- 1Department of Neurology, Cognitive Disorders Center, Huadong Hospital Affiliated to Fudan University, Shanghai, China
- 2Department of Endocrinology, Huadong Hospital Affiliated to Fudan University, Shanghai, China
Background: Vascular dementia (VaD) and carotid atherosclerotic plaques are common in the elderly population, conferring a heavy burden on families and society. Accumulating evidence indicates carotid atherosclerotic plaques to be a risk factor for VaD. However, the underlying mechanisms for this association are mainly unknown.
Materials and methods: We analyzed temporal cortex gene expression data of the GSE122063 dataset and gene expression data of the GSE163154 dataset to identify commonly differentially expressed genes (DEGs). Then we performed functional enrichment analysis, immune cell infiltration and evaluation, correlation analysis between differentially expressed immune-related genes (DEIRGs) and immune cells, receiver operating characteristic (ROC) analysis, and drug-gene analysis.
Results: We identified 41 overlapped DEGs between the VaD and carotid atherosclerosis plaque datasets. Functional enrichment analyses revealed that these overlapped DEGs were mainly enriched in inflammatory and immune-related processes. Immunocyte infiltration and evaluation results showed that M0 macrophages, M2 macrophages, and T cells gamma delta had a dominant abundance in carotid atherosclerosis plaque samples, and M0 macrophages showed a significantly different infiltration percentage between the early and advanced stage plaques group. Resting CD4 memory T cells, M2 macrophages, and naive B cells were the top three highest infiltrating fractions in VaD. Furthermore, B cells and NK cells showed a different infiltration percentage between VaD and matched controls. We identified 12 DEIRGs, and the result of correlation analysis revealed that these DEIRGs were closely related to differentially expressed immune cells. We identified five key DEIRGs based on ROC analysis. The drug-gene interaction analysis showed that four drugs (avacopan, CCX354, BMS-817399, and ASK-8007) could be potential drugs for VaD and carotid atherosclerotic plaques treatment.
Conclusion: Collectively, these findings indicated that inflammatory and immune-related processes be a crucial common pathophysiological mechanism shared by VaD and carotid plaques. This study might provide new insights into common molecular mechanisms between VaD and carotid plaques and potential targets for the treatment.
Introduction
Vascular dementia (VaD) is not a specific nosological entity but a syndrome encompassing a number of diseases caused by insufficient blood supply to the brain. It is widely regarded as the second most common type of dementia after Alzheimer’s disease (AD), and there are no licensed treatments for it (Iadecola et al., 2019). Carotid atherosclerotic plaques is a chronic inflammatory disease and might be implicated in VaD risk. A recent study found that subclinical carotid plaque burden is related to reduced brain metabolism (Cortes-Canteli et al., 2021). A meta-analysis of three studies assessing carotid plaque showed an association between carotid plaque and impaired cognitive function (Anbar et al., 2022). However, little is known about the underlying mechanisms responsible for this association.
Previous studies showed that VaD and carotid plaque shared some common risk factors, including diabetes, cholesterol levels, smoking, and a sedentary lifestyle (Baumgart et al., 2015). Bioinformatic analysis of transcriptomes has been instrumental in investigating shared biological mechanisms of comorbid diseases. Chung and Lee (2021) analyzed blood transcriptomic signatures between AD and diabetes mellitus (DM) and revealed common dysregulated gene modules between AD and DM. Several studies conducted transcriptome analysis on VaD and found that immunity and inflammation, oxidative stress, and apoptosis were involved in VaD (Chen et al., 2022; Shu et al., 2022; Wang et al., 2022). Recent studies also found that immune and inflammation process played vital roles in the progression (Dong et al., 2017), instability (Zheng et al., 2022), and rupture (Li et al., 2022) of carotid atherosclerotic plaques based on bioinformatic analysis. However, to our knowledge, few studies have compared transcriptome signatures between VaD and carotid atherosclerotic plaques. Therefore, our study aimed to analyze transcriptome signatures between VaD and carotid atherosclerotic plaques to shed light on the underlying pathology of cognitive decline associated with carotid atherosclerotic plaques and provide new candidate targets for therapeutic intervention.
We analyzed the two datasets GSE122063 and GSE163154 to identify commonly differentially expressed genes (DEGs). Then we performed functional enrichment analysis, immunocyte infiltration and evaluation, receiver operating characteristic (ROC) analysis, and drug-gene analysis to identify underlying common pathophysiological mechanisms and potential targets for the treatment.
Materials and methods
Data acquisition and descriptions
The gene expression profiles of GSE122063, GSE163154, and GSE28829 were downloaded from the Gene Expression Omnibus (GEO)1 (Barrett et al., 2013). The dataset GSE122063 contains gene expression profiles of the frontal cortex and temporal cortex samples from 18 VaD and 22 matched controls. The GSE163154 dataset includes 16 no-intraplaque hemorrhage (the early-stage) samples and 27 intraplaque hemorrhage (the advanced-stage) samples from symptomatic patients undergoing carotid endarterectomy surgery. The GSE28829 dataset consists of 16 clinically proven advanced-stage atheromatous carotid plaques and 13 early-stage atheromatous carotid plaques. These three datasets were selected because they all contained both case groups and matched control groups, and the number of case samples and control group samples were both greater than 10, and the update time of these datasets was after 2019. In the current study, the gene expression data of the temporal cortex from VaD and matched control patients in the GSE122063 dataset and the GSE163154 dataset were designated as the exploring datasets. The gene expression data of the frontal cortex in the GSE122063 and the GSE28829 dataset were used as the validating datasets.
Identification of differentially expressed genes
Differentially expressed genes (DEG) of the microarray dataset GSE122063 and GSE163154 were identified with NCBI’s GEO2R tool2 using the Limma package. A Venn diagram (Bardou et al., 2014) was used to identify the overlapping DEGs between the GSE122063 dataset and the GSE163154 dataset.
Gene ontology and pathway enrichment analysis
Gene Ontology (GO) and pathway enrichment analysis were performed to obtain a better understanding of the biological mechanisms of the overlapping genes using Metascape3 (Zhou et al., 2019). The Kyoto Encyclopedia of Genes and Genomes (KEGG) (Kanehisa et al., 2017), Reactome (Jassal et al., 2020), and WikiPathways (Martens et al., 2021) databases were used for pathway annotations.
Immune cell infiltration and evaluation
The relative proportions of 22 infiltrating immune cell types were estimated using the CIBERSORT algorithm, by which the normalized gene expression matrix can be transformed into the composition of infiltrating immune cells (Chen et al., 2018). LM22 was used as a reference expression signature with 1,000 permutations. The correlation between immune cells was determined, and the R package ggplot2 visualized the results in R software. Significant immune cells were screened with the threshold Wilcoxon test at the p-value < 0.05.
Correlation analysis between differentially expressed immune-related genes and immune cells
A total of 1,793 immune-related genes (IRGs) were downloaded from ImmPort (Bhattacharya et al., 2018). The overlapping DEGs were intersected with IRGs to obtain differentially expressed immune-related genes (DEIRGs). The relationship between DEIRGs expression and relative percentages of immune cells was investigated using the Spearman method. A p-value < 0.05 was considered statistically significant.
Receiver operating characteristic analysis
To identify the potential key DEIRGs, we evaluated the diagnostic values of these DEIRGs in VaD and carotid plaques by applying “pROC” packages. The key DEIRGs were defined as the area under the curve (AUC) higher than 0.8 in both GSE122063 and GSE163154 datasets. Frontal cortex expression data of the GSE122063 and the GSE28829 dataset were used as the validating datasets.
Gene-drug interaction analysis
The key DEIRGs were imported into the Drug-Gene Interaction Database (DGIdb)4 (Freshour et al., 2021) as potential targets to search for existing drugs that could interact with them. Drugs that showed specific types of interactions with the key genes were selected. To identify related clinical trials, the selected drugs were input into the ClinicalTrials.govregistry (clinicaltrials.gov) which contains over 329,000 trials worldwide.
Results
Identification of differentially expressed genes
A schematic representation of this study is shown in Figure 1.
The GSE163154 dataset and temporal cortex gene expression data of the GSE122063 dataset were analyzed to identify DEGs, respectively. The GSE163154 dataset detected 284 upregulated and 236 downregulated genes, and temporal cortex gene expression data of the GSE122063 dataset detected 174 upregulated and 277 downregulated genes. A total of 41 overlapping DEGs were identified between the above two datasets. Among them, 34 genes were upregulated and five were downregulated in both datasets; one gene was upregulated in the GSE163154 dataset but downregulated in the GSE122063 dataset, and one gene was downregulated in the GSE163154 dataset but upregulated in the GSE122063 dataset (Figure 2 and Table 1).
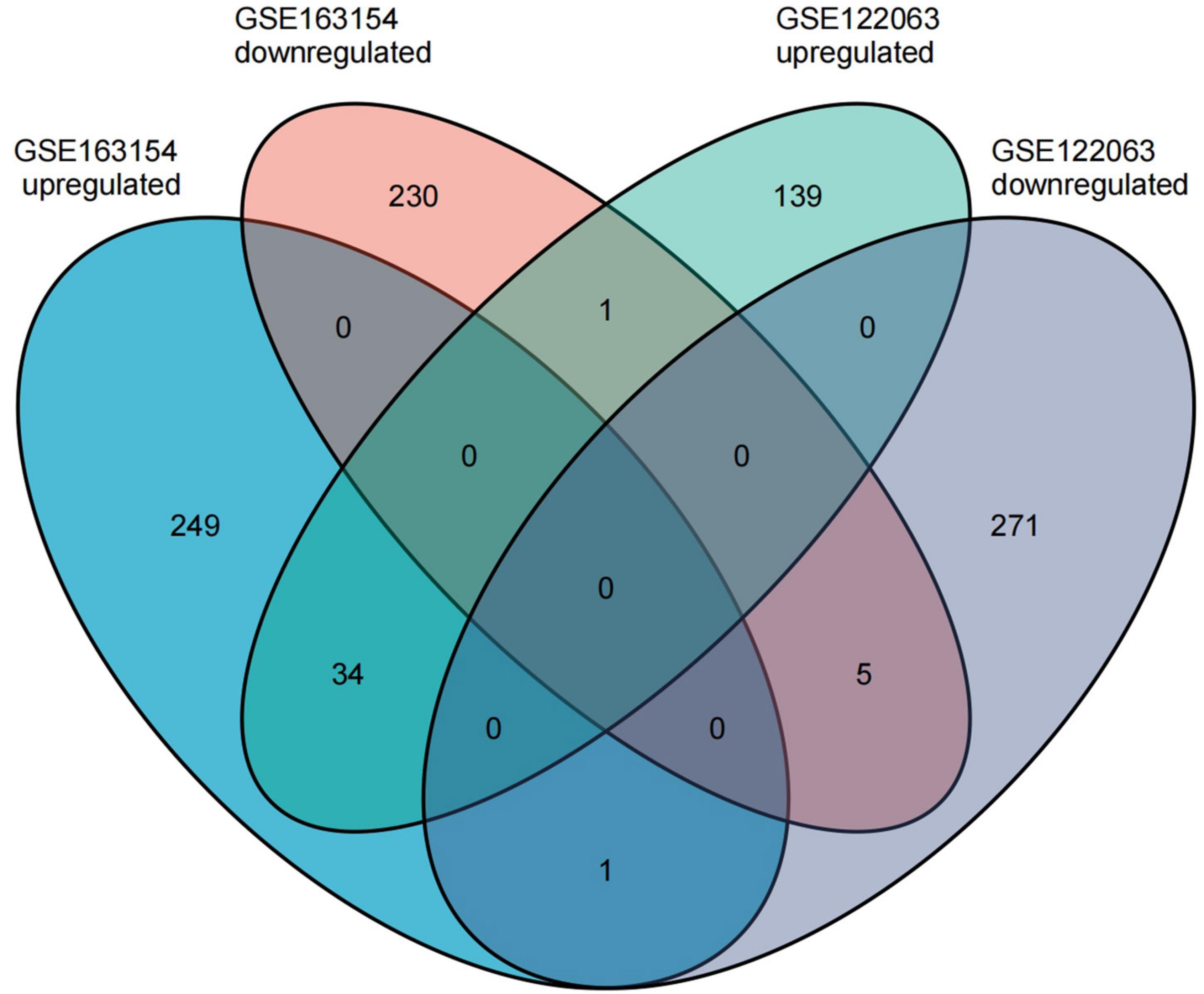
Figure 2. The common differentially expressed genes (DEGs) were identified between the two datasets.
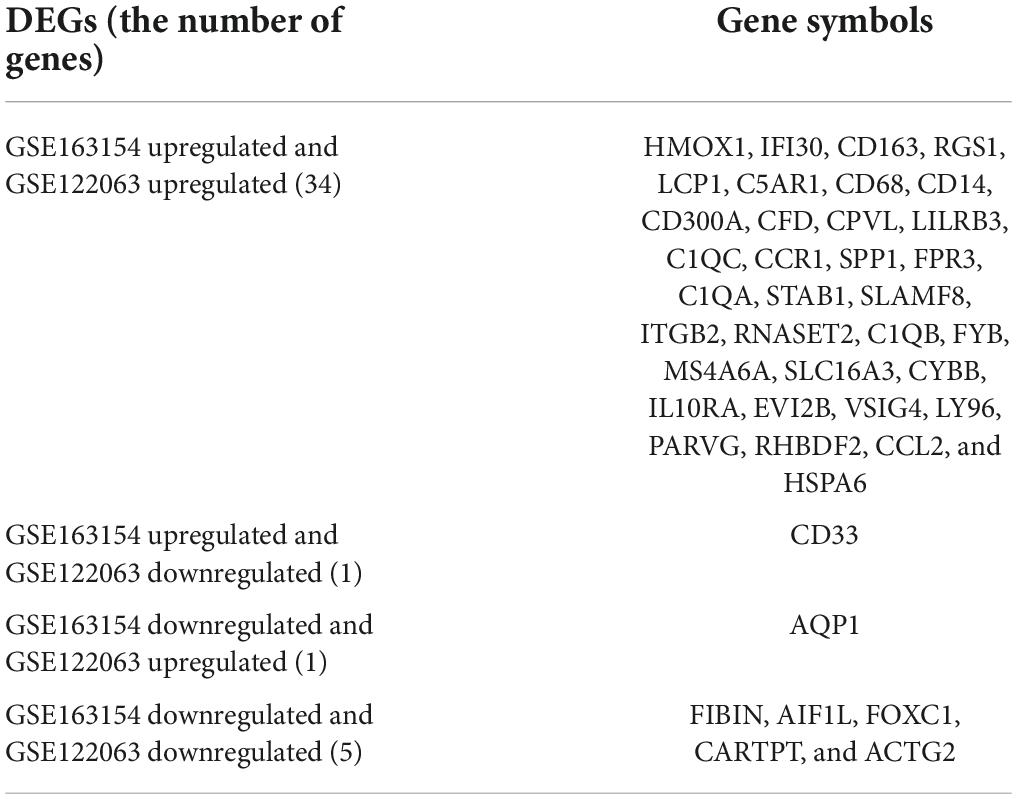
Table 1. Overlapping differentially expressed genes both in vascular dementia (VaD) and carotid atherosclerotic plaques datasets.
Gene ontology and pathway enrichment analysis
To further explore the potential functions of these overlapping genes, functional enrichment analysis was performed using the online database Metascape. The results revealed that these overlapping genes were enriched in inflammatory and immune-related processes, such as inflammatory response, negative regulation of immune system process, regulation of leukocyte migration, regulation of myeloid leukocyte differentiation, and leukocyte chemotaxis (Figure 3A and Table 2). Pathway enrichment analysis revealed that these overlapping genes were mainly involved in complement and coagulation cascades, lipid and atherosclerosis, neutrophil extracellular trap formation, and neutrophil degranulation (Figure 3B and Table 3).
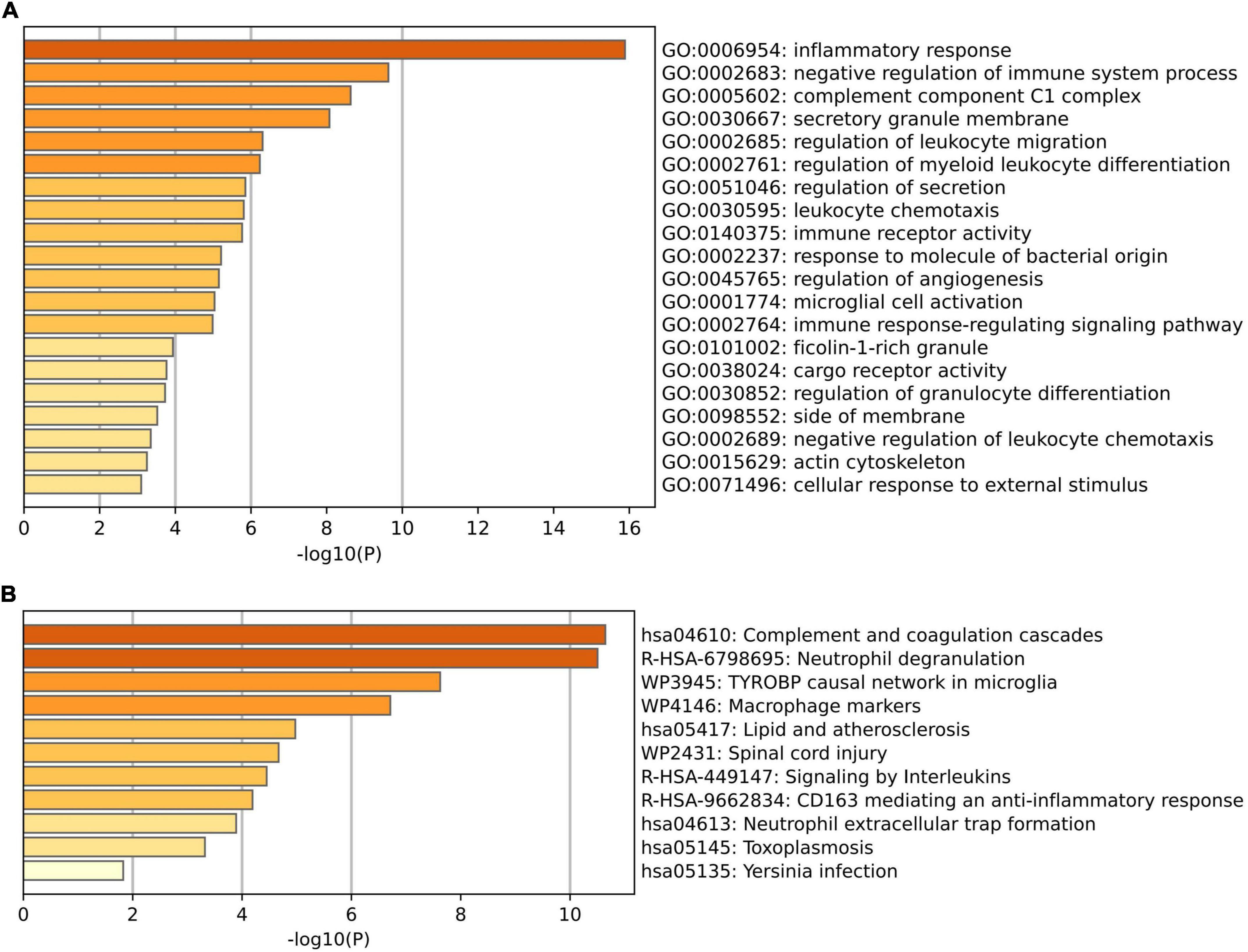
Figure 3. Functional enrichment analysis of the overlapped differentially expressed genes (DEGs) between VaD and carotid atherosclerotic plaques datasets. (A) Significant enriched GO terms of the overlapped DEGs. (B) Significantly enriched pathways of the overlapped DEGs.
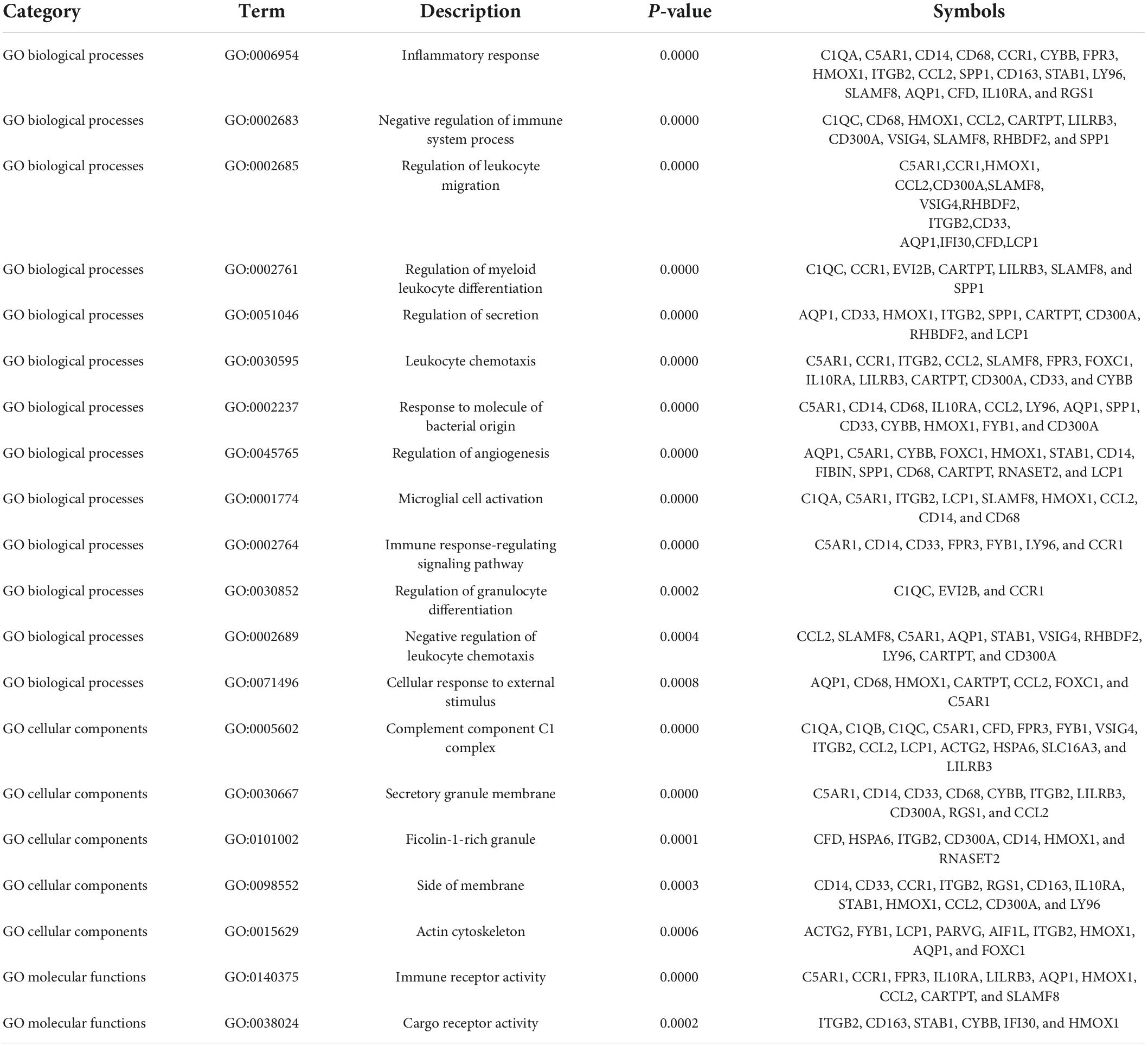
Table 2. Significantly enriched GO terms of the overlapping differentially expressed genes (DEGs) between vascular dementia (VaD) and carotid atherosclerotic plaques datasets.
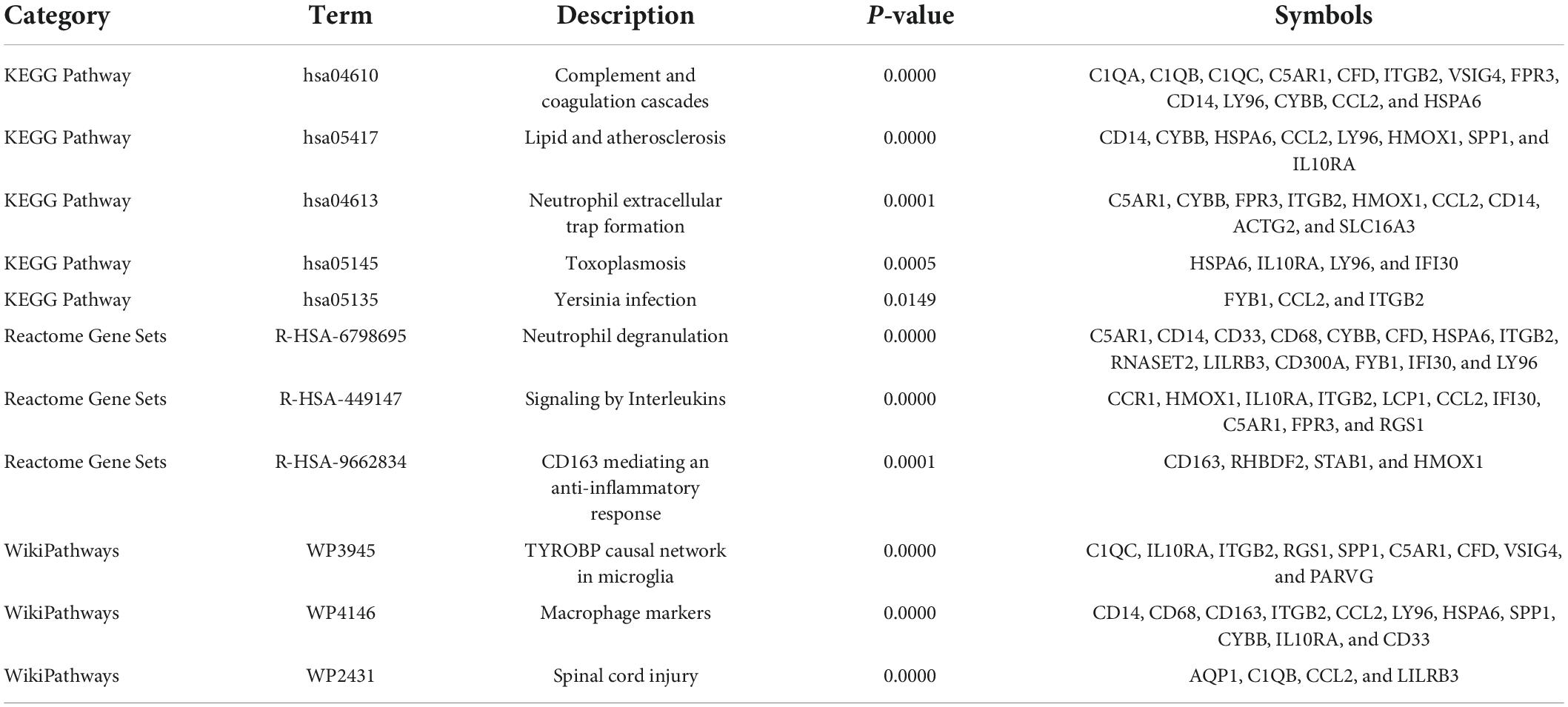
Table 3. Significantly enriched pathways of the overlapping differentially expressed genes (DEGs) between vascular dementia (VaD) and carotid atherosclerotic plaques datasets.
Immune cell infiltration and evaluation
Functional enrichment analysis indicated that inflammatory and immune processes played a vital role in the development of VaD and carotid atherosclerosis plaques. Therefore, we evaluated the infiltration of 22 immune cell types in the GSE163154 dataset and GSE122063 dataset using the “CIBERSORT” package in R software. Figure 4A showed the relative proportions of 22 immune cells types in 16 early-stage and 27 advanced-stage carotid atherosclerotic plaque samples. The top five highest infiltrating fractions in carotid plaque were M0 macrophages, M2 macrophages, resting mast cells, T cells gamma delta, and monocyte cells. Five immune cells, including M0 macrophages, T cells gamma delta, plasma cells, naive CD4 T cells, and resting CD4 memory T cells, were detected differentially infiltrated between the early-stage and advanced-stage groups (Figure 4B). Figure 5A showed the relative proportions of 22 immune cells types in temporal cortex samples from 18 VaD and 22 matched controls. The top five abundant subtypes were resting CD4 memory T cells, M2 macrophages, naive B cells, activated mast cells, and neutrophils. The percentage of B cells naive, resting NK cells, and activated dendritic cells were significantly higher in the VaD group than in the matched control group, while activated NK cells and plasma cells displayed the opposite proportion (Figure 5B).
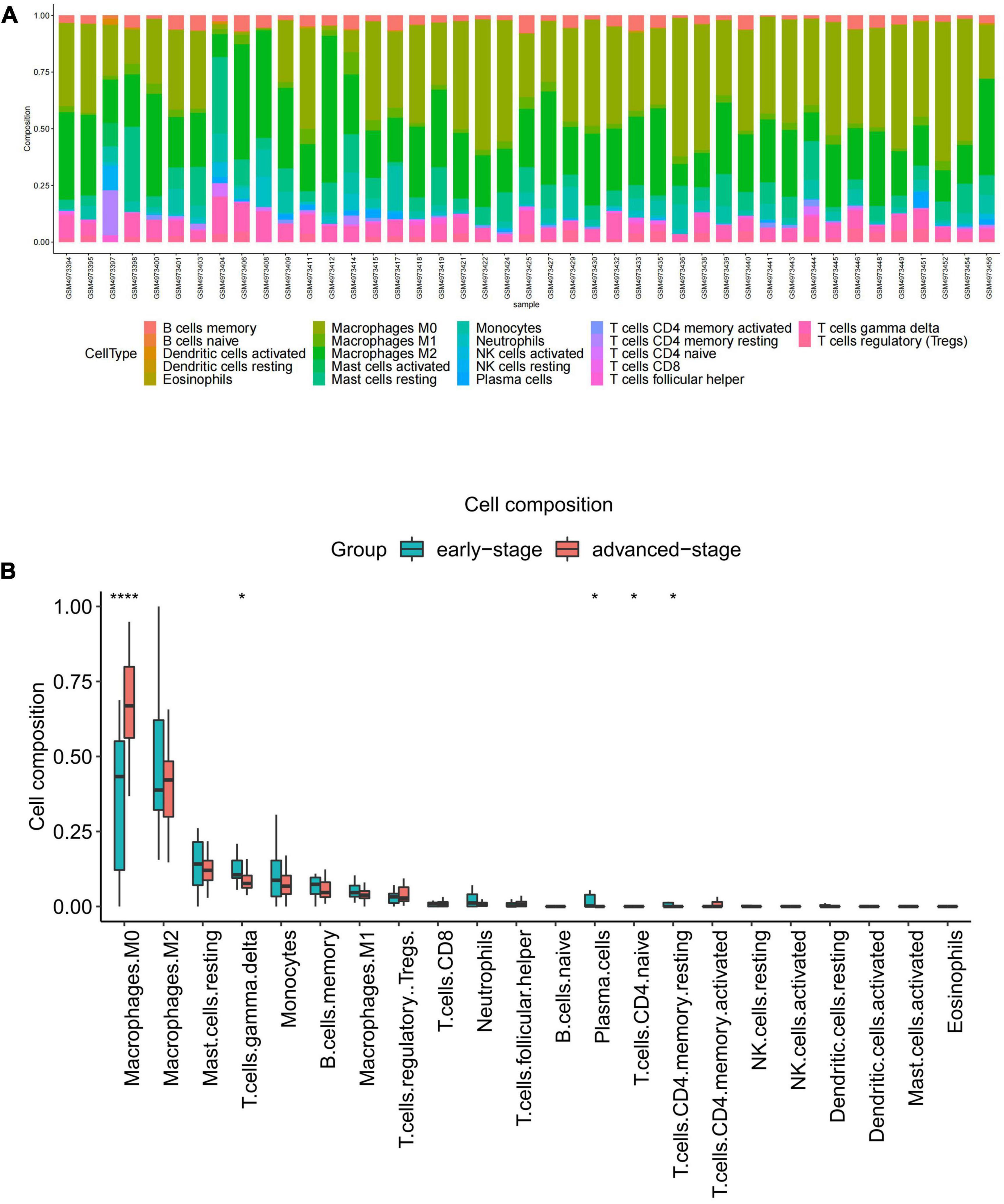
Figure 4. Immune cell Infiltration and evaluation in carotid atherosclerotic plaque samples. (A) Relative proportion of immune infiltration of 22 immune cell types in carotid atherosclerotic plaques samples. (B) The differential expression of 22 immune cells in the early-stage and advanced-stage carotid plaque samples. Significance level was denoted by *p-value < 0.05, **p-value < 0.01, ***p-value < 0.001.
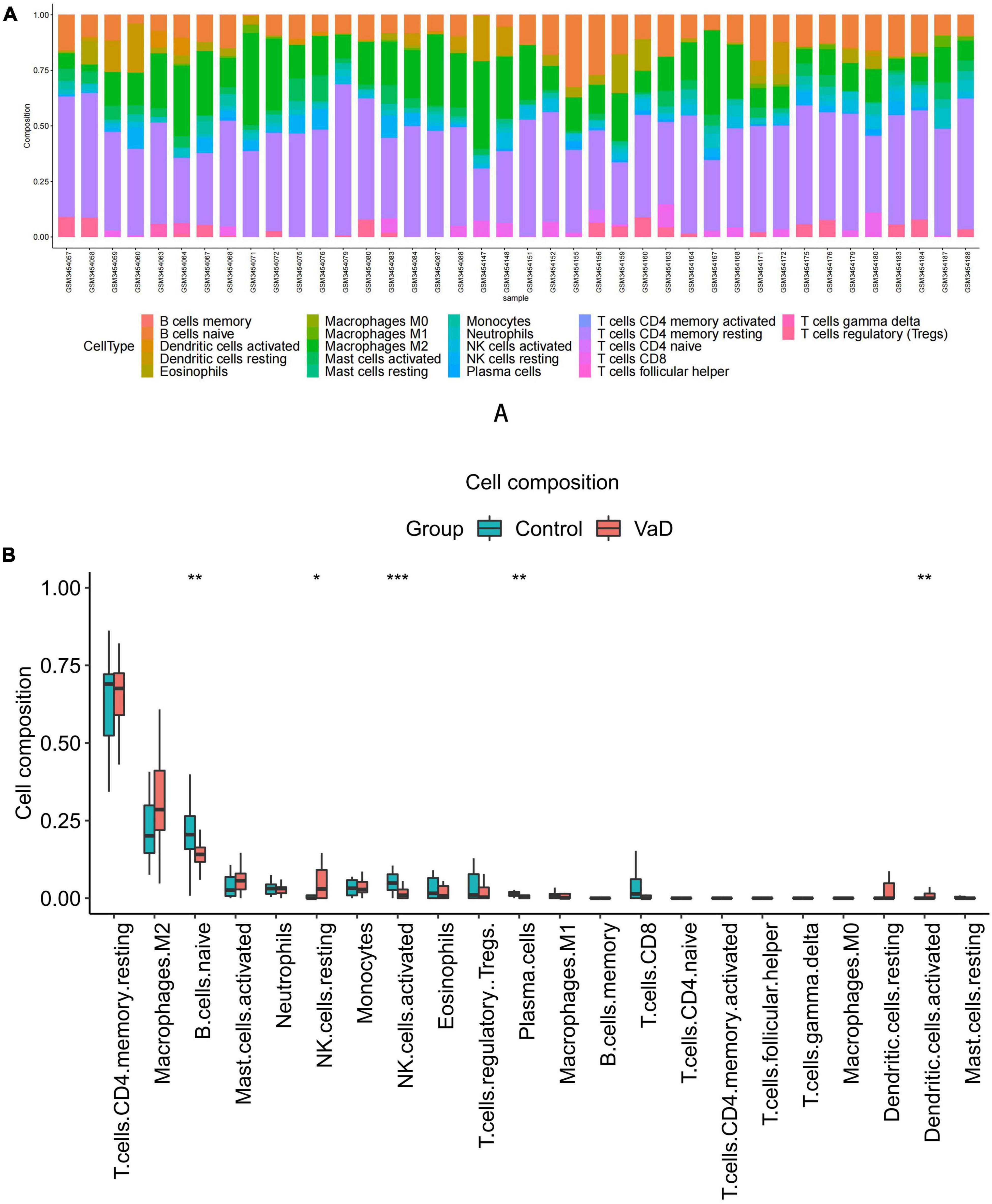
Figure 5. Immune cell Infiltration and evaluation in vascular dementia (VaD) dataset. (A) Relative proportion of immune infiltration of 22 immune cell types in VaD and matched controls samples. (B) The differential expression of 22 immune cells in the VaD and matched controls samples. Significance level was denoted by *p-value < 0.05, **p-value < 0.01, ***p-value < 0.001.
Additionally, we conducted a correlation analysis of 22 immune cells types in VaD dataset and carotid atherosclerosis dataset, and positive and negative correlations between immune cells were displayed in Figure 6. These results revealed that different kinds of immune cells interfered with each other in VaD and carotid atherosclerosis plaques.
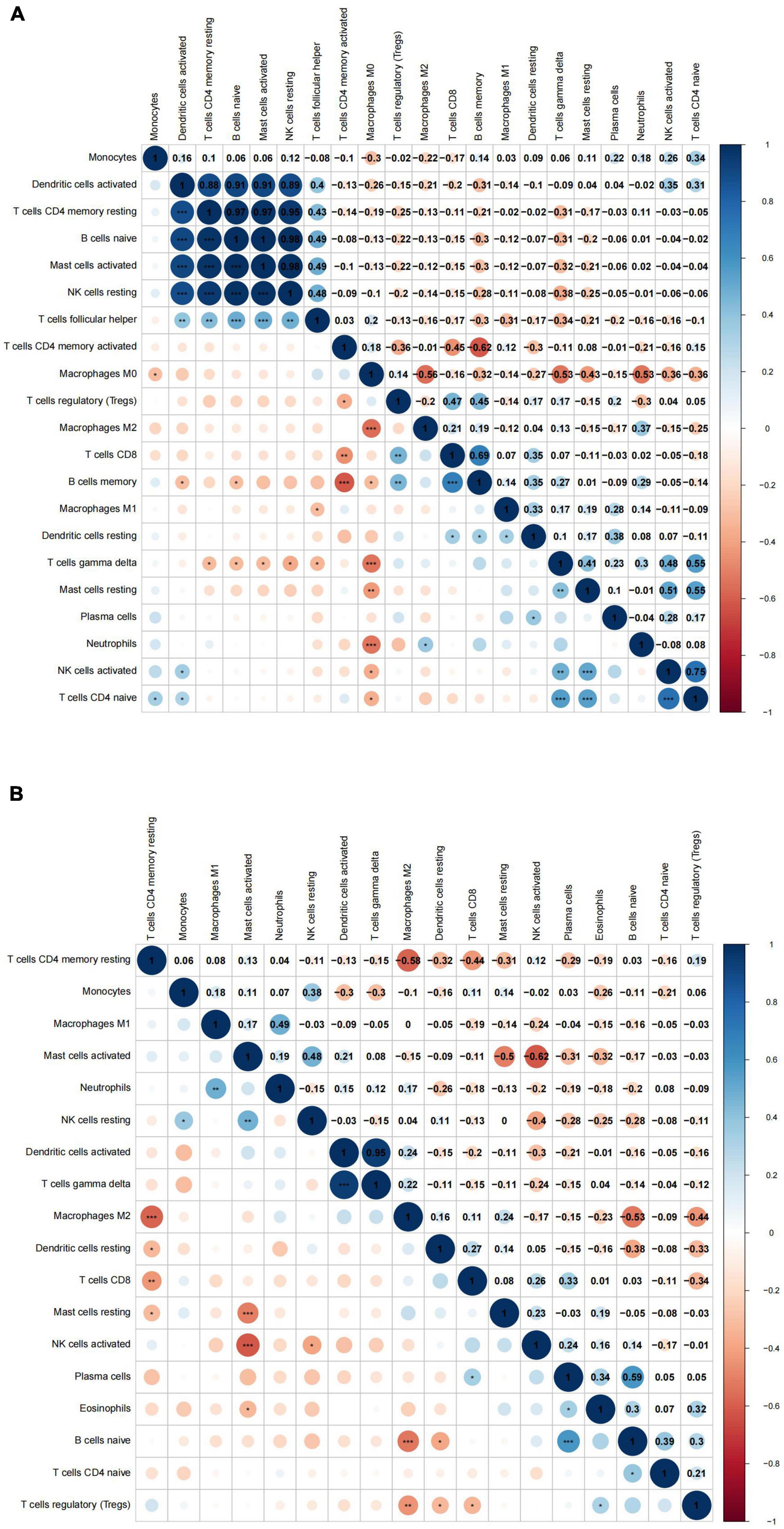
Figure 6. The correlation analysis between 22 immune cell types in the carotid atherosclerotic plaques and vascular dementia (VaD) datasets. (A) Heat map of the correlation between 22 immune cell types in the carotid atherosclerotic plaques dataset. (B) Heat map of the correlation between 22 immune cell types in the vascular dementia dataset. Significance level was denoted by *p-value < 0.05, **p-value < 0.01, ***p-value < 0.001.
Correlation analysis between differentially expressed immune-related genes and immune cells
Twelve DEIRGs were identified by intersecting these overlapping genes with IRGs. We investigated the correlation between these DEIRGs and found tight connectivity of these DEIRGs (Figures 7A,B). In addition, we investigated the correlation between these DEIRGs and differentially expressed immune cells of the corresponding groups. The results revealed that these DEIRGs were positively correlated with M0 macrophages while negatively with gamma delta T cells, resting CD4 memory T cells, plasma cells, and CD4 naive T cells in the carotid plaques group (Figure 7C). In the VaD group, these DEIRGs except the ITGB2 gene were positively correlated with resting NK cells and activated dendritic cells while negatively with naive B cells, activated NK cells, and plasma cells (Figure 7D).
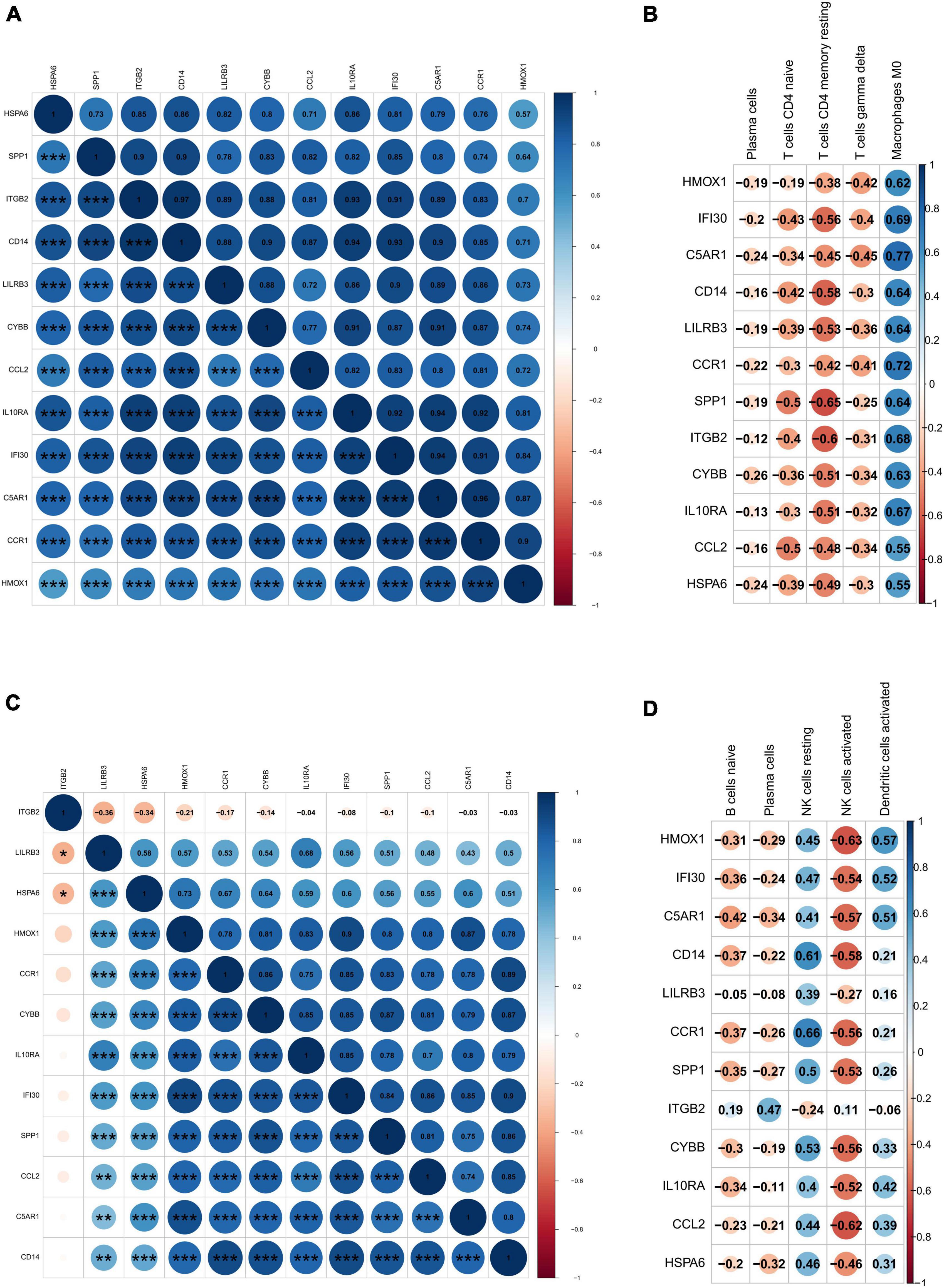
Figure 7. Correlation analysis of differentially expressed immune-related genes and immune cells. (A) Heat map of the correlation between differentially expressed immune-related genes in carotid artery plaque samples. (B) Heat map of the correlation between differentially expressed immune-related genes in vascular dementia samples. (C) Heat map of the correlation between differentially expressed immune-related genes and differentially expressed immune cells in carotid artery plaque samples. (D) Heat map of the correlation between differentially expressed immune-related genes and immune cells in vascular dementia samples. Significance level was denoted by *p-value < 0.05, **p-value < 0.01, ***p-value < 0.001.
Receiver operating characteristic analysis
To identify the key DEIRGs, we performed the ROC analysis of these DEIRGs (HMOX1, IFI30, C5AR1, CD14, LILRB3, CCR1, SPP1, ITGB2, CYBB, IL10RA, CCL2, and HSPA6) in the VaD (Figure 8) and carotid plaques samples (Figure 9). The results showed that the five genes, including HMOX1, C5AR1, CCR1, SPP1, and CYBB showed high accuracy in both groups (AUC > 0.80). To further verify the accuracy of these five genes, ROC analysis was performed in the GSE28829 dataset and frontal cortex of the GSE122063 datasets. These five genes showed strong accuracy in identifying vascular dementia (Figures 10A,B) and carotid plaque progression (Figures 10C,D), which indicated the involvement of these five key genes in both carotid plaques and VaD.
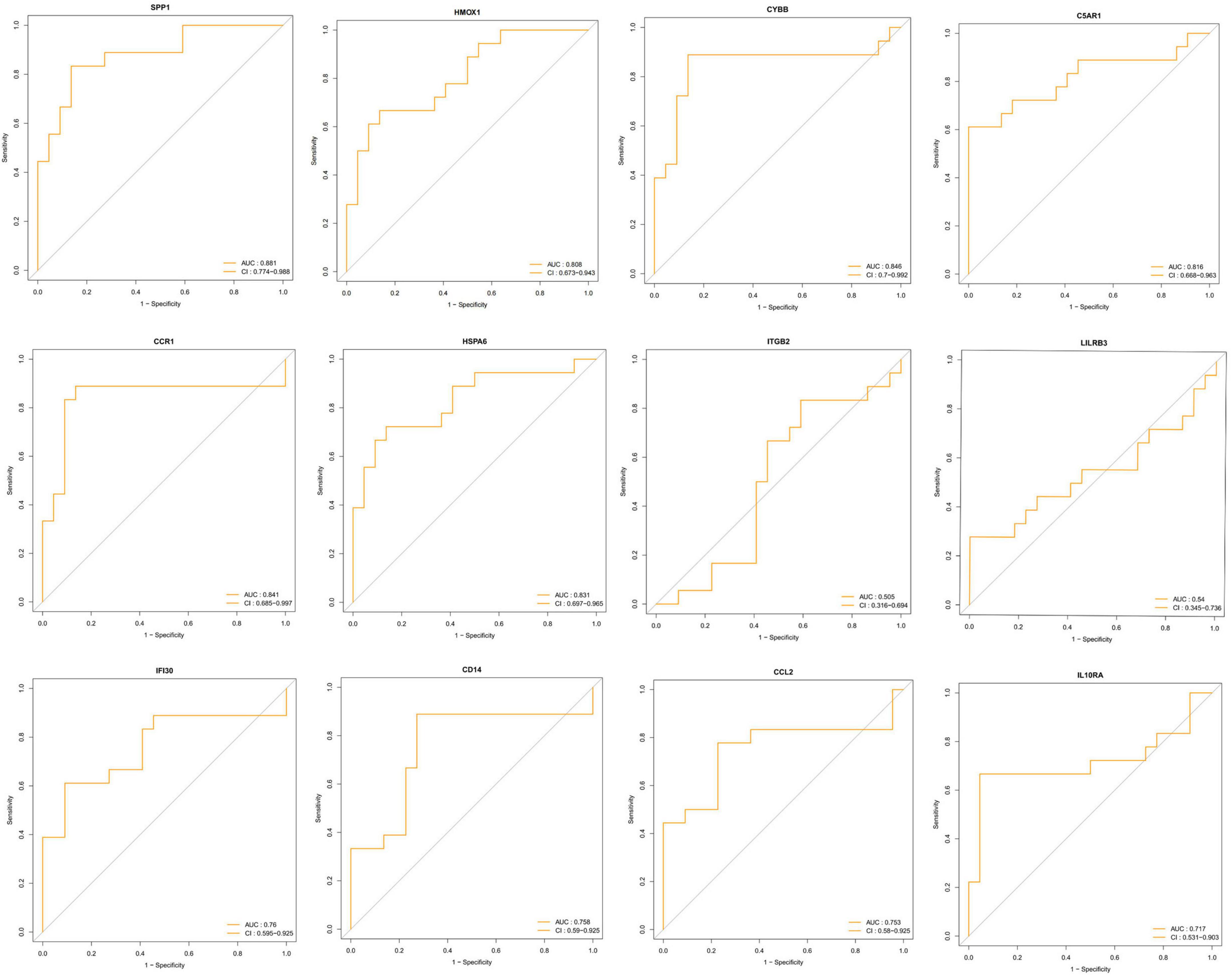
Figure 8. Receiver operating characteristic (ROC) analysis of differentially expressed immune-related genes in the vascular dementia group.
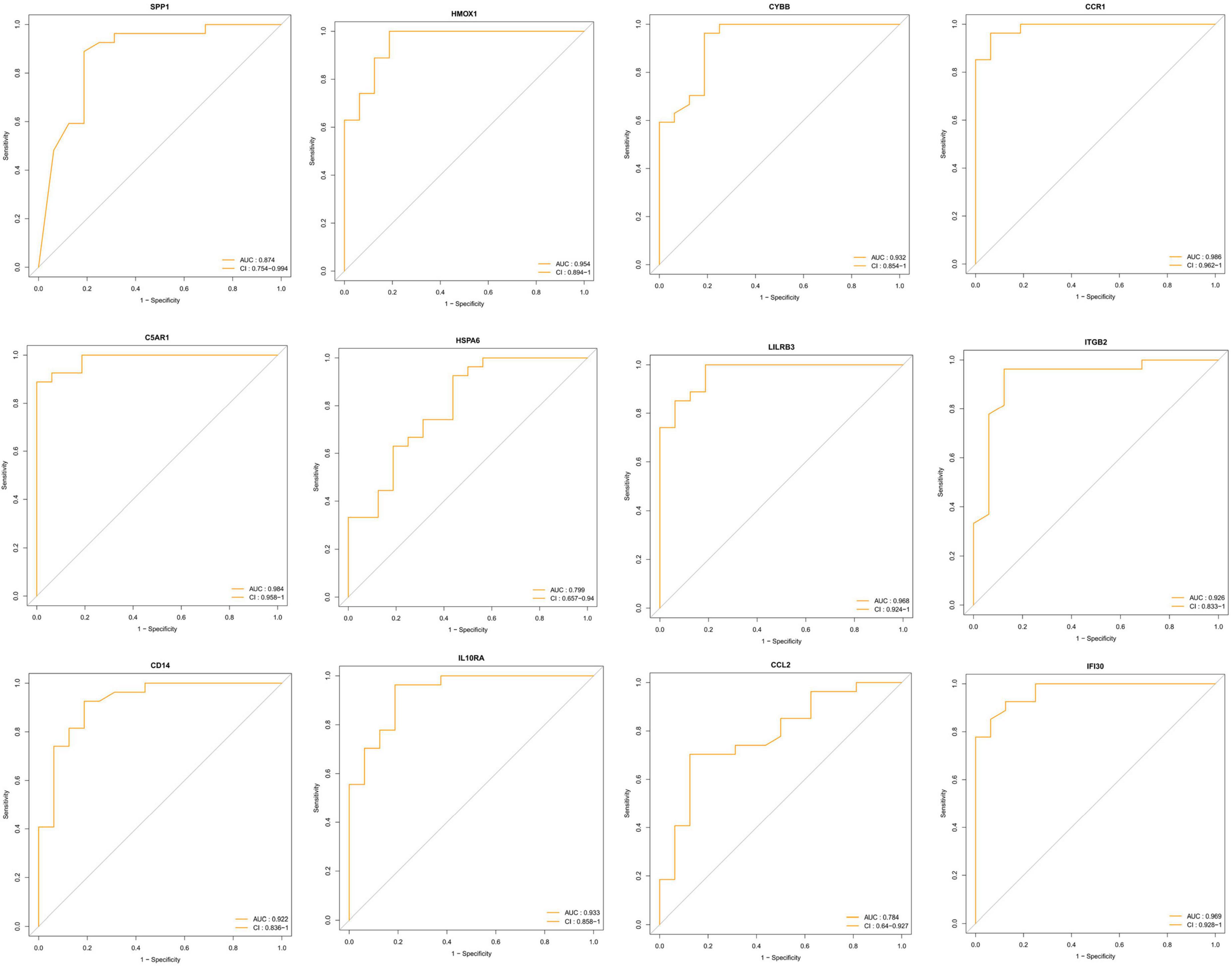
Figure 9. Receiver operating characteristic (ROC) analysis of differentially expressed immune-related genes in the carotid plaque group.
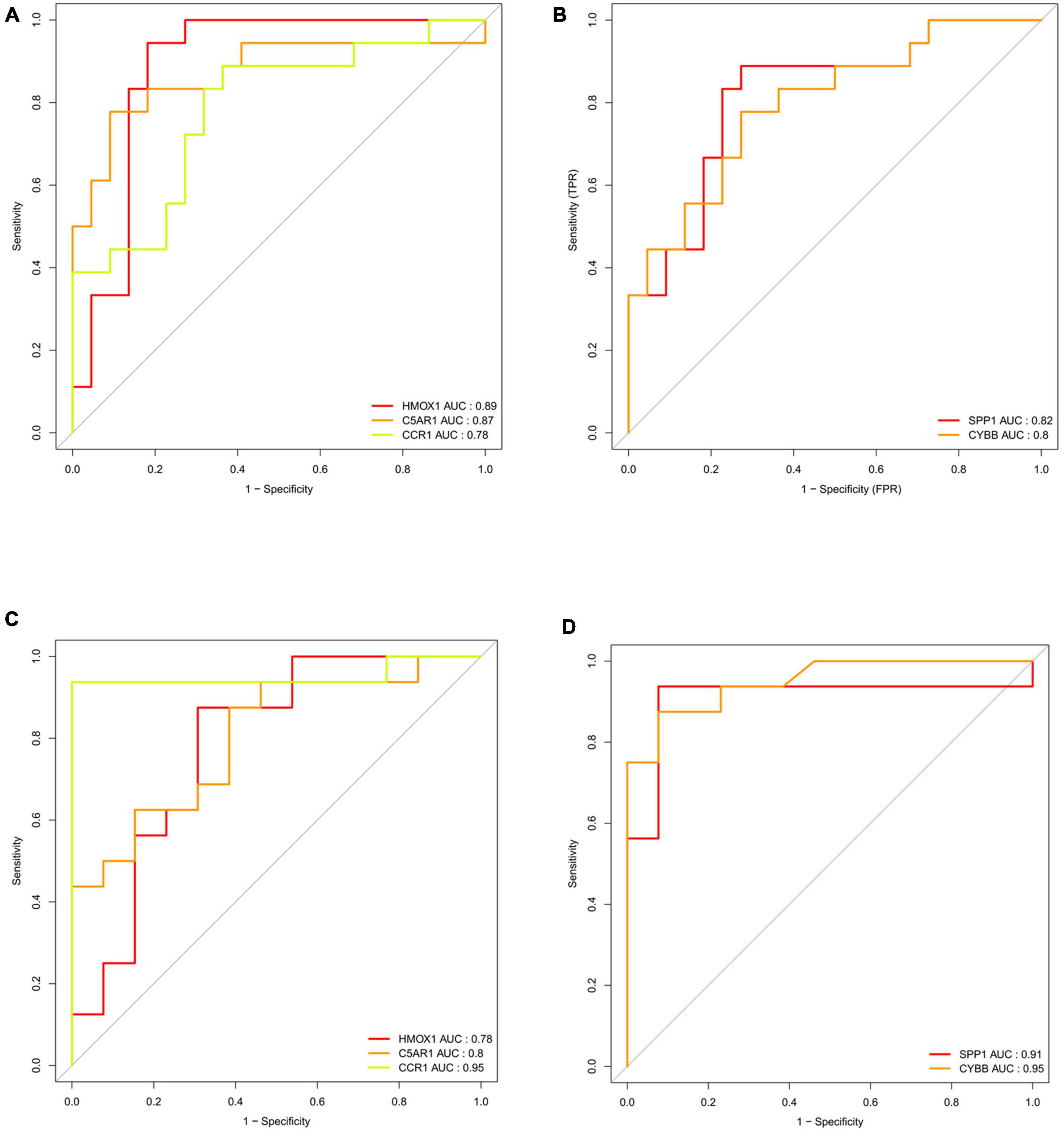
Figure 10. Validation of key genes (HMOX1, C5AR1, CCR1, SPP1, and CYBB) in the validation datasets. (A) Receiver operating characteristic (ROC) analysis of HMOX1, C5AR1, and CCR1 in the validation dataset of vascular dementia. (B) ROC analysis of SPP1 and CYBB in the validation dataset of vascular dementia. (C) ROC analysis of HMOX1, C5AR1, and CCR1 in the validation dataset of carotid plaque. (D) ROC analysis of SPP1 and CYBB in the validation dataset of carotid plaque.
Gene-drug interaction analysis
The five key DEIRGs were considered as potential druggable targets for VaD or carotid plaques treatment. The drug-gene interaction analysis found 19 candidate target drugs/compounds for treatment. Among them, four targeted HMOX1, five targeted CCR1, five targeted SPP1, three targeted CYBB, and one targeted C5AR1. For 14 of these candidate drugs, no specific interactions with these five key genes have been reported; therefore, these drugs might need further investigation. For the other five candidate drugs, specific types of interactions with key genes have been reported (Table 4). Until now, none of the five drugs have been used directly to treat VaD or carotid plaques, as was shown by searching the ClinicalTrials.gov registry.
Discussion
In this study, we investigated gene expression patterns from the brain cortex of VaD and atheromatous carotid plaques of symptomatic patients undergoing carotid endarterectomy surgery using a systems biology approach to identify biomolecular signatures that underlie common pathophysiological mechanisms between the two diseases.
We identified 41 overlapped DEGs between the VaD and carotid atherosclerosis plaque datasets. GO enrichment analyses revealed that these overlapped DEGs were mainly enriched in inflammatory and immune-related processes. Previous studies showed that inflammatory and immune-related processes played vital roles in the development of VaD and carotid atherosclerosis plaques (Ammirati et al., 2015; Poh et al., 2021). Our results aligned with previous studies and indicated that inflammatory and immune-related processes might be a crucial common pathophysiological mechanism that VaD and atheromatous carotid plaques shared.
Considering the above functional enrichment analysis results, we further evaluated the infiltration of 22 immune cell types in two datasets. The results revealed that the top five highest infiltrating fractions in carotid plaque and VaD samples differed, indicating that although inflammatory and immune processes were a common pathophysiological mechanism between two diseases, they might be mediated by distinct immune cell types. In carotid atherosclerosis plaque samples, M0 macrophages, M2 macrophages, and T cells gamma delta have a dominant abundance, consistent with a previous study that showed that T cells and macrophages dominated the immune landscape of atherosclerotic plaques (Fernandez et al., 2019). Moreover, M0 macrophages showed a significantly different infiltration percentage between the early and advanced stage plaques group. Plaque macrophages are a functionally heterogeneous group of immune cells, and M0 macrophages were considered non-differentiated macrophages, which could polarize toward pro-inflammatory M1 macrophages or anti-inflammatory M2 macrophages. M1 macrophages could contribute to an increased and sustained inflammatory response via the secretion of pro-inflammatory cytokines and promote the progression of atherosclerotic plaques. In contrast, the M2 macrophages are considered anti-atherogenic by producing anti-inflammatory cytokines and promoting tissue repair (Colin et al., 2014). Therefore, it is necessary to elucidate the molecular mechanisms of macrophage phenotype switching in the future, which might be a potential target for delaying plaque progression.
In VaD samples, resting CD4 memory T cells, M2 macrophages, and naive B cells were the top three highest infiltrating fractions. Furthermore, B cells and NK cells showed a different infiltration percentage between VaD and matched controls. Previous studies have rarely focused on the infiltration of immune cell types in VaD. Busse et al. (2017, 2021) investigated the frequency of innate and adaptive immune cell populations in whole blood and CSF from different forms of dementia, including VaD. They found alterations of innate and adaptive immune cells in the CSF and peripheral immune system. Compared to healthy persons, B cells, T cells, monocytes, and NK cells in whole blood were diminished in VaD, and central memory CD4 + T cells in CSF were reduced, and NK and NKT cells were enhanced in VaD; the number of B lymphocytes was unchanged between the VaD and controls. Together, these results indicated that these immune cell types might be involved in the pathogenesis of VaD, and their role needs to clarify in the future. We identified 12 DEIRGs and investigated the correlation between these DEIRGs and differentially expressed immune cells of the corresponding groups. The results revealed that these DEIRGs were closely related to differentially expressed immune cells, indicating that these DEIRGs might have an essential role in altering the infiltration percentage of these immune cells. Figure 11 briefly summarizes the association between inflammation pathways and the immune system as well as the development of vascular dementia and carotid plaque.
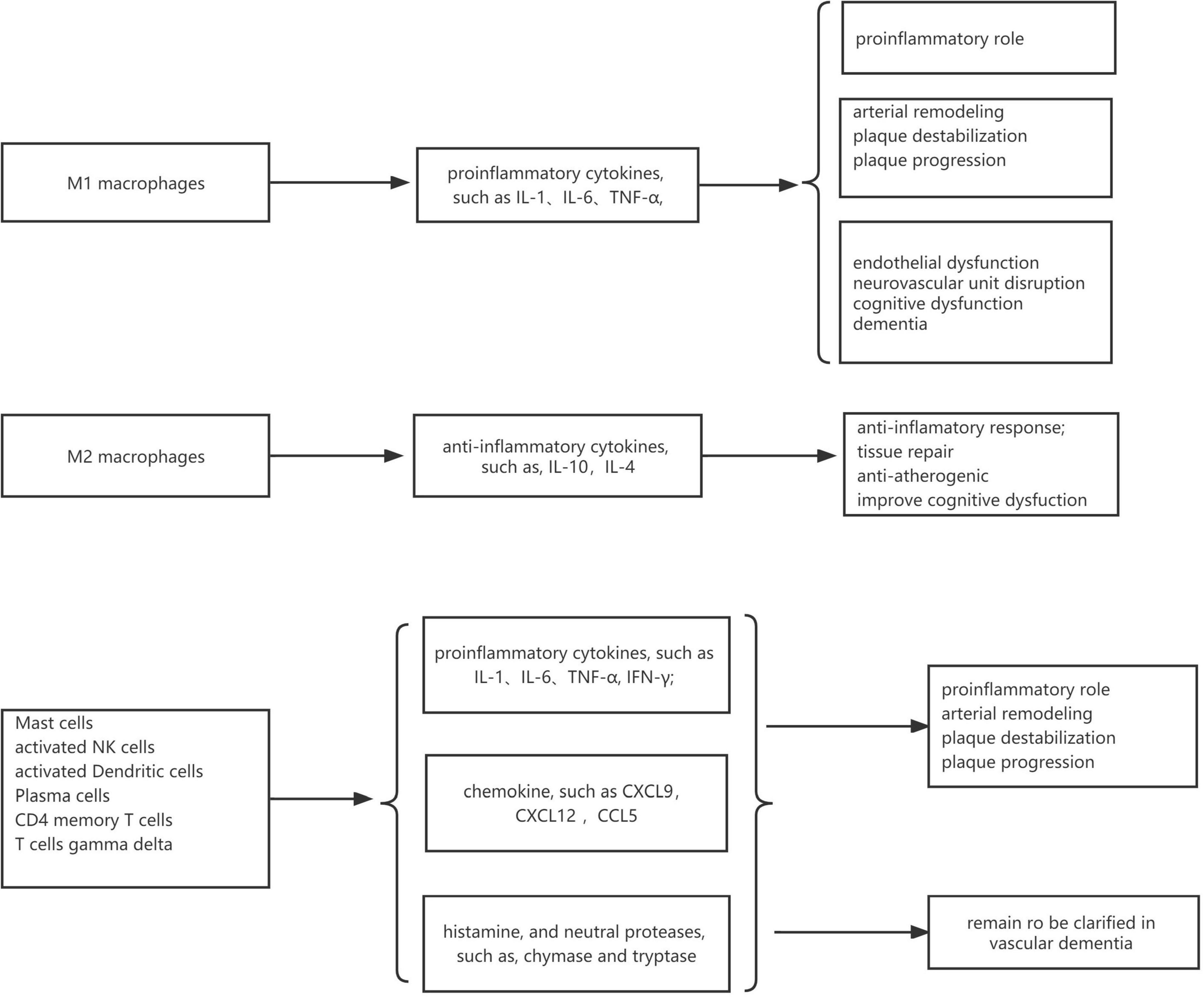
Figure 11. The association between inflammation pathways and the immune system as well as the development of vascular dementia and carotid plaque.
We identified five key DEIRGs based on ROC analysis. The gene HMOX1 also named HO-1, encodes the enzyme that could exert its anti-oxidant and anti-inflammatory effects by several pathways, including degrading the pro-inflammatory heme and producing potent anti-oxidants (Nath et al., 2000). Moreover, as one of the Nrf2 targets, HO-1 had neuroprotective effects. HO-1 knockout worsens infarcts (Shah et al., 2011), and HO-1 overexpression reduces infarcts in mice (Zhang et al., 2012). Mao et al. (2019) induced experimental vascular cognitive impairment in rats by permanent occlusion of both common carotid arteries for 6 weeks. They found sulforaphane could alleviate neuronal damage, white matter injury, blood-brain barrier disruption cognitive impairment by activating the Nrf2/HO-1 pathway. HO-1 also played a protective role in the pathophysiology of atherosclerosis. In APOE-/- mice, a lack of HO-1 accelerated atherosclerosis (Yet et al., 2003), whereas HO-1 induction inhibited atherosclerotic lesion formation in low-density lipoprotein receptor knockout mice (Ishikawa et al., 2001). A study found that plasma HO-1 levels were significantly higher in subjects with carotid plaque than without plaque and were a significant factor for carotid plaque independent of atherosclerotic risk factors (Kishimoto et al., 2018). The protein CD88 encoded by the gene C5AR1 is a classical C5a receptor that has a role in CNS disease processes by binding to C5a. A previous study showed increased expression of CD88 in VaD brain tissue compared to age-matched controls or AD samples by Western blot and immunohistochemistry, which was consistent with our results (Fonseca et al., 2013). Another study showed that treatment with a CD88-specific antagonist enhanced behavioral performance in murine AD models, suggesting that C5a/CD88 interaction had an essential role in the progression of AD (Fonseca et al., 2009). However, the role of CD88 in VaD was not clear. Pavlovski et al. (2012) found that the C5a and CD88 were up-regulated in ischemic neurons and promoted neuronal apoptosis. Pretreatment of the cells with the specific CD88 receptor antagonist PMX53 significantly blocked ischemia-induced apoptosis. They also found that CD88–/– mice subjected to middle cerebral artery occlusion (MCAO) had significantly reduced infarct volumes and improved neurological scores. Stroke is one of the leading causes of VaD; thus, reducing ischemic injury might help prevent or delay the progression of VaD. Accumulating evidence in humans and experimental animal models revealed that the C5a–C5aR axis is involved in the development of atherosclerosis lesions (An et al., 2014). A recent study found that C5aR1 was higher in carotid plaques than in control arteries (Niyonzima et al., 2020), which was in line with our results. The gene CCR1 encodes a member of beta chemokine receptor family that belongs to G protein-coupled receptors (White et al., 2013). The activation of CCR1 was pro-inflammatory in several animal models of neurological diseases. A previous study reported that the expression of CCR1 was increased in the brain after MCAO (Offner et al., 2006) and intracerebral hemorrhage (ICH) in mice (Yan et al., 2020). In the mouse model of ICH, CCR1 activation could promote acute neuroinflammation, and the inhibition of CCR1 improved neurobehavioral deficits and attenuated brain edema and neuroinflammation (Yan et al., 2020). These results indicated that CCR1 might be involved in the pathophysiological mechanism of stroke. There are few studies on the role of CCR1 in atherosclerosis. A study found that blood-borne CCR1 altered the immuno-inflammatory response in atherosclerosis and prevented excessive plaque growth and inflammation (Potteaux et al., 2005). A recent study also found that CCR1 was up-regulated in carotid atherosclerosis, but the exact mechanism was unclear (Meng et al., 2021). The gene SPP1 encodes the protein secreted phosphoprotein 1, also known as osteopontin (OPN). OPN is an extracellular phosphoprotein expressed in various tissues and cells in response to stress and injury and is also a soluble cytokine involved in inflammation. Previous studies revealed that it was upregulated in symptomatic carotid atherosclerosis (Golledge et al., 2004), stroke (Carbone et al., 2015), and vascular cognitive impairment (Chai et al., 2021), suggesting the involvement of OPN in these inflammation-associated neurological diseases, which supported our results. The gene CYBB encodes the protein cytochrome b-245 heavy chain, also named NADPH oxidase 2 (Nox 2), which is one of the NADPH oxidase (Nox) isoforms. It was reported that Nox 2 had been implicated in the VaD and atherosclerotic plaques. Previous studies indicated that Nox2 hereditary deficiency in humans was associated with reduced atherosclerotic burden (Violi et al., 2013). Specific Nox2 inactivation would arrest atheroma plaque progression and instability in animal models (Quesada et al., 2015). Another study revealed that Ling-Yang-Gou-Teng -Decoction, a well-known traditional Chinese formula, exhibited beneficial effects on the VaD by decreasing NOX2 expression (Zhao et al., 2018). Collectively, these results indicated that the five key genes played vital roles in the pathogenesis of VaD and carotid atherosclerotic plaques and might be potential targets for both diseases’ treatment.
We performed a drug-gene interaction analysis to detect potential target drugs/compounds for VaD and carotid atherosclerotic plaques treatment and identified five drugs that were predicted to have specific types of interactions with these key genes. We checked the five candidate drugs in the ClinicalTrials.gov registry. Four drugs (avacopan, CCX354, BMS-817399, and ASK-8007) could be potential drugs for VaD and carotid atherosclerotic plaques treatment. Avacopan was a C5AR1 antagonist, CCX354 and BMS-817399 were CCR1 antagonists, and ASK-8007 was an SPP1 inhibitor. A randomized, placebo-controlled trial showed that C5a receptor inhibition with avacopan was effective in replacing high-dose glucocorticoids in treating antineutrophil cytoplasmic antibody (ANCA)-associated vasculitis. CCX354-C, BMS-817399, and ASK-8007 were all studied to treat Rheumatoid arthritis (RA). ANCA-associated vasculitis and RA were both chronic inflammatory diseases and associated with atherosclerosis and VaD (Atzeni et al., 2017). A systematic review with meta-analysis showed a statistically significant increase in the risk of dementia among patients with RA compared with non-RA controls (Ungprasert et al., 2016). Bilateral carotid plaque was more than twice in RA than in controls (Wah-Suarez et al., 2019). In future studies, these four drugs need to be evaluated as potential drugs in VaD and carotid atherosclerotic plaques treatment.
There are some limitations to the present study. Firstly, the study is only based on bioinformatics analysis; no clinical or experimental confirmation of the roles of key genes was attempted. Secondly, the sample size in this study is not very large, and it will be necessary to replicate our results in more datasets with larger sample size when available. Notwithstanding these limitations, this study offers some insights into the underlying common pathophysiological mechanism shared by VaD and carotid plaques and might provide potential targets for the treatment.
Conclusion
We identified overlapping DEGs between VaD and carotid plaques. These overlapping DEGs were enriched mainly in inflammatory and immune-related processes, indicating it might be a crucial common pathophysiological mechanism shares by VaD and carotid plaques. We identified DEIRGs, evaluated the infiltration of immune cells, and investigated the correlation between DEIRGs and differentially expressed immune cells to gain greater insight into the role of inflammatory and immune-related processes in vascular dementia and carotid plaque progression. We identified five key DEIRGs and performed gene-drug analysis, which might provide potential targets and drugs for therapeutic intervention.
Data availability statement
The original contributions presented in this study are included in the article/supplementary material, further inquiries can be directed to the corresponding authors.
Author contributions
JS, YR, WT, WW, LZ, and JC conceived and designed the study, analyzed the data, wrote the manuscript, contributed to the designs of the methods used in this study, read, and agreed to the publication of this version of the manuscript.
Funding
This work was funded by Shanghai Municipal Key Clinical Specialty (Grant No. shslczdzk02801), the Shanghai Municipal Health Commission (Grant No. 2020YJZX0109), the Shanghai Pujiang Program (Grant No. 2019PJD013), and the National Natural Science Foundation of China (NSFC, Grant No. 81471103).
Conflict of interest
The authors declare that the research was conducted in the absence of any commercial or financial relationships that could be construed as a potential conflict of interest.
Publisher’s note
All claims expressed in this article are solely those of the authors and do not necessarily represent those of their affiliated organizations, or those of the publisher, the editors and the reviewers. Any product that may be evaluated in this article, or claim that may be made by its manufacturer, is not guaranteed or endorsed by the publisher.
Footnotes
- ^ https://www.ncbi.nlm.nih.gov/geo/
- ^ https://www.ncbi.nlm.nih.gov/geo/geo2r/
- ^ http://metascape.org
- ^ www.dgidb.org
References
Ammirati, E., Moroni, F., Norata, G. D., Magnoni, M., and Camici, P. G. (2015). Markers of inflammation associated with plaque progression and instability in patients with carotid atherosclerosis. Mediators Inflamm. 2015:718329. doi: 10.1155/2015/718329
An, G., Ren, G., An, F., and Zhang, C. (2014). Role of C5a-C5aR axis in the development of atherosclerosis. Sci. China Life Sci. 57, 790–794. doi: 10.1007/s11427-014-4711-5
Anbar, R., Sultan, S. R., Al, S. L., Alkharaiji, M., Chaturvedi, N., Hardy, R., et al. (2022). Is carotid artery atherosclerosis associated with poor cognitive function assessed using the mini-mental state examination? a systematic review and meta-analysis. BMJ Open 12:e55131. doi: 10.1136/bmjopen-2021-055131
Atzeni, F., Pipitone, N., Iaccarino, L., Masala, I. F., Weiss, R., Alciati, A., et al. (2017). Rheumatic diseases and autoimmune vascular dementia. Autoimmun. Rev. 16, 1265–1269. doi: 10.1016/j.autrev.2017.10.011
Bardou, P., Mariette, J., Escudie, F., Djemiel, C., and Klopp, C. (2014). Jvenn: An interactive Venn diagram viewer. BMC Bioinf. 15:293. doi: 10.1186/1471-2105-15-293
Barrett, T., Wilhite, S. E., Ledoux, P., Evangelista, C., Kim, I. F., Tomashevsky, M., et al. (2013). NCBI GEO: archive for functional genomics data sets–update. Nucleic Acids Res. 41:D991–D995. doi: 10.1093/nar/gks1193
Baumgart, M., Snyder, H. M., Carrillo, M. C., Fazio, S., Kim, H., and Johns, H. (2015). Summary of the evidence on modifiable risk factors for cognitive decline and dementia: A population-based perspective. Alzheimers Dement. 11, 718–726. doi: 10.1016/j.jalz.2015.05.016
Bhattacharya, S., Dunn, P., Thomas, C. G., Smith, B., Schaefer, H., Chen, J., et al. (2018). ImmPort, toward repurposing of open access immunological assay data for translational and clinical research. Sci. Data 5:180015. doi: 10.1038/sdata.2018.15
Busse, M., Michler, E., von Hoff, F., Dobrowolny, H., Hartig, R., Frodl, T., et al. (2017). Alterations in the peripheral immune system in dementia. J. Alzheimers Dis. 58, 1303–1313. doi: 10.3233/JAD-161304
Busse, S., Hoffmann, J., Michler, E., Hartig, R., Frodl, T., and Busse, M. (2021). Dementia-associated changes of immune cell composition within the cerebrospinal fluid. Brain Behav. Immunity Health 14:100218. doi: 10.1016/j.bbih.2021.100218
Carbone, F., Vuilleumier, N., Burger, F., Roversi, G., Tamborino, C., Casetta, I., et al. (2015). Serum osteopontin levels are upregulated and predict disability after an ischaemic stroke. Eur. J. Clin. Invest. 45, 579–586. doi: 10.1111/eci.12446
Chai, Y. L., Chong, J. R., Raquib, A. R., Xu, X., Hilal, S., Venketasubramanian, N., et al. (2021). Plasma osteopontin as a biomarker of alzheimer’s disease and vascular cognitive impairment. Sci. Rep. 11:4010
Chen, B., Khodadoust, M. S., Liu, C. L., Newman, A. M., and Alizadeh, A. A. (2018). Profiling tumor infiltrating immune cells with cibersort. Methods Mol. Biol. 1711, 243–259. doi: 10.1007/978-1-4939-7493-1_12
Chen, Y., Liu, Q., Liu, J., Wei, P., Li, B., Wang, N., et al. (2022). Revealing the modular similarities and differences among alzheimer’s disease. vascular dementia, and parkinson’s disease in genomic networks. Neuromolecular. Med. 24, 125–138. doi: 10.1007/s12017-021-08670-2
Chung, Y., and Lee, H. (2021). Correlation between alzheimer’s disease and type 2 diabetes using non-negative matrix factorization. Sci. Rep. 11:15265. doi: 10.1038/s41598-021-94048-0
Colin, S., Chinetti-Gbaguidi, G., and Staels, B. (2014). Macrophage phenotypes in atherosclerosis. Immunol. Rev. 262, 153–166. doi: 10.1111/imr.12218
Cortes-Canteli, M., Gispert, J. D., Salvado, G., Toribio-Fernandez, R., Tristao-Pereira, C., Falcon, C., et al. (2021). Subclinical atherosclerosis and brain metabolism in middle-aged individuals: the pesa study. J. Am. Coll. Cardiol. 77, 888–898. doi: 10.1016/j.jacc.2020.12.027
Dong, J., Song, C., Zhang, L., Feng, X., Feng, R., Lu, Q., et al. (2017). Identified key genes related to carotid atheroma plaque from gene expression chip. Artif. Cells Nanomed. Biotechnol. 45, 1–6. doi: 10.1080/21691401.2016.1216858
Fernandez, D. M., Rahman, A. H., Fernandez, N. F., Chudnovskiy, A., Amir Amadori, L., et al. (2019). Single-cell immune landscape of human atherosclerotic plaques. Nat. Med. 25, 1576–1588. doi: 10.1038/s41591-019-0590-4
Fonseca, M. I., Ager, R. R., Chu, S. H., Yazan, O., Sanderson, S. D., LaFerla, F. M., et al. (2009). Treatment with a c5ar antagonist decreases pathology and enhances behavioral performance in murine models of alzheimer’s disease. J. Immunol. 183, 1375–1383. doi: 10.4049/jimmunol.0901005
Fonseca, M. I., McGuire, S. O., Counts, S. E., and Tenner, A. J. (2013). Complement activation fragment c5a receptors, cd88 and c5l2, are associated with neurofibrillary pathology. J. Neuroinflamm. 10:25. doi: 10.1186/1742-2094-10-25
Freshour, S. L., Kiwala, S., Cotto, K. C., Coffman, A. C., McMichael, J. F., Song, J. J., et al. (2021). Integration of the Drug-Gene Interaction Database (DGIdb 4.0) with open crowdsource efforts. Nucleic Acids Res. 49:D1144–D1151. doi: 10.1093/nar/gkaa1084
Golledge, J., McCann, M., Mangan, S., Lam, A., and Karan, M. (2004). Osteoprotegerin and osteopontin are expressed at high concentrations within symptomatic carotid atherosclerosis. Stroke 35, 1636–1641. doi: 10.1161/01.STR.0000129790.00318.a3
Iadecola, C., Duering, M., Hachinski, V., Joutel, A., Pendlebury, S. T., Schneider, J. A., et al. (2019). Vascular cognitive impairment and dementia: Jacc scientific expert panel. J. Am. Coll. Cardiol. 73, 3326–3344. doi: 10.1016/j.jacc.2019.04.034
Ishikawa, K., Sugawara, D., Wang, X., Suzuki, K., Itabe, H., Maruyama, Y., et al. (2001). Heme oxygenase-1 inhibits atherosclerotic lesion formation in ldl-receptor knockout mice. Circ. Res. 88, 506–512. doi: 10.1161/01.RES.88.5.506
Jassal, B., Matthews, L., Viteri, G., Gong, C., Lorente, P., Fabregat, A., et al. (2020). The reactome pathway knowledgebase. Nucleic Acids Res. 48:D498–D503. doi: 10.1093/nar/gkz1031
Kanehisa, M., Furumichi, M., Tanabe, M., Sato, Y., and Morishima, K. K. E. G. G. (2017). New perspectives on genomes, pathways, diseases and drugs. Nucleic Acids Res. 45:D353–D361. doi: 10.1093/nar/gkw1092
Kishimoto, Y., Sasaki, K., Saita, E., Niki, H., Ohmori, R., Kondo, K., et al. (2018). Plasma heme oxygenase-1 levels and carotid atherosclerosis. Stroke 49, 2230–2232. doi: 10.1161/STROKEAHA.118.022256
Li, S., Zhang, Q., Huang, Z., Tao, W., Zeng, C., Yan, L., et al. (2022). Comprehensive analysis of immunocyte infiltration and the key genes associated with intraplaque hemorrhage in carotid atherosclerotic plaques. Int. Immunopharmacol. 106:108633. doi: 10.1016/j.intimp.2022.108633
Mao, L., Yang, T., Li, X., Lei, X., Sun, Y., Zhao, Y., et al. (2019). Protective effects of sulforaphane in experimental vascular cognitive impairment: contribution of the nrf2 pathway. J. Cereb. Blood Flow Metab. 39, 352–366. doi: 10.1177/0271678X18764083
Martens, M., Ammar, A., Riutta, A., Waagmeester, A., Slenter, D. N., Hanspers, K., et al. (2021). WikiPathways: connecting communities. Nucleic Acids Res. 49:D613–D621. doi: 10.1093/nar/gkaa1024
Meng, Y., Zhang, C., Liang, L., Wei, L., Wang, H., Zhou, F., et al. (2021). Identification of potential key genes involved in the carotid atherosclerosis. Clin. Interv. Aging 16, 1071–1084. doi: 10.2147/CIA.S312941
Nath, K. A., Haggard, J. J., Croatt, A. J., Grande, J. P., Poss, K. D., and Alam, J. (2000). The indispensability of heme oxygenase-1 in protecting against acute heme protein-induced toxicity in vivo. Am. J. Pathol. 156, 1527–1535. doi: 10.1016/S0002-9440(10)65024-9
Niyonzima, N., Bakke, S. S., Gregersen, I., Holm, S., Sandanger, O., Orrem, H. L., et al. (2020). Cholesterol crystals use complement to increase NLRP3 signaling pathways in coronary and carotid atherosclerosis. eBiomedicine 60:102985. doi: 10.1016/j.ebiom.2020.102985
Offner, H., Subramanian, S., Parker, S. M., Afentoulis, M. E., Vandenbark, A. A., and Hurn, P. D. (2006). Experimental stroke induces massive, rapid activation of the peripheral immune system. J. Cereb. Blood Flow Metab. 26, 654–665. doi: 10.1038/sj.jcbfm.9600217
Pavlovski, D., Thundyil, J., Monk, P. N., Wetsel, R. A., Taylor, S. M., and Woodruff, T. M. (2012). Generation of complement component C5a by ischemic neurons promotes neuronal apoptosis. Faseb. J. 26, 3680–3690. doi: 10.1096/fj.11-202382
Poh, L., Fann, D. Y., Wong, P., Lim, H. M., Foo, S. L., Kang, S. W., et al. (2021). AIM2 inflammasome mediates hallmark neuropathological alterations and cognitive impairment in a mouse model of vascular dementia. Mol. Psychiatry 26, 4544–4560. doi: 10.1038/s41380-020-00971-5
Potteaux, S., Combadière, C., Esposito, B., Casanova, S., Merval, R., Ardouin, P., et al. (2005). Chemokine receptor CCR1 disruption in bone marrow cells enhances atherosclerotic lesion development and inflammation in mice. Mol. Med. 11, 16–20. doi: 10.2119/2005-00028.Potteaux
Quesada, I. M., Lucero, A., Amaya, C., Meijles, D. N., Cifuentes, M. E., Pagano, P. J., et al. (2015). Selective inactivation of nadph oxidase 2 causes regression of vascularization and the size and stability of atherosclerotic plaques. Atherosclerosis 242, 469–475. doi: 10.1016/j.atherosclerosis.2015.08.011
Shah, Z. A., Nada, S. E., and Dore, S. (2011). Heme oxygenase 1, beneficial role in permanent ischemic stroke and in Gingko biloba (EGb 761) neuroprotection. Neuroscience 180, 248–255. doi: 10.1016/j.neuroscience.2011.02.031
Shu, J., Wei, W., and Zhang, L. (2022). Identification of molecular signatures and candidate drugs in vascular dementia by bioinformatics analyses. Front. Mol. Neurosci. 15:751044. doi: 10.3389/fnmol.2022.751044
Ungprasert, P., Wijarnpreecha, K., and Thongprayoon, C. (2016). Rheumatoid arthritis and the risk of dementia: A systematic review and meta-analysis. Neurol India 64, 56–61. doi: 10.4103/0028-3886.173623
Violi, F., Pignatelli, P., Pignata, C., Plebani, A., Rossi, P., Sanguigni, V., et al. (2013). Reduced atherosclerotic burden in subjects with genetically determined low oxidative stress. Arterioscler. Thromb. Vasc. Biol. 33, 406–412. doi: 10.1161/ATVBAHA.112.300438
Wah-Suarez, M. I., Galarza-Delgado, D. A., Azpiri-Lopez, J. R., Colunga-Pedraza, I. J., Abundis-Marquez, E. E., Davila-Jimenez, J. A., et al. (2019). Carotid ultrasound findings in rheumatoid arthritis and control subjects: A case-control study. Int. J. Rheum. Dis. 22, 25–31. doi: 10.1111/1756-185X.13377
Wang, Y., Lv, S., Zhou, X., Niu, X., Chen, L., Yang, Z., et al. (2022). Identification of tlr2 as a key target in neuroinflammation in vascular dementia. Front. Genet. 13:860122. doi: 10.3389/fgene.2022.860122
White, G. E., Iqbal, A. J., and Greaves, D. R. C. C. (2013). Chemokine receptors and chronic inflammation–therapeutic opportunities and pharmacological challenges. Pharmacol. Rev. 65, 47–89. doi: 10.1124/pr.111.005074
Yan, J., Zuo, G., Sherchan, P., Huang, L., Ocak, U., Xu, W., et al. (2020). Ccr1 activation promotes neuroinflammation through ccr1/tpr1/erk1/2 signaling pathway after intracerebral hemorrhage in mice. Neurotherapeutics 17, 1170–1183. doi: 10.1007/s13311-019-00821-5
Yet, S. F., Layne, M. D., Liu, X., Chen, Y. H., Ith, B., Sibinga, N. E., et al. (2003). Absence of heme oxygenase-1 exacerbates atherosclerotic lesion formation and vascular remodeling. Faseb J. 17, 1759–1761. doi: 10.1096/fj.03-0187fje
Zhang, F., Wang, S., Zhang, M., Weng, Z., Li, P., Gan, Y., et al. (2012). Pharmacological induction of heme oxygenase-1 by a triterpenoid protects neurons against ischemic injury. Stroke 43, 1390–1397. doi: 10.1161/STROKEAHA.111.647420
Zhao, X., Liu, J., Yang, S., Song, D., Wang, C., Chen, C., et al. (2018). Ling-Yang-Gou-Teng-decoction prevents vascular dementia through inhibiting oxidative stress induced neurovascular coupling dysfunction. J. Ethnopharmacol. 222, 229–238. doi: 10.1016/j.jep.2018.03.015
Zheng, L., Yue, X., Li, M., Hu, J., Zhang, B., Zhang, R., et al. (2022). Contribution of fbln5 to unstable plaques in carotid atherosclerosis via mir128 and mir532–3p based on bioinformatics prediction and validation. Front. Genet. 13:821650. doi: 10.3389/fgene.2022.821650
Keywords: vascular dementia, carotid plaques, immune cell infiltration, gene-drug interaction analysis, bioinformatics
Citation: Shu J, Ren Y, Tan W, Wei W, Zhang L and Chang J (2022) Identification of potential drug targets for vascular dementia and carotid plaques by analyzing underlying molecular signatures shared by them. Front. Aging Neurosci. 14:967146. doi: 10.3389/fnagi.2022.967146
Received: 12 June 2022; Accepted: 05 September 2022;
Published: 03 October 2022.
Edited by:
Suzana Makpol, National University of Malaysia, MalaysiaReviewed by:
Noor Akmal Shareela Ismail, National University of Malaysia, MalaysiaJaya Kumar, National University of Malaysia, Malaysia
Copyright © 2022 Shu, Ren, Tan, Wei, Zhang and Chang. This is an open-access article distributed under the terms of the Creative Commons Attribution License (CC BY). The use, distribution or reproduction in other forums is permitted, provided the original author(s) and the copyright owner(s) are credited and that the original publication in this journal is cited, in accordance with accepted academic practice. No use, distribution or reproduction is permitted which does not comply with these terms.
*Correspondence: Wenshi Wei, wenshiwei1999@163.com; Li Zhang, lizhang_huadong@163.com; Jie Chang, changjie69@163.com
†These authors have contributed equally to this work and share first authorship