Z., Guan, H., Wu, Z., Zhu, H., Jiang, J., et al. (2021). Brain age estimation from MRI using cascade networks with ranking loss. IEEE Trans. Med. Imaging 40, 3400–3412. doi: 10.1109/TMI.2021.3085948
Cheng, J., Yang, H., and Zhang, J. (2019). Donepezil's effects on brain functions of patients with Alzheimer disease: a regional homogeneity study based on resting-state functional magnetic resonance imaging. Clin. Neuropharmacol. 42, 42–48. doi: 10.1097/WNF.0000000000000324
De Reuck, J. (2020). “Chapter 1 - Mixed dementia: a neuropathological overview,” in Diagnosis and Management in Dementia, eds C. R. Martin, and V. R. Preedy (Lille: Academic Press), 3–15. doi: 10.1016/B978-0-12-815854-8.00001-X
Dunne, R. A., Aarsland, D., O'Brien, J. T., Ballard, C., Banerjee, S., Fox, N. C., et al. (2021). Mild cognitive impairment: the Manchester consensus. Age Ageing 50, 72–80. doi: 10.1093/ageing/afaa228
Edemekong, P. F., Bomgaars, D. L., Sukumaran, S., and Levy, S. B. (2022). Activities of Daily Living. Treasure Island, FL: StatPearls.
Ekman, U., Eriksson, J., Forsgren, L., Mo, S. J., Riklund, K., Nyberg, L., et al. (2012). Functional brain activity and presynaptic dopamine uptake in patients with Parkinson's disease and mild cognitive impairment: a cross-sectional study. Lancet Neurol. 11, 679–687. doi: 10.1016/S1474-4422(12)70138-2
Fisher, H. E., Aron, A., and Brown, L. L. (2006). Romantic love: a mammalian brain system for mate choice. Philos. Trans. R Soc. Lond. B Biol. Sci. 361, 2173–2186. doi: 10.1098/rstb.2006.1938
Franzmeier, N., Koutsouleris, N., Benzinger, T., Goate, A., Karch, C. M., Fagan, A. M., et al. (2020). Predicting sporadic Alzheimer's disease progression via inherited Alzheimer's disease-informed machine-learning. Alzheimers Dement 16, 501–511. doi: 10.1002/alz.12032
Gao, Y., Xiong, Z., Wang, X., Ren, H., Liu, R., Bai, B., et al. (2022). Abnormal degree centrality as a potential imaging biomarker for right temporal lobe epilepsy: a resting-state functional magnetic resonance imaging study and support vector machine analysis. Neuroscience 487, 198–206. doi: 10.1016/j.neuroscience.2022.02.004
Geng, J., Yan, R., Shi, J., Chen, Y., Mo, Z., Shao, J., et al. (2019). Altered regional homogeneity in patients with somatic depression: A resting-state fMRI study. J. Affect Disord. 246, 498–505. doi: 10.1016/j.jad.2018.12.066
Gillis, C., Mirzaei, F., Potashman, M., Ikram, M. A., and Maserejian, N. (2019). The incidence of mild cognitive impairment: A systematic review and data synthesis. Alzheimers Dement (Amst) 11, 248–256. doi: 10.1016/j.dadm.2019.01.004
Gori, I., Giuliano, A., Muratori, F., Saviozzi, I., Oliva, P., Tancredi, R., et al. (2015). Gray matter alterations in young children with autism spectrum disorders: comparing morphometry at the voxel and regional level. J. Neuroimaging 25, 866–874. doi: 10.1111/jon.12280
Graff-Radford, J., Williams, L., Jones, D. T., and Benarroch, E. E. (2017). Caudate nucleus as a component of networks controlling behavior. Neurology 89, 2192–2197. doi: 10.1212/WNL.0000000000004680
Grahn, J. A., Parkinson, J. A., and Owen, A. M. (2008). The cognitive functions of the caudate nucleus. Prog. Neurobiol. 86, 141–155. doi: 10.1016/j.pneurobio.2008.09.004
Grueso, S., and Viejo-Sobera, R. (2021). Machine learning methods for predicting progression from mild cognitive impairment to Alzheimer's disease dementia: a systematic review. Alzheimers Res. Ther. 13, 162. doi: 10.1186/s13195-021-00900-w
Hamilton, M. (1967). Development of a rating scale for primary depressive illness. Br. J. Soc. Clin. Psychol. 6, 278–296. doi: 10.1111/j.2044-8260.1967.tb00530.x
Hou, Z., Kong, Y., Yin, Y., Zhang, Y., and Yuan, Y. (2021). Identification of first-episode unmedicated major depressive disorder using pretreatment features of dominant coactivation patterns. Prog. Neuropsychopharmacol. Biol. Psychiat. 104, 110038. doi: 10.1016/j.pnpbp.2020.110038
Jia, L., Du, Y., Chu, L., Zhang, Z., Li, F., Lyu, D., et al. (2020). Prevalence, risk factors, and management of dementia and mild cognitive impairment in adults aged 60 years or older in China: a cross-sectional study. Lancet Public Health 5, e661–e671. doi: 10.1016/S2468-2667(20)30185-7
Johnson, L. A., Cushing, B., Rohlfing, G., Edwards, M., Davenloo, H., D'Agostino, D., et al. (2014). The Hachinski ischemic scale and cognition: the influence of ethnicity. Age Ageing 43, 364–369. doi: 10.1093/ageing/aft189
Khan, T. K. (2016). “Chapter 2 - Clinical Diagnosis of Alzheimer's Disease,” in Biomarkers in Alzheimer's Disease, ed T. K. Khan (Morgantown, WV: Academic Press), 27–48. doi: 10.1016/B978-0-12-804832-0.00002-X
Kueper, J. K., Speechley, M., and Montero-Odasso, M. (2018). The Alzheimer's disease assessment scale-cognitive subscale (ADAS-Cog): modifications and responsiveness in pre-dementia populations. a narrative review. J. Alzheimers Dis. 63, 423–444. doi: 10.3233/JAD-170991
Lei, D., Qin, K., Pinaya, W. H. L., Young, J., Van Amelsvoort, T., Marcelis, M., et al. (2022). Graph convolutional networks reveal network-level functional dysconnectivity in schizophrenia. Schizophrenia Bull. doi: 10.1093/schbul/sbac047. [Epub ahead of print].
Livingston, G., Sommerlad, A., Orgeta, V., Costafreda, S. G., Huntley, J., Ames, D., et al. (2017). Dementia prevention, intervention, and care. Lancet 390, 2673–2734. doi: 10.1016/S0140-6736(17)31363-6
Madsen, S. K., Ho, A. J., Hua, X., Saharan, P. S., Toga, A. W., Jack, C. R., et al. (2010). 3D maps localize caudate nucleus atrophy in 400 Alzheimer's disease, mild cognitive impairment, and healthy elderly subjects. Neurobiol. Aging 31, 1312–1325. doi: 10.1016/j.neurobiolaging.2010.05.002
McWhirter, L., Ritchie, C., Stone, J., and Carson, A. (2020). Functional cognitive disorders: a systematic review. Lancet Psychiat. 7, 191–207. doi: 10.1016/S2215-0366(19)30405-5
Orru, G., Pettersson-Yeo, W., Marquand, A. F., Sartori, G., and Mechelli, A. (2012). Using Support Vector Machine to identify imaging biomarkers of neurological and psychiatric disease: a critical review. Neurosci. Biobehav. Rev. 36, 1140–1152. doi: 10.1016/j.neubiorev.2012.01.004
Pan, Y., Liu, Z., Xue, Z., Sheng, Y., Cai, Y., Cheng, Y., et al. (2021). Abnormal network properties and fiber connections of DMN across major mental disorders: a probability tracing and graph theory study. Cereb Cortex. 32, 3127–3136. doi: 10.1093/cercor/bhab405
Park, B. Y., Kim, M., Seo, J., Lee, J. M., and Park, H. (2016). Connectivity analysis and feature classification in attention deficit hyperactivity disorder sub-types: a task functional magnetic resonance imaging study. Brain Topogr. 29, 429–439. doi: 10.1007/s10548-015-0463-1
Paul, R. H., Cohen, R. A., Moser, D. J., Ott, B. R., Sethi, M., Sweet, L., et al. (2003). Clinical correlates of cognitive decline in vascular dementia. Cogn. Behav. Neurol. 16, 40–46. doi: 10.1097/00146965-200303000-00005
Pellegrini, E., Ballerini, L., Hernandez, M., Chappell, F. M., Gonzalez-Castro, V., Anblagan, D., et al. (2018). Machine learning of neuroimaging for assisted diagnosis of cognitive impairment and dementia: A systematic review. Alzheimers Dement (Amst) 10, 519–535. doi: 10.1016/j.dadm.2018.07.004
Petersen, R. C. (2006). Mild cognitive impairment. Lancet 367, 1979. doi: 10.1016/S0140-6736(06)68881-8
Pina-Camacho, L., Garcia-Prieto, J., Parellada, M., Castro-Fornieles, J., Gonzalez-Pinto, A. M., Bombin, I., et al. (2015). Predictors of schizophrenia spectrum disorders in early-onset first episodes of psychosis: a support vector machine model. Eur. Child Adolesc. Psychiatry 24, 427–440. doi: 10.1007/s00787-014-0593-0
Pisner, D. A., and Schnyer, D. M. (2020). “Chapter 6 - Support vector machine,” in Machine Learning, eds A. Mechelli and S. Vieira (Austin, TX: Academic Press), 101–121. doi: 10.1016/B978-0-12-815739-8.00006-7
Qiu, T., Xie, F., Zeng, Q., Shen, Z., Du, G., Xu, X., et al. (2022). Interactions between cigarette smoking and cognitive status on functional connectivity of the cortico-striatal circuits in individuals without dementia: A resting-state functional MRI study. CNS Neurosci Ther. doi: 10.1111/cns.13852. [Epub ahead of print].
Sachdev, P. S., Lipnicki, D. M., Kochan, N. A., Crawford, J. D., Thalamuthu, A., Andrews, G., et al. (2015). The prevalence of Memory in an International, The Prevalence of Mild Cognitive Impairment in Diverse Geographical and Ethnocultural Regions: The COSMIC Collaboration. PLoS ONE 10, e0142388. doi: 10.1371/journal.pone.0142388
Seger, C. A., and Cincotta, C. M. (2005). The roles of the caudate nucleus in human classification learning. J. Neurosci. 25, 2941–2951. doi: 10.1523/JNEUROSCI.3401-04.2005
Shan, X., Cui, X., Liu, F., Li, H., Huang, R., Tang, Y., et al. (2021). Shared and distinct homotopic connectivity changes in melancholic and non-melancholic depression. J. Affect. Disord. 287, 268–275. doi: 10.1016/j.jad.2021.03.038
Smitha, K. A., Akhil Raja, K., Arun, K. M., Rajesh, P. G., Thomas, B., Kapilamoorthy, T. R., et al. (2017). Resting state fMRI: A review on methods in resting state connectivity analysis and resting state networks. Neuroradiol. J. 30, 305–317. doi: 10.1177/1971400917697342
Tao, W., Li, H., Li, X., Huang, R., Shao, W., Guan, Q., et al. (2021). Progressive brain degeneration from subjective cognitive decline to amnestic mild cognitive impairment: evidence from large-scale anatomical connection classification analysis. Front. Aging Neurosci. 13, 687530. doi: 10.3389/fnagi.2021.687530
van den Heuvel, M. P., and Hulshoff Pol, H. E. (2010). Exploring the brain network: a review on resting-state fMRI functional connectivity. Eur. Neuropsychopharmacol. 20, 519–534. doi: 10.1016/j.euroneuro.2010.03.008
WHO. (2022). Dementia. Available online at: https://www.who.int/health-topics/dementia (accessed June 26, 2022).
Winblad, B., Palmer, K., Kivipelto, M., Jelic, V., Fratiglioni, L., Wahlund, L. O., et al. (2004). Mild cognitive impairment–beyond controversies, towards a consensus: report of the International Working Group on Mild Cognitive Impairment. J. Intern. Med. 256, 240–246. doi: 10.1111/j.1365-2796.2004.01380.x
Xie, C., Zhuang, X. X., Niu, Z., Ai, R., Lautrup, S., Zheng, S., et al. (2022). Amelioration of Alzheimer's disease pathology by mitophagy inducers identified via machine learning and a cross-species workflow. Nat. Biomed. Eng. 6, 76–93. doi: 10.1038/s41551-021-00819-5
Yan, C. G., Wang, X. D., Zuo, X. N., and Zang, Y. F. (2016). DPABI: data processing and analysis for (Resting-State) brain imaging. Neuroinformatics 14, 339–351. doi: 10.1007/s12021-016-9299-4
Zhang, D., Shen, D., and Alzheimer's Disease Neuroimaging, I. (2012). Multi-modal multi-task learning for joint prediction of multiple regression and classification variables in Alzheimer's disease. Neuroimage 59, 895–907. doi: 10.1016/j.neuroimage.2011.09.069
Keywords: mild cognitive impairment, neuroimaging, fMRI, regional homogeneity, support vector machine, machine learning, biomarker
Citation: Gao Y, Zhao X, Huang J, Wang S, Chen X, Li M, Sun F, Wang G and Zhong Y (2022) Abnormal regional homogeneity in right caudate as a potential neuroimaging biomarker for mild cognitive impairment: A resting-state fMRI study and support vector machine analysis. Front. Aging Neurosci. 14:979183. doi: 10.3389/fnagi.2022.979183
Received: 27 June 2022; Accepted: 12 August 2022;
Published: 01 September 2022.
Edited by:
Yuzhen Xu, The Second Affiliated Hospital of Shandong First Medical University, ChinaReviewed by:
Xize Jia, Hangzhou Normal University, ChinaFrancesco Amenta, University of Camerino, Italy
Copyright © 2022 Gao, Zhao, Huang, Wang, Chen, Li, Sun, Wang and Zhong. This is an open-access article distributed under the terms of the Creative Commons Attribution License (CC BY). The use, distribution or reproduction in other forums is permitted, provided the original author(s) and the copyright owner(s) are credited and that the original publication in this journal is cited, in accordance with accepted academic practice. No use, distribution or reproduction is permitted which does not comply with these terms.
*Correspondence: Fengjiao Sun, bydxsfj@126.com; Gaohua Wang, wgh6402@163.com; Yi Zhong, zhongyisq@gmail.com
†These authors have contributed equally to this work and share first authorship