- 1Department of Rheumatology and Immunology, Affiliated Hospital of Jiangsu University, Zhenjiang, China
- 2School of Life Sciences, Jiangsu University, Zhenjiang, China
- 3Institute of Animal Husbandry, Jiangsu Academy of Agricultural Sciences, Nanjing, China
- 4Children’s Center, The Affiliated Taian City Central Hospital of Qingdao University, Taian, China
The preclinical diagnosis and clinical practice for Alzheimer’s disease (AD) based on liquid biopsy have made great progress in recent years. As liquid biopsy is a fast, low-cost, and easy way to get the phase of AD, continual efforts from intense multidisciplinary studies have been made to move the research tools to routine clinical diagnostics. On one hand, technological breakthroughs have brought new detection methods to the outputs of liquid biopsy to stratify AD cases, resulting in higher accuracy and efficiency of diagnosis. On the other hand, diversiform biofluid biomarkers derived from cerebrospinal fluid (CSF), blood, urine, Saliva, and exosome were screened out and biologically verified. As a result, more detailed knowledge about the molecular pathogenesis of AD was discovered and elucidated. However, to date, how to weigh the reports derived from liquid biopsy for preclinical AD diagnosis is an ongoing question. In this review, we briefly introduce liquid biopsy and the role it plays in research and clinical practice. Then, we summarize the established fluid-based assays of the current state for AD diagnostic such as ELISA, single-molecule array (Simoa), Immunoprecipitation–Mass Spectrometry (IP–MS), liquid chromatography–MS, immunomagnetic reduction (IMR), multimer detection system (MDS). In addition, we give an updated list of fluid biomarkers in the AD research field. Lastly, the current outstanding challenges and the feasibility to use a stand-alone biomarker in the joint diagnostic strategy are discussed.
Introduction
Alzheimer’s disease (AD) is a neurologic condition in which the brain shrinks and brain cells necrotize, which is characterized by a progressive loss of cognitive, behavioral, and social abilities (Soria Lopez et al., 2019). In the United States, AD is recognized as the most common cause of dementia, which affects about 5.1 million people aged 65 and up, impairing their ability to work and live independently (Lane et al., 2018). Memory loss, intellectual disability, various cognitive abnormalities, and disorientation are among the symptoms of AD, and risk factors include age, sex, down syndrome, familial inheritance, and poor sleeping habits (Silva et al., 2019). Furthermore, the death rate from AD has been growing substantially, with a 71% increase from 2000 to 2013 (Cass, 2017; Tiwari et al., 2019). In the regions of the brain affected by this disease, the accumulation of intracellular neurofibrillary tangles (NFT) and extracellular amyloid plaques was found. These plaques are mainly comprised of neurotoxic amyloid-beta protein 40 (Aβ40) and amyloid-beta protein 42 (Aβ42), which would have a devastating effect on neuronal cells (Hodson, 2018; Breijyeh and Karaman, 2020). Though AD remains a major public health problem, there are only two classes of pharmacologic therapy available for treating patients with AD, which include inhibitors to cholinesterase enzyme and antagonists to N-methyl-D-aspartate (NMDA). It was proved that these two classes can only be used to treat the symptoms of AD but not to cure it (Chu, 2012; Birks and Harvey, 2018; Atri, 2019). Due to the difficulty of treating AD, special attention should be paid to developing methods for accurate and timely diagnosis.
Normally, the diagnosis of AD is based on clinical evaluation, with several tests required for a conclusive diagnosis, including neuropsychological examination, magnetic resonance imaging (MRI) or computed tomography (CT) for neurons, and serum vitamin B12 level test (Hane et al., 2017). However, early diagnosis of AD is also complicated since early symptoms are shared by several diseases with similar neuropathological features (Weller and Budson, 2018). The identification of measurable, non-invasive, and reliable biomarkers for AD early diagnosis must help address this problem. Biological markers, or biomarkers, are widely used in human pathology for both diagnosis and disease monitoring. They are parameters determined by biological methods and primarily used to determine whether a certain disease exists or not, as well as the likelihood of contracting it (Wu and Qu, 2015). Actually, in the fields of lung cancer, colorectal cancer, and pancreatic, research has already been conducted to discover liquid biopsy biomarkers for cancer prognosis (Vaidyanathan et al., 2018). Unlike tumor tissue biopsy, liquid biopsy is a non-invasive detection method that can enable researchers to get information from body fluid samples by sampling and analyzing these samples and monitoring the patient condition in real-time (Poulet et al., 2019). In addition, tumor tissue biopsy is rather difficult to conduct in several cases. Because liquid biopsy is easier, quicker, and less painful than conventional biopsy, it is a promising alternative to existing surgical biopsies (Mader and Pantel, 2017).
Given the breakthrough nature of liquid biopsy in Oncology, there is a promising rationale for trans-fertilization and validation of blood-based liquid biopsy in AD (Hampel et al., 2019). Since there are no specific signs or symptoms associated with AD, the discovery of blood biomarkers for AD allows for a less invasive and more precise diagnosis, which may aid in identifying patients at risk before clinical symptoms and complications occur and defining disease stages (Lashley et al., 2018). The liquid biopsy would be especially useful in identifying people with preclinical AD, namely those with AD neuropathology but no clinical symptoms (Markesbery et al., 2006). In this review, we introduced liquid biopsy and the role it plays in disease diagnosis. We also reviewed diversified liquid biopsy tools which sensitively reflect a person’s health status and would be used for AD diagnosis. Finally, we discussed challenges and envisioned the future of liquid biopsy.
What is liquid biopsy?
Biopsy is a set of processes that include removing cells or tissues from the primary or metastatic mass and analyzing these samples to find out the causes of patients (De Rubis et al., 2019). In a traditional biopsy, the acquisition of specimens requires a biopsy needle or surgical procedure (see Figure 1). Given their invasive nature, tissue biopsy presents several limitations, which include patient risk, procedural costs, and invasive testing (Chen et al., 2020). In this regard, liquid biopsy can overcome these disadvantages and provide patients with a minimally invasive approach capable of cancer diagnosis (Smania, 2020).
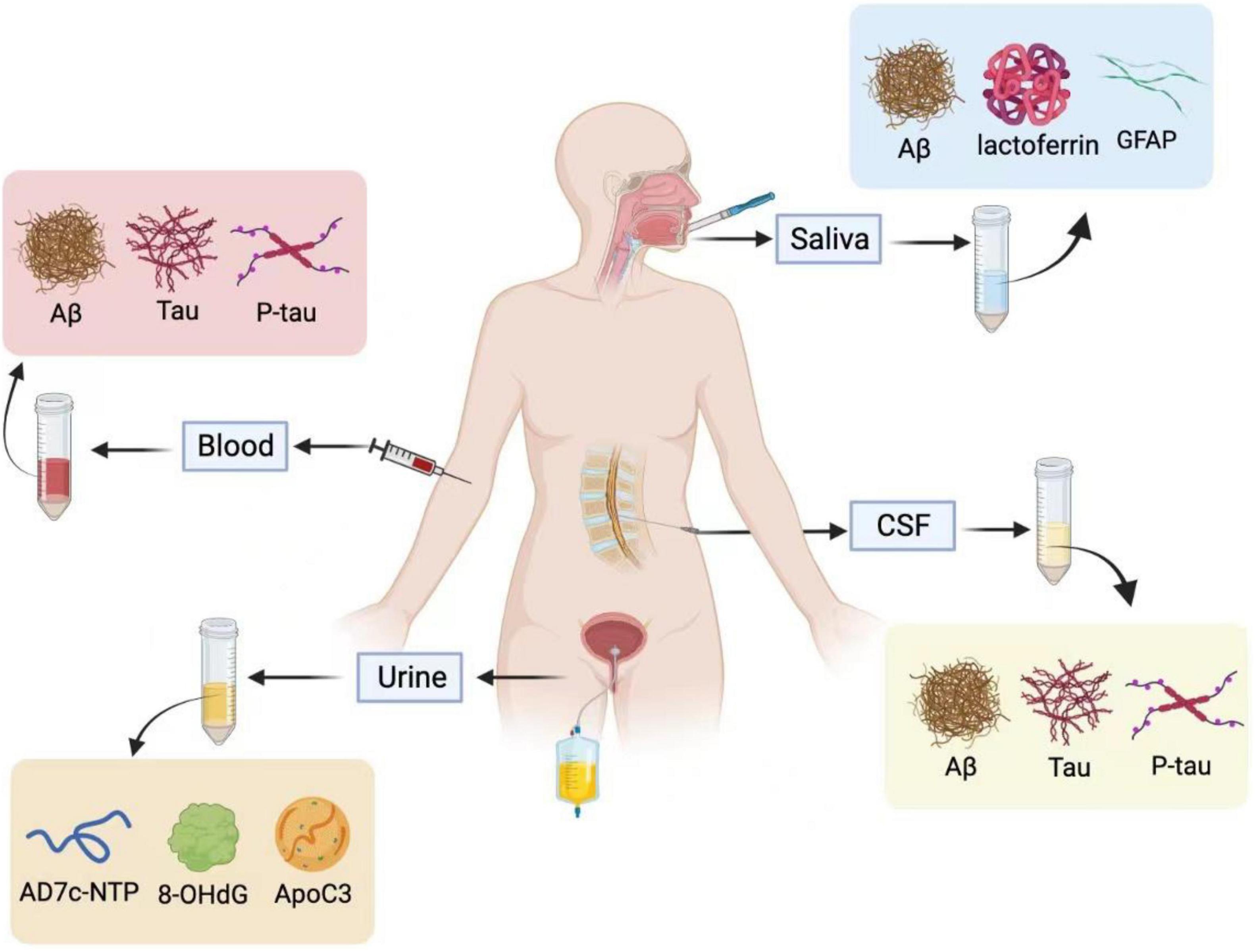
Figure 1. Saliva, blood, cerebrospinal fluid (CSF), and urine are used for Alzheimer’s fluid biopsy. In the CSF and blood, Aβ, T-tau, and P-tau are used as biochemical indicators for the early diagnosis of Alzheimer’s disease. The specific biomarkers in the urine are AD7c-NTP, 8-OHdG, and ApoC3, and salivary biomarkers are Aβ, Lactoferrin, and GFAP. Illustrations were generated using BioRender.
Liquid biopsy is defined by the National Cancer Institute (NCI) as “a test done on a sample of blood to look for cancer cells from a tumor that are circulating in the blood or for pieces of DNA from tumor cells that are in the blood” (Underwood et al., 2020). Though blood is most commonly used, the use of other kinds of body fluids such as CSF, urine, saliva, and stool are also involved in liquid biopsy (Blanco and Wolfgang, 2019). Compared with samples taken from the primary tumor, body fluid reflects a much broader range of tumor properties. The tumor circulome is defined as a portion of circulating components shed from tumor tissue that can be collected from body fluid (Mader and Pantel, 2017). Analysis of elements of tumor circulome including tumor circulome comprises of circulating tumor cells (CTCs), circulating tumor DNA (ctDNA), circulating tumor RNA (ctRNA), and extracellular vesicles (EVs) serves as a superb way for screening, diagnosis, and prognosis of many cancer types (Rohanizadegan and Kulkarni, 2018; Poulet et al., 2019). The number of free CTCs and EVs can both inform the presence of malignant disease and tumor burden (Kloten et al., 2019). Similarly, ctDNA can be used for the early detection of cancer. Besides, nearly all known classes of ctRNA have the potential to serve as biomarkers in cancer diagnosis (Pessoa et al., 2020).
The idea of liquid biopsy is not restricted to oncology but applies to AD diagnosis. Though a definitive method of AD diagnosis is brain tissue evaluation, it is impossible to take samples of living patients’ brain tissue (Weller and Budson, 2018). Biomarkers derived from CSF analysis have been used for in vivo AD detection (Hampel et al., 2021). Because it is generally believed that the onset of AD relates to the deposition of amyloid-β (Aβ) in extracellular plaques, aggregation of tau into neurofibrillary tangles in neurons, and abnormal phosphorylation of tau, the measurements of Aβ1–42, hyperphosphorylated tau, and total tau proteins are options for AD early diagnosis (Hersi et al., 2017; Park et al., 2020). However, this method is risky and inconvenient due to the invasive nature of its lumbar puncture procedure (Li et al., 2019). It often takes weeks to obtain final results due to the deficiency of laboratory facilities, which makes CSF analysis unsuitable for broad clinical implementation (Delgado-Peraza et al., 2021). Blood-based liquid biopsy is an ideal substitute for CSF-based liquid biopsy since it is non-invasive and cost-effective (Abdel-Haq, 2020).
Blood-based liquid biopsy for preclinical or clinical AD diagnosis is a multistep work-up (Baldacci et al., 2019). For example, EVs are regarded as attractive tools for non-invasive diagnosis since they are stable and contain diverse molecules from their parental cells. To use blood-derived EVs to determine if a person suffers from AD, blood samples should be obtained through venipuncture, and EVs should be isolated from blood (Mustapic et al., 2017). The major isolation strategies include ultracentrifugation, polymer precipitation, ultrafiltration, size-exclusion chromatography, affinity isolation, and microfluidics-based techniques. Finally, clinicians can gain a view of an individual’s health status by detecting the neurogranin level in blood-derived EVs, since it is downregulated in AD patients (Liu J. et al., 2021).
While liquid biopsy is a minimally invasive and cost-effective method that could be used to detect disease before patients have symptoms, many problems still need to be solved before liquid biopsy become a great alternative to tissue biopsy (De Rubis et al., 2019; Smania, 2020). First and foremost, the sensitivity and specificity of liquid biopsy assays need to be refined (Underwood et al., 2020). Meanwhile, protocols, pre-analytical, and analytical procedures need to be standardized (Blanco and Wolfgang, 2019). These procedures may include sample collection, liquid biopsy components isolation, analysis, data organization, and sharing (Poulet et al., 2019).
The cutting-edge liquid biopsy technologies for Alzheimer’s disease
Blood sampling may be preferable to CSF sampling due to its convenient, inexpensive, safe, and minimally invasive nature, but measuring brain disease biomarkers in the blood presents a variety of challenges. Since the blood-brain barrier prevents molecules from freely passing between the central nervous system (CNS) and blood, brain-derived biomarkers are usually found in low concentrations in blood (Lista et al., 2013). Also, biomarkers linked to AD pathology are rarely concentrated in non-cerebral tissues, making blood measurements challenging. Even though certain biomarkers linked to Alzheimer’s disease pathology are expressed in non-cerebral tissues, their measurement in the blood could be muddled (Blennow and Zetterberg, 2018a; Liu L. et al., 2021). To solve this problem, several ultra-high-sensitivity technologies capable of quantifying concentrations of biomarkers not only in CSF but also in blood have been developed. These innovative technologies can be applied to the early stage diagnosis of AD (Kulichikhin et al., 2021).
Stable isotope labeling kinetics
The Stable Isotope Labeling Kinetics (SILK) technique has been widely used to measure the protein turnover in human plasma and CSF such as three Aβ peptides (Aβ38, Aβ40, and Aβ42), tau and/or p-tau, superoxide dismutase 1 (SOD1) and three ApoE isoforms (ApeE2, ApoE3, ApoE4) (Crisp et al., 2015; Paterson et al., 2019; Dincer et al., 2020; Li et al., 2021; Elbert et al., 2022). In the process of using this technique, the different Aβ were immunoprecipitated simultaneously via monoclonal anti-Aβ mid-domain antibodies and later quantified by the liquid chromatography mass spectrometry (LC-MS/MS). In amyloid positive individuals, the Aβ42 concentrations and Aβ42/Aβ40 concentration ratios are significantly lower than those in amyloid negative individuals, which is consistent with that in CSF. Though the differences in plasma Aβ42/Aβ40 ratios between amyloid positive and negative individuals are of lesser magnitude compared to CSF, the LC-MS/MS ensures specificity and precision of results, which makes SILK a reliable tool for AD diagnosis (Potter et al., 2013; Baker-Nigh et al., 2016; Ovod et al., 2017). In the early stages, Basak et al. (2012) successfully measured the clearance rates of ApoE and Aβ in the mouse brain pulse-labeled by 13C6-leucine. Combining with the nanoscale secondary ion mass spectrometry (NanoSIMS) imaging technique, Wildburger et al. (2018) proposed a strategy termed SILK-SIMS to detect plaque dynamics in human AD brains. On the cellular level, the SILK technique can also be employed to measure tau kinetics with multiple isoforms and fragments in the induced pluripotent stem cell (iPSC)-derived neurons (Sato et al., 2018). The big limitation of this technique is the high labeling costs.
Fully automated assays
With the rapid development of technology, fully automated immunoassays such as Elecsys immunoassays (Roche Diagnostics) are now available to measure the CSF or plasma biomarkers with high precision and stability (Asberg et al., 2019; Blennow and Zetterberg, 2019). Using this newly developed Elecsys immunoassay (Palmqvist et al., 2019) examined the Aβ42, Aβ40, total levels of tau protein (T-tau), and phosphorylated tau (P-tau) in the CSF and plasma in two cohorts. It was found that plasma Aβ42 and Aβ40 detected by the fully automated Elecsys platform could be used to predict brain Aβ positivity. Furthermore, additional analysis of other blood biomarkers such as ApoE genotype, T-tau, and neurofilament light chain (NFL) can increase the accuracy of Aβ status prediction (Palmqvist et al., 2019). Meanwhile, the fully automated immunoassay system termed High Sensitivity Chemiluminescence Enzyme Immunoassay (HISCL™ series) based on the plasma biomarkers has been emerging in the diagnosis market (Goto et al., 2019; Miura et al., 2020; Watanabe et al.; Noda et al., 2021).1 Yamashita et al. (2021) declared that they had developed Aβ40 and Aβ42 immunoassays using the HISCL™ series, which has higher reproducibility, wider dynamic range, and less reaction time compared with the conventional enzyme-linked immunosorbent assays (ELISA). Hence, the fully automated plasma assays as a high-throughput and highly reliable screening tool might be used for clinical AD trials, which would help the patients get rid of unnecessary lumber punctures and save the expanse of examination.
Superconducting quantum interference device-based immunomagnetic reduction (SQUID IMR)
The immunomagnetic reduction (IMR) biomarker detection system was established in the early 2000s (Yang et al., 2017). Magnetic nanoparticles used in IMR are particles whose core comprises Fe3O4 and surface consists of bio-probes and hydrophilic surfactants. The phosphate-buffered saline (PBS) solution where these particles are suspended presents a magnetic property under external alternative-current (ac) magnetic fields, which is termed as xac. When magnetic nanoparticles and target molecules bond together, these particles will become larger and result in a reduction in xac. IMR (%) is the percentage of the reduction in magnetic signals during immune reaction divided by magnetic signals before immune reaction. The reduction in xac could be quite low, which requires magnetic sensors with high sensitivity (Lue et al., 2019). The superconducting-quantum interference-device (SQUID) magnetometer is capable of measuring IMR. The biomarker concentrations can be calculated from IMR via standard curves. Because SQUID IMR can be applied to quantify molecules of any size in human plasma, researchers have utilized this technology to predict the progression of dementia in high-risk groups. They found that the plasma Aβ42 level is an intriguing index for early detection of cognitive decline in patients with Down syndrome, stroke, or amnestic mild cognitive impairment (aMCI). In addition, the SQUID IMR technology is suitable for predicting Aβ status and brain atrophy (Yang et al., 2020).
Multimer detection system—Oligomerized amyloid beta (MDS-OAβ)
Meng et al. (2019) and Youn et al. (2019) presented the association between Aβ oligomerization in blood and AD neurodegeneration. An et al. (2017) found that Aβ oligomerization was significantly higher in AD patients than in normal people. Plus, the high correlations between Aβ oligomerization levels and other AD biomarkers, such as phosphorylated tau protein (pTau), amyloid positron emission tomography (PET) imaging, CSF biomarkers for Aβ42, total tau, and brain magnetic resonance imaging (MRI) were confirmed in many studies (Choi et al., 2021; Cheng et al., 2022).
Multimer detection system (MDS) is a traditional analytical platform based on modified ELISA to measure Aβ oligomerization tendency (An et al., 2010; Lim et al., 2015; Pyun et al., 2021). The epitope-overlapping detection antibodies applied in MDS are specifically designed for the N-terminus of Aβ for the selection of oligomerized formation over monomeric formation (Wang et al., 2017). Since the Aβ monomers have only one epitope available, once it has associated with a capturing antibody, it has no exposed epitopes to react with the antibodies (see Figure 2). Rather, the Aβ multimers with overlapping epitopes which bind the antibodies can be easily detected (An et al., 2010). Because of the ability to screen multimers, MDS has been utilized to differentiate the oligomeric Aβs (OAβ) from the monomeric Aβ in blood. Specific antibodies are used in MDS, and the level of OAβ can be obtained by using a spectrophotometer. As the OAβ test has the potential for distinguishing between AD patients and healthy controls, MDS would be a promising method for AD diagnosis, for example, AlphalLISA assay and the Blood™ OAβ test (PeopleBio Inc., South Korea) (Lee et al., 2020; Babapour Mofrad et al., 2021; Dominguez et al., 2022).
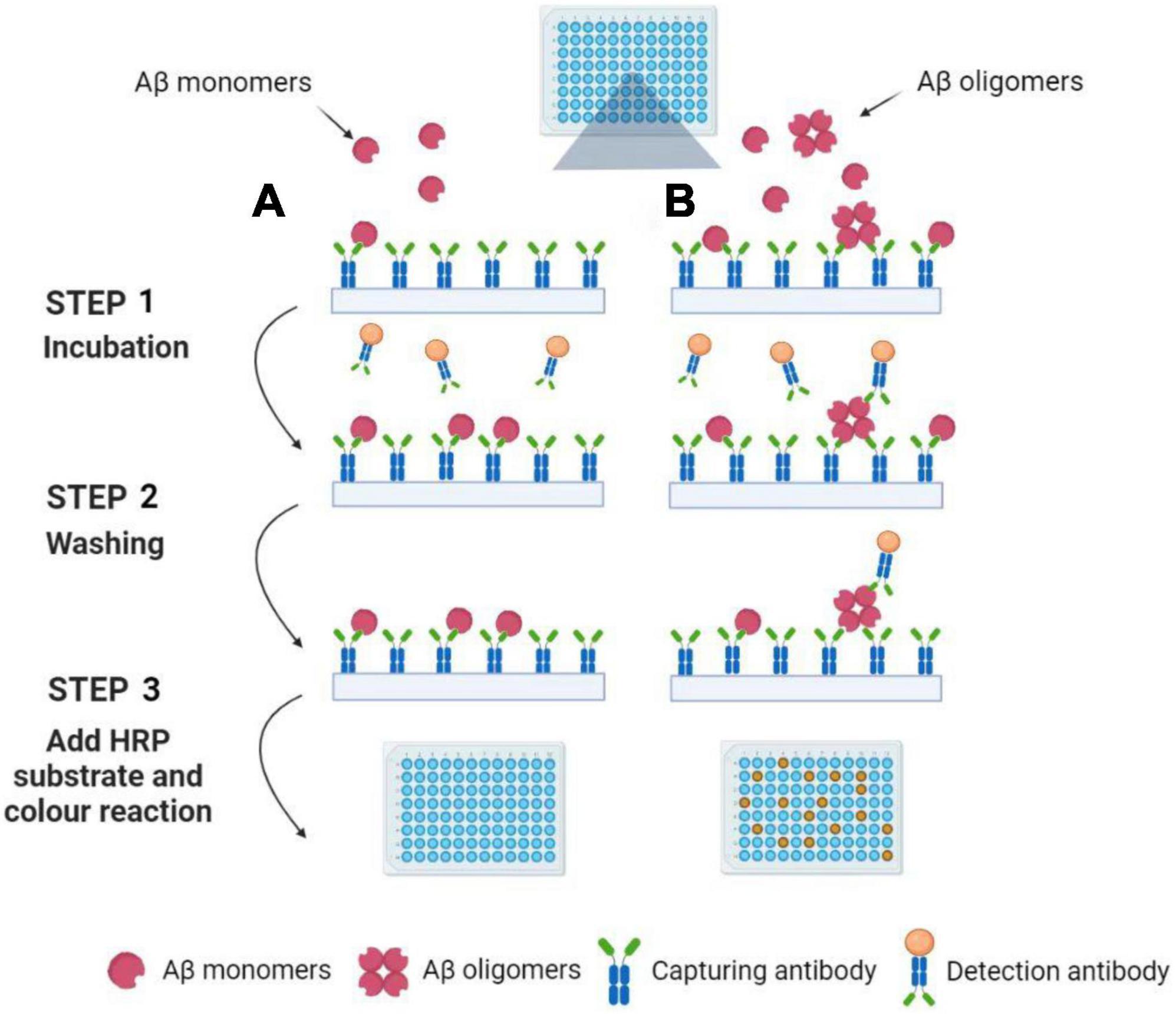
Figure 2. Detection of Aβ oligomers using the Multimer Detection System. (A) Aβ oligomers do not exist in blood plasma samples. Aβ monomers can be captured by an antibody attached to the ELISA plate’s surface. After incubation and washing, the detection antibodies are not present in the well because the capture antibodies have already occupied the single epitope. Finally, the color reaction can be not observed with the addition of HRP substrate. (B) Aβ oligomers exist in blood plasma samples. Aβ monomers and oligomers can be captured by an antibody attached to the ELISA plate’s surface. After incubation and washing, the detection antibodies are still present in the well because the Aβ oligomers have a lot of epitopes. Finally, the color reaction can be observed with the addition of HRP substrate. Illustrations were generated using BioRender.
Single molecule array
The single molecule array (Simoa), also known as the single molecule enzyme-linked immunosorbent assay, is a bead-based immunoassay with the ability to detect protein biomarkers in blood at subfemtomolar concentrations (Cohen and Walt, 2017). The specific steps of this approach are as follows: Firstly, paramagnetic beads coated with capture antibodies are added to a sample. The amount of these capture beads is far beyond the number of target analytes contained in a sample so that each capture bead binds to zero or one target analyte. Next, each capture bead is incubated with a biotinylated detection antibody and an enzyme, streptavidin-β-galactosidase (SβG), to form an enzyme-labeled immunocomplex. Thirdly, the beads are suspended in a fluorogenic substrate solution and then loaded into an array (see Figure 3). Finally, fluorescence images of the assay are utilized to determine the concentration of a protein biomarker (Cohen et al., 2018, 2020).
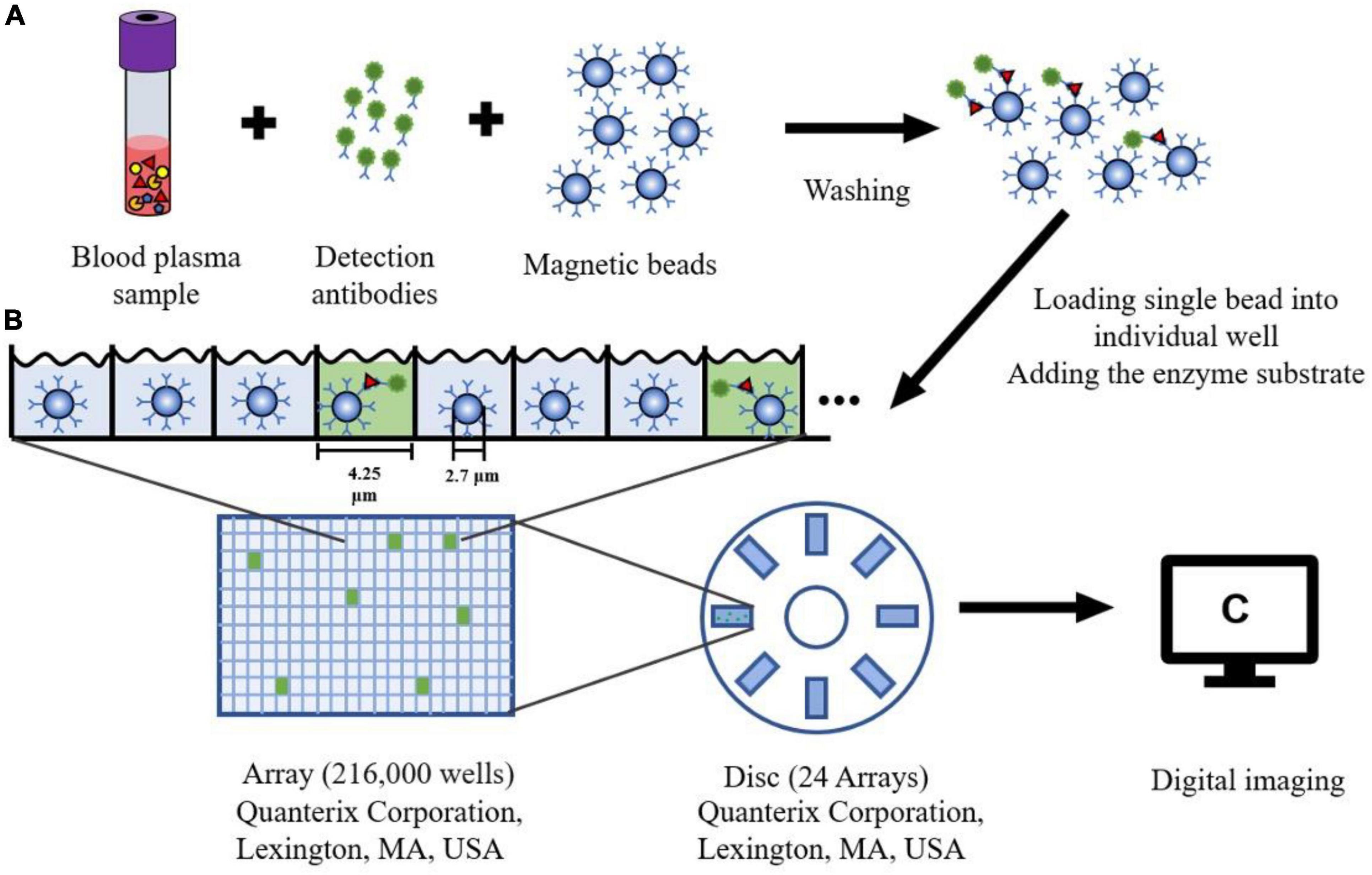
Figure 3. The schematic diagram of Single Molecule Array. (A) Blood plasma sample is mixed with the magnetic microbeads (2.7 μm) with capture antibodies and detection antibodies. After washing the beads three times, the protein can be captured specifically bound by the antibody. (B) Each Single magnetic bead is loaded into one well (4.25 μm diameter, 3.25 μm depth) of the array (216,000 wells). The substrate for the enzyme is added to the well sealing with oil. (C) After fluorescence detection of each well, a single molecule signal image can be obtained on this array.
Many studies verify that the Simoa assays examining different P-tau isoforms (P-tau181, P-tau217, P-tau231) have high accuracy and specificity to detect early Alzheimer’s pathology (Barthelemy et al., 2020b; Karikari et al., 2020, 2021a, 2021b; Palmqvist et al., 2020; Suarez-Calvet et al., 2020; Thijssen et al., 2020; Janelidze et al., 2021; Keshavan et al., 2021; Rauchmann et al., 2021; Ashton et al., 2022). Barthelemy et al. (2020a) and Janelidze et al. (2020b) declared that P-tau217 has higher accuracy than P-tau181 in CSF. Also, Palmqvist et al. (2020) believed that P-tau217 was a better biomarker than P-tau181 in a comparative plasma study, while it was not reproductive with an updated assay (Brickman et al., 2021). In an overall study, Bayoumy et al. (2021) constructed another comparative plasma study to test three P-tau181 assays (Eli Lilly, ADx, and Quanterix), a P-tau217 assay (Eli Lilly), and two P-tau231 assays (ADx, Gothenburg). All of the six Simoa assays showed robust clinical performance and high-sensitive. Notably, Lantero-Rodriguez et al. (2021) developed a new immunoassay targeting P-tau235 for staging preclinical AD. Currently, the Simoa NF-light Advantage Kit comes on the market, which quantifies the Neurofilament Light (Nf-L) in serum and plasma with high sensitivity (Hendricks et al., 2019; Ashton et al., 2020; Benussi et al., 2020; Manouchehrinia et al., 2020). The blood Nf-L level is becoming a supplemental biomarker of AD, there is little doubt that Simoa is a practical tool for AD diagnosis (Illan-Gala et al., 2021; Leuzy et al., 2021; Zetterberg and Blennow, 2021).
Immunoprecipitation–mass spectrometry
Immunoprecipitation combined with matrix-assisted-laser desorption/ionization time of flight mass spectrometry, or called immunoprecipitation–mass spectrometry (IP–MS) for short, to examine the truncated Aβ peptides is an approach for characterizing protein and peptide mixtures in body fluid (Portelius et al., 2006; Guard et al., 2019). During the IP, target proteins or peptides at low concentrations are enriched using antibodies (see Figure 4). Then MS allows an accurate analysis of levels of these proteins or peptides (Uljon et al., 2000; Campbell et al., 2021). Richard et al. (2019) proposed a simplified ad detailed protocol for robust immunoprecipitation of Aβ before mass spectrometric detection. They clarified that the employment of murine monoclonal and rabbit polyclonal antibodies can yield reproducible and high-resolution pictures with low background noise (Richard et al., 2019). The IP–MS method is commonly used to analyze the Aβ38, Aβ40, and Aβ42 levels in plasma, and companies with SILK method in CSF (Ovod et al., 2017). To reduce the amount of lab workload and accelerate the SILK studies, Mawuenyega et al. (2013) developed an efficient and specific quantitative isoform characterization and kinetics method. Plenty of studies adopting the IP-MS method elucidated that plasma Aβ42 concentration and Aβ42/Aβ40 rate are promising biomarkers for predicting Aβ status in individuals (Portelius et al., 2006, 2015; Ovod et al., 2017; Cheng et al., 2022). Furthermore, immunoprecipitation together with SampleStream mass spectrometry (SampleStream-MS) was established. The streamlined workflow could be used to profile protein products of genes efficiently (Santos Seckler et al., 2021).
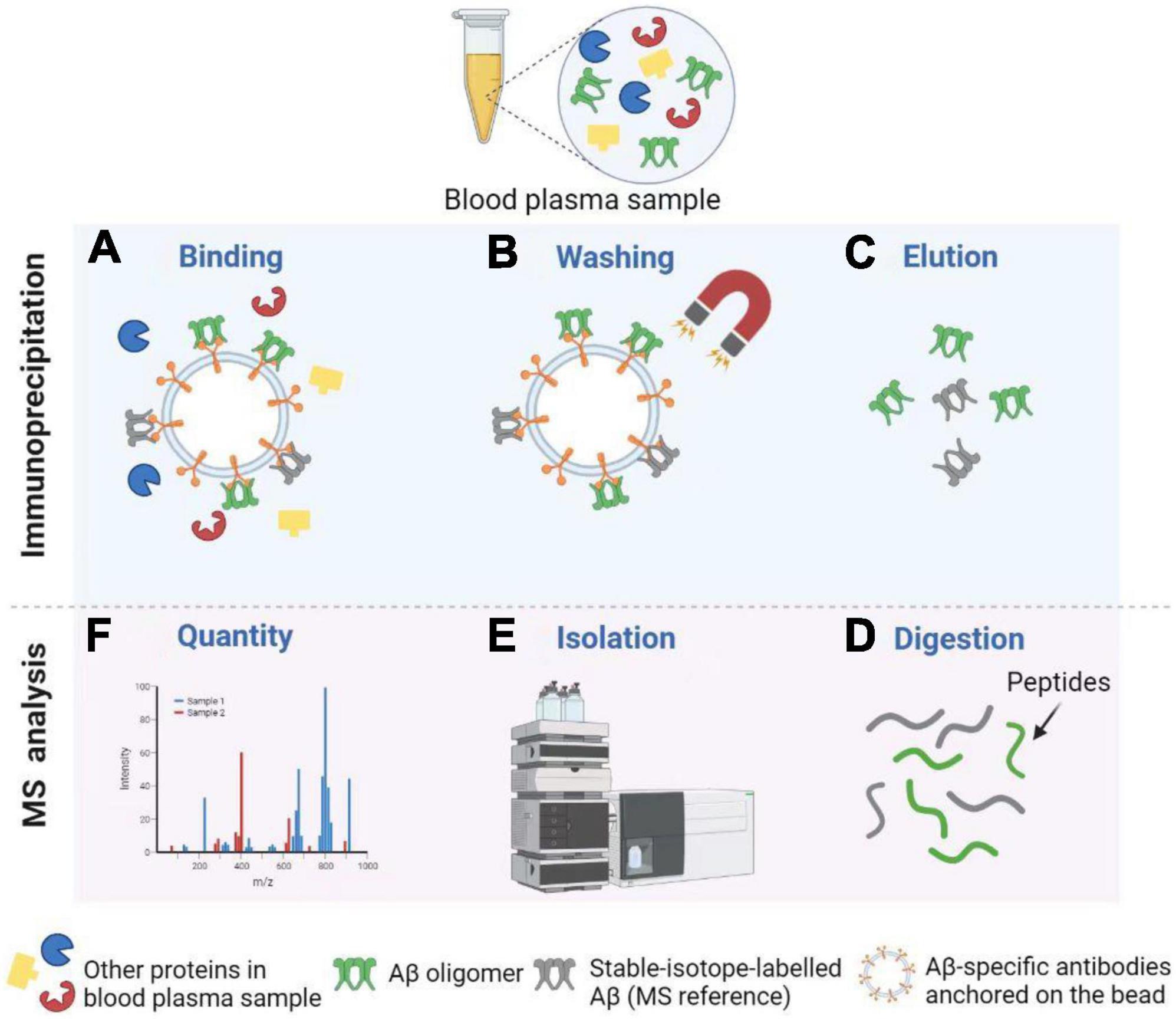
Figure 4. Detection of Aβ oligomers using Immunoprecipitation and Mass Spectrometry (IP-MS). (A) Aβ oligomers and stable-isotope-labeled Aβ (MS reference) can be captured by Aβ-specific antibodies anchored on the bead. (B) After washing, other proteins in the blood plasma sample are removed from the solution. (C) Next, Aβ oligomers and MS reference can be separated from the bead for further MS analysis. (D,E) After enzymatic digestion, peptides are isolated by High Performance Liquid Chromatography (HPLC). (F) Finally, MS profiles can be obtained to carry out the Aβ oligomer quantity. Illustrations were generated using BioRender.
In sum, a huge development has taken place in the liquid biopsy platform in the past two decades. Researchers used to apply the traditional ELISA to detect and measure core CSF biomarkers, including Aβ42, T-tau, and P-tau (Yoo et al., 2018). While CSF possesses the advantage of being continuous with the brain extracellular fluid, there is little doubt that blood is a more ideal subject for liquid biopsy. However, studying biomarkers in the blood is more challenging than in CSF, for blood only contains a tiny amount of brain protein (Blennow and Zetterberg, 2018b). The advancements in the field of ultra-high-sensitivity technologies provided a solution to this problem. Although these screening tools can accurately quantify biomolecules at ultralow levels, some shortcomings limit their utility in AD biomarker studies (Hampel et al., 2021). For example, due to the spectral overlap of fluorescent dyes, they cannot be used for the simultaneous detection of multiple analytes. In addition, interactions between non-target molecules during the assay can generate erroneous signals, thereby having a negative effect on their sensitivity and specificity (Cohen and Walt, 2017).
Histologic origin used in liquid biopsy and biological markers involved
A biological marker, or biomarker (Cedazo-Minguez and Winblad, 2010), is an indication of normal biological processes, pathogenic processes, or pharmacological responses to a therapeutic intervention that is objectively assessed and evaluated. The most important factors that determine a biomarker’s diagnostic utility are its sensitivity, specificity, and ease of use. Since the cognitive symptoms of AD are often diffuse and overlap with other disorders, and the clinical progression is intermittent and variable even among patients with the same disease, biomarkers for AD are extremely important. Biomarkers representing various forms of pathophysiology in the brain can be used for clinical diagnosis, particularly in the early stages of the disorder, to forecast progression, to observe the effects of novel drug candidates in clinical trials, and finally, to further our understanding of the disease’s pathogenesis in clinical science (Table 1, Blennow et al., 2010). Aiming to gain an insight into biomarkers for the early diagnosis of AD, molecules in CSF and blood have been intensively studied.
Cerebrospinal fluid
CSF can be obtained by lumbar puncture, a diagnostic technique in which a needle is inserted into the subarachnoid space in the lumbar area (L3/L4 or L4/L5), securely below the spinal cord’s end.
Since CSF is in indirect contact with the brain’s extracellular space, biochemical changes in the brain are expressed in the CSF. In neurodegenerative diseases, neurovascular and blood-brain barrier dysfunction may occur. As a result, CSF is the best source of AD biomarkers (Molinuevo et al., 2014; Ferreri et al., 2021). T-tau, P-tau, and the 42-amino acid long amyloid beta peptide (Aβ42) are the most common AD CSF biomarkers.
In cognitively normal people, Aβ is formed in the brain and diffuses into the CSF, where it appears in moderate concentrations with multiple forms. Among them, the levels of Aβ42 and Aβ40 are relatively high. The former is a major component of amyloid plaques, which is regarded as an abnormal pathological lesion (Tiwari et al., 2019). The first step of amyloid pathogenesis is the generation of insoluble Aβ fibrils from amyloid precursor protein (APP) via cleavage. After oligomerization and subsequent polymerization, they transform into amyloid plaques (O’Brien and Wong, 2011). The toxicity of these plaques is associated with some mechanisms, including microglia infiltration, oxidative stress, and synaptic damage (Reiss et al., 2018).
The earliest studies of Aβ being secreted into the CSF date back to 1992, which encouraged researchers to develop corresponding immunoassays (Nitsch et al., 1992). Subsequently, decreased Aβ42 levels in the CSF of AD patients were revealed and validated, yet the cause was unknown for a long time (Gouras et al., 2015). In 2003, an autopsy study discovered a connection between low postmortem ventricular CSF Aβ42 levels and high plaque counts (Strozyk et al., 2003), a finding that was later confirmed in a study revealing a link between reduced CSF Aβ42 measured in ante mortem lumbar CSF samples and amyloid plaque counts measured autopsy (Tapiola et al., 2009). CSF Aβ42 levels can increase and then begin to decline 25 years before the onset of AD symptoms, according to a FAD cohort study from the Dominantly Inherited Alzheimer Network, while amyloid deposition measured with PET and Pittsburgh compound B, as well as an increased concentration of T-tau in the CSF, can be detected 15 years before expected symptom onset (Bateman et al., 2012). These results suggest that a decrease in CSF Aβ42 may be the first sign of preclinical Alzheimer’s disease. Low CSF Aβ42 levels have been linked to cognitive impairment in older women (Gustafson et al., 2007). CSF Aβ42 can also be used to predict cognitive impairment in healthy elderly people (Stomrud et al., 2007). In most cases, CSF Aβ42 and amyloid PET status have a high degree of concordance (Blennow et al., 2015). With sensitivity and precision of over 80%, a significant decrease in CSF Aβ42 and a significant increase in CSF t-tau and p-tau can be used to distinguish symptomatic AD patients (Blennow and Hampel, 2003). Hence, in 2012, the utility of CSF Aβ42 in predicting preclinical Alzheimer’s disease was verified in cognitively normal individuals with inherited AD genes.
In the brain (Suzuki et al., 1994), CSF (Portelius et al., 2006), and plasma (Zhang et al., 2018), Aβ40 is the most common source of Aβ peptide, although it does not appear to be as pathogenic as Aβ42 (McGowan et al., 2005). The CSF Aβ40 level in Alzheimer’s patients is higher than in patients with Parkinson’s disease dementia (PDD) or with dementia with Lewy bodies (DLB), implying that the Aβ42/Aβ40 ratio will help distinguish AD from PDD and DLB (Nutu et al., 2013). Also, the Aβ42/Aβ40 ratio could be used to differentiate AD from non-AD dementias better than CSF Aβ42 alone (Janelidze et al., 2016). The ratio of CSF Aβ42/Aβ40 is a better indicator of Aβ-positive PET than CSF Aβ42 alone (Pannee et al., 2016; Lewczuk et al., 2017), and is comparable to the ratios of t-tau/Aβ42 and p-tau/Aβ42 (Racine et al., 2016). Currently, fluid-based assays of Aβ40 and Aβ42 are commercially available.
Tau proteins are found in neuronal axons and play roles in preserving the cohesion of microtubules in neurons of the CNS. Tau proteins in the human brain are composed of six soluble isoforms and various phosphorylation sites (Khan and Bloom, 2016). Western blotting was used to classify tau proteins of Alzheimer’s disease in CSF in 1987 (Wolozin and Davies, 1987). The development of quantitative immunoassays made the measurement of tau proteins in CSF easier. ELISA method was used to quantify tau in CSF, this method was based on the sandwich format of a monoclonal anti-tau antibody against the mid-domain combined with a polyclonal anti-tau antiserum (Vandermeeren et al., 1993). Apart from acute brain trauma, T-tau CSF levels are emphatic, rising within days of injury and then remaining rapt for weeks before homogenization (Zetterberg et al., 2006). CSF T-tau levels are highest in diseases with the most severe neurodegeneration, such as Creutzfeldt–Jakob disease, Alzheimer’s disease, DLB, and frontotemporal dementia (FTD). Since it predicts more rapid clinical disease progression, CSF T-tau has been used as a biomarker for the severity of neurodegeneration (Buchhave et al., 2012; Hansson et al., 2018).
Tau is a phosphoprotein, indicating that its biological activity is completely regulated by its phosphorylation state (Gao et al., 2018). Highly phosphorylated tau proteins tend to form tangles rather than bind to microtubules, which may affect inter-synaptic signaling in brain neurons (Naseri et al., 2019). Since they were found in large numbers in neurofibrillary tangles in the brains of AD patients, they have been believed to be a reliable biomarker for AD (Lindwall and Cole, 1984; Bramblett et al., 1993). For different phosphorylated epitopes of tau proteins, ELISA methods have been established for threonine 181 and 231 (Blennow et al., 1995), threonine 181 (Vanmechelen et al., 2000), threonine 231 and serine 235 (Ishiguro et al., 1999), serine 199 [54], threonine 231 (Kohnken et al., 2000), and serine 396 and 404 (Hu et al., 2002). The phosphorylation state of tau in the brain is reflected in the concentration of phosphorylated tau protein in the CSF. The concentration of phosphorylated tau protein does not alter after an acute stroke, unlike the overall concentration of tau protein (Hesse et al., 2001). Sandwich ELISA was used to detect P-tau 181 in CSF in 2000, and it was discovered that levels were higher in AD patients relative to age-matched controls, but lower in FTD patients, indicating that CSF P-tau 181 may be a more specific marker for AD (Vanmechelen et al., 2000). Another sandwich ELISA for detecting P-tau threonine 231 was produced the same year, with 85 percent sensitivity and 97 percent specificity for distinguishing AD from non-AD controls (Kohnken et al., 2000).
Aβ42, T-tau, and P-tau levels are all considered practical biomarkers for AD (Lee et al., 2019). Moreover, the combined use of the three biomarkers in the diagnosis of AD has provided excellent results. For example, mild cognitive impairment (MCI) is the earliest clinical stage of AD, which is characterized by episodic memory problems. During clinical follow-up, MCI patients who progress to AD with dementia have the standard AD CSF biomarker profile (decreased CSF Aβ42 with increased T-tau and P-tau) already at this stage of the disease (Andreasen et al., 1999).
Blood (plasma/serum)
Blood sampling would be preferable to CSF sampling when taking fluid samples to measure AD biomarkers, both for clinical diagnosis or screening and for repeated sampling in clinical trials, since blood is more accessible than CSF. However, blood-based biomarkers, on the other hand, encounter some challenges. Firstly, blood is a complicated fluid containing many cellular compartments as well as a constantly evolving environment of proteins, lipids, and other biochemical entities, which may intensify issues of reproducibility and necessitate concerted efforts to match methodology throughout studies (Thambisetty and Lovestone, 2010). Secondly, since the blood-brain barrier prevents molecules from freely passing between the CNS and the blood compartments, brain-derived biomarkers are found in low concentrations in the blood. Furthermore, certain biomarkers linked to Alzheimer’s disease pathology are expressed in non-cerebral tissues, which may make blood measurements difficult. Consequently, the quantities of brain proteins entering the blood must be calculated in a matrix containing extremely high levels of plasma proteins, posing a significant risk of analytical method intervention (Blennow and Zetterberg, 2015). Thirdly, brain proteins released into the bloodstream can be degraded by proteases, metabolized in the liver, or cleared by the kidneys, resulting in a variation that is unrelated to brain changes and difficult to control. Finally, heterophilic antibodies (endogenous antibodies which respond with the antibodies of the immunochemical test to measure the biomarker) can be present in the blood, resulting in falsely high or low performance. In CSF, where antibody levels are much lower, these types of antibodies are much less of a concern. Despite all the aforementioned issues that limit the possibility of discovering blood biomarkers for AD (O’Bryant et al., 2015), several biomarkers for AD diagnosis have been discovered.
The most widely studied blood markers for the diagnosis of symptomatic and prodromal AD are Aβ42 and Aβ40. Plasma Aβ42 levels are higher than plasma Aβ40 levels in AD patients at baseline and in those who develop AD within 3 years in a follow-up analysis (Mayeux et al., 2003). Individuals with elevated plasma Aβ42 had a more than twofold increased chance of AD onset relative to those with low plasma Aβ42 (Mayeux et al., 2003). A high plasma Aβ40 concentration has also been linked to an increased risk of dementia (van Oijen et al., 2006). Discovered that a lower plasma Aβ42/Aβ40 ratio is linked to greater cognitive impairment in elderly people without dementia over 9 years. The clinical stage of the examination and/or the combination of other forms of dementia can cause this dissimilitude. When compared to age-matched standard controls, Aβ42 levels are lower and Aβ43 levels are higher in the serum of AD patients, indicating that a lower Aβ42/Aβ43 ratio may be used as a blood marker for AD diagnosis (Zou et al., 2013).
Plasma tau concentrations, as determined by ultrasensitive assays, are higher in dementia patients than in cognitively normal controls, but not as significantly as in CSF (Pereira et al., 2021). Plasma tau has become a candidate blood marker for AD diagnosis due to the invasiveness and high costs of testing CSF tau, and several studies have concentrated on the quantitation of tau in AD, MCI, and normal classes. Since tau levels in plasma are much lower than in CSF (Karikari et al., 2020), ultrasensitive assays were created, which can be used to discover higher levels of T-tau in plasma from AD patients compared to control or MCI patients. However, there is no difference between MCI patients who developed AD and stable MCI patients.
Tau phosphorylated at threonine 181 (P-tau181) in blood has been widely recognized as a simple, accessible, and highly specific biomarker for AD diagnosis (Moscoso et al., 2021). Normally, plasma P-tau181 is higher in Alzheimer’s patients compared to people with normal cognitive and will increase as the disease progresses. Therefore, it may be used to estimate the likelihood of people AD in the future, regardless of disease stage (Brickman et al., 2021). Since the increase in plasma P-tau181 is related to Aβ and tau pathologies, it could also be utilized as a screening tool to study the pathophysiology of AD in vivo (Janelidze et al., 2020a). Of all the promising clinical applications for plasma P-tau181, the most important one might be to differentiate AD from other neurodegenerative disorders (Simrén et al., 2021). Another likely application for plasma P-tau181 is to determine whether patients need more detailed tau diagnostics testing with PET scans (Thebault et al., 2020).
Neurofilaments (Nfs) are the structural proteins of the neural cytoskeleton (Thebault et al., 2020). The constitutions of Nfs can be divided into three categories according to their molecular weight, which are Nf light (NfL), Nf medium (NfM), and Nf heavy (NfH) chains (Hendricks et al., 2019). Among the three subunits of Nfs, NfL is the most promising biomarker for the diagnosis of neurodegenerative diseases, which plays important role in neuron growth (Bacioglu et al., 2016). Because of the fairly low concentration of NfL in blood, it was not until the development of highly sensitive assays such as Simoa that researchers were able to perform NfL evaluations in blood (Mattsson et al., 2019). Dynamic changes in plasma NfL concentrations are correlated with neurodegeneration in AD, as Alzheimer’s patients had higher plasma NfL levels compared to healthy individuals (Mattsson et al., 2017; Preische et al., 2019). While plasma NfL cannot be used to distinguish AD from other dementias, it may serve as a practical tool for neurodegeneration detection. In addition, it could be used in combination with other available AD biomarkers for AD diagnosis (Blennow and Zetterberg, 2018b).
Urine and saliva
Urine is a desired substitute for blood since it can be collected in large quantities easily and non-invasively (Hartmann and Kist, 2018). Although the composition of organic compounds in urine is less complex than that of CSF and blood, changes in metabolites and hormones among these compounds can reflect the metabolic and pathophysiological state of a person (Sancesario and Bernardini, 2019). At the same time, the relatively low compositional complexity means that fewer proteins capable of interfering with measurements are present in the urine (Bǎlaşa et al., 2020; Buccellato et al., 2021). Some AD biomarkers have already been identified in urine.
Alzheimer-associated neuronal thread protein (AD7c-NTP) has attracted a great deal of attention from researchers because of the correlation between its concentration changes in urine and the severity of AD (Wang et al., 2019; Ausó et al., 2020). Elevated urinary AD7c-NTP levels can be used in the early diagnosis of AD with high accuracy (Li Y. et al., 2020; Seol et al., 2020). Monocyte chemoattractant proteinx (MCP-1) also showed promise as a biomarker for AD. Its concentration in the urine of AD patients was discovered to be significantly higher than that of cognitively normal individuals (Xu et al., 2020). In addition, Watanabe et al. (2020) found that the AD group had significantly higher levels of apolipoprotein C3 (ApoC3) in urine than the control group did. Therefore, it could be utilized to differentiate patients with AD. Recently, Yao et al. (2018) applied a combination of computational and experimental methods to identify three differentially expressed proteins. To be specific, in the urine of AD patients, secreted phosphoprotein 1 (SPP1) was downregulated, whereas gelsolin (GSN) and insulin-like growth factor-binding protein-7 (IGFBP7) were upregulated, which made them promising biomarkers for AD.
In addition to the aforementioned proteins, several indicators of other disorders in urine can be considered candidates to be biomarkers for AD early detection. For example, the levels of formaldehyde and 8-hydroxy-2′-deoxyguanosine (8-OHdG) can be used to access the status of formaldehyde metabolism and oxidative DNA damage, respectively. They were significantly higher in the urine of AD patients compared to cognitively normal controls (Pena-Bautista et al., 2019; Wang et al., 2022). AD would interact with osteoporosis during its progression. Pu et al. (2020) found that urinary biomarkers reflecting osteoporosis and bone loss, such as deoxypyridinoline (DPD), creatinine (Cr), and calcium (Ca), can also be used in the early diagnosis of AD in male populations.
Similar to urine sampling, saliva sampling is non-invasive, stress-free, and easy to obtain (Lee et al., 2019; Veerabhadrappa et al., 2020; Francois et al., 2021). The origin of AD-related molecules in the saliva is unknown. It is speculated that they are secreted by the salivary glands or transported from the blood (Schepici et al., 2020). In addition, the flow and composition of saliva are directly regulated by the glossopharyngeal nerve and the facial nerve (Farah et al., 2018; Pawlik and Blochowiak, 2021). Given their proximity and relation, biomarkers may be secreted from the nerves into the salivary glands (Gleerup et al., 2019; Paraskevaidi et al., 2020). These characteristics may render saliva a promising matrix for biomarker discovery. Although saliva has quite a few unique advantages, the relatively low concentration of analytes in saliva makes detection more difficult (Reale et al., 2020). In recent years, developments in detection methods have provided a tempting opportunity to use saliva to diagnose diseases (Ashton et al., 2019). A growing number of researchers have been working to explore the association between salivary biomarkers and AD.
In exploring urinary biomarkers for AD diagnosis, many researchers have focused on Aβ42 quantification. A collection of studies has illustrated the potential use of salivary Aβ42 as a diagnostic tool for AD. Bermejo-Pareja et al. (2010); Kim et al. (2014), and Sabbagh et al. (2018) found that the salivary Aβ42 was significantly higher in AD patients than in healthy participants, although controversy exists regarding changes in Aβ42 secretion with the development of AD. In addition, according to the study by McGeer et al. (2018) salivary Aβ42 levels can be used to predict the likelihood of future AD onset. Furthermore, Lee et al. (2017) revealed that salivary Aβ42 concentrations in patients with Parkinson’s disease were identical to those in healthy subjects, indicating that Aβ42 was specific for AD. However, Shi et al. (2011) and Lau et al. (2015) found that Aβ42 in saliva was undetectable, whereas the finding of Tvarijonaviciute et al. (2020) showed that salivary Aβ42 levels were significantly lower in AD patients than in controls. The inconsistency between these experimental results may be due to differences in saliva collection, storage, and testing methods, as well as differences in the subjects screened.
Lactoferrin is an iron-binding glycoprotein that is abundantly expressed in saliva. It has been considered a potential biomarker for AD because of two properties, namely anti-microbial and Aβ-binding (Zhang et al., 2021). Carro et al. (2017) measured salivary lactoferrin levels in some healthy individuals, patients with AD, patients with MCI, and patients with PD. The results showed that salivary lactoferrin concentrations were highest in PD patients, while patients with AD or MCI had lower salivary lactoferrin concentrations than the controls did. Low lactoferrin concentration in saliva was hypothesized to be associated with the risk of developing AD or MCI. In addition, Gonzalez-Sanchez et al. (2020) found that participants with FTD did not experience a significant decrease in salivary lactoferrin concentrations. These two studies demonstrate the value of this biomarker in diagnosing the early clinical stages of AD.
Glial fibrillar acidic protein (GFAP) is a component of the cytoskeleton of astrocytes, which plays an important role in modulating neuronal inflammation (Yang and Wang, 2015). According to the study of Katsipis et al. (2021) two different immunological assays, namely ELISA and Dot Blot, were applied to detect GFAP levels in the saliva of participants in the experiment. The results obtained by both methods showed a decrease in GFAP levels in patients with AD or MCI compared to healthy subjects. In addition, GFAP levels in the saliva of AD patients are significantly lower than those of MCI patients. The possible reason for this was that GFAP were susceptible to several post-transcriptional modifications, which would hinder their transfer from the brain to the saliva and reduce their solubility (Katsipis et al., 2021). Since salivary GFAP can be used to differentiate between healthy individuals, people suffering from MCI, and AD patients, it may present as a reliable biomarker for the screen of the neurological status of people.
Although researchers have uncovered these urinary and salivary biomarkers that are of value for the early diagnosis of AD, there is still much work to be done in the future. Firstly, standards for sampling, processing, storage, and analysis methods need to be developed (Bouftas, 2021; Goldoni et al., 2022). Secondly, before these novel biomarkers can be in the clinic, large-scale as well as longitudinal research is needed to further determine their reliability in detecting AD pathology (Liang and Lu, 2019). Finally, to test their specificity for AD, it is imperative to study the levels of the biomarkers in other neurodegenerative conditions (Ashton et al., 2021).
Exosomes (extracellular vesicles)
The application of exosomes derived from human cells to examine an individual’s health status is an emerging molecular diagnostic (Figure 5, Trams et al., 1981; Johnstone et al., 1987; Sokolova et al., 2011; van der Pol et al., 2014; Greening et al., 2015; Pegtel and Gould, 2019). The focus of the published papers is related to cancer to parse the roles of exosomes in pathophysiology, autophagy, diagnosis, and therapeutic applications (Colletti et al., 2020; Jafari et al., 2021; Kugeratski and Kalluri, 2021; Lakshmi et al., 2021; Liu T. et al., 2021; Sandua et al., 2021; Xu et al., 2021; Zhou et al., 2021). As the exosomes are also synthesized and released from brain cells to blood or CSF through the BBB, alterations in the contents of exosomes may serve as biomarkers to detect the pathological progress of the CNS (Kanninen et al., 2016; Jiang et al., 2019; Vandendriessche et al., 2020; Pinnell et al., 2021). Structurally, exosomes are rich in certain tetraspanin proteins such as CD81, CD82, CD37, and CD63, which mediate the other protein inclusion, including immunoglobulin superfamily member 8 (IGSF8) major histocompatibility complex (MHC) class II proteins, syndecans (SDC1–4) and integrins (Raposo et al., 1996; Escola et al., 1998; Hemler, 2003; Segura et al., 2005; Liang et al., 2014; Kalluri and LeBleu, 2020). Notably, a growing number of studies certify that some inclusions in exosomes are RNAs in functional form with the ability to influence other cells and tissues (Ratajczak et al., 2006; Valadi et al., 2007; Skog et al., 2008; Pegtel et al., 2010). In addition, the exosomes cover a variety of DNAs which can be used to infer tissue-of-origin and molecular classification (Balaj et al., 2011; Kahlert et al., 2014; Thakur et al., 2014; Sansone et al., 2017). Taken together, exosomes have the potential to expand the central paradigm of liquid biopsy for preclinical AD diagnosis and the biomarker library (Yin et al., 2020; Guo et al., 2021).
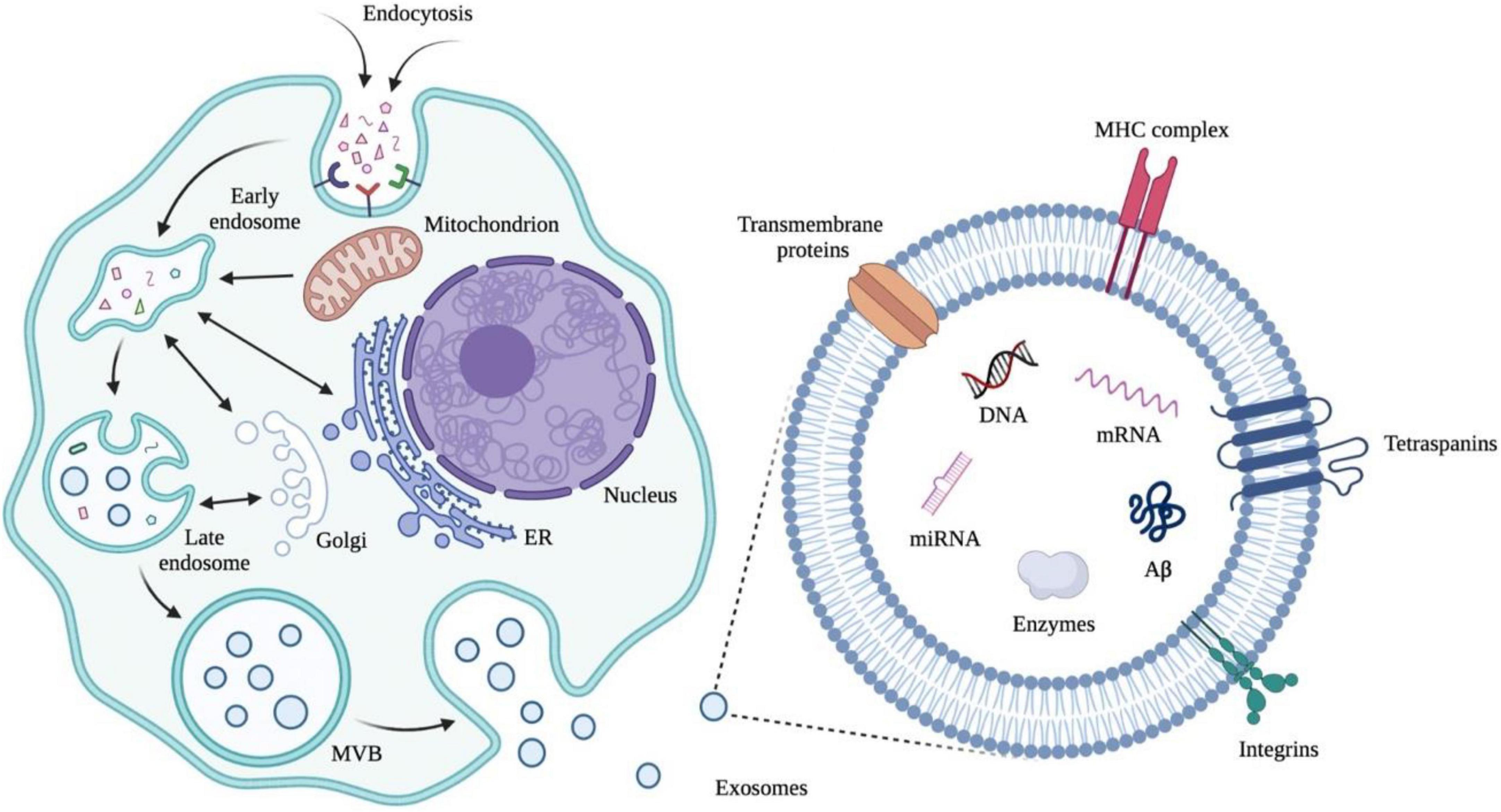
Figure 5. Early endosomes are generated from extracellular cargos as well as cell surface proteins through endocytosis. Mitochondria, endoplasmic reticulum (ER), and Golgi are involved in the formation of multivesicular bodies (MVBs) from early endosomes through fusion. MVBs can fuse with plasma membranes and release exosomes, which are membrane nanovesicles that contain plasma-membrane- and cytosol-associated molecules such as nuclear acids and proteins both inside and outside the vesicles. Illustrations were generated using BioRender.
In addition to biomarkers, clinical, physiological, and neuropsychological tests are also an important part of detecting clinically symptomatic persons. However, waiting periods for specialist appointments in hospitals can be very lengthy, causing severe and sometimes life-threatening delays for AD patients (Patterson et al., 2004). Besides, due to a lack of experience and skill, cognitive tests are often performed, scored, and interpreted inappropriately in primary care centers (Cannon and Larner, 2016), which gives rise to differences in diagnosis, evaluation, and assistance between primary care and specialty care systems (Garcia-Ptacek et al., 2017). Consequently, both physicians and patients will benefit a lot from a relatively simple first-line test determining which patients require more detailed diagnostics testing by measuring biomarkers in body fluid. Such a procedure would reduce the number of needless referrals and diagnostic tests and thus decrease the total pressure on healthcare systems (Hampel et al., 2017; O’Bryant et al., 2017). Furthermore, improvements in AD diagnosis produced by liquid biopsy could in turn promote symptomatic treatment (Hampel et al., 2021).
Conclusion
Undoubtedly, fluid-based biomarkers are crucial in the preclinical diagnosis of AD. For patients with cognitive problems, particularly those with mild memory loss as the only objective symptom, the current diagnostic method is incapable of predicting whether the end is Alzheimer’s disease or not (Tan et al., 2014). Having outlined a huge collection of the literature, a good deal of analytes that had been claimed to have the diagnosis value for AD were revealed. As we summarized above, accompanying the theory innovation, the liquid biopsy technologies including the equipment and kits emerge in endlessly. However, the practice has proven that the relentless efforts worldwide have not reached a satisfactory conclusion. In other words, no one strategy has “saved” the doctors and researchers from the current plight. The cause is varied and super-complicated.
First of all, the etiology of AD has many risk factors or heterogeneity, including but not limited to smoking, drinking, aging, education, and underlying medical conditions (obesity, smoking, diabetes, hypertension, and cardiac disease). Importantly, the principal cause has not been deduced. Even the well-established suspicious loci only account for 15∼20% of the AD pathology, which was often obtained from population-based studies and taken as the fluid biomarkers. As a result, the model in mathematical statistics is hard to build because of too many variables, especially the unknowns.
Secondly, none of the current technologies in liquid biopsy can perfectly meet the clinical demand. On one hand, the CSF biomarkers Aβ42, total tau, P-tau181, P-tau217, and P-tau231 show a high diagnostic accuracy for AD, even for prodromal AD patients with MCI. Therefore, they are increasingly being used in the clinic and valued as a similar gold standard. However, the procedure for acquiring CSF is expensive and the individuals should suffer a lumbar puncture and extra risk. On the other hand, fluid-based biomarkers significantly boost AD diagnosis by lowering expense and increasing ease of testing. As opposed to CSF markers, they may provide a minimally invasive, time- and cost-effective method of early detection and diagnosis of AD (Olsson et al., 2016). Taking the three aforementioned phosphor-tau in blood as an example, only in specific scenarios, they can show good performance by independent use.
Thirdly, several joint diagnostic strategies have been applied to reveal the full view of AD pathologies. The biomarker-based amyloid-tangle-neurodegeneration (A-T-N) framework proposed by Jack et al. (2016) and updated by NIA-AA (Jack et al., 2018) can capture the whole spectrum pathophysiological features of AD. By which, AD can be distinguished from other neurodegenerative diseases. Even so, in this scheme, not all of the pathologies and related biomarkers are included. Therefore, Huang et al. (2022) suggested adding an X to form the A-T-N-X framework, focusing on the CNS and periphery biomarkers associated with inflammation, systemic immunity, metabolism, and synaptic damage, neuroinflammation, glial cells. In the mid-to-long-term future, these frameworks should be validated in many clinical trials.
Lastly, many studies predict that faulty autophagy occurs before the formation of the fluid biomarkers such as Aβ or senile plaques, in the development of AD (Li W. et al., 2020; Lee et al., 2022). If in that case, the overwhelming majority of the aforementioned biomarkers cannot be employed in the routine clinical diagnostic for the very early stage of AD.
For now, it is foreseeable that an increasing number of blood-based biomarkers would be incorporated into diagnostic criteria and research frameworks for AD, in combination with current CSF biomarkers, to improve the preclinical and clinical diagnosis of AD, the world’s most common age-related neurodegenerative disease.
Author contributions
MT and HTZ designed the study. JL performed the data analysis. YL and XG wrote the manuscript All authors made a direct and intellectual contribution to this topic and approved the article for publication.
Funding
This work was financially supported by grants from the National Natural Science Foundation of China (32002235) and the Natural Science Foundation of Shandong Province (No. ZR2020MH141).
Acknowledgments
We thank all the individuals who have helped us in this study. We acknowledge the valuable work of the many investigators whose published articles we were unable to cite owing to space limitations.
Conflict of interest
The authors declare that the research was conducted in the absence of any commercial or financial relationships that could be construed as a potential conflict of interest.
Publisher’s note
All claims expressed in this article are solely those of the authors and do not necessarily represent those of their affiliated organizations, or those of the publisher, the editors and the reviewers. Any product that may be evaluated in this article, or claim that may be made by its manufacturer, is not guaranteed or endorsed by the publisher.
Footnotes
References
Abdel-Haq, H. (2020). The potential of liquid biopsy of the brain using blood extracellular vesicles: The first step toward effective neuroprotection against neurodegenerative diseases. Mol. Diagn. Ther. 24, 703–713. doi: 10.1007/s40291-020-00493-4
An, S. S., Lim, K. T., Oh, H. J., Lee, B. S., Zukic, E., Ju, Y. R., et al. (2010). Differentiating blood samples from scrapie infected and non-infected hamsters by detecting disease-associated prion proteins using Multimer Detection System. Biochem. Biophys. Res. Commun. 392, 505–509. doi: 10.1016/j.bbrc.2010.01.053
An, S. S. A., Lee, B. S., Yu, J. S., Lim, K., Kim, G. J., Lee, R., et al. (2017). Dynamic changes of oligomeric amyloid beta levels in plasma induced by spiked synthetic Abeta42. Alzheimers Res. Ther. 9:86. doi: 10.1186/s13195-017-0310-6
Andreasen, N., Minthon, L., Vanmechelen, E., Vanderstichele, H., Davidsson, P., Winblad, B., et al. (1999). Cerebrospinal fluid tau and Aβ42 as predictors of development of Alzheimer’s disease in patients with mild cognitive impairment. Neurosci. Lett. 273, 5–8. doi: 10.1016/S0304-3940(99)00617-5
Asberg, A., Lofblad, L., Felic, A., and Hov, G. G. (2019). Measuring calprotectin in plasma and blood with a fully automated turbidimetric assay. Scand. J. Clin. Lab. Invest. 79, 50–57. doi: 10.1080/00365513.2018.1550810
Ashton, N. J., Benedet, A. L., Pascoal, T. A., Karikari, T. K., Lantero-Rodriguez, J., Brum, W. S., et al. (2022). Cerebrospinal fluid p-tau231 as an early indicator of emerging pathology in Alzheimer’s disease. EBioMedicine 76:103836. doi: 10.1016/j.ebiom.2022.103836
Ashton, N. J., Blennow, K., and Zetterberg, H. (2021). Spitting image: Can saliva biomarkers reflect Alzheimer’s disease? EBioMedicine 68:103437. doi: 10.1016/j.ebiom.2021.103437
Ashton, N. J., Hye, A., Rajkumar, A. P., Leuzy, A., Snowden, S., Suarez-Calvet, M., et al. (2020). An update on blood-based biomarkers for non-Alzheimer neurodegenerative disorders. Nat. Rev. Neurol. 16, 265–284. doi: 10.1038/s41582-020-0348-0
Ashton, N. J., Ide, M., Zetterberg, H., and Blennow, K. (2019). Salivary biomarkers for Alzheimer’s disease and related disorders. Neurol. Ther. 8, 83–94. doi: 10.1007/s40120-019-00168-1
Atri, A. (2019). The Alzheimer’s disease clinical spectrum: Diagnosis and management. Med. Clin. North Am. 103, 263–293. doi: 10.1016/j.mcna.2018.10.009
Ausó, E., Gómez-Vicente, V., and Esquiva, G. (2020). Biomarkers for Alzheimer’s disease early diagnosis. J. Pers. Med. 10:114. doi: 10.3390/jpm10030114
Babapour Mofrad, R., Scheltens, P., Kim, S., Kang, S., Youn, Y. C., An, S. S. A., et al. (2021). Plasma amyloid-beta oligomerization assay as a pre-screening test for amyloid status. Alzheimers Res. Ther. 13:133.
Bacioglu, M., Maia, L. F., Preische, O., Schelle, J., Apel, A., Kaeser, S. A., et al. (2016). Neurofilament light chain in blood and CSF as marker of disease progression in mouse models and in neurodegenerative diseases. Neuron 91, 56–66. doi: 10.1186/s13195-021-00873-w
Baker-Nigh, A. T., Mawuenyega, K. G., Bollinger, J. G., Ovod, V., Kasten, T., Franklin, E. E., et al. (2016). Human Central Nervous System (CNS) ApoE isoforms are increased by age, differentially altered by amyloidosis, and relative amounts reversed in the CNS compared with plasma. J. Biol. Chem. 291, 27204–27218. doi: 10.1074/jbc.M116.721779
Balaj, L., Lessard, R., Dai, L., Cho, Y. J., Pomeroy, S. L., Breakefield, X. O., et al. (2011). Tumour microvesicles contain retrotransposon elements and amplified oncogene sequences. Nat. Commun. 2:180. doi: 10.1038/ncomms1180
Bǎlaşa, A. F., Chircov, C., and Grumezescu, A. M. (2020). Body fluid biomarkers for Alzheimer’s disease-an up-to-date overview. Biomedicines 8:421. doi: 10.3390/biomedicines8100421
Baldacci, F., Lista, S., Vergallo, A., Palermo, G., Giorgi, F. S., and Hampel, H. (2019). A frontline defense against neurodegenerative diseases:the development of early disease detection methods. Expert Rev. Mol. Diagn. 19, 559–563. doi: 10.1080/14737159.2019.1627202
Barbagallo, C., Mostile, G., Baglieri, G., Giunta, F., Luca, A., Raciti, L., et al. (2020). Specific signatures of serum miRNAs as potential biomarkers to discriminate clinically similar neurodegenerative and vascular-related diseases. Cell. Mol. Neurobiol. 40, 531–546. doi: 10.1007/s10571-019-00751-y
Barthelemy, N. R., Li, Y., Joseph-Mathurin, N., Gordon, B. A., Hassenstab, J., Benzinger, T. L. S., et al. (2020b). A soluble phosphorylated tau signature links tau, amyloid and the evolution of stages of dominantly inherited Alzheimer’s disease. Nat. Med. 26, 398–407. doi: 10.1038/s41591-020-0781-z
Barthelemy, N. R., Bateman, R. J., Hirtz, C., Marin, P., Becher, F., Sato, C., et al. (2020a). Cerebrospinal fluid phospho-tau T217 outperforms T181 as a biomarker for the differential diagnosis of Alzheimer’s disease and PET amyloid-positive patient identification. Alzheimers Res. Ther. 12:26. doi: 10.1186/s13195-020-00596-4
Basak, J. M., Kim, J., Pyatkivskyy, Y., Wildsmith, K. R., Jiang, H., Parsadanian, M., et al. (2012). Measurement of apolipoprotein E and amyloid β clearance rates in the mouse brain using bolus stable isotope labeling. Mol. Neurodegener. 7:14. doi: 10.1186/1750-1326-7-14
Bateman, R. J., Xiong, C., Benzinger, T. L., Fagan, A. M., Goate, A., Fox, N. C., et al. (2012). Clinical and biomarker changes in dominantly inherited Alzheimer’s disease. N. Engl. J. Med. 367, 795–804. doi: 10.1056/NEJMoa1202753
Bayoumy, S., Verberk, I. M. W., Den Dulk, B., Hussainali, Z., Zwan, M., Van Der Flier, W. M., et al. (2021). Clinical and analytical comparison of six Simoa assays for plasma P-tau isoforms P-tau181, P-tau217, and P-tau231. Alzheimers Res. Ther. 13:198. doi: 10.1186/s13195-021-00939-9
Benussi, A., Karikari, T. K., Ashton, N., Gazzina, S., Premi, E., Benussi, L., et al. (2020). Diagnostic and prognostic value of serum NfL and p-Tau181 in frontotemporal lobar degeneration. J. Neurol. Neurosurg. Psychiatry 91, 960–967. doi: 10.1136/jnnp-2020-323487
Bermejo-Pareja, F., Antequera, D., Vargas, T., Molina, J. A., and Carro, E. (2010). Saliva levels of Abeta1-42 as potential biomarker of Alzheimer’s disease: A pilot study. BMC Neurol. 10:108. doi: 10.1186/1471-2377-10-108
Bettcher, B. M., Johnson, S. C., Fitch, R., Casaletto, K. B., Heffernan, K. S., Asthana, S., et al. (2018). Cerebrospinal Fluid and Plasma Levels of Inflammation Differentially Relate to CNS Markers of Alzheimer’s Disease Pathology and Neuronal Damage. J. Alzheimers Dis. 62, 385–397. doi: 10.3233/JAD-170602
Birks, J. S., and Harvey, R. J. (2018). Donepezil for dementia due to Alzheimer’s disease. Cochrane Database Syst. Rev. 6:CD001190. doi: 10.1002/14651858.CD001190.pub3
Blanco, B. A., and Wolfgang, C. L. (2019). Liquid biopsy for the detection and management of surgically resectable tumors. Langenbecks Arch. Surg. 404, 517–525. doi: 10.1007/s00423-019-01788-9
Blennow, K., and Hampel, H. (2003). CSF markers for incipient Alzheimer’s disease. Lancet Neurol. 2, 605–613. doi: 10.1016/S1474-4422(03)00530-1
Blennow, K., Hampel, H., Weiner, M., and Zetterberg, H. (2010). Cerebrospinal fluid and plasma biomarkers in Alzheimer disease. Nat. Rev. Neurol. 6, 131–144. doi: 10.1038/nrneurol.2010.4
Blennow, K., Mattsson, N., Schöll, M., Hansson, O., and Zetterberg, H. (2015). Amyloid biomarkers in Alzheimer’s disease. Trends Pharmacol. Sci. 36, 297–309. doi: 10.1016/j.tips.2015.03.002
Blennow, K., Wallin, A., Ågren, H., Spenger, C., Siegfried, J., Vanmechelen, E. J. M., et al. (1995). Tau protein in cerebrospinal fluid. Mol. Chem. Neuropathol. 26, 231–245. doi: 10.1007/BF02815140
Blennow, K., and Zetterberg, H. (2015). Understanding biomarkers of neurodegeneration: Ultrasensitive detection techniques pave the way for mechanistic understanding. Nat. Med. 21, 217–219. doi: 10.1038/nm.3810
Blennow, K., and Zetterberg, H. (2018a). Biomarkers for Alzheimer’s disease: Current status and prospects for the future. J. Intern. Med. 284, 643–663. doi: 10.1111/joim.12816
Blennow, K., and Zetterberg, H. (2018b). The past and the future of Alzheimer’s disease fluid biomarkers. J. Alzheimers Dis. 62, 1125–1140. doi: 10.3233/JAD-170773
Blennow, K., and Zetterberg, H. (2019). Fluid biomarker-based molecular phenotyping of Alzheimer’s disease patients in research and clinical settings. Prog. Mol. Biol. Transl. Sci. 168, 3–23. doi: 10.1016/bs.pmbts.2019.07.006
Bouftas, M. (2021). A systematic review on the feasibility of salivary biomarkers for Alzheimer’s disease. J. Prev. Alzheimers Dis. 8, 84–91. doi: 10.14283/jpad.2020.57
Bramblett, G. T., Goedert, M., Jakes, R., Merrick, S. E., Trojanowski, J. Q., and Lee, V. M. (1993). Abnormal tau phosphorylation at Ser396 in Alzheimer’s disease recapitulates development and contributes to reduced microtubule binding. Neuron 10, 1089–1099. doi: 10.1016/0896-6273(93)90057-x
Breijyeh, Z., and Karaman, R. (2020). Comprehensive review on Alzheimer’s disease: Causes and treatment. Molecules 25:5789. doi: 10.3390/molecules25245789
Brickman, A. M., Manly, J. J., Honig, L. S., Sanchez, D., Reyes-Dumeyer, D., Lantigua, R. A., et al. (2021). Plasma p-tau181, p-tau217, and other blood-based Alzheimer’s disease biomarkers in a multi-ethnic, community study. Alzheimers Dement. 17, 1353–1364. doi: 10.1002/alz.12301
Buccellato, F. R., D’anca, M., Fenoglio, C., Scarpini, E., and Galimberti, D. (2021). Role of oxidative damage in Alzheimer’s disease and neurodegeneration: From pathogenic mechanisms to biomarker discovery. Antioxidants 10:1353. doi: 10.3390/antiox10091353
Buchhave, P., Minthon, L., Zetterberg, H., Wallin, Å. K., Blennow, K., and Hansson, O. J. (2012). Cerebrospinal fluid levels of β-amyloid 1-42, but not of tau, are fully changed already 5 to 10 years before the onset of Alzheimer dementia. Arch. Gen. Psychiatry 69, 98–106. doi: 10.1001/archgenpsychiatry.2011.155
Campbell, L., Simpson, D., Ramasamy, K., and Sadler, R. (2021). Using quantitative immunoprecipitation mass spectrometry (QIP-MS) to identify low level monoclonal proteins. Clin. Biochem. 95, 81–83. doi: 10.1016/j.clinbiochem.2021.05.012
Cannon, P., and Larner, A. J. (2016). Errors in the scoring and reporting of cognitive screening instruments administered in primary care. Neurodegener. Dis. Manag. 6, 271–276. doi: 10.2217/nmt-2016-0004
Carro, E., Bartolome, F., Bermejo-Pareja, F., Villarejo-Galende, A., Molina, J. A., Ortiz, P., et al. (2017). Early diagnosis of mild cognitive impairment and Alzheimer’s disease based on salivary lactoferrin. Alzheimers Dement. 8, 131–138. doi: 10.1016/j.dadm.2017.04.002
Cass, S. P. (2017). Alzheimer’s disease and exercise: A literature review. Curr. Sports Med. Rep. 16, 19–22. doi: 10.1249/JSR.0000000000000332
Cedazo-Minguez, A., and Winblad, B. (2010). Biomarkers for Alzheimer’s disease and other forms of dementia: Clinical needs, limitations and future aspects. Exp. Gerontol. 45, 5–14. doi: 10.1016/j.exger.2009.09.008
Cha, D. J., Mengel, D., Mustapic, M., Liu, W., Selkoe, D. J., Kapogiannis, D., et al. (2019). miR-212 and miR-132 are downregulated in neurally derived plasma exosomes of Alzheimer’s patients. Front. Neurosci. 13:1208. doi: 10.3389/fnins.2019.01208
Chen, D., Xu, T., Wang, S., Chang, H., Yu, T., Zhu, Y., et al. (2020). Liquid biopsy applications in the clinic. Mol. Diagn. Ther. 24, 125–132. doi: 10.1007/s40291-019-00444-8
Chen, X., Ren, G., Li, Y., Chao, W., Chen, S., Li, X., et al. (2022). Level of LncRNA GAS5 and hippocampal volume are associated with the progression of Alzheimer’s disease. Clin. Interv. Aging 17, 745–753. doi: 10.2147/CIA.S363116
Cheng, L., Doecke, J. D., Sharples, R. A., Villemagne, V. L., Fowler, C. J., Rembach, A., et al. (2015). Prognostic serum miRNA biomarkers associated with Alzheimer’s disease shows concordance with neuropsychological and neuroimaging assessment. Mol. Psychiatry 20, 1188–1196. doi: 10.1038/mp.2014.127
Cheng, L., Li, W., Chen, Y., Lin, Y., Wang, B., Guo, Q., et al. (2022). Plasma Abeta as a biomarker for predicting Abeta-PET status in Alzheimer’s diseasea systematic review with meta-analysis. J. Neurol. Neurosurg. Psychiatry 93, 513–520. doi: 10.1136/jnnp-2021-327864
Choi, Y., Joh, Y., Ryu, J. S., Kim, K., Seo, D., and Kim, S. (2021). Endogenous Abeta peptide promote Abeta oligomerization tendency of spiked synthetic Abeta in Alzheimer’s disease plasma. Mol. Cell. Neurosci. 111:103588. doi: 10.1016/j.mcn.2021.103588
Chu, L. W. (2012). Alzheimer’s disease: Early diagnosis and treatment. Hong Kong Med. J. 18, 228–237.
Cogswell, J. P., Ward, J., Taylor, I. A., Waters, M., Shi, Y., Cannon, B., et al. (2008). Identification of miRNA changes in Alzheimer’s disease brain and CSF yields putative biomarkers and insights into disease pathways. J. Alzheimers Dis. 14, 27–41. doi: 10.3233/jad-2008-14103
Cohen, L., Keegan, A., and Walt, D. R. (2020). Single-molecule arrays for ultrasensitive detection of blood-based biomarkers for immunotherapy. Methods Mol. Biol. 2055, 399–412. doi: 10.1007/978-1-4939-9773-2_18
Cohen, L., and Walt, D. R. (2017). Single-molecule arrays for protein and nucleic acid analysis. Annu. Rev. Anal. Chem. 10, 345–363. doi: 10.1146/annurev-anchem-061516-045340
Cohen, L., Xie, L., Xylas, M. E., and Walt, D. R. (2018). Single Molecule Arrays for ultra-sensitive detection of rat cytokines in serum. J. Immunol. Methods 452, 20–25. doi: 10.1016/j.jim.2017.10.002
Colletti, M., Ceglie, D., Di Giannatale, A., and Nazio, F. (2020). Autophagy and exosomes relationship in cancer: Friends or foes? Front. Cell Dev. Biol. 8:614178. doi: 10.3389/fcell.2020.614178
Cosin-Tomas, M., Antonell, A., Llado, A., Alcolea, D., Fortea, J., Ezquerra, M., et al. (2017). Plasma miR-34a-5p and miR-545-3p as early biomarkers of Alzheimer’s disease: Potential and limitations. Mol. Neurobiol. 54, 5550–5562. doi: 10.1007/s12035-016-0088-8
Crisp, M. J., Mawuenyega, K. G., Patterson, B. W., Reddy, N. C., Chott, R., Self, W. K., et al. (2015). In vivo kinetic approach reveals slow SOD1 turnover in the CNS. J. Clin. Invest. 125, 2772–2780. doi: 10.1172/JCI80705
De Rubis, G., Rajeev Krishnan, S., and Bebawy, M. (2019). Liquid biopsies in cancer diagnosis, monitoring, and prognosis. Trends Pharmacol. Sci. 40, 172–186. doi: 10.1016/j.tips.2019.01.006
Delgado-Peraza, F., Nogueras-Ortiz, C. J., Volpert, O., Liu, D., Goetzl, E. J., Mattson, M. P., et al. (2021). Neuronal and astrocytic extracellular vesicle biomarkers in blood reflect brain pathology in mouse models of Alzheimer’s disease. Cells 10:993. doi: 10.3390/cells10050993
Dhiman, K., Blennow, K., Zetterberg, H., Martins, R. N., and Gupta, V. B. (2019). Cerebrospinal fluid biomarkers for understanding multiple aspects of Alzheimer’s disease pathogenesis. Cell. Mol. Life Sci. 76, 1833–1863. doi: 10.1007/s00018-019-03040-5
Dincer, A., Gordon, B. A., Hari-Raj, A., Keefe, S. J., Flores, S., Mckay, N. S., et al. (2020). Comparing cortical signatures of atrophy between late-onset and autosomal dominant Alzheimer disease. Neuroimage Clin. 28:102491. doi: 10.1016/j.nicl.2020.102491
Dominguez, J. C., Yu, J. R. T., De Guzman, M. F., Ampil, E., Guevarra, A. C., Joson, M. L., et al. (2022). Multimer detection system-oligomerized amyloid beta (MDS-OAbeta): A plasma-based biomarker differentiates Alzheimer’s disease from other etiologies of dementia. Int. J. Alzheimers Dis. 2022:9960832. doi: 10.1155/2022/9960832
Dong, H., Li, J., Huang, L., Chen, X., Li, D., Wang, T., et al. (2015). Serum MicroRNA profiles serve as novel biomarkers for the diagnosis of Alzheimer’s disease. Dis. Markers 2015:625659. doi: 10.1155/2015/625659
Dubois, B., Feldman, H. H., Jacova, C., Hampel, H., Molinuevo, J. L., Blennow, K., et al. (2014). Advancing research diagnostic criteria for Alzheimer’s disease: The IWG-2 criteria. Lancet Neurol. 13, 614–629. doi: 10.1016/S1474-4422(14)70090-0
Elbert, D. L., Patterson, B. W., Lucey, B. P., Benzinger, T. L. S., and Bateman, R. J. (2022). Importance of CSF-based Abeta clearance with age in humans increases with declining efficacy of blood-brain barrier/proteolytic pathways. Commun. Biol. 5:98. doi: 10.1038/s42003-022-03037-0
Escola, J. M., Kleijmeer, M. J., Stoorvogel, W., Griffith, J. M., Yoshie, O., and Geuze, H. J. (1998). Selective enrichment of tetraspan proteins on the internal vesicles of multivesicular endosomes and on exosomes secreted by human B-lymphocytes. J. Biol. Chem. 273, 20121–20127. doi: 10.1074/jbc.273.32.20121
Ewers, M., Zhong, Z., Burger, K., Wallin, A., Blennow, K., Teipel, S. J., et al. (2008). Increased CSF-BACE 1 activity is associated with ApoE-epsilon 4 genotype in subjects with mild cognitive impairment and Alzheimer’s disease. Brain 131, 1252–1258. doi: 10.1093/brain/awn034
Farah, R., Haraty, H., Salame, Z., Fares, Y., Ojcius, D. M., and Said Sadier, N. (2018). Salivary biomarkers for the diagnosis and monitoring of neurological diseases. Biomed. J. 41, 63–87. doi: 10.1016/j.bj.2018.03.004
Femminella, G. D., Ferrara, N., and Rengo, G. (2015). The emerging role of microRNAs in Alzheimer’s disease. Front. Physiol. 6:40. doi: 10.3389/fphys.2015.00040
Ferreri, A. J. M., Calimeri, T., Lopedote, P., Francaviglia, I., Daverio, R., Iacona, C., et al. (2021). MYD88 L265P mutation and interleukin-10 detection in cerebrospinal fluid are highly specific discriminating markers in patients with primary central nervous system lymphoma: Results from a prospective study. Br. J. Haematol. 193, 497–505. doi: 10.1111/bjh.17357
Fotuhi, S. N., Khalaj-Kondori, M., Hoseinpour Feizi, M. A., and Talebi, M. (2019). Long non-coding RNA BACE1-AS may serve as an Alzheimer’s disease blood-based biomarker. J. Mol. Neurosci. 69, 351–359. doi: 10.1007/s12031-019-01364-2
Francois, M., Karpe, A., Liu, J. W., Beale, D., Hor, M., Hecker, J., et al. (2021). Salivaomics as a potential tool for predicting Alzheimer’s disease during the early stages of neurodegeneration. J. Alzheimers Dis. 82, 1301–1313. doi: 10.3233/JAD-210283
Gamez-Valero, A., Campdelacreu, J., Vilas, D., Ispierto, L., Rene, R., Alvarez, R., et al. (2019). Exploratory study on microRNA profiles from plasma-derived extracellular vesicles in Alzheimer’s disease and dementia with Lewy bodies. Transl. Neurodegener. 8:31. doi: 10.1186/s40035-019-0169-5
Gao, Y., Tan, L., Yu, J. T., and Tan, L. (2018). Tau in Alzheimer’s disease: Mechanisms and therapeutic strategies. Curr. Alzheimer Res. 15, 283–300. doi: 10.2174/1567205014666170417111859
García-Ayllón, M.-S., Campanari, M.-L., Brinkmalm, G., Rábano, A., Alom, J., Saura, C. A., et al. (2013). CSF Presenilin-1 complexes are increased in Alzheimer’s disease. Acta Neuropathol. Commun. 1:46. doi: 10.1186/2051-5960-1-46
Garcia-Ptacek, S., Modéer, I. N., Kåreholt, I., Fereshtehnejad, S.-M., Farahmand, B., Religa, D., et al. (2017). Differences in diagnostic process, treatment and social Support for Alzheimer’s dementia between primary and specialist care: Resultss from the Swedish Dementia Registry. Age Ageing 46, 314–319. doi: 10.1093/ageing/afw189
Gleerup, H. S., Hasselbalch, S. G., and Simonsen, A. H. (2019). Biomarkers for Alzheimer’s disease in saliva: A systematic review. Dis. Markers 2019:4761054. doi: 10.1155/2019/4761054
Goetzl, E. J., Boxer, A., Schwartz, J. B., Abner, E. L., Petersen, R. C., Miller, B. L., et al. (2015a). Low neural exosomal levels of cellular survival factors in Alzheimer’s disease. Ann. Clin. Transl. Neurol. 2, 769–773. doi: 10.1002/acn3.211
Goetzl, E. J., Boxer, A., Schwartz, J. B., Abner, E. L., Petersen, R. C., Miller, B. L., et al. (2015b). Altered lysosomal proteins in neural-derived plasma exosomes in preclinical Alzheimer disease. Neurology 85, 40–47. doi: 10.1212/WNL.0000000000001702
Goetzl, E. J., Kapogiannis, D., Schwartz, J. B., Lobach, I. V., Goetzl, L., Abner, E. L., et al. (2016). Decreased synaptic proteins in neuronal exosomes of frontotemporal dementia and Alzheimer’s disease. FASEB J. 30, 4141–4148. doi: 10.1096/fj.201600816R
Goetzl, E. J., Nogueras-Ortiz, C., Mustapic, M., Mullins, R. J., Abner, E. L., Schwartz, J. B., et al. (2019). Deficient neurotrophic factors of CSPG4-type neural cell exosomes in Alzheimer disease. FASEB J. 33, 231–238. doi: 10.1096/fj.201801001
Goldoni, R., Dolci, C., Boccalari, E., Inchingolo, F., Paghi, A., Strambini, L., et al. (2022). Salivary biomarkers of neurodegenerative and demyelinating diseases and biosensors for their detection. Ageing Res. Rev. 76:101587. doi: 10.1016/j.arr.2022.101587
Gonzalez-Sanchez, M., Bartolome, F., Antequera, D., Puertas-Martin, V., Gonzalez, P., Gomez-Grande, A., et al. (2020). Decreased salivary lactoferrin levels are specific to Alzheimer’s disease. EBioMedicine 57:102834. doi: 10.1016/j.ebiom.2020.102834
Goto, M., Chamoto, K., Higuchi, K., Yamashita, S., Noda, K., Iino, T., et al. (2019). Analytical performance of a new automated chemiluminescent magnetic immunoassays for soluble PD-1, PD-L1, and CTLA-4 in human plasma. Sci. Rep. 9:10144. doi: 10.1038/s41598-019-46548-3
Gouras, G. K., Olsson, T. T., and Hansson, O. (2015). beta-Amyloid peptides and amyloid plaques in Alzheimer’s disease. Neurotherapeutics 12, 3–11. doi: 10.1007/s13311-014-0313-y
Greening, D. W., Xu, R., Ji, H., Tauro, B. J., and Simpson, R. J. (2015). A protocol for exosome isolation and characterization: Evaluation of ultracentrifugation, density-gradient separation, and immunoaffinity capture methods. Methods Mol. Biol. 1295, 179–209. doi: 10.1007/978-1-4939-2550-6_15
Guard, S. E., Ebmeier, C. C., and Old, W. M. (2019). Label-free immunoprecipitation mass spectrometry workflow for large-scale nuclear interactome profiling. J. Vis. Exp. e60432. doi: 10.3791/60432
Guo, M., Hao, Y., Feng, Y., Li, H., Mao, Y., Dong, Q., et al. (2021). Microglial exosomes in neurodegenerative disease. Front. Mol. Neurosci. 14:630808. doi: 10.3389/fnmol.2021.630808
Gustafson, D. R., Skoog, I., Rosengren, L., Zetterberg, H., Blennow, K. J. J. O. N., and Neurosurgery, et al. (2007). Cerebrospinal fluid β-amyloid 1–42 concentration may predict cognitive decline in older women. J. Neurol. Neurosurg. Psychiatry 78, 461–464. doi: 10.1136/jnnp.2006.100529
Hampel, H., Lista, S., and Vergallo, A. (2019). Commentary: Development of the blood-based Alzheimer’s disease liquid biopsy. J. Prev. Alzheimers Dis. 6, 182–184. doi: 10.14283/jpad.2019.22
Hampel, H., O’Bryant, S. E., Durrleman, S., Younesi, E., Rojkova, K., Escott-Price, V., et al. (2017). A precision medicine initiative for Alzheimer’s disease: The road ahead to biomarker-guided integrative disease modeling. Climacteric 20, 107–118. doi: 10.1080/13697137.2017.1287866
Hampel, H., Vergallo, A., Caraci, F., Cuello, A. C., Lemercier, P., Vellas, B., et al. (2021). Future avenues for Alzheimer’s disease detection and therapy: Liquid biopsy, intracellular signaling modulation, systems pharmacology drug discovery. Neuropharmacology 185:108081. doi: 10.1016/j.neuropharm.2020.108081
Hane, F. T., Robinson, M., Lee, B. Y., Bai, O., Leonenko, Z., and Albert, M. S. (2017). Recent progress in Alzheimer’s disease research, Part 3: Diagnosis and treatment. J. Alzheimers Dis. 57, 645–665. doi: 10.3233/JAD-160907
Hansson, O., Seibyl, J., Stomrud, E., Zetterberg, H., Trojanowski, J. Q., Bittner, T., et al. (2018). CSF biomarkers of Alzheimer’s disease concord with amyloid−β PET and predict clinical progression: A study of fully automated immunoassays in BioFINDER and ADNI cohorts. Alzheimers Dement. 14, 1470–1481. doi: 10.1016/j.jalz.2018.01.010
Hansson, O., Zetterberg, H., Buchhave, P., Londos, E., Blennow, K., and Minthon, L. (2006). Association between CSF biomarkers and incipient Alzheimer’s disease in patients with mild cognitive impairment: A follow-up study. Lancet Neurol. 5, 228–234. doi: 10.1016/S1474-4422(06)70355-6
Hara, N., Kikuchi, M., Miyashita, A., Hatsuta, H., Saito, Y., Kasuga, K., et al. (2017). Serum microRNA miR-501-3p as a potential biomarker related to the progression of Alzheimer’s disease. Acta Neuropathol. Commun. 5:10. doi: 10.1186/s40478-017-0414-z
Hartmann, S., and Kist, T. B. L. (2018). A review of biomarkers of Alzheimer’s disease in noninvasive samples. Biomark. Med. 12, 677–690. doi: 10.2217/bmm-2017-0388
Hemler, M. E. (2003). Tetraspanin proteins mediate cellular penetration, invasion, and fusion events and define a novel type of membrane microdomain. Annu. Rev. Cell Dev. Biol. 19, 397–422. doi: 10.1146/annurev.cellbio.19.111301.153609
Hendricks, R., Baker, D., Brumm, J., Davancaze, T., Harp, C., Herman, A., et al. (2019). Establishment of neurofilament light chain Simoa assay in cerebrospinal fluid and blood. Bioanalysis 11, 1405–1418. doi: 10.4155/bio-2019-0163
Hersi, M., Irvine, B., Gupta, P., Gomes, J., Birkett, N., and Krewski, D. (2017). Risk factors associated with the onset and progression of Alzheimer’s disease: A systematic review of the evidence. Neurotoxicology 61, 143–187. doi: 10.1016/j.neuro.2017.03.006
Hesse, C., Rosengren, L., Andreasen, N., Davidsson, P., Vanderstichele, H., Vanmechelen, E., et al. (2001). Transient increase in total tau but not phospho-tau in human cerebrospinal fluid after acute stroke. Neurosci. Lett. 297, 187–190. doi: 10.1016/S0304-3940(00)01697-9
Hu, W. T., Chen-Plotkin, A., Arnold, S. E., Grossman, M., Clark, C. M., Shaw, L. M., et al. (2010). Novel CSF biomarkers for Alzheimer’s disease and mild cognitive impairment. Acta Neuropathol. 119, 669–678. doi: 10.1007/s00401-010-0667-0
Hu, Y. Y., He, S. S., Wang, X., Duan, Q. H., Grundke-Iqbal, I., Iqbal, K., et al. (2002). Levels of nonphosphorylated and phosphorylated tau in cerebrospinal fluid of Alzheimer’s disease patients: An ultrasensitive bienzyme-substrate-recycle enzyme-linked immunosorbent assay. Am. J. Pathol. 160, 1269–1278. doi: 10.1016/S0002-9440(10)62554-0
Huang, S., Wang, Y. J., and Guo, J. (2022). Biofluid biomarkers of Alzheimer’s disease: Progress, problems, and perspectives. Neurosci. Bull. 38, 677–691. doi: 10.1007/s12264-022-00836-7
Illan-Gala, I., Lleo, A., Karydas, A., Staffaroni, A. M., Zetterberg, H., Sivasankaran, R., et al. (2021). Plasma tau and neurofilament light in frontotemporal lobar degeneration and Alzheimer disease. Neurology 96, e671–e683. doi: 10.1212/WNL.0000000000011226
Ishiguro, K., Ohno, H., Arai, H., Yamaguchi, H., Urakami, K., Park, J.-M., et al. (1999). Phosphorylated tau in human cerebrospinal fluid is a diagnostic marker for Alzheimer’s disease. Neurosci. Lett. 270, 91–94. doi: 10.1016/S0304-3940(99)00476-0
Jack, C. R. Jr., Bennett, D. A., Blennow, K., Carrillo, M. C., Dunn, B., et al. (2018). NIA-AA research framework: Toward a biological definition of Alzheimer’s disease. Alzheimers Dement. 14, 535–562. doi: 10.1016/j.jalz.2018.02.018
Jafari, A., Babajani, A., Abdollahpour-Alitappeh, M., Ahmadi, N., and Rezaei-Tavirani, M. (2021). Exosomes and cancer: From molecular mechanisms to clinical applications. Med. Oncol. 38:45. doi: 10.1007/s12032-021-01491-0
Janelidze, S., Berron, D., Smith, R., Strandberg, O., Proctor, N. K., Dage, J. L., et al. (2021). Associations of Plasma Phospho-Tau217 levels with tau positron emission tomography in early Alzheimer disease. JAMA Neurol. 78, 149–156. doi: 10.1001/jamaneurol.2020.4201
Janelidze, S., Mattsson, N., Palmqvist, S., Smith, R., Beach, T. G., Serrano, G. E., et al. (2020a). Plasma P-tau181 in Alzheimer’s disease: Relationship to other biomarkers, differential diagnosis, neuropathology and longitudinal progression to Alzheimer’s dementia. Nat. Med. 26, 379–386. doi: 10.1038/s41591-020-0755-1
Janelidze, S., Stomrud, E., Smith, R., Palmqvist, S., Mattsson, N., Airey, D. C., et al. (2020b). Cerebrospinal fluid p-tau217 performs better than p-tau181 as a biomarker of Alzheimer’s disease. Nat. Commun. 11:1683. doi: 10.1038/s41467-020-15436-0
Janelidze, S., Zetterberg, H., Mattsson, N., Palmqvist, S., Vanderstichele, H., Lindberg, O., et al. (2016). CSF Aβ42/Aβ40 and Aβ42/Aβ38 ratios: Better diagnostic markers of Alzheimer disease. Ann. Clin. Transl. Neurol. 3, 154–165. doi: 10.1002/acn3.274
Jann, M. W. (2000). Rivastigmine, a new-generation cholinesterase inhibitor for the treatment of Alzheimer’s disease. Pharmacotherapy 20, 1–12. doi: 10.1592/phco.20.1.1.34664
Jia, L., Qiu, Q., Zhang, H., Chu, L., Du, Y., Zhang, J., et al. (2019). Concordance between the assessment of Abeta42, T-tau, and P-T181-tau in peripheral blood neuronal-derived exosomes and cerebrospinal fluid. Alzheimers Dement. 15, 1071–1080. doi: 10.1016/j.jalz.2019.05.002
Jia, L. H., and Liu, Y. N. (2016). Downregulated serum miR-223 servers as biomarker in Alzheimer’s disease. Cell Biochem. Funct. 34, 233–237. doi: 10.1002/cbf.3184
Jiang, L., Dong, H., Cao, H., Ji, X., Luan, S., and Liu, J. (2019). Exosomes in pathogenesis, diagnosis, and treatment of Alzheimer’s disease. Med. Sci. Monit. 25, 3329–3335. doi: 10.12659/MSM.914027
Johnstone, R. M., Adam, M., Hammond, J. R., Orr, L., and Turbide, C. (1987). Vesicle formation during reticulocyte maturation. Association of plasma membrane activities with released vesicles (exosomes). J. Biol. Chem. 262, 9412–9420. doi: 10.1016/S0021-9258(18)48095-7
Jack, C. R. jr., Bennett, D. A., Blennow, K., Carrillo, M. C., Feldman, H. H., Frisoni, G. B., et al. (2016). A/T/N- An unbiased descriptive classification scheme for Alzheimer disease biomarkers. Neurology 87, 539–547. doi: 10.1212/WNL.0000000000002923
Kahlert, C., Melo, S. A., Protopopov, A., Tang, J., Seth, S., Koch, M., et al. (2014). Identification of double-stranded genomic DNA spanning all chromosomes with mutated KRAS and p53 DNA in the serum exosomes of patients with pancreatic cancer. J. Biol. Chem. 289, 3869–3875. doi: 10.1074/jbc.C113.532267
Kalluri, R., and LeBleu, V. S. (2020). The biology, function, and biomedical applications of exosomes. Science 367:eaau6977. doi: 10.1126/science.aau6977
Kanninen, K. M., Bister, N., Koistinaho, J., and Malm, T. (2016). Exosomes as new diagnostic tools in CNS diseases. Biochim. Biophys. Acta 1862, 403–410. doi: 10.1016/j.bbadis.2015.09.020
Karikari, T. K., Benedet, A. L., Ashton, N. J., Lantero Rodriguez, J., Snellman, A., Suarez-Calvet, M., et al. (2021a). Diagnostic performance and prediction of clinical progression of plasma phospho-tau181 in the Alzheimer’s Disease Neuroimaging Initiative. Mol. Psychiatry 26, 429–442. doi: 10.1038/s41380-020-00923-z
Karikari, T. K., Emersic, A., Vrillon, A., Lantero-Rodriguez, J., Ashton, N. J., Kramberger, M. G., et al. (2021b). Head-to-head comparison of clinical performance of CSF phospho-tau T181 and T217 biomarkers for Alzheimer’s disease diagnosis. Alzheimers Dement. 17, 755–767. doi: 10.1002/alz.12236
Karikari, T. K., Pascoal, T. A., Ashton, N. J., Janelidze, S., Benedet, A. L., Rodriguez, J. L., et al. (2020). Blood phosphorylated tau 181 as a biomarker for Alzheimer’s disease: A diagnostic performance and prediction modelling study using data from four prospective cohorts. Lancet Neurol. 19, 422–433. doi: 10.1016/S1474-4422(20)30071-5
Katsipis, G., Tzekaki, E. E., Tsolaki, M., and Pantazaki, A. A. (2021). Salivary GFAP as a potential biomarker for diagnosis of mild cognitive impairment and Alzheimer’s disease and its correlation with neuroinflammation and apoptosis. J. Neuroimmunol. 361:577744. doi: 10.1016/j.jneuroim.2021.577744
Kenny, A., Mcardle, H., Calero, M., Rabano, A., Madden, S. F., Adamson, K., et al. (2019). Elevated plasma microRNA-206 levels predict cognitive decline and progression to dementia from mild cognitive impairment. Biomolecules 9:734. doi: 10.3390/biom9110734
Keshavan, A., Pannee, J., Karikari, T. K., Rodriguez, J. L., Ashton, N. J., Nicholas, J. M., et al. (2021). Population-based blood screening for preclinical Alzheimer’s disease in a British birth cohort at age 70. Brain 144, 434–449.
Kester, M. I., Teunissen, C. E., Sutphen, C., Herries, E. M., Ladenson, J. H., Xiong, C., et al. (2015). Cerebrospinal fluid VILIP-1 and YKL-40, candidate biomarkers to diagnose, predict and monitor Alzheimer’s disease in a memory clinic cohort. Alzheimers Res. Ther. 7:59. doi: 10.1186/s13195-015-0142-1
Khan, S. S., and Bloom, G. S. (2016). Tau: The center of a signaling nexus in Alzheimer’s disease. Front. Neurosci. 10:31. doi: 10.3389/fnins.2016.00031
Kim, C. B., Choi, Y. Y., Song, W. K., and Song, K. B. (2014). Antibody-based magnetic nanoparticle immunoassay for quantification of Alzheimer’s disease pathogenic factor. J. Biomed. Opt. 19:051205. doi: 10.1117/1.JBO.19.5.051205
Kloten, V., Lampignano, R., Krahn, T., and Schlange, T. (2019). Circulating tumor cell PD-L1 expression as biomarker for therapeutic efficacy of immune checkpoint inhibition in NSCLC. Cells 8:809. doi: 10.3390/cells8080809
Knapskog, A. B., Henjum, K., Idland, A. V., Eldholm, R. S., Persson, K., Saltvedt, I., et al. (2020). Cerebrospinal fluid sTREM2 in Alzheimer’s disease: Comparisons between clinical presentation and AT classification. Sci. Rep. 10:15886. doi: 10.1038/s41598-020-72878-8
Kohnken, R., Buerger, K., Zinkowski, R., Miller, C., Kerkman, D., Debernardis, J., et al. (2000). Detection of tau phosphorylated at threonine 231 in cerebrospinal fluid of Alzheimer’s disease patients. Neurosci. Lett. 287, 187–190. doi: 10.1016/S0304-3940(00)01178-2
Kugeratski, F. G., and Kalluri, R. (2021). Exosomes as mediators of immune regulation and immunotherapy in cancer. FEBS J. 288, 10–35. doi: 10.1111/febs.15558
Kulichikhin, K. Y., Fedotov, S. A., Rubel, M. S., Zalutskaya, N. M., Zobnina, A. E., Malikova, O. A., et al. (2021). Development of molecular tools for diagnosis of Alzheimer’s disease that are based on detection of amyloidogenic proteins. Prion 15, 56–69. doi: 10.1080/19336896.2021.1917289
Kumar, S., and Reddy, P. H. (2018). MicroRNA-455-3p as a potential biomarker for Alzheimer’s disease: An update. Front. Aging Neurosci. 10:41. doi: 10.3389/fnagi.2018.00041
Lakshmi, S., Hughes, T. A., and Priya, S. (2021). Exosomes and exosomal RNAs in breast cancer: A status update. Eur. J. Cancer 144, 252–268. doi: 10.1016/j.ejca.2020.11.033
Lane, C. A., Hardy, J., and Schott, J. M. (2018). Alzheimer’s disease. Eur. J. Neurol. 25, 59–70. doi: 10.1111/ene.13439
Lantero-Rodriguez, J., Snellman, A., Benedet, A. L., Mila-Aloma, M., Camporesi, E., Montoliu-Gaya, L., et al. (2021). P-tau235: A novel biomarker for staging preclinical Alzheimer’s disease. EMBO Mol. Med. 13:e15098. doi: 10.15252/emmm.202115098
Lashley, T., Schott, J. M., Weston, P., Murray, C. E., Wellington, H., Keshavan, A., et al. (2018). Molecular biomarkers of Alzheimer’s disease: Progress and prospects. Dis. Model. Mech. 11:dmm031781. doi: 10.1242/dmm.031781
Lau, H. C., Lee, I. K., Ko, P. W., Lee, H. W., Huh, J. S., Cho, W. J., et al. (2015). Non-invasive screening for Alzheimer’s disease by sensing salivary sugar using Drosophila cells expressing gustatory receptor (Gr5a) immobilized on an extended gate ion-sensitive field-effect transistor (EG-ISFET) biosensor. PLoS One 10:e0117810. doi: 10.1371/journal.pone.0117810
Lee, H., Ugay, D., Hong, S., and Kim, Y. (2020). Alzheimer’s disease diagnosis using misfolding proteins in Blood. Dement. Neurocogn. Disord. 19, 1–18. doi: 10.12779/dnd.2020.19.1.1
Lee, J. C., Kim, S. J., Hong, S., and Kim, Y. (2019). Diagnosis of Alzheimer’s disease utilizing amyloid and tau as fluid biomarkers. Exp. Mol. Med. 51, 1–10. doi: 10.1038/s12276-019-0299-y
Lee, J. H., Yang, D. S., Goulbourne, C. N., Im, E., Stavrides, P., Pensalfini, A., et al. (2022). Faulty autolysosome acidification in Alzheimer’s disease mouse models induces autophagic build-up of Abeta in neurons, yielding senile plaques. Nat. Neurosci. 25, 688–701. doi: 10.1038/s41593-022-01084-8
Lee, M., Guo, J. P., Kennedy, K., Mcgeer, E. G., and Mcgeer, P. L. (2017). A method for diagnosing Alzheimer’s disease based on salivary amyloid-β protein 42 levels. J. Alzheimers Dis. 55, 1175–1182. doi: 10.3233/JAD-160748
Leuzy, A., Ashton, N. J., Mattsson-Carlgren, N., Dodich, A., Boccardi, M., Corre, J., et al. (2021). 2020 update on the clinical validity of cerebrospinal fluid amyloid, tau, and phospho-tau as biomarkers for Alzheimer’s disease in the context of a structured 5-phase development framework. Eur. J. Nucl. Med. Mol. Imaging 48, 2121–2139. doi: 10.1007/s00259-021-05258-7
Lewczuk, P., Matzen, A., Blennow, K., Parnetti, L., Molinuevo, J. L., Eusebi, P., et al. (2017). Cerebrospinal Fluid Aβ 42/40 Corresponds Better than Aβ 42 to Amyloid PET in Alzheimer’s Disease. J. Alzheimers Dis. 55, 813–822. doi: 10.3233/JAD-160722
Li, Y., Guan, S., Jin, H., Liu, H., Kang, M., Wang, X., et al. (2020). The relationship between urinary Alzheimer-associated neuronal thread protein and blood biochemical indicators in the general population. Aging 12, 15260–15280. doi: 10.18632/aging.103356
Li, W., Kui, L., Demetrios, T., Gong, X., and Tang, M. (2020). A glimmer of hope: Maintain mitochondrial homeostasis to mitigate Alzheimer’s disease. Aging Dis. 11, 1260–1275. doi: 10.14336/AD.2020.0105
Li, Y., Lim, E., Fields, T., Wu, H., Xu, Y., Wang, Y. A., et al. (2019). Improving sensitivity and specificity of amyloid-beta peptides and tau protein detection with antibiofouling magnetic nanoparticles for liquid biopsy of Alzheimer’s disease. ACS Biomater. Sci. Eng. 5, 3595–3605. doi: 10.1021/acsbiomaterials.9b00086
Li, Y., Xiong, C., Aschenbrenner, A. J., Chang, C. H., Weiner, M. W., Nosheny, R. L., et al. (2021). Item response theory analysis of the Clinical Dementia Rating. Alzheimers Dement. 17, 534–542. doi: 10.1002/alz.12210
Liang, D., and Lu, H. (2019). Salivary biological biomarkers for Alzheimer’s disease. Arch. Oral Biol. 105, 5–12. doi: 10.1016/j.archoralbio.2019.06.004
Liang, Y., Eng, W. S., Colquhoun, D. R., Dinglasan, R. R., Graham, D. R., and Mahal, L. K. (2014). Complex N-linked glycans serve as a determinant for exosome/microvesicle cargo recruitment. J. Biol. Chem. 289, 32526–32537. doi: 10.1074/jbc.M114.606269
Lim, K., Kim, S. Y., Lee, B., Segarra, C., Kang, S., Ju, Y., et al. (2015). Magnetic microparticle-based multimer detection system for the detection of prion oligomers in sheep. Int. J. Nanomedicine 10, 241–250. doi: 10.2147/IJN.S88377
Lindwall, G., and Cole, R. D. (1984). Phosphorylation affects the ability of tau protein to promote microtubule assembly. J. Biol. Chem. 259, 5301–5305. doi: 10.1016/S0021-9258(17)42989-9
Lista, S., Faltraco, F., Prvulovic, D., and Hampel, H. (2013). Blood and plasma-based proteomic biomarker research in Alzheimer’s disease. Prog. Neurobiol. 101-102, 1–17. doi: 10.1016/j.pneurobio.2012.06.007
Liu, C. G., Song, J., Zhang, Y. Q., and Wang, P. C. (2014). MicroRNA-193b is a regulator of amyloid precursor protein in the blood and cerebrospinal fluid derived exosomal microRNA-193b is a biomarker of Alzheimer’s disease. Mol. Med. Rep. 10, 2395–2400. doi: 10.3892/mmr.2014.2484
Liu, J., Chen, Y., Pei, F., Zeng, C., Yao, Y., Liao, W., et al. (2021). Extracellular vesicles in liquid biopsies: Potential for disease diagnosis. Biomed Res. Int. 2021:6611244. doi: 10.1155/2021/6611244
Liu, L., Liu, L., Lu, Y., Zhang, T., and Zhao, W. (2021). Serum aberrant expression of miR-24-3p and its diagnostic value in Alzheimer’s disease. Biomark. Med. 15, 1499–1507. doi: 10.2217/bmm-2021-0098
Liu, T., Hooda, J., Atkinson, J. M., Whiteside, T. L., Oesterreich, S., and Lee, A. V. (2021). Exosomes in breast cancer - mechanisms of action and clinical potential. Mol. Cancer Res. 19, 935–945. doi: 10.1158/1541-7786.MCR-20-0952
Liu, L., Chen, X., Chen, Y. H., and Zhang, K. (2020). Identification of Circular RNA hsa_Circ_0003391 in peripheral blood is potentially associated with Alzheimer’s disease. Front. Aging Neurosci. 12:601965. doi: 10.3389/fnagi.2020.601965
Lue, L. F., Kuo, Y. M., and Sabbagh, M. (2019). Advance in plasma AD core biomarker development: Current findings from immunomagnetic reduction-based SQUID technology. Neurol. Ther. 8, 95–111. doi: 10.1007/s40120-019-00167-2
Lugli, G., Cohen, A. M., Bennett, D. A., Shah, R. C., Fields, C. J., Hernandez, A. G., et al. (2015). Plasma Exosomal miRNAs in persons with and without Alzheimer disease: Altered expression and prospects for biomarkers. PLoS One 10:e0139233. doi: 10.1371/journal.pone.0139233
Lukiw, W. J. (2007). Micro-RNA speciation in fetal, adult and Alzheimer’s disease hippocampus. Neuroreport 18, 297–300. doi: 10.1097/WNR.0b013e3280148e8b
Lukiw, W. J., Alexandrov, P. N., Zhao, Y., Hill, J. M., and Bhattacharjee, S. (2012a). Spreading of Alzheimer’s disease inflammatory signaling through soluble micro-RNA. Neuroreport 23, 621–626. doi: 10.1097/00001756-201207110-00009
Lukiw, W. J., Andreeva, T. V., Grigorenko, A. P., and Rogaev, E. I. (2012b). Studying micro RNA function and dysfunction in Alzheimer’s disease. Front. Genet. 3:327. doi: 10.3389/fgene.2012.00327
Mader, S., and Pantel, K. (2017). Liquid biopsy: Current status and future perspectives. Oncol. Res. Treat. 40, 404–408. doi: 10.1159/000478018
Manouchehrinia, A., Piehl, F., Hillert, J., Kuhle, J., Alfredsson, L., Olsson, T., et al. (2020). Confounding effect of blood volume and body mass index on blood neurofilament light chain levels. Ann. Clin. Transl. Neurol. 7, 139–143. doi: 10.1002/acn3.50972
Markesbery, W. R., Schmitt, F. A., Kryscio, R. J., Davis, D. G., Smith, C. D., and Wekstein, D. R. (2006). Neuropathologic substrate of mild cognitive impairment. Arch. Neurol. 63, 38–46. doi: 10.1001/archneur.63.1.38
Mattsson, N., Andreasson, U., Zetterberg, H., and Blennow, K., Alzheimer’s Disease, and Neuroimaging I. (2017). Association of plasma neurofilament light with neurodegeneration in patients with Alzheimer disease. JAMA Neurol. 74, 557–566. doi: 10.1001/jamaneurol.2016.6117
Mattsson, N., Cullen, N. C., Andreasson, U., Zetterberg, H., and Blennow, K. (2019). Association between longitudinal plasma neurofilament light and neurodegeneration in patients with Alzheimer disease. JAMA Neurol. 76, 791–799. doi: 10.1001/jamaneurol.2019.0765
Mawuenyega, K. G., Kasten, T., Sigurdson, W., and Bateman, R. J. (2013). Amyloid-beta isoform metabolism quantitation by stable isotope-labeled kinetics. Anal. Biochem. 440, 56–62. doi: 10.1016/j.ab.2013.04.031
Mayeux, R., Honig, L. S., Tang, M.-X., Manly, J., Stern, Y., Schupf, N., et al. (2003). Plasma Aβ40 and Aβ42 and Alzheimer’s disease: Relation to age, mortality, and risk. Neurology 61, 1185–1190. doi: 10.1212/01.WNL.0000091890.32140.8F
McGeer, P. L., Guo, J. P., Lee, M., Kennedy, K., and Mcgeer, E. G. (2018). Alzheimer’s disease can be spared by nonsteroidal anti-inflammatory drugs. J. Alzheimers Dis. 62, 1219–1222. doi: 10.3233/JAD-170706
McGowan, E., Pickford, F., Kim, J., Onstead, L., Eriksen, J., Yu, C., et al. (2005). Aβ42 is essential for parenchymal and vascular amyloid deposition in mice. Neuron 47, 191–199. doi: 10.1016/j.neuron.2005.06.030
Meng, X., Li, T., Wang, X., Lv, X., Sun, Z., Zhang, J., et al. (2019). Association between increased levels of amyloid-beta oligomers in plasma and episodic memory loss in Alzheimer’s disease. Alzheimers Res. Ther. 11:89. doi: 10.1186/s13195-019-0535-7
Miura, M., Fujinami, N., Shimizu, Y., Mizuno, S., Saito, K., Suzuki, T., et al. (2020). Usefulness of plasma full-length glypican-3 as a predictive marker of hepatocellular carcinoma recurrence after radial surgery. Oncol. Lett. 19, 2657–2666. doi: 10.3892/ol.2020.11371
Molinuevo, J. L., Ayton, S., Batrla, R., Bednar, M. M., Bittner, T., Cummings, J., et al. (2018). Current state of Alzheimer’s fluid biomarkers. Acta Neuropathol. 136, 821–853. doi: 10.1007/s00401-018-1932-x
Molinuevo, J. L., Blennow, K., Dubois, B., Engelborghs, S., Lewczuk, P., Perret-Liaudet, A., et al. (2014). The clinical use of cerebrospinal fluid biomarker testing for Alzheimer’s disease diagnosis: A consensus paper from the Alzheimer’s Biomarkers Standardization Initiative. Alzheimers Dement. 10, 808–817. doi: 10.1016/j.jalz.2014.03.003
Monte, S. M. D. L., and Wands, J. R. (2001). Alzheimer-associated neuronal thread protein-induced apoptosis and impaired mitochondrial function in human central nervous system-derived neuronal cells. J. Neuropathol. Exp. Neurol. 60, 195–207. doi: 10.1093/jnen/60.2.195
Moscoso, A., Grothe, M. J., Ashton, N. J., Karikari, T. K., Rodriguez, J. L., Snellman, A., et al. (2021). Time course of phosphorylated-tau181 in blood across the Alzheimer’s disease spectrum. Brain 144, 325–339. doi: 10.1093/brain/awaa399
Mullins, R. J., Mustapic, M., Goetzl, E. J., and Kapogiannis, D. (2017). Exosomal biomarkers of brain insulin resistance associated with regional atrophy in Alzheimer’s disease. Hum. Brain Mapp. 38, 1933–1940. doi: 10.1002/hbm.23494
Muraoka, S., Jedrychowski, M. P., Yanamandra, K., Ikezu, S., Gygi, S. P., and Ikezu, T. (2020). Proteomic profiling of extracellular vesicles derived from cerebrospinal fluid of Alzheimer’s disease patients: A pilot study. Cells 9:1959. doi: 10.3390/cells9091959
Mustapic, M., Eitan, E., Werner, J. K. Jr., Berkowitz, S. T., Lazaropoulos, M. P., et al. (2017). Plasma extracellular vesicles enriched for neuronal origin: A potential window into brain pathologic processes. Front. Neurosci. 11:278. doi: 10.3389/fnins.2017.00278
Naseri, N. N., Wang, H., Guo, J., Sharma, M., and Luo, W. (2019). The complexity of tau in Alzheimer’s disease. Neurosci. Lett. 705, 183–194. doi: 10.1016/j.neulet.2019.04.022
Nitsch, R. M., Blusztajn, J. K., Pittas, A. G., Slack, B. E., Growdon, J. H., and Wurtman, R. J. (1992). Evidence for a membrane defect in Alzheimer disease brain. Proc. Natl. Acad. Sci. U.S.A. 89, 1671–1675. doi: 10.1073/pnas.89.5.1671
Noda, K., Matsuda, K., Yagishita, S., Maeda, K., Akiyama, Y., Terada-Hirashima, J., et al. (2021). A novel highly quantitative and reproducible assay for the detection of anti-SARS-CoV-2 IgG and IgM antibodies. Sci. Rep. 11:5198. doi: 10.1038/s41598-021-84387-3
Nutu, M., Zetterberg, H., Londos, E., Minthon, L., Nägga, K., Blennow, K., et al. (2013). Evaluation of the cerebrospinal fluid amyloid-β1-42/amyloid-β1-40 ratio measured by alpha-LISA to distinguish Alzheimer’s disease from other dementia disorders. Dement. Geriatr. Cogn. Disord. 36, 99–110. doi: 10.1159/000353442
O’Brien, R. J., and Wong, P. C. (2011). Amyloid precursor protein processing and Alzheimer’s disease. Annu. Rev. Neurosci. 34, 185–204. doi: 10.1146/annurev-neuro-061010-113613
O’Bryant, S. E., Gupta, V., Henriksen, K., Edwards, M., Jeromin, A., Lista, S., et al. (2015). Guidelines for the standardization of preanalytic variables for blood-based biomarker studies in Alzheimer’s disease research. Alzheimers Dement. 11, 549–560. doi: 10.1016/j.jalz.2014.08.099
O’Bryant, S. E., Mielke, M. M., Rissman, R. A., Lista, S., Vanderstichele, H., Zetterberg, H., et al. (2017). Blood-based biomarkers in Alzheimer disease: Current state of the science and a novel collaborative paradigm for advancing from discovery to clinic. Alzheimers Dement. 13, 45–58. doi: 10.1016/j.jalz.2016.09.014
Olsson, B., Lautner, R., Andreasson, U., Öhrfelt, A., Portelius, E., Bjerke, M., et al. (2016). CSF and blood biomarkers for the diagnosis of Alzheimer’s disease: A systematic review and meta-analysis. Lancet Neurol. 15, 673–684. doi: 10.1016/S1474-4422(16)00070-3
Ovod, V., Ramsey, K. N., Mawuenyega, K. G., Bollinger, J. G., Hicks, T., Schneider, T., et al. (2017). Amyloid beta concentrations and stable isotope labeling kinetics of human plasma specific to central nervous system amyloidosis. Alzheimers Dement. 13, 841–849. doi: 10.1016/j.jalz.2017.06.2266
Palmqvist, S., Janelidze, S., Quiroz, Y. T., Zetterberg, H., Lopera, F., Stomrud, E., et al. (2020). Discriminative accuracy of plasma phospho-tau217 for Alzheimer disease vs other neurodegenerative disorders. JAMA 324, 772–781. doi: 10.1001/jama.2020.12134
Palmqvist, S., Janelidze, S., Stomrud, E., Zetterberg, H., Karl, J., Zink, K., et al. (2019). Performance of fully automated plasma assays as screening tests for Alzheimer disease-related beta-amyloid status. JAMA Neurol. 76, 1060–1069. doi: 10.1001/jamaneurol.2019.1632
Pannee, J., Portelius, E., Minthon, L., Gobom, J., Andreasson, U., Zetterberg, H., et al. (2016). Reference measurement procedure for CSF amyloid beta (Aβ) 1–42 and the CSF Aβ1–42/Aβ1–40 ratio–a cross-validation study against amyloid PET. J. Neurochem. 139, 651–658. doi: 10.1111/jnc.13838
Paraskevaidi, M., Allsop, D., Karim, S., Martin, F. L., and Crean, S. (2020). Diagnostic biomarkers for Alzheimer’s disease using non-invasive specimens. J. Clin. Med. 9:1673. doi: 10.3390/jcm9061673
Park, S. A., Han, S. M., and Kim, C. E. (2020). New fluid biomarkers tracking non-amyloid-beta and non-tau pathology in Alzheimer’s disease. Exp. Mol. Med. 52, 556–568. doi: 10.1038/s12276-020-0418-9
Paterson, R. W., Gabelle, A., Lucey, B. P., Barthelemy, N. R., Leckey, C. A., Hirtz, C., et al. (2019). SILK studies - capturing the turnover of proteins linked to neurodegenerative diseases. Nat. Rev. Neurol. 15, 419–427. doi: 10.1038/s41582-019-0222-0
Patterson, V., Humphreys, J., and Chua, R. J. J. O. N., Neurosurgery, and Psychiatry. (2004). Email triage of new neurological outpatient referrals from general practice. J. Neurol. Neurosurg. Psychiatry <refvol> 75, 617–620. doi: 10.1136/jnnp.2003.024489
Pawlik, P., and Blochowiak, K. (2021). The role of salivary biomarkers in the early diagnosis of Alzheimer’s disease and Parkinson’s disease. Diagnostics 11:371. doi: 10.3390/diagnostics11020371
Pegtel, D. M., Cosmopoulos, K., Thorley-Lawson, D. A., Van Eijndhoven, M. A., Hopmans, E. S., Lindenberg, J. L., et al. (2010). Functional delivery of viral miRNAs via exosomes. Proc. Natl. Acad. Sci. U.S.A. 107, 6328–6333. doi: 10.1073/pnas.0914843107
Pegtel, D. M., and Gould, S. J. (2019). Exosomes. Annu. Rev. Biochem. 88, 487–514. doi: 10.1146/annurev-biochem-013118-111902
Pena-Bautista, C., Tirle, T., Lopez-Nogueroles, M., Vento, M., Baquero, M., and Chafer-Pericas, C. (2019). Oxidative damage of DNA as early marker of Alzheimer’s disease. Int. J. Mol. Sci. 20:6136. doi: 10.3390/ijms20246136
Pereira, J. B., Janelidze, S., Stomrud, E., Palmqvist, S., Van Westen, D., Dage, J. L., et al. (2021). Plasma markers predict changes in amyloid, tau, atrophy and cognition in non-demented subjects. Brain 144, 2826–2836. doi: 10.1093/brain/awab163
Pessoa, L. S., Heringer, M., and Ferrer, V. P. (2020). ctDNA as a cancer biomarker: A broad overview. Crit. Rev. Oncol. Hematol. 155:103109. doi: 10.1016/j.critrevonc.2020.103109
Pinnell, J. R., Cui, M., and Tieu, K. (2021). Exosomes in Parkinson disease. J. Neurochem. 157, 413–428. doi: 10.1111/jnc.15288
Platenik, J., Fisar, Z., Buchal, R., Jirak, R., Kitzlerova, E., Zverova, M., et al. (2014). GSK3beta, CREB, and BDNF in peripheral blood of patients with Alzheimer’s disease and depression. Prog. Neuropsychopharmacol. Biol. Psychiatry 50, 83–93. doi: 10.1016/j.pnpbp.2013.12.001
Portelius, E., Lashley, T., Westerlund, A., Persson, R., Fox, N. C., Blennow, K., et al. (2015). Brain amyloid-beta fragment signatures in pathological ageing and Alzheimer’s disease by hybrid immunoprecipitation mass spectrometry. Neurodegener. Dis. 15, 50–57. doi: 10.1159/000369465
Portelius, E., Westman-Brinkmalm, A., Zetterberg, H., and Blennow, K. (2006). Determination of beta-amyloid peptide signatures in cerebrospinal fluid using immunoprecipitation-mass spectrometry. J. Proteome Res. 5, 1010–1016. doi: 10.1021/pr050475v
Potter, R., Patterson, B. W., Elbert, D. L., Ovod, V., Kasten, T., Sigurdson, W., et al. (2013). Increased in vivo amyloid-β42 production, exchange, and loss in presenilin mutation carriers. Sci. Transl. Med. 5:189ra177. doi: 10.1126/scitranslmed.3005615
Poulet, G., Massias, J., and Taly, V. (2019). Liquid biopsy: General concepts. Acta Cytol. 63, 449–455. doi: 10.1159/000499337
Preische, O., Schultz, S. A., Apel, A., Kuhle, J., Kaeser, S. A., Barro, C., et al. (2019). Serum neurofilament dynamics predicts neurodegeneration and clinical progression in presymptomatic Alzheimer’s disease. Nat. Med. 25, 277–283. doi: 10.1038/s41591-018-0304-3
Pu, Z., Tang, X., Fei, Y., Hou, Q., Lin, Y., and Zha, X. (2020). Bone metabolic biomarkers and bone mineral density in male patients with early-stage Alzheimer’s disease. Eur. Geriatr. Med. 11, 403–408. doi: 10.1007/s41999-020-00289-z
Pyun, J. M., Ryu, J. S., Lee, R., Shim, K. H., Youn, Y. C., Ryoo, N., et al. (2021). Plasma amyloid-beta oligomerization tendency predicts amyloid PET positivity. Clin. Interv. Aging 16, 749–755. doi: 10.2147/CIA.S312473
Racine, A. M., Koscik, R. L., Nicholas, C. R., Clark, L. R., Okonkwo, O. C., Oh, J. M., et al. (2016). Cerebrospinal fluid ratios with Aβ42 predict preclinical brain β−amyloid accumulation. Alzheimers Dement. 2, 27–38. doi: 10.1016/j.dadm.2015.11.006
Rani, A., O’Shea, A., Ianov, L., Cohen, R. A., Woods, A. J., and Foster, T. C. (2017). miRNA in circulating microvesicles as biomarkers for age-related cognitive decline. Front. Aging Neurosci. 9:323. doi: 10.3389/fnagi.2017.00323
Raposo, G., Nijman, H. W., Stoorvogel, W., Liejendekker, R., Harding, C. V., Melief, C. J., et al. (1996). B lymphocytes secrete antigen-presenting vesicles. J. Exp. Med. 183, 1161–1172. doi: 10.1084/jem.183.3.1161
Rastogi, S., Sharma, V., Bharti, P. S., Rani, K., Modi, G. P., Nikolajeff, F., et al. (2021). The evolving landscape of exosomes in neurodegenerative diseases: Exosomes characteristics and a promising role in early diagnosis. Int. J. Mol. Sci. 22:440. doi: 10.3390/ijms22010440
Ratajczak, J., Miekus, K., Kucia, M., Zhang, J., Reca, R., Dvorak, P., et al. (2006). Embryonic stem cell-derived microvesicles reprogram hematopoietic progenitors: Evidence for horizontal transfer of mRNA and protein delivery. Leukemia 20, 847–856. doi: 10.1038/sj.leu.2404132
Rauchmann, B. S., Schneider-Axmann, T., and Perneczky, R., Alzheimer’s Disease Neuroimaging Initiative (2021). Associations of longitudinal plasma p-tau181 and NfL with tau-PET, Abeta-PET and cognition. J. Neurol. Neurosurg. Psychiatry 92, 1289–1295. doi: 10.1136/jnnp-2020-325537
Reale, M., Gonzales-Portillo, I., and Borlongan, C. V. (2020). Saliva, an easily accessible fluid as diagnostic tool and potent stem cell source for Alzheimer’s Disease: Present and future applications. Brain Res. 1727:146535. doi: 10.1016/j.brainres.2019.146535
Reiss, A. B., Arain, H. A., Stecker, M. M., Siegart, N. M., and Kasselman, L. J. (2018). Amyloid toxicity in Alzheimer’s disease. Rev. Neurosci. 29, 613–627. doi: 10.1515/revneuro-2017-0063
Richard, B. C., Bayer, T. A., Lind, S. B., Shevchenko, G., and Bergquist, J. (2019). A simplified and sensitive immunoprecipitation mass spectrometry protocol for the analysis of amyloid-beta peptides in brain tissue. Clin. Mass Spectrom. (14 Pt B), 83–88. doi: 10.1016/j.clinms.2019.07.001
Rohanizadegan, M., and Kulkarni, S. (2018). Transformational role of liquid biopsy in diagnosis and treatment of cancer. Cancer Genet. 228-229, 129–130. doi: 10.1016/j.cancergen.2018.11.006
Sabbagh, M. N., Shi, J., Lee, M., Arnold, L., Al-Hasan, Y., Heim, J., et al. (2018). Salivary beta amyloid protein levels are detectable and differentiate patients with Alzheimer’s disease dementia from normal controls: Preliminary findings. BMC Neurol. 18:155. doi: 10.1186/s12883-018-1160-y
Sancesario, G., and Bernardini, S. (2019). AD biomarker discovery in CSF and in alternative matrices. Clin. Biochem. 72, 52–57. doi: 10.1016/j.clinbiochem.2019.08.008
Sandua, A., Alegre, E., and Gonzalez, A. (2021). Exosomes in lung cancer: Actors and heralds of tumor development. Cancers 13:4330. doi: 10.3390/cancers13174330
Sansone, P., Savini, C., Kurelac, I., Chang, Q., Amato, L. B., Strillacci, A., et al. (2017). Packaging and transfer of mitochondrial DNA via exosomes regulate escape from dormancy in hormonal therapy-resistant breast cancer. Proc. Natl. Acad. Sci. U.S.A. 114, E9066–E9075. doi: 10.1073/pnas.1704862114
Santos Seckler, H. D., Park, H. M., Lloyd-Jones, C. M., Melani, R. D., Camarillo, J. M., Wilkins, J. T., et al. (2021). New interface for faster proteoform analysis: Immunoprecipitation coupled with samplestream-mass spectrometry. J. Am. Soc. Mass Spectrom. 32, 1659–1670. doi: 10.1021/jasms.1c00026
Sato, C., Barthelemy, N. R., Mawuenyega, K. G., Patterson, B. W., Gordon, B. A., Jockel-Balsarotti, J., et al. (2018). Tau kinetics in neurons and the human central nervous system. Neuron 97, 1284–1298.e7. doi: 10.1016/j.neuron.2018.02.015
Schepici, G., Silvestro, S., Trubiani, O., Bramanti, P., and Mazzon, E. (2020). Salivary biomarkers: Future approaches for early diagnosis of neurodegenerative diseases. Brain Sci. 10:245. doi: 10.3390/brainsci10040245
Segura, E., Nicco, C., Lombard, B., Veron, P., Raposo, G., Batteux, F., et al. (2005). ICAM-1 on exosomes from mature dendritic cells is critical for efficient naive T-cell priming. Blood 106, 216–223. doi: 10.1182/blood-2005-01-0220
Seol, W., Kim, H., and Son, I. (2020). Urinary biomarkers for neurodegenerative diseases. Exp. Neurobiol. 29, 325–333. doi: 10.5607/en20042
Shi, M., Sui, Y. T., Peskind, E. R., Li, G., Hwang, H., Devic, I., et al. (2011). Salivary tau species are potential biomarkers of Alzheimer’s disease. J. Alzheimers Dis. 27, 299–305. doi: 10.3233/JAD-2011-110731
Shioya, M., Obayashi, S., Tabunoki, H., Arima, K., Saito, Y., Ishida, T., et al. (2010). Aberrant microRNA expression in the brains of neurodegenerative diseases: miR-29a decreased in Alzheimer disease brains targets neurone navigator 3. Neuropathol. Appl. Neurobiol. 36, 320–330. doi: 10.1111/j.1365-2990.2010.01076.x
Silva, M. V. F., Loures, C. M. G., Alves, L. C. V., De Souza, L. C., Borges, K. B. G., and Carvalho, M. D. G. (2019). Alzheimer’s disease: Risk factors and potentially protective measures. J. Biomed. Sci. 26:33. doi: 10.1186/s12929-019-0524-y
Simrén, J., Leuzy, A., Karikari, T. K., Hye, A., Benedet, A. L., Lantero-Rodriguez, J., et al. (2021). The diagnostic and prognostic capabilities of plasma biomarkers in Alzheimer’s disease. Alzheimers Dement. 17, 1145–1156. doi: 10.1002/alz.12283
Skog, J., Wurdinger, T., Van Rijn, S., Meijer, D. H., Gainche, L., Sena-Esteves, M., et al. (2008). Glioblastoma microvesicles transport RNA and proteins that promote tumour growth and provide diagnostic biomarkers. Nat. Cell Biol. 10, 1470–1476. doi: 10.1038/ncb1800
Smania, M. A. (2020). Liquid biopsy for cancer screening, diagnosis, and treatment. J. Am. Assoc. Nurse Pract. 32, 5–7. doi: 10.1097/JXX.0000000000000359
Sokolova, V., Ludwig, A. K., Hornung, S., Rotan, O., Horn, P. A., Epple, M., et al. (2011). Characterisation of exosomes derived from human cells by nanoparticle tracking analysis and scanning electron microscopy. Colloids Surf. B Biointerfaces 87, 146–150. doi: 10.1016/j.colsurfb.2011.05.013
Song, Z., Xu, Y., Zhang, L., Zhou, L., Zhang, Y., Han, Y., et al. (2020). Comprehensive proteomic profiling of urinary exosomes and identification of potential non-invasive early biomarkers of Alzheimer’s disease in 5XFAD mouse model. Front. Genet. 11:565479. doi: 10.3389/fgene.2020.565479
Sorensen, S. S., Nygaard, A. B., and Christensen, T. (2016). miRNA expression profiles in cerebrospinal fluid and blood of patients with Alzheimer’s disease and other types of dementia - an exploratory study. Transl. Neurodegener. 5:6. doi: 10.1186/s40035-016-0053-5
Soria Lopez, J. A., Gonzalez, H. M., and Leger, G. C. (2019). Alzheimer’s disease. Handb. Clin. Neurol. 167, 231–255. doi: 10.1016/B978-0-12-804766-8.00013-3
Stomrud, E., Hansson, O., Blennow, K., Minthon, L., Londos, E. J. D., and Disorders, G. C. (2007). Cerebrospinal fluid biomarkers predict decline in subjective cognitive function over 3 years in healthy elderly. Dement. Geriatr. Cogn. Disord. 24, 118–124. doi: 10.1159/000105017
Strozyk, D., Blennow, K., White, L., and Launer, L. J. N. (2003). CSF Aβ 42 levels correlate with amyloid-neuropathology in a population-based autopsy study. Neurology 60, 652–656. doi: 10.1212/01.WNL.0000046581.81650.D0
Suarez-Calvet, M., Karikari, T. K., Ashton, N. J., Lantero Rodriguez, J., Mila-Aloma, M., Gispert, J. D., et al. (2020). Novel tau biomarkers phosphorylated at T181, T217 or T231 rise in the initial stages of the preclinical Alzheimer’s continuum when only subtle changes in Abeta pathology are detected. EMBO Mol. Med. 12:e12921.
Suarez-Calvet, M., Kleinberger, G., Araque Caballero, M. A., Brendel, M., Rominger, A., Alcolea, D., et al. (2016). sTREM2 cerebrospinal fluid levels are a potential biomarker for microglia activity in early-stage Alzheimer’s disease and associate with neuronal injury markers. EMBO Mol. Med. 8, 466–476. doi: 10.15252/emmm.201506123
Sun, R., Wang, H., Shi, Y., Gao, D., Sun, Z., Chen, Z., et al. (2019). A pilot study of urinary exosomes in Alzheimer’s disease. Neurodegener. Dis. 19, 184–191. doi: 10.1159/000505851
Suzuki, N., Iwatsubo, T., Odaka, A., Ishibashi, Y., Kitada, C., and Ihara, Y. (1994). High tissue content of soluble beta 1-40 is linked to cerebral amyloid angiopathy. Am. J. Pathol. 145, 452–460.
Tan, C. C., Yu, J. T., and Tan, L. (2014). Biomarkers for preclinical Alzheimer’s disease. J. Alzheimers Dis. 42, 1051–1069. doi: 10.3233/JAD-140843
Tapiola, T., Alafuzoff, I., Herukka, S.-K., Parkkinen, L., Hartikainen, P., Soininen, H., et al. (2009). Cerebrospinal fluid β-amyloid 42 and tau proteins as biomarkers of Alzheimer-type pathologic changes in the brain. Arch. Neurol. 66, 382–389. doi: 10.1001/archneurol.2008.596
Tariciotti, L., Casadei, M., Honig, L. S., Teich, A. F., Mckhann, Ii, G. M., et al. (2018). Clinical experience with cerebrospinal fluid Abeta42, total and phosphorylated Tau in the evaluation of 1,016 individuals for suspected dementia. J. Alzheimers Dis. 65, 1417–1425. doi: 10.3233/JAD-180548
Thakur, B. K., Zhang, H., Becker, A., Matei, I., Huang, Y., Costa-Silva, B., et al. (2014). Double-stranded DNA in exosomes: A novel biomarker in cancer detection. Cell Res. 24, 766–769. doi: 10.1038/cr.2014.44
Thambisetty, M., and Lovestone, S. J. B. I. M. (2010). Blood-based biomarkers of Alzheimer’s disease: Challenging but feasible. Biomark. Med. 4, 65–79. doi: 10.2217/bmm.09.84
Thebault, S., Booth, R. A., and Freedman, M. S. (2020). Blood neurofilament light chain: The Neurologist’s troponin? Biomedicines 8:523. doi: 10.3390/biomedicines8110523
Thijssen, E. H., La Joie, R., Wolf, A., Strom, A., Wang, P., Iaccarino, L., et al. (2020). Diagnostic value of plasma phosphorylated tau181 in Alzheimer’s disease and frontotemporal lobar degeneration. Nat. Med. 26, 387–397. doi: 10.1038/s41591-020-0762-2
Tiwari, S., Atluri, V., Kaushik, A., Yndart, A., and Nair, M. (2019). Alzheimer’s disease: Pathogenesis, diagnostics, and therapeutics. Int. J. Nanomedicine 14, 5541–5554. doi: 10.2147/IJN.S200490
Trams, E. G., Lauter, C. J., Salem, N. Jr., and Heine, U. (1981). Exfoliation of membrane ecto-enzymes in the form of micro-vesicles. Biochim. Biophys. Acta 645, 63–70. doi: 10.1016/0005-2736(81)90512-5
Tvarijonaviciute, A., Zamora, C., Ceron, J. J., Bravo-Cantero, A. F., Pardo-Marin, L., Valverde, S., et al. (2020). Salivary biomarkers in Alzheimer’s disease. Clin. Oral Investig. 24, 3437–3444. doi: 10.1007/s00784-020-03214-7
Uljon, S. N., Mazzarelli, L., Chait, B. T., and Wang, R. (2000). Analysis of proteins and peptides directly from biological fluids by immunoprecipitation/mass spectrometry. Methods Mol. Biol. 146, 439–452. doi: 10.1385/1-59259-045-4:439
Underwood, J. J., Quadri, R. S., Kalva, S. P., Shah, H., Sanjeevaiah, A. R., Beg, M. S., et al. (2020). Liquid biopsy for cancer: Review and implications for the radiologist. Radiology 294, 5–17. doi: 10.1148/radiol.2019182584
Vaidyanathan, R., Soon, R. H., Zhang, P., Jiang, K., and Lim, C. T. (2018). Cancer diagnosis: From tumor to liquid biopsy and beyond. Lab Chip 19, 11–34. doi: 10.1039/C8LC00684A
Valadi, H., Ekstrom, K., Bossios, A., Sjostrand, M., Lee, J. J., and Lotvall, J. O. (2007). Exosome-mediated transfer of mRNAs and microRNAs is a novel mechanism of genetic exchange between cells. Nat. Cell Biol. 9, 654–659. doi: 10.1038/ncb1596
van der Pol, E., Coumans, F. A., Grootemaat, A. E., Gardiner, C., Sargent, I. L., Harrison, P., et al. (2014). Particle size distribution of exosomes and microvesicles determined by transmission electron microscopy, flow cytometry, nanoparticle tracking analysis, and resistive pulse sensing. J. Thromb. Haemost. 12, 1182–1192. doi: 10.1111/jth.12602
van Oijen, M., Hofman, A., Soares, H. D., Koudstaal, P. J., and Breteler, M. M. (2006). Plasma Aβ1–40 and Aβ1–42 and the risk of dementia: A prospective case-cohort study. Lancet Neurol. 5, 655–660. doi: 10.1016/S1474-4422(06)70501-4
Vandendriessche, C., Bruggeman, A., Van Cauwenberghe, C., and Vandenbroucke, R. E. (2020). Extracellular vesicles in Alzheimer’s and Parkinson’s disease: Small entities with large consequences. Cells 9:2485. doi: 10.3390/cells9112485
Vandermeeren, M., Mercken, M., Vanmechelen, E., Six, J., Van De Voorde, A., Martin, J. J., et al. (1993). Detection of proteins in normal and Alzheimer’s disease cerebrospinal fluid with a sensitive sandwich enzyme-linked immunosorbent assay. J. Neurochem. 61, 1828–1834. doi: 10.1111/j.1471-4159.1993.tb09823.x
Vanmechelen, E., Vanderstichele, H., Davidsson, P., Van Kerschaver, E., Van Der Perre, B., Sjögren, M., et al. (2000). Quantification of tau phosphorylated at threonine 181 in human cerebrospinal fluid: A sandwich ELISA with a synthetic phosphopeptide for standardization. Neurosci. Lett. 285, 49–52. doi: 10.1016/S0304-3940(00)01036-3
Veerabhadrappa, B., Delaby, C., Hirtz, C., Vialaret, J., Alcolea, D., Lleo, A., et al. (2020). Detection of amyloid beta peptides in body fluids for the diagnosis of Alzheimer’s disease: Where do we stand? Crit. Rev. Clin. Lab. Sci. 57, 99–113. doi: 10.1080/10408363.2019.1678011
Wang, M. J., Yi, S., Han, J. Y., Park, S. Y., Jang, J. W., Chun, I. K., et al. (2017). Oligomeric forms of amyloid-beta protein in plasma as a potential blood-based biomarker for Alzheimer’s disease. Alzheimers Res. Ther. 9:98. doi: 10.1186/s13195-017-0324-0
Wang, N., Chen, J., Xiao, H., Wu, L., Jiang, H., and Zhou, Y. (2019). Application of artificial neural network model in diagnosis of Alzheimer’s disease. BMC Neurol. 19:154. doi: 10.1186/s12883-019-1377-4
Wang, Y., Pan, F., Xie, F., He, R., and Guo, Q. (2022). Correlation between urine formaldehyde and cognitive abilities in the clinical spectrum of Alzheimer’s disease. Front. Aging Neurosci. 14:820385. doi: 10.3389/fnagi.2022.820385
Watanabe, Y., Hirao, Y., Kasuga, K., Tokutake, T., Kitamura, K., Niida, S., et al. (2020). Urinary apolipoprotein C3 is a potential biomarker for Alzheimer’s disease. Dement. Geriatr. Cogn. Dis. Extra 10, 94–104. doi: 10.1159/000509561
Wei, H., Xu, Y., Xu, W., Zhou, Q., Chen, Q., Yang, M., et al. (2018). Serum Exosomal miR-223 Serves as a potential diagnostic and prognostic biomarker for dementia. Neuroscience 379, 167–176. doi: 10.1016/j.neuroscience.2018.03.016
Weller, J., and Budson, A. (2018). Current understanding of Alzheimer’s disease diagnosis and treatment. F1000Res. 7:F1000 Faculty Rev-1161. doi: 10.12688/f1000research.14506.1
Wildburger, N. C., Gyngard, F., Guillermier, C., Patterson, B. W., Elbert, D., Mawuenyega, K. G., et al. (2018). Amyloid-beta plaques in clinical Alzheimer’s disease brain incorporate stable isotope tracer in vivo and exhibit nanoscale heterogeneity. Front. Neurol. 9:169. doi: 10.3389/fneur.2018.00169
Winston, C. N., Goetzl, E. J., Akers, J. C., Carter, B. S., Rockenstein, E. M., Galasko, D., et al. (2016). Prediction of conversion from mild cognitive impairment to dementia with neuronally derived blood exosome protein profile. Alzheimers Dement. 3, 63–72. doi: 10.1016/j.dadm.2016.04.001
Winston, C. N., Goetzl, E. J., Schwartz, J. B., Elahi, F. M., and Rissman, R. A. (2019). Complement protein levels in plasma astrocyte-derived exosomes are abnormal in conversion from mild cognitive impairment to Alzheimer’s disease dementia. Alzheimers Dement. 11, 61–66. doi: 10.1016/j.dadm.2018.11.002
Wolozin, B., and Davies, P. J. A. O. N. (1987). Alzheimer-related neuronal protein A68: Specificity and distribution. Ann. Neurol. 22, 521–526.
Wu, L., and Qu, X. (2015). Cancer biomarker detection: Recent achievements and challenges. Chem. Soc. Rev. 44, 2963–2997. doi: 10.1039/C4CS00370E
Wu, Y., Xu, J., Xu, J., Cheng, J., Jiao, D., Zhou, C., et al. (2017). Lower serum levels of miR-29c-3p and miR-19b-3p as biomarkers for Alzheimer’s disease. Tohoku J. Exp. Med. 242, 129–136. doi: 10.1620/tjem.242.129
Xu, K., Zhang, C., Du, T., Gabriel, A. N. A., Wang, X., Li, X., et al. (2021). Progress of exosomes in the diagnosis and treatment of lung cancer. Biomed. Pharmacother. 134:111111. doi: 10.1016/j.biopha.2020.111111
Xu, Y., Shen, Y. Y., Zhang, X. P., Gui, L., Cai, M., Peng, G. P., et al. (2020). Diagnostic potential of urinary monocyte chemoattractant protein-1 for Alzheimer’s disease and amnestic mild cognitive impairment. Eur. J. Neurol. 27, 1429–1435. doi: 10.1111/ene.14254
Yamashita, K., Watanabe, S., Ishiki, K., Miura, M., Irino, Y., Kubo, T., et al. (2021). Fully automated chemiluminescence enzyme immunoassays showing high correlation with immunoprecipitation mass spectrometry assays for β-amyloid (1–40) and (1–42) in plasma samples. Biochem. Biophys. Res. Commun. 576, 22–26. doi: 10.1016/j.bbrc.2021.08.066
Yan, Z., Zhou, Z., Wu, Q., Chen, Z. B., Koo, E. H., and Zhong, S. (2020). Presymptomatic increase of an extracellular RNA in blood plasma associates with the development of Alzheimer’s disease. Curr. Biol. 30, 1771–1782.e3. doi: 10.1016/j.cub.2020.02.084
Yang, S. Y., Chiu, M. J., Chen, T. F., and Horng, H. E. (2017). Detection of plasma biomarkers using immunomagnetic reduction: A promising method for the early diagnosis of Alzheimer’s disease. Neurol. Ther. 6, 37–56. doi: 10.1007/s40120-017-0075-7
Yang, S. Y., Liu, H. C., and Chen, W. P. (2020). Immunomagnetic reduction detects plasma Abeta1-42 levels as a potential dominant indicator predicting cognitive decline. Neurol. Ther. 9, 435–442. doi: 10.1007/s40120-020-00215-2
Yang, T. T., Liu, C. G., Gao, S. C., Zhang, Y., and Wang, P. C. (2018). The serum exosome derived microRNA-135a, -193b, and -384 Were potential Alzheimer’s disease biomarkers. Biomed. Environ. Sci. 31, 87–96.
Yang, Z., and Wang, K. K. (2015). Glial fibrillary acidic protein: From intermediate filament assembly and gliosis to neurobiomarker. Trends Neurosci. 38, 364–374. doi: 10.1016/j.tins.2015.04.003
Yao, F., Hong, X., Li, S., Zhang, Y., Zhao, Q., Du, W., et al. (2018). Urine-based biomarkers for Alzheimer’s disease identified through coupling computational and experimental methods. J. Alzheimers Dis. 65, 421–431. doi: 10.3233/JAD-180261
Yin, Q., Ji, X., Lv, R., Pei, J. J., Du, Y., Shen, C., et al. (2020). Targetting exosomes as a new biomarker and therapeutic approach for Alzheimer’s disease. Clin. Interv. Aging 15, 195–205. doi: 10.2147/CIA.S240400
Yoo, Y. K., Lee, J., Kim, H., Hwang, K. S., Yoon, D. S., and Lee, J. H. (2018). Toward exosome-based neuronal diagnostic devices. Micromachines 9:634. doi: 10.3390/mi9120634
Youn, Y. C., Kang, S., Suh, J., Park, Y. H., Kang, M. J., Pyun, J. M., et al. (2019). Blood amyloid-beta oligomerization associated with neurodegeneration of Alzheimer’s disease. Alzheimers Res. Ther. 11:40. doi: 10.1186/s13195-019-0499-7
Zetterberg, H., and Blennow, K. (2021). Moving fluid biomarkers for Alzheimer’s disease from research tools to routine clinical diagnostics. Mol. Neurodegener. 16:10. doi: 10.1186/s13024-021-00430-x
Zetterberg, H., Hietala, M. A., Jonsson, M., Andreasen, N., Styrud, E., Karlsson, I., et al. (2006). Neurochemical aftermath of amateur boxing. Arch. Neurol. 63, 1277–1280. doi: 10.1001/archneur.63.9.1277
Zetterberg, H., Skillback, T., Mattsson, N., Trojanowski, J. Q., Portelius, E., Shaw, L. M., et al. (2016). Association of cerebrospinal fluid neurofilament light concentration with Alzheimer disease progression. JAMA Neurol. 73, 60–67. doi: 10.1001/jamaneurol.2015.3037
Zhang, A. H., Sharma, G., Undheim, E. A., Jia, X., and Mobli, M. J. N. L. (2018). A complicated complex: Ion channels, voltage sensing, cell membranes and peptide inhibitors. Neurosci. Lett. 679, 35–47. doi: 10.1016/j.neulet.2018.04.030
Zhang, Y., Li, Q., Liu, C., Gao, S., Ping, H., Wang, J., et al. (2016). MiR-214-3p attenuates cognition defects via the inhibition of autophagy in SAMP8 mouse model of sporadic Alzheimer’s disease. Neurotoxicology 56, 139–149. doi: 10.1016/j.neuro.2016.07.004
Zhang, Y., Lu, C., and Zhang, J. (2021). Lactoferrin and its detection methods: A review. Nutrients 13:2492. doi: 10.3390/nu13082492
Zhou, Y., Zhang, Y., Gong, H., Luo, S., and Cui, Y. (2021). The role of exosomes and their applications in cancer. Int. J. Mol. Sci. 22:12204. doi: 10.3390/ijms222212204
Zhuang, J., Cai, P., Chen, Z., Yang, Q., Chen, X., Wang, X., et al. (2020). Long noncoding RNA MALAT1 and its target microRNA-125b are potential biomarkers for Alzheimer’s disease management via interactions with FOXQ1, PTGS2 and CDK5. Am. J. Transl. Res. 12, 5940–5954.
Keywords: Alzheimer’s disease, liquid biopsy, blood, CSF, biomarkers
Citation: Gong X, Zhang H, Liu X, Liu Y, Liu J, Fapohunda FO, Lü P, Wang K and Tang M (2022) Is liquid biopsy mature enough for the diagnosis of Alzheimer’s disease? Front. Aging Neurosci. 14:977999. doi: 10.3389/fnagi.2022.977999
Received: 25 June 2022; Accepted: 18 July 2022;
Published: 05 August 2022.
Edited by:
Jun Xu, Capital Medical University, ChinaReviewed by:
Weinan Zhou, University of Illinois at Urbana-Champaign, United StatesFeng Wang, Emory University, United States
Copyright © 2022 Gong, Zhang, Liu, Liu, Liu, Fapohunda, Lü, Wang and Tang. This is an open-access article distributed under the terms of the Creative Commons Attribution License (CC BY). The use, distribution or reproduction in other forums is permitted, provided the original author(s) and the copyright owner(s) are credited and that the original publication in this journal is cited, in accordance with accepted academic practice. No use, distribution or reproduction is permitted which does not comply with these terms.
*Correspondence: Kun Wang, d2t0YXd4QDE2My5jb20=; Min Tang, bXQzMTM4QHVqcy5lZHUuY24=