- 1Unidad de Investigación Epidemiológica y en Servicios de Salud, Área Envejecimiento, Centro Médico Nacional Siglo XXI, Instituto Mexicano del Seguro Social, Mexico City, Mexico
- 2Epidemiology, Endocrinology, and Nutrition Research Unit, Hospital Infantil de México Federico Gomez, Ministry of Health (SSA), Mexico City, Mexico
- 3Department of Molecular Biology, Instituto Nacional de Cardiología Ignacio Chávez, Mexico City, Mexico
- 4Sección de Estudios de Posgrado e Investigación, Escuela Superior de Medicina, Instituto Politécnico Nacional, Mexico City, Mexico
- 5Facultad de Estudios Superiores Zaragoza, Universidad Nacional Autónoma de México, Mexico City, Mexico
- 6Division of Neurogenetics and Molecular Psychiatry, Department of Psychiatry and Psychotherapy, University of Cologne, Köln, Germany
Objective: Evidence supports the important role of neuroinflammation in some types of dementia. This study aimed to evaluate the effect of epistasis of gene cytokines such as interleukin (IL)-α, IL-6, tumor necrosis factor (TNFα), and interferon-gamma (IFN-γ) on the susceptibility to the development of dementia.
Materials and methods: In the study, 221 patients diagnosed with dementia and 710 controls were included. The multifactor-dimensionality reduction (MDR) analysis was performed to identify the epistasis between SNP located in genes of IL-α (rs1800587), IL-6 (rs1800796), TNFα (rs361525 and rs1800629), and IFNγ (rs2069705). The best risk prediction model was identified based on precision and cross-validation consistency.
Results: Multifactor-dimensionality reduction analysis detected a significant model with the genes TNFα, IFNγ, IL1α, and IL6 (prediction success: 72%, p < 0.0001). When risk factors were analyzed with these polymorphisms, the model achieved a similar prediction for dementia as the genes-only model.
Conclusion: These data indicate that gene–gene interactions form significant models to identify populations susceptible to dementia.
Introduction
Dementia is a neurodegenerative disorder that results in a decreased quality of life and increased disability and mortality. The reported prevalence of dementia worldwide is varied; in Mexico, it ranges between 6.1 and 7.9% (Gutierrez-Robledo and Arrieta-Cruz, 2015).
The etiology of dementia is unknown; however, it has been related to both environmental and genetic factors (Harman, 2006; Fleming et al., 2022). Some cases are neuropathologically characterized by the presence of extracellular amyloid plaques and neurofibrillary tangles containing phosphorylated tau protein, followed by alterations and neuronal degeneration (da Cruz e Silva et al., 2010; Henriques et al., 2015).
The ApoE ε4 allele is currently the only genetic factor directly associated with the risk of dementia. Still, only 50% of the patients with dementia carry the allele, so other genetic factors must be related to this disease (Bertram, 2009; Zekanowski and Wojda, 2009; Nazarian et al., 2019).
Recent studies have reported that inflammatory responses play a key role in the neurodegenerative cascades of dementias (Heneka et al., 2015; Darweesh et al., 2018), and some of these markers have been associated with the severity and progression of the disease (Hesse et al., 2016; Olsson et al., 2016).
Genome-wide association studies (GWAS) support the interaction between the inflammatory process and dementia. In these studies, several inflammation-related genes have been identified. Among them are CR1 (complement receptor 1); MS4A (membrane-spanning 4-domains subfamily A), which crosses the membrane; TREM2 (triggering receptor expressed on myeloid cells 2); and CD33, mainly found in Caucasian and Chinese populations (Karch and Goate, 2015; Toral-Rios et al., 2015; Gillespie et al., 2022).
Other reported findings indicate that cytokines are key components of neuroinflammation and their levels are altered in patients with Alzheimer’s disease (AD). In addition, several case-control studies have been conducted to explore the relationship between cytokine polymorphisms and the risk of developing dementia.
Inflammatory markers, such as the tumor necrosis factor-α (TNF-α), interleukins (IL-1 and IL-6), and interferon-gamma (IFN-γ) have been reported as major molecules in inflammation. They are known to affect the brains of patients with dementia (O’Bryant et al., 2016; Zhu et al., 2017; Zheng et al., 2022). Cytokines, a large family of proteins, include interleukins, TNF, and IFN-γ. Given the cytokine response to inflammation, they have been classified as pro-inflammatory, such as IL-1, IL-6, and TNF-α, while others, such as IFN-γ, present anti-inflammatory properties (Zheng et al., 2016, 2022).
Then, some cytokine genes involved in inflammatory responses are linked to the susceptibility to dementia. They are highly polymorphic, and these polymorphisms affect cytokine expression (Bertram, 2009).
Since IL-1 has been linked to AD, any changes in the genes altering IL-1 production may be risk factors for the development of this disease (Rothwell and Luheshi, 2000). The IL-1 gene contains several polymorphisms; however, studies have only considered −889C/T to investigate the association with susceptibility to the disease.
The anti-inflammatory cytokine IL-6 is increased in dementia brains (Rubio-Perez and Morillas-Ruiz, 2012); nevertheless, some studies have demonstrated differential effects. Specifically, IL-6 has been shown to induce the cortical expression of the amyloid precursor protein (APP), while the overexpression of this cytokine suppresses amyloid β (Aβ) deposition in vivo (Chakrabarty et al., 2010).
TNF-α mediates the proliferation of neurons and induces the production of neurotoxic Aβ. Some polymorphisms of probable functional significance have been identified in the promoter region of the TNF gene, but the published results show conflicting data (Shawkatova et al., 2010; Ardebili et al., 2011).
IFN-γ may inhibit the secretion of soluble APP (sAPP). The IFN-γ gene is on chromosome 12 (Scott et al., 2000), with intronic-located polymorphisms. Some of these polymorphisms may affect IFN-γ secretion and the inflammatory process, triggering a pathologic change in dementia (Oda et al., 2002). Previous analyses have assessed the associations between polymorphisms of cytokine genes but individually.
We investigate the combinatorial effect of four (IL-1α, IL-6, TNFα, and IFN-γ) genetic polymorphisms involved in the inflammatory processes of microglial cells in the brain on the susceptibility to dementia in Mexican individuals.
Materials and methods
Study design, base, and participants
The population of this study comes from the Study of Aging and Dementia in Mexico (SADEM) (Juarez-Cedillo et al., 2012). A total of 931 individuals were included: 221 were diagnosed with dementia and 710 were controls (negative diagnosis for dementia or cognitive problems). Informed consent was obtained from all subjects involved in the study.
Dementia was diagnosed in two steps; the Mini-Mental State Examination (MMSE), along with a battery of neuropsychological tests, was first applied to identify older adults with cognitive problems. Second, dementia was confirmed by the consensus of a panel of experts. They followed the criteria for dementia in the Diagnostic and Statistical Manual of Mental Disorders (DSM-IV-TR) and the AD criteria proposed by the National Institute of Neurological and Communicative Disorders and Stroke and the Alzheimer’s Disease and Related Disorders Association (NINCDS-ADRDA) (McKhann et al., 1984). The Clinical Dementia Rating (CDR) (Hughes et al., 1982) was also used.
All the patients were born in Mexico; they became participants once they were informed of the study and provided their signed consent. The study was conducted according to the guidelines of the Declaration of Helsinki and approved by the National Commission of Scientific Research as well as by the IMSS Ethics Commission, registration number R-2015-785-012.
Clinical characteristics and biochemical analysis
The interview specifically designed for this study included the following: age, sex, and body mass index (BMI) calculated as weight (kg)/height (m2) and divided into three groups, namely, normal weight (BMI < 25 kg/m2), overweight group (BMI of 25–29.9 kg/m2), and obesity (BMI > 30 kg/m2).
Venous blood samples from the subjects were obtained after fasting (10 h). Glucose, triglycerides, total cholesterol, and high-density lipoprotein cholesterol (HDL-c) were determined using an Ekem Control Lab semi-auto chemistry analyzer. The Friedewald equation and the De Long modification were used to calculate the low-density lipoprotein cholesterol (LDL-c) (Romano et al., 1993).
Genetic polymorphism detection
Genomic DNA from peripheral blood was obtained using the Lahiri and Numberger method (Lahiri and Nurnberger, 1991). The DNA purity was verified in a NanoDrop® 1000 spectrophotometer at a wavelength of 260 and 280 nm. To identify potential gene-gene interactions, the SNPs were selected considering prior publications and those previously reported on the SNP website.1 They showed an LD threshold of r2 ≤ 0.1 and a minor allele frequency (MAF) ≥ 1%; they were also clinically relevant to neurological diseases. Quality control procedures included MAF (Skol et al., 2006) and the Hardy-Weinberg equilibrium (HWE) (P < 0.05) (Ryckman and Williams, 2008).
The SNPs IL1-α rs1800587, IL-6, rs1800796, TNF-α rs361525, rs1800629, and IFN-γ rs2069705 were selected from the four cytokine genes included in this study (see Table 1).2 Their frequencies for Africa, Asia, Eastern Europe, South Asia, and the United States have been reported by the 1000 Genome Project (Genomes Project et al., 2012). In addition, the biological effects of these variants and their link with AD were considered.
The samples were genotyped using a real-time PCR system according to the manufacturer’s instructions (Applied Biosystems, CA, USA). Standard DNA controls were used in each real-time PCR assay.
Statistical analysis
The statistical analysis was performed using IBM SPSS Statistics version 23.0 (IBM Corp., Armonk, NY, USA). The Student’s t-test was used for continuous variables, and the results were expressed as the mean standard deviation, while categorical variables were presented as n (%). Fisher’s exact test was used to compare allele and genotype frequencies between cases and controls. Hardy–Weinberg equilibrium was tested by Fisher’s exact test X2 analysis for a 2 × 2 table. The odds ratio (OR) was calculated to evaluate the correlation between the genotypic/allelic frequencies of each gene and the susceptibility to develop dementia. It was adjusted for sex, age, and other factors that showed a statistically significant association with susceptibility to dementia. Bonferroni test was applied. Differences and associations between or among variables were considered significant when P-value is <0.05.
Statistical analysis for gene interactions
Epistasis was evaluated by multifactor-dimensionality reduction analysis was performed using the MDR 3.0.2 software (Computational Genetics Laboratory, Institute for Quantitative Biomedical Sciences, Dartmouth, NH, USA)3 (Ritchie et al., 2003). The best model to predict the susceptibility to dementia was screened based on the minimum classification error in the training set, and for evaluating the predictive accuracy, 10-fold cross-validation was applied.
All the statistical results were significant at p < 0.05. For risk assessment, the genotype combination was identified as a high or low risk depending on the proportion of cases to controls.
Finally, a dendrogram and circle graph were used to display the selected model based on information theory (Moore et al., 2006). All interactions between SNPs show a percentage of the entropy risk. A positive percentage score was designated as synergistic interaction, while a score of 0 or below was designated redundant or antagonistic. The better model showed that paired percentage score is greater than the individual score (Hahn et al., 2003). All genetic models were adjusted for age, sex, BMI, glucose, cholesterol, and triglycerides.
Results
Subject characteristics
The frequency of women was higher in both groups; however, there was no significant difference. Participants with dementia were older as compared to the control group (76.9 ± 7.9 vs. 71.5 ± 7.8, p < 0.001).
There were significant differences between the dementia patients and controls regarding biochemical indicators. They showed higher glucose levels of 110 (95–148), total cholesterol of 247.0 ± 31.6, HDL-c of 48.0 ± 9.8, and triglycerides of 167 (132–240), all with p < 0.001 as shown in Table 2.
Association between dementia and the polymorphisms
The genotypic and allelic frequency distributions of each polymorphism are shown in Table 3. We found that IL-1a rs1800587 is associated with the susceptibility to developing dementia (OR = 1.55, 95%; CI = 1.20–2.00; p = 0.001). Similarly, IL-6 rs1800796 showed a risk effect on the susceptibility to dementia under the additive model (OR = 2.066, 95%; CI = 1.51–2.82; p = 0.001). In TNF-α, the strongest association was found using a recessive model with rs361525 (OR = 3.16, 95%; CI = 2.10–8.08; p < 0.001) and rs1800629 (OR = 4.39, 95%; CI = 2.10–9.02; p < 0.001). Finally, IFN-γ rs2069705 showed a protective effect on the susceptibility to dementia in subjects with the G allele vs. carriers of the A allele, under an overdominant inheritance model (OR = 0.59, 95%; CI = 0.43–0.81; p = 0.001). The models were independent of the Hardy–Weinberg equilibrium. Additionally, models were adjusted for the variables which were significantly different between groups and had been linked to dementia in previous studies.
Gene–gene interactions according to multifactor-dimensionality reduction analysis
The MDR identified the interaction between TNF-α rs1800629, TNF-α rs361525, IFNG rs2069705, IL1-a rs1800587, and IL6 rs1800796 as the best prediction model with an accuracy of 72.2%, a significance of p < 0.0001, and cross-validation of 10/10. The models with 2, 3, and 4 interactions showed a decrease in precision, with statistical significance. Only the five-locus model gave the highest sensitivity and specificity. Table 4 shows the results for each SNP.
We could see from the circle diagram information scores that the TNF-rs361525 percentage score (3.18%) was the most important among all gene variants in the study. IFN-γ-rs2069705 and TNF-rs361525 SNPs’ scores, shown in red for the interaction between the two, proved to be more significant than the individual contributions of each (Figure 1).
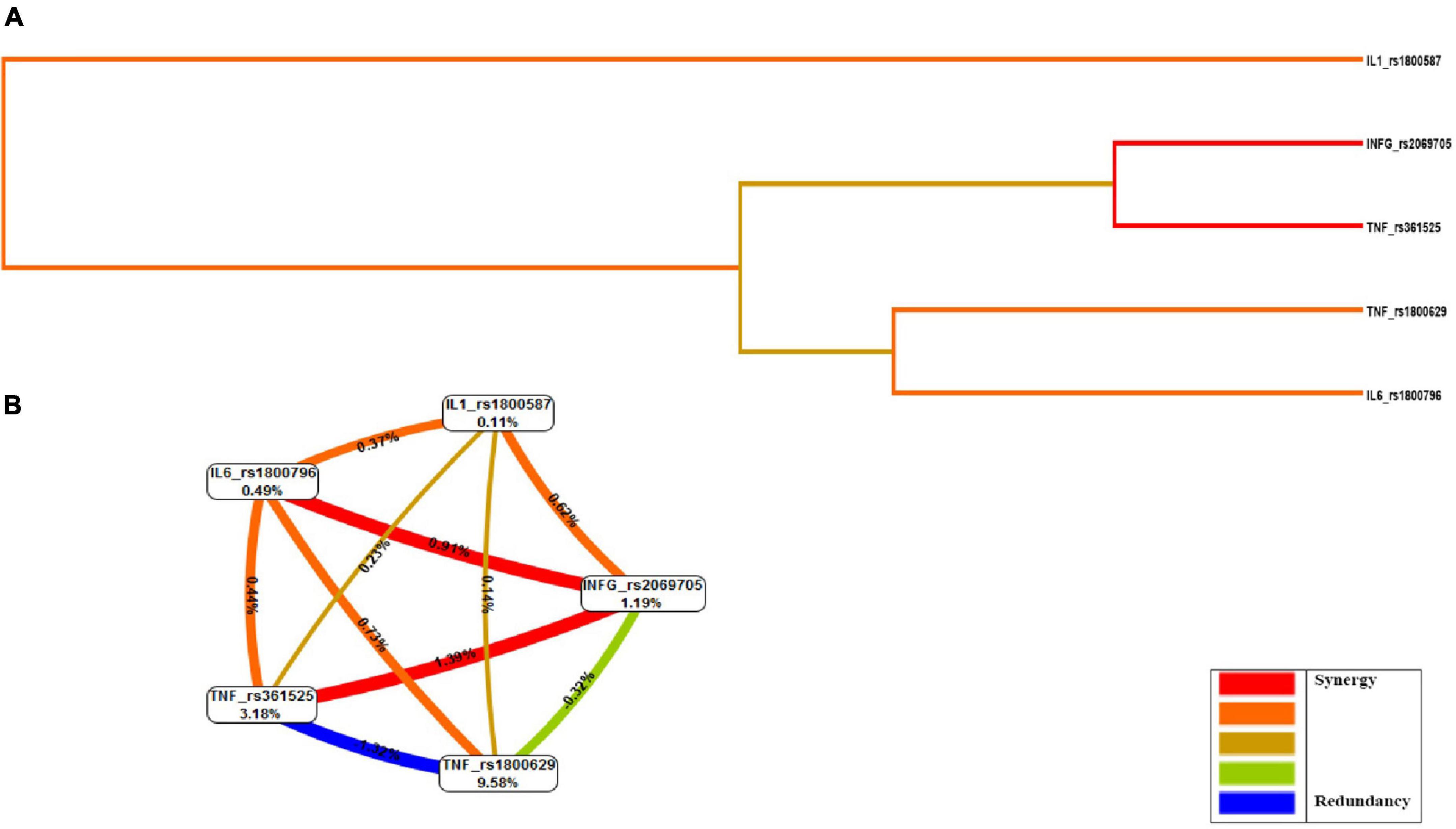
Figure 1. Evaluation of gene–gene interactions: multifactorial dimensionality reduction analysis (MDR). (A) Dendrogram, (B) Fruchterman-Rheingold graph. MDR combined attribute network was calculated. The interaction I (A; C) is represented as a percentage (%) between the five vertices. This graphical model explains the percent of the entropy in case-control removed by each factor (independent effect) and by each pair-wise combination of attributes (interaction effect). A positive percentage of entropy indicates synergistic interaction and negative values of entropy represent redundancy. The red color indicates a high degree of synergistic interaction, orange indicates a lesser degree, whereas gold represents the midpoint; blue represents the highest level of redundancy, followed by green.
Of the estimated genotypic pools, individuals with homozygous alleles for the IL-1α (rs1800587), IL-6 (rs1800796), TNFα (rs361525), and IFNγ (rs2069705) genes were identified in the high-risk group for dementia. The model was considered high risk when the ratio of the percentage of cases vs. controls was greater than 1.05. Fourteen genotype combinations resulted in a significantly high risk for dementia (Figure 2).
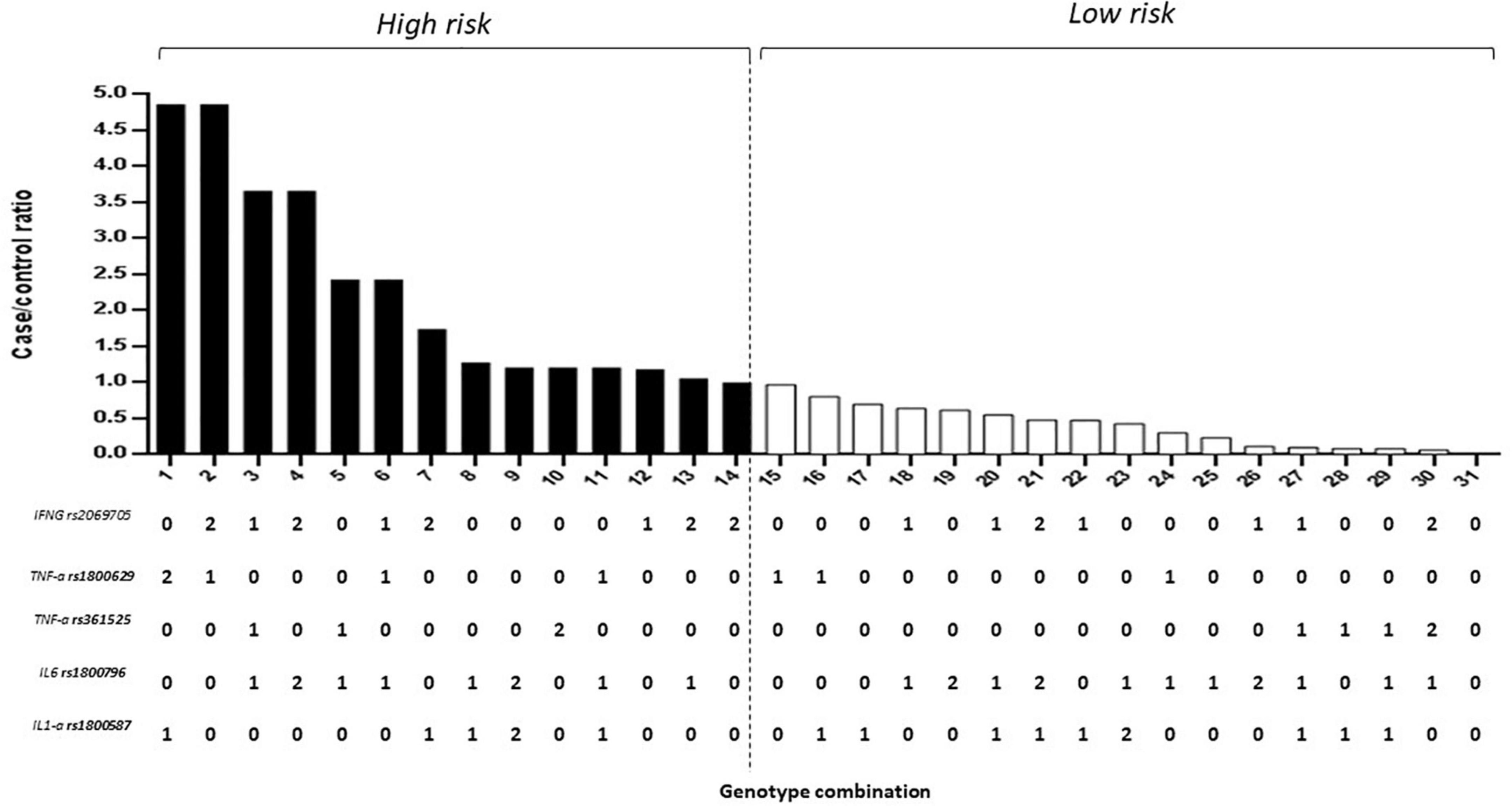
Figure 2. Five-locus gene–gene interaction between IL-1α (rs1800587), IL-6 (rs1800796), TNFα (rs361525 and rs1800629), and IFNγ (rs2069705) for dementia. The bar graph shows the validation of high and low-risk classifications. Genotype coding is as follows: 0, homozygous common allele; 1, heterozygous; and 2, homozygous variant allele.
The combination that gave the highest risk (5-fold) was the haplotype heterozygote for the IL-6 (rs1800796), TNFα (rs361525), IFNγ (rs2069705), heterozygous IL-1α (rs1800587), and homozygous variant allele TNFα (rs1800629). Similarly, the combination of heterozygote for the IL-1α (rs1800587), IL-6 (rs1800796), and TNFα (rs361525) with heterozygous for TNFα (rs1800629) and the homozygous variant allele for IFNγ (rs2069705) with risks from 4.90.
When including in the analysis the MDR (gene-environment interaction) of the risk factors age, sex, BMI, glucose, cholesterol, and triglycerides, the best prediction model (p < 0.0001) maintained the same interaction model.
Discussion
Dementia is a multifactorial disease; therefore, it is necessary to propose risk prediction models that consider both genetic and environmental aspects. The MDR analysis has already been used in association studies of other complex diseases such as diabetes, hypertension, and coronary heart disease, among others (Moore and Williams, 2002; Cho et al., 2004). The MDR allows us to detect and characterize patterns of epistasis in the genetic association (Cordell, 2009).
Previously, we reported the possible association of the IL10 (−1,082 and −819) polymorphisms in conferring dementia in a Mexica population (Vargas-Alarcon et al., 2016). In this study, the independent association between the genotypes (TNF-α rs1800629, TNF-α rs361525, IFNG rs2069705, IL1-a rs1800587, and IL6 rs1800796) was confirmed in the multilocus epistasis analysis, which may indicate that, at least in this population, the combination of these polymorphisms could constitute a genetic risk marker for the development of dementia. Previous studies have evaluated the association between some single-nucleotide polymorphisms (SNPs) in inflammatory cytokine genes and susceptibility to dementia, but the results are not conclusive. Some studies have found a significant association and others have not (Ravaglia et al., 2006; Di Bona et al., 2009; Swardfager et al., 2010; Hua et al., 2012; Toral-Rios et al., 2015; Sathyan et al., 2017). These inconsistencies can be attributed to the small effect of polymorphism on the disease, the small sample sizes, and the methodologies used.
From the pathophysiological point of view, the relationship between cytokines and dementia is established. The overexpressed IL-1 restrains the function of cholinergic systems (Li et al., 2000) and favors the formation of Aβ plaques and accumulation of neurofibrillary tangles (Sheng et al., 2001; Griffin and Mrak, 2002). This has been supported by gene association analysis. The relationship between the IL1A-889 C/T polymorphism, with dementia, has been tested in different ethnic groups from different regions, and the results vary (Combarros et al., 2002, 2003). Our study identifies the allele of IL1-α rs1800587 associated with the risk of dementia, which can facilitate the neuroinflammatory processes in the pathology.
Associations between dementia and IL-6 suggest that overexpressed IL-6 influences the cdk5/p53 pathway to induce the phosphorylation of tau, increasing the dementia risk (Quintanilla et al., 2004). Regarding the polymorphisms of the IL-6 gene, its protective role has been highlighted (Fishman et al., 1998; Darweesh et al., 2018). However, inconsistent results have also been reported (Fishman et al., 1998). Several meta-analyses have suggested that the CC genotype is likely a protective factor for dementia (van Oijen et al., 2006; Qi et al., 2012). Our finding supports a protective effect of the C allele in the IL-6 gene on dementia risk, which can favor the liberation of IL-6 protein release in the brain, playing an important role in the neuroinflammatory cascade and the pathology.
Increased TNF-α increases the Aβ and tau pathology and mediates the microglial phagocytosis of Aβ (Janelsins et al., 2008; Montgomery et al., 2011). Concerning genetic polymorphisms, some SNPs have been reported to influence the risk of dementia. A meta-analysis supported the association between the TNF-α rs361525 polymorphism and dementia risk involving different ethnic groups (Manoochehri et al., 2009; Ribizzi et al., 2010; Flex et al., 2014). Our study also identified the presence of the allele G in this polymorphism with a significantly increased risk of dementia. This genetic variant could modulate TNF-α expression and promote Aβ deposits which, in consequence, induce neuronal death (Di Bona et al., 2009).
The interferon (Abbas et al., 2002) activated by brain microglia exacerbates neurodegeneration, is neuroprotective, and helps clear neuronal debris and Aβ. Recently, some alleles in the first intron of the IFN-γ gene are associated with a higher level of cytokine production in vitro (Yamamoto et al., 2007). Our results showed a protective effect for dementia with the dominant over a model for the G allele, complementing existing information on the role of IFN-γ in neurodegeneration.
In Mexico, less has been explored about the relationship of the genes’ pro-inflammatory cytokines released from activated microglia (IL-1α, IL-6, TNFα, and IFNγ) with dementia. In our study, four of the polymorphisms studied showed an independent risk associated with dementia. However, in the results of the multilocus analysis, we could consider that the genes studied are not sufficient but necessary for the development of dementia, at least in this population. In this sense, MDR analysis can be useful as an analytical strategy to investigate the simultaneous effects of different loci and thus detect risk relationships with complex diseases, such as dementia.
Regarding the epistasis analysis, the MDR identified the interaction between the IL-6 (rs1800796), TNFα (rs361525), and IFNγ (rs2069705) polymorphisms as the best model. In contrast to the high- and low-risk genotypes obtained, we can point out that the polymorphisms studied can act synergistically: subjects carrying the genotypic combination will have a greater susceptibility to developing dementia, while those who do not present this combination could be protected (Figure 3).
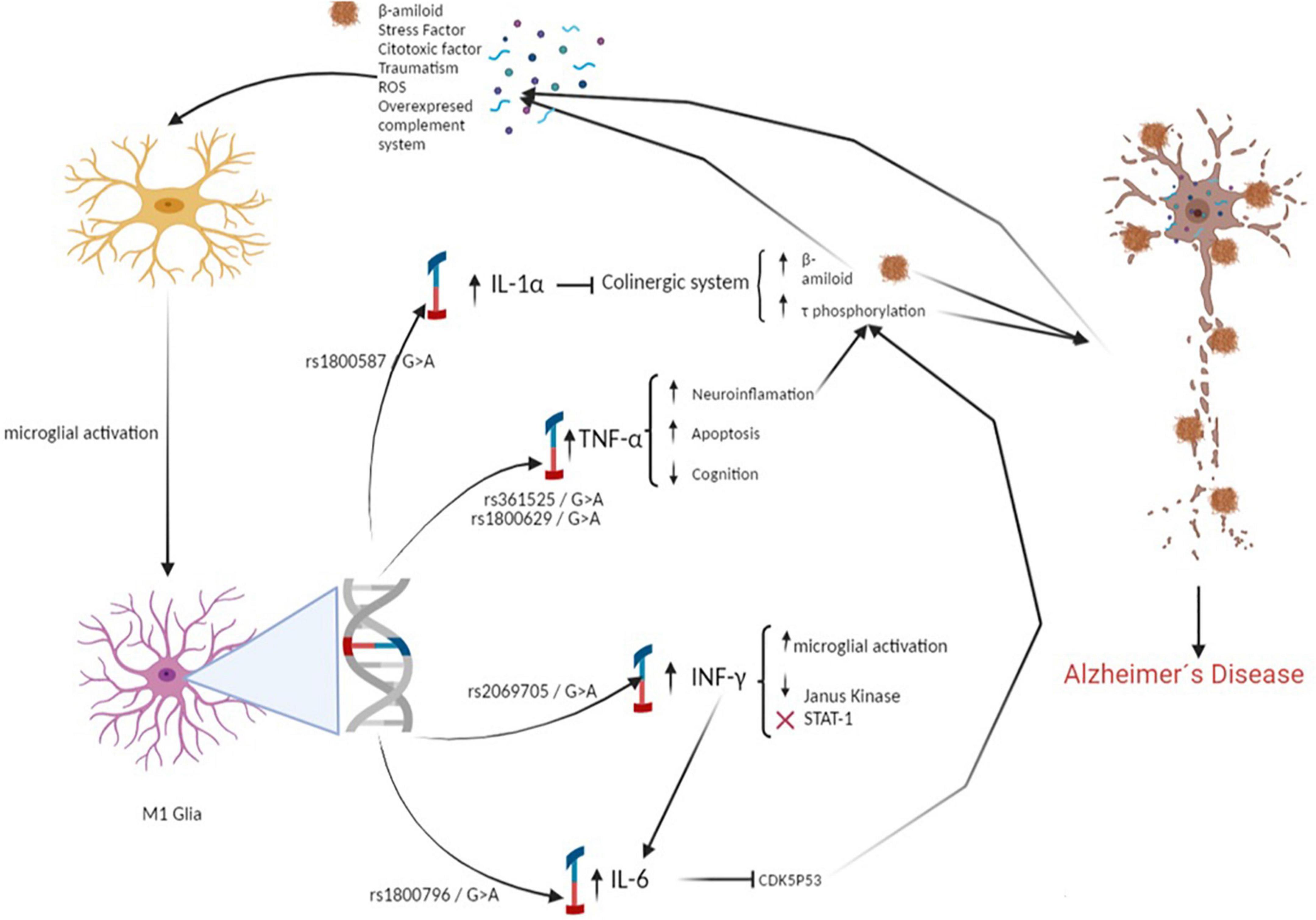
Figure 3. Polymorphisms associated with the development of dementia increases the proliferation of β-amyloid and promotes Tau hyperphosphorylation. Causing a pathological increase in the inflammatory response, which generates neuroinflammation and neuronal death.
A possible explanation for the combined effect of these SNPs could be in the relationship that the enzymes encoded by these genes have in the immunological pathways involved in protection against neurotoxicity, thus contributing to tau phosphorylation and accumulation of Aβ deposits, which, in consequence, induces neuronal death (Di Bona et al., 2009).
Another explanation could be that the combination likely affects the transcriptional efficiency of these cytokines by modifying their activity levels and promoting the circulation of inflammatory proteins in the body. This could lead to increased Aβ deposits and a decrease in the elimination of this protein in the brain.
The difference between our results and others previously reported for these polymorphisms can be explained by the ethnic characteristics of the populations. This could lay the groundwork for new therapeutic targets based on ethnic characteristics.
Limitations
This study only analyzed the SNPs that had previously been reported. Thus, other SNPs can potentially show genetic interactions and cause dementia. Other studies to characterize the interactions with other SNPs are necessary.
Overall, our model was based on the integration of both main effects and epistasis to increase the predictive value of genetic risk. Finally, a more detailed analysis should be conducted in other populations to evaluate the effect of race on this relationship.
Conclusion
The genetic contributions to the susceptibility to dementia were visualized thanks to computational techniques. We identified the strongest interactions between combinations of SNPs (IL-6 rs1800796, TNFα rs361525, and IFNγ rs2069705) associated with the risk of suffering from dementia. Furthermore, we identified and characterized patterns of epistasis in the genetic association between dementia and cytokines through the algorithm, including MDR. In combination with clinical factors, our findings can potentially help to better understand the pathophysiology of dementias and confirm the role cytokines seem to play in the predisposition and development of the disease.
Data availability statement
The original contributions presented in this study are included in the article/supplementary material, further inquiries can be directed to the corresponding author.
Ethics statement
The studies involving human participants were reviewed and approved by the National Commission of Scientific Research, as well as by the IMSS Ethics Commission. The patients/participants provided their written informed consent to participate in this study.
Author contributions
TJ-C participated in the conceptualization, acquisition of funds, and wrote and edited the manuscript. NM-R participated in the methodology, analysis, and wrote the manuscript. GV-A participated in the methodology and analysis. EJ-C and OG-A participated in the methodology and supervision of the work. AV-M participated in the supervision and analysis. AR participated in the investigation and reviewed and edited the manuscript. All authors contributed to the article and approved the submitted version.
Funding
This project was supported by grants from SSA/IMSS/ISSSTE-CONACYT (Mexico) SALUD-233065.
Conflict of interest
The authors declare that the research was conducted in the absence of any commercial or financial relationships that could be construed as a potential conflict of interest.
Publisher’s note
All claims expressed in this article are solely those of the authors and do not necessarily represent those of their affiliated organizations, or those of the publisher, the editors and the reviewers. Any product that may be evaluated in this article, or claim that may be made by its manufacturer, is not guaranteed or endorsed by the publisher.
Footnotes
References
Abbas, N., Bednar, I., Mix, E., Marie, S., Paterson, D., Ljungberg, A., et al. (2002). Up-regulation of the inflammatory cytokines IFN-gamma and IL-12 and down-regulation of IL-4 in cerebral cortex regions of APP(SWE) transgenic mice. J. Neuroimmunol. 126, 50–57. doi: 10.1016/s0165-5728(02)00050-4
Ardebili, S. M., Yeghaneh, T., Gharesouran, J., Rezazadeh, M., Farhoudi, M., Ayromlou, H., et al. (2011). Genetic association of TNF-alpha-308 G/A and -863 C/A polymorphisms with late onset Alzheimer’s disease in azeri turk population of Iran. J. Res. Med. Sci. 16, 1006–1013.
Bertram, L. (2009). Alzheimer’s disease genetics current status and future perspectives. Int. Rev. Neurobiol. 84, 167–184. doi: 10.1016/S0074-7742(09)00409-7
Chakrabarty, P., Jansen-West, K., Beccard, A., Ceballos-Diaz, C., Levites, Y., Verbeeck, C., et al. (2010). Massive gliosis induced by interleukin-6 suppresses abeta deposition in vivo: Evidence against inflammation as a driving force for amyloid deposition. FASEB J. 24, 548–559. doi: 10.1096/fj.09-141754
Cho, Y. M., Ritchie, M. D., Moore, J. H., Park, J. Y., Lee, K. U., Shin, H. D., et al. (2004). Multifactor-dimensionality reduction shows a two-locus interaction associated with type 2 diabetes mellitus. Diabetologia 47, 549–554. doi: 10.1007/s00125-003-1321-3
Combarros, O., Llorca, J., Sanchez-Guerra, M., Infante, J., and Berciano, J. (2003). Age-dependent association between interleukin-1A (-889) genetic polymorphism and sporadic Alzheimer’s disease. A meta-analysis. J. Neurol. 250, 987–989. doi: 10.1007/s00415-003-1136-7
Combarros, O., Sanchez-Guerra, M., Infante, J., Llorca, J., and Berciano, J. (2002). Gene dose-dependent association of interleukin-1A [-889] allele 2 polymorphism with Alzheimer’s disease. J. Neurol. 249, 1242–1245. doi: 10.1007/s00415-002-0819-9
Cordell, H. J. (2009). Detecting gene-gene interactions that underlie human diseases. Nat. Rev. Genet. 10, 392–404. doi: 10.1038/nrg2579
da Cruz e Silva, O. A., Henriques, A. G., Domingues, S. C., and da Cruz e Silva, E. F. (2010). Wnt signalling is a relevant pathway contributing to amyloid beta- peptide-mediated neuropathology in Alzheimer’s disease. CNS Neurol. Disord. Drug Targets 9, 720–726. doi: 10.2174/187152710793237458
Darweesh, S. K. L., Wolters, F. J., Ikram, M. A., de Wolf, F., Bos, D., and Hofman, A. (2018). Inflammatory markers and the risk of dementia and Alzheimer’s disease: A meta-analysis. Alzheimers Dement 14, 1450–1459. doi: 10.1016/j.jalz.2018.02.014
Di Bona, D., Candore, G., Franceschi, C., Licastro, F., Colonna-Romano, G., Camma, C., et al. (2009). Systematic review by meta-analyses on the possible role of TNF-alpha polymorphisms in association with Alzheimer’s disease. Brain Res. Rev. 61, 60–68. doi: 10.1016/j.brainresrev.2009.05.001
Fishman, D., Faulds, G., Jeffery, R., Mohamed-Ali, V., Yudkin, J. S., Humphries, S., et al. (1998). The effect of novel polymorphisms in the interleukin-6 (IL-6) gene on IL-6 transcription and plasma IL-6 levels, and an association with systemic-onset juvenile chronic arthritis. J. Clin. Invest. 102, 1369–1376. doi: 10.1172/JCI2629
Fleming, A., Bourdenx, M., Fujimaki, M., Karabiyik, C., Krause, G. J., Lopez, A., et al. (2022). The different autophagy degradation pathways and neurodegeneration. Neuron 110, 935–966. doi: 10.1016/j.neuron.2022.01.017
Flex, A., Giovannini, S., Biscetti, F., Liperoti, R., Spalletta, G., Straface, G., et al. (2014). Effect of proinflammatory gene polymorphisms on the risk of Alzheimer’s disease. Neurodegener. Dis. 13, 230–236. doi: 10.1159/000353395
Genomes Project, C., Abecasis, G. R., Auton, A., Brooks, L. D., DePristo, M. A., Durbin, R. M., et al. (2012). An integrated map of genetic variation from 1,092 human genomes. Nature 491, 56–65. doi: 10.1038/nature11632
Gillespie, N. A., Hatton, S. N., Hagler, D. J. Jr., Dale, A. M., Elman, J. A., McEvoy, L. K., et al. (2022). The impact of genes and environment on brain ageing in males aged 51 to 72 years. Front. Aging Neurosci. 14:831002. doi: 10.3389/fnagi.2022.831002
Griffin, W. S., and Mrak, R. E. (2002). Interleukin-1 in the genesis and progression of and risk for development of neuronal degeneration in Alzheimer’s disease. J. Leukoc. Biol. 72, 233–238.
Gutierrez-Robledo, L. M., and Arrieta-Cruz, I. (2015). [Dementia in Mexico: The need for a national Alzheimer s plan]. Gac. Med. Mex. 151, 667–673.
Hahn, L. W., Ritchie, M. D., and Moore, J. H. (2003). Multifactor dimensionality reduction software for detecting gene-gene and gene-environment interactions. Bioinformatics 19, 376–382. doi: 10.1093/bioinformatics/btf869
Harman, D. (2006). Alzheimer’s disease pathogenesis: Role of aging. Ann. N. Y. Acad. Sci. 1067, 454–460. doi: 10.1196/annals.1354.065
Heneka, M. T., Carson, M. J., El Khoury, J., Landreth, G. E., Brosseron, F., Feinstein, D. L., et al. (2015). Neuroinflammation in Alzheimer’s disease. Lancet Neurol. 14, 388–405. doi: 10.1016/S1474-4422(15)70016-5
Henriques, A. G., Oliveira, J. M., Carvalho, L. P., and da Cruz E Silva, O. A. B. (2015). Abeta influences cytoskeletal signaling cascades with consequences to Alzheimer’s Disease. Mol. Neurobiol. 52, 1391–1407. doi: 10.1007/s12035-014-8913-4
Hesse, R., Wahler, A., Gummert, P., Kirschmer, S., Otto, M., Tumani, H., et al. (2016). Decreased IL-8 levels in CSF and serum of AD patients and negative correlation of MMSE and IL-1beta. BMC Neurol. 16:185. doi: 10.1186/s12883-016-0707-z
Hua, Y., Zhao, H., Kong, Y., and Lu, X. (2012). Meta-analysis of the association between the interleukin-1A -889C/T polymorphism and Alzheimer’s disease. J. Neurosci. Res. 90, 1681–1692. doi: 10.1002/jnr.23062
Hughes, C. P., Berg, L., Danziger, W. L., Coben, L. A., and Martin, R. L. (1982). A new clinical scale for the staging of dementia. Br. J. Psychiatry 140, 566–572. doi: 10.1192/bjp.140.6.566
Janelsins, M. C., Mastrangelo, M. A., Park, K. M., Sudol, K. L., Narrow, W. C., Oddo, S., et al. (2008). Chronic neuron-specific tumor necrosis factor-alpha expression enhances the local inflammatory environment ultimately leading to neuronal death in 3xTg-AD mice. Am. J. Pathol. 173, 1768–1782. doi: 10.2353/ajpath.2008.080528
Juarez-Cedillo, T., Sanchez-Arenas, R., Sanchez-Garcia, S., Garcia-Pena, C., Hsiung, G. Y., Sepehry, A. A., et al. (2012). Prevalence of mild cognitive impairment and its subtypes in the Mexican population. Dement Geriatr. Cogn. Disord. 34, 271–281. doi: 10.1159/000345251
Karch, C. M., and Goate, A. M. (2015). Alzheimer’s disease risk genes and mechanisms of disease pathogenesis. Biol. Psychiatry 77, 43–51. doi: 10.1016/j.biopsych.2014.05.006
Lahiri, D. K., and Nurnberger, J. I. Jr. (1991). A rapid non-enzymatic method for the preparation of HMW DNA from blood for RFLP studies. Nucleic Acids Res. 19:5444. doi: 10.1093/nar/19.19.5444
Li, Y., Liu, L., Kang, J., Sheng, J. G., Barger, S. W., Mrak, R. E., et al. (2000). Neuronal-glial interactions mediated by interleukin-1 enhance neuronal acetylcholinesterase activity and mRNA expression. J. Neurosci. 20, 149–155. doi: 10.1523/JNEUROSCI.20-01-00149.2000
Manoochehri, M., Kamali, K., Rahgozar, M., Ohadi, M., Farrokhi, H., and Khorshid, H. R. (2009). Lack of association between tumor necrosis factor-alpha -308 G/A polymorphism and risk of developing late-onset Alzheimer’s disease in an Iranian population. Avicenna. J. Med. Biotechnol. 1, 193–197.
McKhann, G., Drachman, D., Folstein, M., Katzman, R., Price, D., and Stadlan, E. M. (1984). Clinical diagnosis of Alzheimer’s disease: Report of the NINCDS-ADRDA work group under the auspices of department of health and human services task force on Alzheimer’s disease. Neurology 34, 939–944. doi: 10.1212/wnl.34.7.939
Montgomery, S. L., Mastrangelo, M. A., Habib, D., Narrow, W. C., Knowlden, S. A., Wright, T. W., et al. (2011). Ablation of TNF-RI/RII expression in Alzheimer’s disease mice leads to an unexpected enhancement of pathology: Implications for chronic pan-TNF-alpha suppressive therapeutic strategies in the brain. Am. J. Pathol. 179, 2053–2070. doi: 10.1016/j.ajpath.2011.07.001
Moore, J. H., and Williams, S. M. (2002). New strategies for identifying gene-gene interactions in hypertension. Ann. Med. 34, 88–95. doi: 10.1080/07853890252953473
Moore, J. H., Gilbert, J. C., Tsai, C. T., Chiang, F. T., Holden, T., Barney, N., et al. (2006). A flexible computational framework for detecting, characterizing, and interpreting statistical patterns of epistasis in genetic studies of human disease susceptibility. J. Theor. Biol. 241, 252–261. doi: 10.1016/j.jtbi.2005.11.036
Nazarian, A., Yashin, A. I., and Kulminski, A. M. (2019). Genome-wide analysis of genetic predisposition to Alzheimer’s disease and related sex disparities. Alzheimers Res. Ther. 11:5. doi: 10.1186/s13195-018-0458-8
O’Bryant, S. E., Lista, S., Rissman, R. A., Edwards, M., Zhang, F., Hall, J., et al. (2016). Comparing biological markers of Alzheimer’s disease across blood fraction and platforms: Comparing apples to oranges. Alzheimers Dement 3, 27–34. doi: 10.1016/j.dadm.2015.12.003
Oda, M., Morino, H., Maruyama, H., Terasawa, H., Izumi, Y., Torii, T., et al. (2002). Dinucleotide repeat polymorphisms in the neprilysin gene are not associated with sporadic Alzheimer’s disease. Neurosci. Lett. 320, 105–107. doi: 10.1016/s0304-3940(02)00057-5
Olsson, B., Lautner, R., Andreasson, U., Ohrfelt, A., Portelius, E., Bjerke, M., et al. (2016). CSF and blood biomarkers for the diagnosis of Alzheimer’s disease: A systematic review and meta-analysis. Lancet Neurol. 15, 673–684. doi: 10.1016/S1474-4422(16)00070-3
Qi, H. P., Qu, Z. Y., Duan, S. R., Wei, S. Q., Wen, S. R., and Bi, S. (2012). IL-6-174 G/C and -572 C/G polymorphisms and risk of Alzheimer’s disease. PLoS One 7:e37858. doi: 10.1371/journal.pone.0037858
Quintanilla, R. A., Orellana, D. I., Gonzalez-Billault, C., and Maccioni, R. B. (2004). Interleukin-6 induces Alzheimer-type phosphorylation of tau protein by deregulating the cdk5/p35 pathway. Exp. Cell Res. 295, 245–257. doi: 10.1016/j.yexcr.2004.01.002
Ravaglia, G., Paola, F., Maioli, F., Martelli, M., Montesi, F., Bastagli, L., et al. (2006). Interleukin-1beta and interleukin-6 gene polymorphisms as risk factors for AD: A prospective study. Exp. Gerontol. 41, 85–92. doi: 10.1016/j.exger.2005.10.005
Ribizzi, G., Fiordoro, S., Barocci, S., Ferrari, E., and Megna, M. (2010). Cytokine polymorphisms and Alzheimer disease: Possible associations. Neurol. Sci. 31, 321–325. doi: 10.1007/s10072-010-0221-9
Ritchie, M. D., Hahn, L. W., and Moore, J. H. (2003). Power of multifactor dimensionality reduction for detecting gene-gene interactions in the presence of genotyping error, missing data, phenocopy, and genetic heterogeneity. Genet. Epidemiol. 24, 150–157. doi: 10.1002/gepi.10218
Romano, P. S., Roos, L. L., and Jollis, J. G. (1993). Adapting a clinical comorbidity index for use with ICD-9-CM administrative data: Differing perspectives. J. Clin. Epidemiol. 46, 1075–1079; discussion 1081–1090. doi: 10.1016/0895-4356(93)90103-8
Rothwell, N. J., and Luheshi, G. N. (2000). Interleukin 1 in the brain: Biology, pathology and therapeutic target. Trends Neurosci. 23, 618–625. doi: 10.1016/s0166-2236(00)01661-1
Rubio-Perez, J. M., and Morillas-Ruiz, J. M. (2012). A review: Inflammatory process in Alzheimer’s disease, role of cytokines. ScientificWorldJournal 2012:756357. doi: 10.1100/2012/756357
Ryckman, K., and Williams, S. M. (2008). Calculation and use of the hardy-weinberg model in association studies. Curr. Protoc. Hum. Genet. 1:1.18. doi: 10.1002/0471142905.hg0118s57
Sathyan, S., Barzilai, N., Atzmon, G., Milman, S., Ayers, E., and Verghese, J. (2017). Association of anti-inflammatory cytokine IL10 polymorphisms with motoric cognitive risk syndrome in an ashkenazi jewish population. Neurobiol. Aging 58, 238.e1–238.e8. doi: 10.1016/j.neurobiolaging.2017.06.006
Scott, W. K., Grubber, J. M., Conneally, P. M., Small, G. W., Hulette, C. M., Rosenberg, C. K., et al. (2000). Fine mapping of the chromosome 12 late-onset Alzheimer disease locus: Potential genetic and phenotypic heterogeneity. Am. J. Hum. Genet. 66, 922–932. doi: 10.1086/302828
Shawkatova, I., Javor, J., Parnicka, Z., Vrazda, L., Novak, M., and Buc, M. (2010). No association between cytokine gene polymorphism and risk of Alzheimer’s disease in slovaks. Acta Neurobiol. Exp. 70, 303–307.
Sheng, J. G., Jones, R. A., Zhou, X. Q., McGinness, J. M., Van Eldik, L. J., Mrak, R. E., et al. (2001). Interleukin-1 promotion of MAPK-p38 overexpression in experimental animals and in Alzheimer’s disease: Potential significance for tau protein phosphorylation. Neurochem. Int. 39, 341–348. doi: 10.1016/s0197-0186(01)00041-9
Skol, A. D., Scott, L. J., Abecasis, G. R., and Boehnke, M. (2006). Joint analysis is more efficient than replication-based analysis for two-stage genome-wide association studies. Nat. Genet. 38, 209–213. doi: 10.1038/ng1706
Swardfager, W., Lanctot, K., Rothenburg, L., Wong, A., Cappell, J., and Herrmann, N. (2010). A meta-analysis of cytokines in Alzheimer’s disease. Biol. Psychiatry 68, 930–941. doi: 10.1016/j.biopsych.2010.06.012
Toral-Rios, D., Franco-Bocanegra, D., Rosas-Carrasco, O., Mena-Barranco, F., Carvajal-Garcia, R., Meraz-Rios, M. A., et al. (2015). Evaluation of inflammation-related genes polymorphisms in Mexican with Alzheimer’s disease: A pilot study. Front. Cell Neurosci. 9:148. doi: 10.3389/fncel.2015.00148
van Oijen, M., Arp, P. P., de Jong, F. J., Hofman, A., Koudstaal, P. J., Uitterlinden, A. G., et al. (2006). Polymorphisms in the interleukin 6 and transforming growth factor beta1 gene and risk of dementia. The rotterdam study. Neurosci. Lett. 402, 113–117. doi: 10.1016/j.neulet.2006.03.050
Vargas-Alarcon, G., Juarez-Cedillo, E., Martinez-Rodriguez, N., Fragoso, J. M., Garcia-Hernandez, N., and Juarez-Cedillo, T. (2016). Association of interleukin-10 polymorphisms with risk factors of Alzheimer’s disease and other dementias (sadem study). Immunol. Lett. 177, 47–52. doi: 10.1016/j.imlet.2016.07.011
Yamamoto, M., Kiyota, T., Horiba, M., Buescher, J. L., Walsh, S. M., Gendelman, H. E., et al. (2007). Interferon-gamma and tumor necrosis factor-alpha regulate amyloid-beta plaque deposition and beta-secretase expression in Swedish mutant APP transgenic mice. Am. J. Pathol. 170, 680–692. doi: 10.2353/ajpath.2007.060378
Zekanowski, C., and Wojda, U. (2009). Aneuploidy, chromosomal missegregation, and cell cycle reentry in Alzheimer’s disease. Acta Neurobiol. Exp. 69, 232–253.
Zheng, C., Zhou, X. W., and Wang, J. Z. (2016). The dual roles of cytokines in Alzheimer’s disease: Update on interleukins, TNF-alpha, TGF-beta and IFN-gamma. Transl. Neurodegener. 5:7. doi: 10.1186/s40035-016-0054-4
Zheng, X., Wu, Y., Bi, J., Huang, Y., Cheng, Y., Li, Y., et al. (2022). The use of supercytokines, immunocytokines, engager cytokines, and other synthetic cytokines in immunotherapy. Cell Mol. Immunol. 19, 192–209. doi: 10.1038/s41423-021-00786-6
Keywords: polymorphism, cytokine genes, dementia, MDR, gene–gene interaction
Citation: Juárez-Cedillo T, Martínez-Rodríguez N, Vargas-Alarcon G, Juárez-Cedillo E, Valle-Medina A, Garrido-Acosta O and Ramirez A (2022) Synergistic influence of cytokine gene polymorphisms over the risk of dementia: A multifactor dimensionality reduction analysis. Front. Aging Neurosci. 14:952173. doi: 10.3389/fnagi.2022.952173
Received: 24 May 2022; Accepted: 03 October 2022;
Published: 26 October 2022.
Edited by:
Ines Moreno-Gonzalez, University of Malaga, SpainReviewed by:
Stephen John Ralph, Griffith University, AustraliaCarlos Sánchez Garibay, Manuel Velasco Suárez Instituto Nacional de Neurología y Neurocirugía, Mexico
Manuel Vicente Camacho, Case Western Reserve University, United States
Copyright © 2022 Juárez-Cedillo, Martínez-Rodríguez, Vargas-Alarcon, Juárez-Cedillo, Valle-Medina, Garrido-Acosta and Ramirez. This is an open-access article distributed under the terms of the Creative Commons Attribution License (CC BY). The use, distribution or reproduction in other forums is permitted, provided the original author(s) and the copyright owner(s) are credited and that the original publication in this journal is cited, in accordance with accepted academic practice. No use, distribution or reproduction is permitted which does not comply with these terms.
*Correspondence: Teresa Juárez-Cedillo, terezillo@exalumno.unam.mx