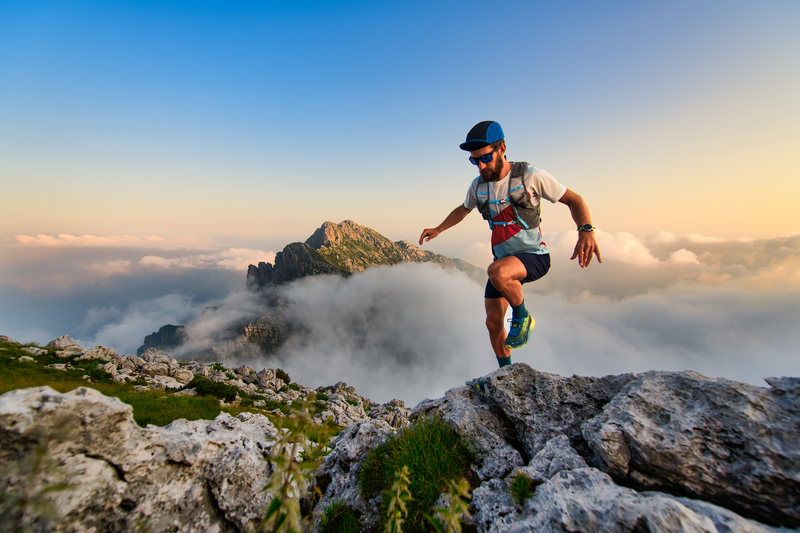
94% of researchers rate our articles as excellent or good
Learn more about the work of our research integrity team to safeguard the quality of each article we publish.
Find out more
ORIGINAL RESEARCH article
Front. Aging Neurosci. , 07 July 2022
Sec. Alzheimer's Disease and Related Dementias
Volume 14 - 2022 | https://doi.org/10.3389/fnagi.2022.949083
This article is part of the Research Topic Integrative Multi-Omics for Diagnosis, Treatments, and Drug Discovery of Aging-Related Neuronal Diseases View all 17 articles
Alzheimer's disease (AD), the most common neurodegenerative disease, remains unclear in terms of its underlying causative genes and effective therapeutic approaches. Meanwhile, abnormalities in iron metabolism have been demonstrated in patients and mouse models with AD. Therefore, this study sought to find hub genes based on iron metabolism that can influence the diagnosis and treatment of AD. First, gene expression profiles were downloaded from the GEO database, including non-demented (ND) controls and AD samples. Fourteen iron metabolism-related gene sets were downloaded from the MSigDB database, yielding 520 iron metabolism-related genes. The final nine hub genes associated with iron metabolism and AD were obtained by differential analysis and WGCNA in brain tissue samples from GSE132903. GO analysis revealed that these genes were mainly involved in two major biological processes, autophagy and iron metabolism. Through stepwise regression and logistic regression analyses, we selected four of these genes to construct a diagnostic model of AD. The model was validated in blood samples from GSE63061 and GSE85426, and the AUC values showed that the model had a relatively good diagnostic performance. In addition, the immune cell infiltration of the samples and the correlation of different immune factors with these hub genes were further explored. The results suggested that these genes may also play an important role in immunity to AD. Finally, eight drugs targeting these nine hub genes were retrieved from the DrugBank database, some of which were shown to be useful for the treatment of AD or other concomitant conditions, such as insomnia and agitation. In conclusion, this model is expected to guide the diagnosis of patients with AD by detecting the expression of several genes in the blood. These hub genes may also assist in understanding the development and drug treatment of AD.
Alzheimer's disease (AD), the most common form of dementia, is a neurodegenerative disease associated with aging. According to a report in 2020, there are an estimated 5.8 million people aged 65 and older with AD in the United States, and this number could increase to 13.8 million by the middle of this century (Zhang T. et al., 2021). Typical AD occurs after the age of 65 years, and <5% of AD cases occur earlier in life, among which 1–2% occur in familial clusters (Long and Holtzman, 2019). The pathology of this disease is characterized by amyloid plaques comprised of amyloid-β (Aβ) peptides and neurofibrillary tangles (NFTs) containing hyperphosphorylated tau proteins (DeTure and Dickson, 2019). Various drugs available to treat AD, such as donepezil, galantamine, memantine, rivastigmine, and aducanumab (also known as Aduhelm), are not very effective (Barthold et al., 2020; Mullard, 2021). Therefore, finding new effective molecules to improve the diagnosis and treatment of AD is urgent.
As the second most abundant metal on the Earth's crust after aluminum, iron is one of the trace metals essential for human beings. Iron is an important component of hemoglobin, which is involved in oxygen transport. Iron is also involved in the metabolism of catecholamine neurotransmitters and the formation of myelin sheaths in the nervous system (Thirupathi and Chang, 2019; Peng et al., 2021). Iron homeostasis is maintained by a variety of complex mechanisms, such as iron regulatory proteins and hepcidin (Pantopoulos et al., 2012). Disruption of this homeostasis leads to excessive accumulation of intracellular iron, which can damage DNA, proteins, and lipids through the production of oxidative stress and free radicals (Ward et al., 2014). Abnormal iron accumulation has been observed in different brain regions of patients with AD (Mills et al., 2010; Apostolakis and Kypraiou, 2017; Lee and Lee, 2019). In AD, excessive accumulation of iron in the brain aggravates amyloid protein deposition and tau protein hyperphosphorylation, which leads to neuronal damage and cognitive impairment (Gong et al., 2019; Yan and Zhang, 2019; Choi et al., 2021; Peng et al., 2021). Iron can also directly induce oxidative damage to neurons (Thirupathi and Chang, 2019). In conclusion, there is a non-negligible relationship between iron metabolism and AD.
Based on abundant public resources and bioinformatics methods, this study identified nine hub genes associated with iron metabolism and AD by differential analysis and WGCNA of GSE132903. GO and GSEA analyses were applied to further investigate the biological processes and pathways. Stepwise regression and logistic regression analyses were performed to screen four of them and construct a diagnostic model for AD, which was validated in two other blood sample datasets, GSE63061 and GSE85426. The AUC values indicated that this model has relatively good diagnostic performance and thus had a potential application in the clinical diagnosis of AD. Neuroinflammation, an important pathological feature of AD, is closely related to immunity. Therefore, we further explored the immune infiltration of these samples and the correlation of different immune factors with these hub genes. In addition, eight drugs targeting these hub genes were retrieved from the DrugBank database, which has implications for the pharmacological treatment of AD. The workflow of this study is shown in Figure 1.
Gene expression data were obtained from the NCBI Gene Expression Omnibus public database (GEO). GSE132903 contained RNA expression data annotated by GPL10558 in the middle temporal gyrus, which included 97 AD samples and 98 ND controls. Samples from two datasets GSE63061 and GSE85426 were extracted from peripheral blood. GSE63061 annotated by GPL10558 included 139 AD samples and 134 ND samples, and GSE85426 annotated by GPL14550 included 90 AD samples and 90 ND samples.
Differential expression analysis of AD and ND samples was performed using the “limma” package in R software. Genes with p_adj < 0.01 and abs(logFC) > 0.585 were considered as DEGs. Heat maps and volcano plots of DEGs were created using the “pheatmap” and “ggplot2” packages.
Fourteen iron metabolism-related gene sets were extracted from the Molecular Signatures Database v7.5.1 (MSigDB; Liberzon et al., 2015), including GOBP_CELLULAR_IRON_ION_HOMEOSTASIS, GOBP_HEME_METABOLIC_PROCESS, GOBP_IRON_COORDINATION_ENTITY_TRANSPORT, GOBP_IRON_ION_HOMEOSTASIS, GOBP_IRON_ION_IMPORT_ACROSS_PLASMA_MEMBRANE, GOBP_IRON_ION_TRANSPORT, GOBP_RESPONSE_TO_IRON_ION, GOMF_2_IRON_2_SULFUR_CLUSTER_BINDING, GOMF_4_IRON_4_SULFUR_CLUSTER_BINDING, GOMF_IRON_ION_BINDING, HALLMARK_HEME_METABOLISM, HEME_BIOSYNTHETIC_PROCESS, MODULE_540, and REACTOME_IRON_UPTAKE_AND_TRANSPORT (Mou et al., 2020). After removing the overlapping genes, the gene sets associated with iron metabolism contained 520 genes (Supplementary Table 1).
To explore the co-expression relationships among the genes and the relationship between the genes and the phenotypes, a gene co-expression network was constructed using the “WGCNA” package in R software (Langfelder and Horvath, 2008). Based on the cluster trees, abnormal samples were removed. The top 5,000 genes with a median absolute deviation (MAD) > 1 were retained. The correlation coefficient between each gene pair was calculated to construct a similarity matrix. To ensure the construction of a scale-free network, a suitable soft threshold was chosen to transform the similarity matrix into an adjacency matrix. Subsequently, a topological overlap matrix (TOM) was created to measure the mean network connectivity of each gene. Based on the relevant parameters of the blockwiseModules function, such as minModuleSize and mergeCutHeight, genes with similar expression profiles were grouped into different modules using the dynamic tree cutting method. Each module was depicted in a different color, where the genes in gray modules represented genes that cannot be assigned to any module. The gene expression profile of each module was represented by the first principal component called the module eigengene (ME). MEs were used to assess the association between the modules and phenotypes. The module with the highest absolute value of the correlation coefficient was identified as the key module for further analysis. Module membership (MM) is the correlation coefficient between the expression value of a gene and the ME of a module, representing the correlation between this gene and this module. Gene significance (GS) was the correlation coefficient between the expression value of a gene and a phenotype, representing the association between genes and phenotypes.
To obtain hub genes associated with both iron metabolism and AD, the intersection of DEGs, genes obtained by WGCNA, and genes in the iron metabolism gene sets were taken using the “VennDiagram” package in R software. Differences in the expressions of hub genes between the AD and ND samples were represented by violin plots. The hypothesis tests used were the t-test and the Mann-Whitney U-test. The former was used if the data conformed to a normal distribution, and the latter if not. Significance was defined as p < 0.05.
To investigate the biological mechanisms of the hub genes affecting AD, functional enrichment analyses were conducted. We first analyzed the biological processes (BP) of Gene Ontology (GO) in which these genes are involved, and the final results were presented in a chord diagram using the “GOplot” package in R software. Next, the respective functions of each gene were revealed by Gene Set Enrichment Analysis (GSEA). Samples were distinguished into two groups based on median values of hub gene expression levels, including the low expression group and the high expression group. Genes were sorted by logFC from the highest to the lowest, and the background gene sets were downloaded from MSigDB (Liberzon et al., 2015). The final results were presented using the “enrichplot” package in R software. All these analyses were conducted using the “clusterProfiler” package in R software, and the screening condition was p_adj <0.05.
Logistic regression is a generalized linear regression analysis model that can be used for the automatic diagnosis of diseases. In this study, logistic regression with two response variables was used, representing the AD sample when the response variable was 1 and the ND sample when it was 0. Stepwise regression analysis was first used to eliminate factors that were not significant for the response variable, and only those that were significant were retained to simplify the model. The stepwise regression iteratively added or removed variables from the model until the statistical value of Akaike information criterion (AIC) was minimized. Afterward, logistic regression was used to fit the relationship between these significant factors and the response variable. Finally, the diagnostic efficacy of the model was evaluated using receiver operating characteristic curves (ROCs) and the area under the ROC curve (Coat et al., 2015; Lai et al., 2021). These analyses were performed with the “stats” and “pROC” packages in R software.
Immune cell infiltration in the microenvironment was assessed using CIBERSORT, which contains 547 biomarkers and 22 human immune cells, including plasma, B cell, T cell, and myeloid cell subpopulations. The tool is based on the linear support vector regression principle for deconvolution analysis of the expression matrix of immune cells. This study used expression data from GSE132903 and quantified the relative proportions of the 22 immune cells in each sample. In addition, Spearman correlation analysis was performed between hub genes and immune infiltration, and immune factors. This analysis was performed using the “psych” package in R software, and the results were displayed as heatmaps. Different immune factors were downloaded from the TISIDB database (Ru et al., 2019), including 24 immunoinhibitors, 45 immunostimulators, and 41 chemokines (Supplementary Table 2).
Drugs targeting hub genes were retrieved from the DrugBank database. The DrugBank database is a cheminformatics and bioinformatics repository containing detailed information on drugs and their targets. The database collects more than 7,800 drugs, including nutraceuticals, experimental drugs, FDA-approved small-molecule drugs, and FDA-approved biotech drugs (Wishart et al., 2018). The DrugBank also has a large collection of SNP drugs useful for pharmacogenomic studies.
All analyses were performed in R software. The t-test and Mann–Whitney U-test were selected according to whether the data conformed to a normal distribution. Significance was usually defined as p < 0.05.
To identify genes related to AD, we first obtained 481 differentially expressed genes from GSE132903 with the screening conditions “p_adj <0.01 and abs(logFC) > 0.585” (Supplementary Table 3). These DEGs were presented in a volcano plot (Figure 2A). A heatmap of the top 20 differentially expressed genes was plotted (Figure 2B). After removing abnormal samples and filtering the genes, the expression profiles of 5,000 genes and 195 samples were extracted from GSE132903 and used for the construction of a weighted gene co-expression network. When the soft threshold power was set to 14, the scale independence reached 0.858 and the average connection value was 30.124 (Figures 3A,B). When the cut height was set to 0.25 and the minimum module size was set to 50, 11 different co-expression modules were obtained by dynamic tree cutting (Figure 3C). Then, correlation analyses of each module with clinical traits were performed. The MEturquoise module had the highest positive correlation with AD (r = 0.47, p = 4e−12), while the MEblue module had the highest negative correlation with AD (r = −0.43, p = 3e−10; Figure 3D). Here, the MEturquoise module, containing 1,578 genes with the largest absolute value of correlation coefficient, was selected for further analysis. Additionally, correlation analysis between MM and GS showed that these genes were highly correlated with both module and phenotype (cor = 0.61, p = 1.8e−161; Figure 3E). Nine hub genes associated with iron metabolism and AD were obtained by taking the intersections of 481 DEs, 1,578 MEturquoise module genes, and 520 iron metabolism-related genes from 14 iron metabolism-related gene sets (Figure 4A). Violin plots showed that TSPO and PLOD1 were highly expressed in AD, and the other seven genes were expressed at lower levels than in the ND group in GSE132903 (Figure 5). In addition, the expression of these genes in blood samples from GSE63061 and GSE85426 is shown in Supplementary Figures 1, 2.
Figure 2. Differentially expressed genes between AD and ND samples. (A) Red genes represent significantly high expression in AD, green genes represent significantly high expression in ND, and gray genes indicate insignificant changes. (B) The heatmap shows the top 20 genes significantly highly expressed in AD or ND samples.
Figure 3. Results of the WGCNA. (A) The corresponding scale-free topological model fit indices at different soft threshold powers. (B) The corresponding mean connectivity values at different soft threshold powers. (C) Cluster dendrogram of genes. (D) Correlations between different modules and clinical traits. Red represents a positive correlation, and blue represents a negative correlation. (E) Correlation of module membership and gene significance in the turquoise module.
Figure 4. Hub genes and GO analysis. (A) Nine hub genes were obtained by taking the intersections of the DEGs, MEturquoise module genes of the WGCNA, and iron metabolism-related genes. (B) Biological processes in which the hub genes were involved.
Figure 5. Expression of hub genes in the AD and ND groups of brain tissue samples GSE132903. (A) ATP6V1D, (B) ATP6V1G2, (C) ATP6V1H, (D) CYP26B1, (E) FBXO34, (F) PGRMC1, (G) PLOD1, (H) SNCA, and (I) TSPO.
To understand the potential biological roles of these genes, enrichment analyses were performed. GO analysis revealed that six of the nine genes were involved in autophagy-related biological processes, including regulation of autophagy and macroautophagy, and iron metabolism-related biological processes, including transferrin transport, iron ion transport, transition metal ion transport, and heme biosynthetic process (Figure 4B). GSEA of these genes has shown that they are associated with several neurodegenerative diseases (AD, amyotrophic lateral sclerosis, Parkinson's disease, Prion disease, and Huntington's disease), neurological related pathways (the synaptic vesicle cycle, retrograde endocannabinoid signaling, retrograde endocannabinoid signaling, and pathway of neurodegeneration), and other pathways (cytokine–cytokine receptor interaction and oxidative phosphorylation; Figure 6).
Figure 6. GSEA revealed the enriched pathways of the hub genes. (A) ATP6V1D, (B) ATP6V1G2, (C) ATP6V1H, (D) CYP26B1, (E) FBXO34, (F) PGRMC1, (G) PLOD1, (H) SNCA, and (I) TSPO.
A multigene prediction model was constructed by a logistic regression algorithm based on GSE132903. Using stepwise regression analysis, four of these nine genes, including ATP6V1D, ATP6V1G2, FBXO34, and TSPO, were selected to obtain the best model. The results showed that the predictive model constructed from these four genes had good diagnostic performance, with an AUC of 0.8973 (Figure 7A). The model was then further validated in blood samples. The AUCs of the models in GSE63061 and GSE85426 were relatively high, 0.7277 and 0.7189, respectively (Figures 7B,C). Brain tissue samples tended to be more representative of AD pathology than blood samples, which may explain the better diagnostic performance of the former. However, it was difficult to obtain brain tissue in vivo. The good results in blood samples suggested that this model has a certain guiding significance for the diagnosis of patients with AD in clinical applications.
Figure 7. ROC curves and corresponding AUC values for the three expression cohorts. (A) Brain tissue samples from GSE132903. (B) Blood samples from GSE63061. (C) Blood samples from GSE85426.
The microenvironment consists of immune cells, extracellular matrix, inflammatory factors, and various growth factors that have an important impact on the clinical therapeutic sensitivity and disease diagnosis. In this study, the proportion of 22 immune cells in 97 AD samples and 98 ND samples was estimated by the CIBERSORT algorithm, which can be seen in the histogram (Figure 8A). The immune cell infiltration of AD and ND samples was compared in a boxplot (Figure 8B). The results showed that the AD group had significantly higher proportions of naive B cells (p = 0.0210), M1 macrophages (p = 0.0090), neutrophils (p = 0.0497), CD4 naive T cells (p = 0.0101), and gamma delta T cells (p = 0.0070), and lower proportions of plasma cells (p = 0.0056) and follicular helper T cells (p = 0.0314) than the ND group. Next, the relationship between the hub genes and immune infiltration, and immune factors was analyzed. PLOD1 and TSPO were significantly negatively associated with follicular helper T cells, CD8 T cells, and activated dendritic cells, and positively associated with resting NK cells, M1 macrophages, M0 macrophages, and naive B cells, while the opposite was true for the other downregulated genes (Figure 9A). The correlation heatmaps showed a significant correlation between the hub genes and most immune factors, including immunoinhibitors, immunostimulators, and chemokines (Figures 9B–D). These results suggested that the hub genes may play an important role in the immune microenvironment.
Figure 8. Immune infiltration between AD and ND samples. (A) The relative percentage of 22 immune cells in each sample. (B) Differences in immune infiltration between AD and ND samples.
Figure 9. Correlation of hub genes with immune cells and different immune factors. (A) Immune cells, (B) immunoinhibitors, (C) immunostimulators, and (D) chemokines.
Based on drug and target information from the DrugBank database, eight drugs targeting these nine hub genes were identified (Figure 10). Among these drugs, six drugs were approved, one was an investigational drug, and one was withdrawn. Tiludronic acid (DB01133), an inhibitor of ATP6V1D, ATP6V1G2, and ATP6V1H, is used for the treatment of Paget's disease of the bone. Tretinoin (DB00755) targeting CYP26B1 is used to treat fine wrinkles, acne vulgaris, and certain types of promyelocytic leukemia. Dextromethorphan (DB00514) is a binder of PGRMC1 used to treat cases of dry cough. Ascorbic acid (DB00126) is a cofactor of PLOD1 used to correct vitamin C deficiency and increase intestinal absorption of iron. Resveratrol (DB02709) targeting SNCA was investigated for the treatment of herpes labialis infections (cold sores). Both chlormezanone (DB01178) and zopiclone (DB01198) are agonists of TSPO. Chlormezanone is used to manage anxiety and treat muscle spasms, while zopiclone is used to treat insomnia (Supplementary Table 4).
Figure 10. Drugs targeting these nine hub genes obtained from the DrugBank database. Drug statuses, including approved, experimental, investigational, nutraceutical, vet_approved and withdrawn, are indicated by colored squares. Drug types, including agonist, binder, cofactor, and inhibitor, are indicated by colored circles.
Iron is involved in myelination, neurotransmitter synthesis, and mitochondrial respiration in the nervous system and could contribute to oxidative stress. Both iron overload and iron deficiency have adverse effects and may lead to neurological disorders. Relevant studies have found that increases in ferritin and iron, most likely ferritin-bound iron, are present in several brain regions involved in AD (Quintana and Gutiérrez, 2010; Galazka-Friedman et al., 2012; Raven et al., 2013).
In this study, nine genes linking AD and iron metabolism were screened. GO analysis showed that they were involved in autophagy-related and iron metabolism-related biological processes. A decrease in neuronal autophagy leads to an inability to clear pathological proteins, such as beta-amyloid (Aβ) and tau proteins (Feng et al., 2015). Several key proteins involved in the regulation of autophagy are closely associated with the development of AD (Majumder et al., 2011; Lucin et al., 2013; Heras-Sandoval et al., 2014; Sepe et al., 2014). Restoration of parkin-mediated mitophagy prevents cognitive decline (Goudarzi et al., 2021). Some neuroprotective drugs exert their effects by modulating mitophagy (Wang et al., 2018; Hirano et al., 2019; Han et al., 2020; Sun C. et al., 2020). When intracellular iron levels fall, ferritin is degraded to release free iron, a process called ferritinophagy. Biasiotto et al. proposed that abnormal ferritinophagy may be a link between impaired autophagy and dysfunctional iron homeostasis in several neurodegenerative diseases (Biasiotto et al., 2016). In addition, the GSEA showed that these hub genes were all associated with neurodegenerative diseases, especially AD, which validates the accuracy of the selection of these genes to some extent (Figure 6).
Four of the nine genes, including ATP6V1D, ATP6V1G2, FBXO34, and TSPO, were screened to construct a diagnostic model, which may be useful to guide the diagnosis of AD in clinical applications. Both ATP6V1G2 and ATP6V1D encode a component of vacuolar ATPase (V-ATPase). In addition to acting as an H+ pump, V-ATPase is also involved in enzyme activity, the coupled transport of substrates across membranes, and the dissociation of ligands from receptors (Huynh and Grinstein, 2007; Vahlensieck et al., 2021). A deficiency of V-ATPase can lead to central nervous system disorders such as AD and PD (Lee et al., 2010; Williamson and Hiesinger, 2010). ATP6V1G2 is downregulated in AD as a key metabolic gene and is involved in lysosomal transport, transporting protons from the cytoplasm to the lysosome, and maintaining lysosomal acidification (Li et al., 2020). ATP6V1D is associated with mitochondrial function and impaired bioenergetic metabolism (Alves et al., 2015). FBXO34 belongs to the F-box protein family, and F-box proteins are junction proteins for Skp1-Cul1-FBP (SCF)-type E3 ubiquitin ligases, directing the ubiquitination of numerous proteins (Randle and Laman, 2016). Ubiquitinated proteins are found in neurofibrillary tangles and oligomeric Aβ plaques, and a mutation of the ubiquitin-B+1 gene leads to neuronal degeneration, which is associated with spatial reference memory impairment (van Tijn et al., 2011) and AD (Tan et al., 2007). The ubiquitin–proteasome system is also related to several neuronal signaling pathways (Zhao et al., 2003; Patrick, 2006). The ubiquitin–proteasome system and tau phosphorylation in AD are closely related (Ciechanover and Kwon, 2015; Kumar P. et al., 2015). Gong et al. found that FBXO2 ubiquitinates β-secretase, which leads to protein degradation and reduced Aβ production (Gong et al., 2016). TSPO is mainly found in the outer mitochondrial membrane. In response to inflammatory stimulation, TSPO expression is highly upregulated in various inflammatory diseases, including AD (Rupprecht et al., 2010; Selvaraj and Stocco, 2015). TSPO is shown to be increased in postmortem brain samples from patients with AD (Venneti et al., 2009; Gui et al., 2020), which is consistent with our study. TSPO deficiency does not affect Aβ production, but it does accelerate Aβ deposition, leading to more senile plaques (Zhang H. et al., 2021). Studies have shown the presence of the rs6971 single nucleotide polymorphism (SNP) in TSPO, which produces different radioligand binding affinities (Owen et al., 2011; Tournier et al., 2020). Currently, TSPO is the primary target for PET and SPECT in vivo neuroinflammatory imaging to monitor the inflammatory state in the brain (Tournier et al., 2020; López-Picón et al., 2022). According to one of the hypotheses of AD pathogenesis, mitochondrial dysfunction occurs in the early stages, and thus, maintaining mitochondrial function may be a therapeutic strategy for AD (Readnower et al., 2011; Eckert et al., 2012; Kumar A. et al., 2015). Kim et al. identified novel TSPO ligands that can restore Aβ-induced mitochondrial dysfunction and improve cognitive impairment in mouse models with AD (Kim et al., 2021).
Intrinsic immune cells such as microglia and astrocytes, as well as peripheral immune cells, are involved in the neuroinflammation associated with AD (Calsolaro and Edison, 2016; Dionisio-Santos et al., 2019; Wyatt-Johnson and Brutkiewicz, 2020; Leng and Edison, 2021). Here, immune cell infiltration and immune factors were further explored. Box plots showed significant differences in multiple immune cells between the AD and the ND groups. M1 macrophages were found to be elevated in AD, which is consistent with a previous study (Liu et al., 2022). When stimulated by pro-inflammatory factors, macrophages are converted to the M1 phenotype. Upon recognition by specific receptors, it in turn secretes other pro-inflammatory mediators that further promote the conversion of unpolarized macrophages to M1, thus forming a positive pro-inflammatory feedback loop (Gate et al., 2010; Weisser et al., 2013; Sanz et al., 2020). In addition, neutrophils and gamma delta T cells were also upregulated in AD. Increased neutrophil accumulation was found in AD brains and AD model mice, and neutrophil hyperactivity is thought to be a feature of AD (Katayama, 2020; Kong et al., 2020). Katayama found that neutrophils appear to be drivers of AD and they secrete large amounts of reactive oxygen species (Katayama, 2020). Interleukin-17 (IL-17)-producing cells, mainly γδ T cells, accumulate in the brain and meninges of women with AD, accompanied by a cognitive decline (Brigas et al., 2021). IL-17-producing cells have been identified as key players in disease progression, as they promote a local immune amplification loop in the meninges and cause disruption of the blood–brain barrier (Shichita et al., 2009; Sutton et al., 2009; Gelderblom et al., 2012; Benakis et al., 2016). Heatmaps showed a strong correlation between these hub genes and different immune factors. IL-10 single nucleotide polymorphisms were found to affect the susceptibility to AD pathology (Babić Leko et al., 2020; Yang et al., 2021). When stimulated by anti-inflammatory cytokines, macrophages are converted to the M2 phenotype. Upon recognition by specific receptors, it in turn secretes other anti-inflammatory factors that promote the conversion of unpolarized macrophages to M2, thus forming a positive feedback mechanism for the anti-inflammatory response (Gordon and Martinez, 2010; Mulder et al., 2014; Murray et al., 2014). In addition, a promising lead compound promoting IL-10 activity was provided, confirming that promoting IL-10 expression may be useful to treat AD and stroke (Sun P. et al., 2020). The interaction between astrocytic CXCL1 and neuronal CXCR2 receptors exacerbates the synaptotoxic effects of Aβ and is expected to serve as a novel target for the treatment of AD (Perez-Nievas et al., 2021). CX3CL1 was found to be decreased in the cerebrospinal fluid of patients with AD (Perea et al., 2018), which is consistent with our findings. CX3CL1 has been shown to be a strong activator of adult neurogenesis, reducing neuronal loss and improving the cognitive function of patients with AD (Fan et al., 2020). In addition, the critical roles of CX3CL1/CX3CR1 and ATP/P2X7R in regulating microglial activation in AD have been reviewed (Suresh et al., 2021).
Finally, eight drugs targeting the above genes were retrieved from the DrugBank database. Zopiclone (DB01198) is a non-benzodiazepine hypnotic used for the short-term treatment of insomnia. Several studies have found zopiclone to be helpful for insomnia in patients with AD, thereby improving their quality of life (Katsunuma et al., 1998; Richardson et al., 2021; Louzada et al., 2022). Tiludronic acid (DB01133), a bisphosphonate, was first described for the treatment of Paget's disease of the bone (Reginster et al., 1988). A study found that tiludronic acid and olsalazine may be potential drugs for the treatment of AD through in silico predictions, but further experimental validation is needed (G et al., 2020). Ascorbic acid (DB00126) is highly concentrated in the brain as a neuroprotective compound and may combat neurotoxic and neurodegenerative diseases, including AD (Moretti and Rodrigues, 2021). Jang et al. found that a mixture of Schisandra Chinensis extract and ascorbic acid improves mitochondrial function and memory and can also be used to alleviate AD and aging-related memory decline (Jang et al., 2020). Dextromethorphan (DB00514) is an NMDA receptor antagonist. Deuterated dextromethorphan/quinidine (AVP-786) is a promising and well-tolerated treatment option for agitation in AD, having completed two phase III trials (Garay and Grossberg, 2017; Khoury et al., 2021; Khoury, 2022). Resveratrol (DB02709), a polyphenolic phytoalexin, has been reported to affect several AD-related- and neuroprotective genes (Dennison et al., 2022). Resveratrol can improve AD-associated pathologies and produce therapeutic effects (Gomes et al., 2018; Rahman et al., 2020; Yan et al., 2020; Fang et al., 2021; Gu et al., 2021; Jang et al., 2021; Abozaid et al., 2022). Copper (DB09130) is a transition metal and a trace element in the body. Copper imbalance is associated with the pathogenesis of AD, which is an avenue for new therapeutic strategies (Ejaz et al., 2020; Zhu et al., 2020; Zubčić et al., 2020; Lei et al., 2021; Pal et al., 2021).
Through bioinformatics approaches, we obtained nine hub genes linking iron metabolism and AD. The biological processes and pathways in which they are involved were explored, which will help in understanding the development of AD. However, further experimental validation is required to verify these functions. Based on the logistic regression analysis, we constructed a diagnostic model that can diagnose patients with AD by detecting the expression of several genes in the blood. In addition, these hub genes have been found to be associated with different immune factors, suggesting that they may also have an important role in the immune microenvironment. However, further studies are needed to explore their specific roles. Currently, only a few drugs targeting these hub genes are predicted to alleviate AD, suggesting that additional drugs need to be developed.
The original contributions presented in the study are included in the article/Supplementary Material, further inquiries can be directed to the corresponding authors.
XG, XC, and CL conceived and designed the study. DL, KC, and SL were responsible for the collection and assembly of data, data analysis, and interpretation. DL and XG were involved in the writing of the manuscript. BC, PZ, and GH provided help in revising the manuscript. All authors read and approved the final manuscript.
This work was supported by the National Natural Science Foundation of China (81772829 and 81830052), the Special Program for Collaborative Innovation, the Construction Project of Shanghai Key Laboratory of Molecular Imaging (18DZ2260400), the Top-100 Talent Cultivation Plan of Shanghai University of Medicine and Health Sciences, Funding Scheme for Training Young Teachers in Shanghai Colleges, and Innovative Team of Intelligent Inspection and Active Health (ITIH).
The authors declare that the research was conducted in the absence of any commercial or financial relationships that could be construed as a potential conflict of interest.
All claims expressed in this article are solely those of the authors and do not necessarily represent those of their affiliated organizations, or those of the publisher, the editors and the reviewers. Any product that may be evaluated in this article, or claim that may be made by its manufacturer, is not guaranteed or endorsed by the publisher.
The Supplementary Material for this article can be found online at: https://www.frontiersin.org/articles/10.3389/fnagi.2022.949083/full#supplementary-material
Supplementary Figure 1. Expression of hub genes in the AD and ND groups of blood samples from GSE63061. (A) ATP6V1D. (B) ATP6V1G2. (C) ATP6V1H. (D) CYP26B1. (E) FBXO34. (F) PGRMC1. (G) PLOD1. (H) SNCA. (I) TSPO.
Supplementary Figure 2. Expression of hub genes in the AD and ND groups of blood samples GSE85426. (A) ATP6V1D. (B) ATP6V1G2. (C) FBXO34. (D) PGRMC1. (E) PLOD1. (F) SNCA. (G) TSPO.
Supplementary Table 1. Five hundred twenty genes obtained from 14 iron metabolism-related gene sets.
Supplementary Table 2. Different immune factors were downloaded from the TISIDB database.
Supplementary Table 3. Differentially expressed genes between AD and ND samples in GSE132903.
Supplementary Table 4. Information on drugs targeting these nine hub genes.
Abozaid, O. A. R., Sallam, M. W., El-Sonbaty, S., Aziza, S., Emad, B., and Ahmed, E. S. A. (2022). Resveratrol-selenium nanoparticles alleviate neuroinflammation and neurotoxicity in a rat model of Alzheimer's disease by regulating Sirt1/miRNA-134/GSK3β expression. Biol. Trace Element Res. 21:7. doi: 10.1007/s12011-021-03073-7
Alves, C. J., Dariolli, R., Jorge, F. M., Monteiro, M. R., Maximino, J. R., Martins, R. S., et al. (2015). Gene expression profiling for human iPS-derived motor neurons from sporadic ALS patients reveals a strong association between mitochondrial functions and neurodegeneration. Front. Cell. Neurosci. 9:289. doi: 10.3389/fncel.2015.00289
Apostolakis, S., and Kypraiou, A. M. (2017). Iron in neurodegenerative disorders: being in the wrong place at the wrong time? Rev. Neurosci. 28, 893–911. doi: 10.1515/revneuro-2017-0020
Babić Leko, M., Nikolac Perković, M., Klepac, N., Štrac, D., Borovečki, F., Pivac, N., et al. (2020). IL-1β, IL-6, IL-10, and TNFα single nucleotide polymorphisms in human influence the susceptibility to Alzheimer's disease pathology. J. Alzheimer's Dis. 75, 1029–1047. doi: 10.3233/JAD-200056
Barthold, D., Joyce, G., Ferido, P., Drabo, E. F., Marcum, Z. A., Gray, S. L., et al. (2020). Pharmaceutical treatment for Alzheimer's disease and related dementias: utilization and disparities. J. Alzheimer's Dis. 76, 579–589. doi: 10.3233/JAD-200133
Benakis, C., Brea, D., Caballero, S., Faraco, G., Moore, J., Murphy, M., et al. (2016). Commensal microbiota affects ischemic stroke outcome by regulating intestinal γδ T cells. Nat. Med. 22, 516–523. doi: 10.1038/nm.4068
Biasiotto, G., Di Lorenzo, D., Archetti, S., and Zanella, I. (2016). Iron and neurodegeneration: is ferritinophagy the link? Mol. Neurobiol. 53, 5542–5574. doi: 10.1007/s12035-015-9473-y
Brigas, H. C., Ribeiro, M., Coelho, J. E., Gomes, R., Gomez-Murcia, V., Carvalho, K., et al. (2021). IL-17 triggers the onset of cognitive and synaptic deficits in early stages of Alzheimer's disease. Cell Rep. 36:109574. doi: 10.1016/j.celrep.2021.109574
Calsolaro, V., and Edison, P. (2016). Neuroinflammation in Alzheimer's disease: current evidence and future directions. Alzheimer's Dement. 12, 719–732. doi: 10.1016/j.jalz.2016.02.010
Choi, D. H., Kwon, K. C., Hwang, D. J., Koo, J. H., Um, H. S., Song, H. S., et al. (2021). Treadmill exercise alleviates brain iron dyshomeostasis accelerating neuronal amyloid-β production, neuronal cell death, and cognitive impairment in transgenic mice model of Alzheimer's disease. Mol. Neurobiol. 58, 3208–3223. doi: 10.1007/s12035-021-02335-8
Ciechanover, A., and Kwon, Y. T. (2015). Degradation of misfolded proteins in neurodegenerative diseases: therapeutic targets and strategies. Exp. Mol. Med. 47:e147. doi: 10.1038/emm.2014.117
Coat, J., Demoersman, J., Beuzit, S., Cornec, D., Devauchelle-Pensec, V., Saraux, A., et al. (2015). Anti-B lymphocyte immunotherapy is associated with improvement of periodontal status in subjects with rheumatoid arthritis. J. Clin. Periodontol. 42, 817–823. doi: 10.1111/jcpe.12433
Dennison, J. L., Volmar, C. H., Ke, D., Wang, J., Gravel, E., Hammond-Vignini, S., et al. (2022). JOTROL, a novel formulation of resveratrol, shows beneficial effects in the 3xTg-AD mouse model. J. Alzheimer's Dis. 86, 173–190. doi: 10.3233/JAD-215370
DeTure, M. A., and Dickson, D. W. (2019). The neuropathological diagnosis of Alzheimer's disease. Mol. Neurodegener. 14:32. doi: 10.1186/s13024-019-0333-5
Dionisio-Santos, D. A., Olschowka, J. A., and O'Banion, M. K. (2019). Exploiting microglial and peripheral immune cell crosstalk to treat Alzheimer's disease. J. Neuroinflam. 16:74. doi: 10.1186/s12974-019-1453-0
Eckert, G. P., Renner, K., Eckert, S. H., Eckmann, J., Hagl, S., Abdel-Kader, R. M., et al. (2012). Mitochondrial dysfunction–a pharmacological target in Alzheimer's disease. Mol. Neurobiol. 46, 136–150. doi: 10.1007/s12035-012-8271-z
Ejaz, H. W., Wang, W., and Lang, M. (2020). Copper toxicity links to pathogenesis of Alzheimer's disease and therapeutics approaches. Int. J. Mol. Sci. 21:20766. doi: 10.3390/ijms21207660
Fan, Q., He, W., Gayen, M., Benoit, M. R., Luo, X., Hu, X., et al. (2020). Activated CX3CL1/Smad2 signals prevent neuronal loss and Alzheimer's tau pathology-mediated cognitive dysfunction. J. Neurosci. 40, 1133–1144. doi: 10.1523/JNEUROSCI.1333-19.2019
Fang, Y., Su, Z., Si, W., Liu, Y., Li, J., and Zeng, P. (2021). Network pharmacology-based study of the therapeutic mechanism of resveratrol for Alzheimer's disease. Nan fang yi ke da xue xue bao 41, 10–19. doi: 10.12122/j.issn.1673-4254.2021.01.02
Feng, L., Zhang, J., Ding, Q., Zhu, N., Wang, P., and Shen, Y. (2015). Autophagy involved in overexpressed tau and okadaic acid-induced hyperphosphorylated tau degradation. Chin Pharm Bull. 31, 356–362. doi: 10.3969/j.issn.1001-1978.2015.03.013
G, N. S. H., Ganesan Rajalekshmi, S., Murahari, M., and Burri, R. R. (2020). Reappraisal of FDA approved drugs against Alzheimer's disease based on differential gene expression and protein interaction network analysis: an in silico approach. J. Biomol. Struct. Dyn. 38, 3972–3989. doi: 10.1080/07391102.2019.1671231
Galazka-Friedman, J., Bauminger, E. R., Szlachta, K., and Friedman, A. (2012). The role of iron in neurodegeneration–Mössbauer spectroscopy, electron microscopy, enzyme-linked immunosorbent assay and neuroimaging studies. J. Phys. 24:244106. doi: 10.1088/0953-8984/24/24/244106
Garay, R. P., and Grossberg, G. T. (2017). AVP-786 for the treatment of agitation in dementia of the Alzheimer's type. Expert Opin. Investig. Drugs 26, 121–132. doi: 10.1080/13543784.2017.1267726
Gate, D., Rezai-Zadeh, K., Jodry, D., Rentsendorj, A., and Town, T. (2010). Macrophages in Alzheimer's disease: the blood-borne identity. J. Neural Transmission 117, 961–970. doi: 10.1007/s00702-010-0422-7
Gelderblom, M., Weymar, A., Bernreuther, C., Velden, J., Arunachalam, P., Steinbach, K., et al. (2012). Neutralization of the IL-17 axis diminishes neutrophil invasion and protects from ischemic stroke. Blood 120, 3793–3802. doi: 10.1182/blood-2012-02-412726
Gomes, B. A. Q., Silva, J. P. B., Romeiro, C. F. R., Dos Santos, S. M., Rodrigues, C. A., Gonçalves, P. R., et al. (2018). Neuroprotective mechanisms of resveratrol in Alzheimer's disease: role of SIRT1. Oxid. Med. Cell. Longevity 2018:8152373. doi: 10.1155/2018/8152373
Gong, B., Radulovic, M., Figueiredo-Pereira, M. E., and Cardozo, C. (2016). The ubiquitin-proteasome system: potential therapeutic targets for Alzheimer's disease and spinal cord injury. Front. Mol. Neurosci. 9:4. doi: 10.3389/fnmol.2016.00004
Gong, N. J., Dibb, R., Bulk, M., van der Weerd, L., and Liu, C. (2019). Imaging beta amyloid aggregation and iron accumulation in Alzheimer's disease using quantitative susceptibility mapping MRI. NeuroImage 191, 176–185. doi: 10.1016/j.neuroimage.2019.02.019
Gordon, S., and Martinez, F. O. (2010). Alternative activation of macrophages: mechanism and functions. Immunity 32, 593–604. doi: 10.1016/j.immuni.2010.05.007
Goudarzi, S., Hosseini, A., Abdollahi, M., and Haghi-Aminjan, H. (2021). Insights into parkin-mediated mitophagy in Alzheimer's disease: a systematic review. Front. Aging Neurosci. 13:674071. doi: 10.3389/fnagi.2021.674071
Gu, J., Li, Z., Chen, H., Xu, X., Li, Y., and Gui, Y. (2021). Neuroprotective effect of trans-resveratrol in mild to moderate Alzheimer disease: a randomized, double-blind trial. Neurol. Therapy 10, 905–917. doi: 10.1007/s40120-021-00271-2
Gui, Y., Marks, J. D., Das, S., Hyman, B. T., and Serrano-Pozo, A. (2020). Characterization of the 18 kDa translocator protein (TSPO) expression in post-mortem normal and Alzheimer's disease brains. Brain Pathol. 30, 151–164. doi: 10.1111/bpa.12763
Han, Y., Wang, N., Kang, J., and Fang, Y. (2020). β-Asarone improves learning and memory in Aβ(1-42)-induced Alzheimer's disease rats by regulating PINK1-Parkin-mediated mitophagy. Metabol. Brain Dis. 35, 1109–1117. doi: 10.1007/s11011-020-00587-2
Heras-Sandoval, D., Pérez-Rojas, J. M., Hernández-Damián, J., and Pedraza-Chaverri, J. (2014). The role of PI3K/AKT/mTOR pathway in the modulation of autophagy and the clearance of protein aggregates in neurodegeneration. Cell. Signal. 26, 2694–2701. doi: 10.1016/j.cellsig.2014.08.019
Hirano, K., Fujimaki, M., Sasazawa, Y., Yamaguchi, A., Ishikawa, K. I., Miyamoto, K., et al. (2019). Neuroprotective effects of memantine via enhancement of autophagy. Biochem. Biophys. Res. Commun. 518, 161–170. doi: 10.1016/j.bbrc.2019.08.025
Huynh, K. K., and Grinstein, S. (2007). Regulation of vacuolar pH and its modulation by some microbial species. Microbiol. Mol. Biol. Rev. 71, 452–462. doi: 10.1128/MMBR.00003-07
Jang, B. G., Lee, J., Choi, B., Koh, Y. H., and Kim, M. J. (2021). Unexpected beta-amyloid production by middle doses of resveratrol through stabilization of APP protein and AMPK-mediated inhibition of trypsin-like proteasome activity in a cell model of Alzheimer's disease. Food Chem. Toxicol. 152:112185. doi: 10.1016/j.fct.2021.112185
Jang, Y., Lee, J. H., Lee, M. J., Kim, S. J., Ju, X., Cui, J., et al. (2020). Schisandra extract and ascorbic acid synergistically enhance cognition in mice through modulation of mitochondrial respiration. Nutrients 12:40897. doi: 10.3390/nu12040897
Katayama, H (2020). Anti-interleukin-17A and anti-interleukin-23 antibodies may be effective against Alzheimer's disease: role of neutrophils in the pathogenesis. Brain Behav. 10:e01504. doi: 10.1002/brb3.1504
Katsunuma, H., Shimizu, T., Ogawa, K., Kubo, H., Ishida, H., and Yoshihama, A. (1998). Treatment of insomnia by concomitant therapy with Zopiclone and Aniracetam in patients with cerebral infarction, cerebroatrophy, Alzheimer's disease and Parkinson's disease. Psychiatr. Clin. Neurosci. 52, 198–200. doi: 10.1111/j.1440-1819.1998.tb01028.x
Khoury, R (2022). Deuterated dextromethorphan/quinidine for agitation in Alzheimer's disease. Neural Regener. Res. 17, 1013–1014. doi: 10.4103/1673-5374.324842
Khoury, R., Marx, C., Mirgati, S., Velury, D., Chakkamparambil, B., and Grossberg, G. T. (2021). AVP-786 as a promising treatment option for Alzheimer's Disease including agitation. Expert Opin. Pharmacother. 22, 783–795. doi: 10.1080/14656566.2021.1882995
Kim, T., Morshed, M. N., Londhe, A. M., Lim, J. W., Lee, H. E., Cho, S., et al. (2021). The translocator protein ligands as mitochondrial functional modulators for the potential anti-Alzheimer agents. J. Enzyme Inhibit. Med. Chem. 36, 831–846. doi: 10.1080/14756366.2021.1900158
Kong, Y., Liu, K., Hua, T., Zhang, C., Sun, B., and Guan, Y. (2020). PET imaging of neutrophils infiltration in Alzheimer's disease transgenic mice. Front. Neurol. 11:523798. doi: 10.3389/fneur.2020.523798
Kumar, A., Singh, A., and Ekavali, A. (2015). A review on Alzheimer's disease pathophysiology and its management: an update. Pharmacol. Rep. 67, 195–203. doi: 10.1016/j.pharep.2014.09.004
Kumar, P., Jha, N. K., Jha, S. K., Ramani, K., and Ambasta, R. K. (2015). Tau phosphorylation, molecular chaperones, and ubiquitin E3 ligase: clinical relevance in Alzheimer's disease. J. Alzheimer's Dis. 43, 341–361. doi: 10.3233/JAD-140933
Lai, D., Tan, L., Zuo, X., Liu, D., Jiao, D., Wan, G., et al. (2021). Prognostic ferroptosis-related lncRNA signatures associated with immunotherapy and chemotherapy responses in patients with stomach cancer. Front. Genet. 12:798612. doi: 10.3389/fgene.2021.798612
Langfelder, P., and Horvath, S. (2008). WGCNA: an R package for weighted correlation network analysis. BMC Bioinformat. 9:559. doi: 10.1186/1471-2105-9-559
Lee, J. H., and Lee, M. S. (2019). Brain iron accumulation in atypical Parkinsonian syndromes: in vivo MRI evidences for distinctive patterns. Front. Neurol. 10:74. doi: 10.3389/fneur.2019.00074
Lee, J. H., Yu, W. H., Kumar, A., Lee, S., Mohan, P. S., Peterhoff, C. M., et al. (2010). Lysosomal proteolysis and autophagy require presenilin 1 and are disrupted by Alzheimer-related PS1 mutations. Cell 141, 1146–1158. doi: 10.1016/j.cell.2010.05.008
Lei, P., Ayton, S., and Bush, A. I. (2021). The essential elements of Alzheimer's disease. J. Biol. Chem. 296:100105. doi: 10.1074/jbc.REV120.008207
Leng, F., and Edison, P. (2021). Neuroinflammation and microglial activation in Alzheimer disease: where do we go from here? Nat. Rev. Neurol. 17, 157–172. doi: 10.1038/s41582-020-00435-y
Li, W. X., Li, G. H., Tong, X., Yang, P. P., Huang, J. F., Xu, L., et al. (2020). Systematic metabolic analysis of potential target, therapeutic drug, diagnostic method and animal model applicability in three neurodegenerative diseases. Aging 12, 9882–9914. doi: 10.18632/aging.103253
Liberzon, A., Birger, C., Thorvaldsdóttir, H., Ghandi, M., Mesirov, J. P., and Tamayo, P. (2015). The molecular signatures database (MSigDB) hallmark gene set collection. Cell Syst. 1, 417–425. doi: 10.1016/j.cels.2015.12.004
Liu, C., Zhang, X., Chai, H., Xu, S., Liu, Q., Luo, Y., et al. (2022). Identification of immune cells and key genes associated with Alzheimer's disease. Int. J. Medical Sci. 19, 112–125. doi: 10.7150/ijms.66422
Long, J. M., and Holtzman, D. M. (2019). Alzheimer disease: an update on pathobiology and treatment strategies. Cell 179, 312–339. doi: 10.1016/j.cell.2019.09.001
López-Picón, F. R., Keller, T., Bocancea, D., Helin, J. S., Krzyczmonik, A., Helin, S., et al. (2022). Direct comparison of [(18)F]F-DPA with [(18)F]DPA-714 and [(11)C]PBR28 for neuroinflammation imaging in the same Alzheimer's disease model mice and healthy controls. Mol. Imaging Biol. 24, 157–166. doi: 10.1007/s11307-021-01646-5
Louzada, L. L., Machado, F. V., Quintas, J. L., Ribeiro, G. A., Silva, M. V., Mendonça-Silva, D. L., et al. (2022). The efficacy and safety of zolpidem and zopiclone to treat insomnia in Alzheimer's disease: a randomized, triple-blind, placebo-controlled trial. Neuropsychopharmacology 47, 570–579. doi: 10.1038/s41386-021-01191-3
Lucin, K. M., O'Brien, C. E., Bieri, G., Czirr, E., Mosher, K. I., Abbey, R. J., et al. (2013). Microglial beclin 1 regulates retromer trafficking and phagocytosis and is impaired in Alzheimer's disease. Neuron 79, 873–886. doi: 10.1016/j.neuron.2013.06.046
Majumder, S., Richardson, A., Strong, R., and Oddo, S. (2011). Inducing autophagy by rapamycin before, but not after, the formation of plaques and tangles ameliorates cognitive deficits. PLoS ONE 6:e25416. doi: 10.1371/journal.pone.0025416
Mills, E., Dong, X.-P., Wang, F., and Xu, H. (2010). Mechanisms of brain iron transport: insight into neurodegeneration and CNS disorders. Future Med Chem. 2, 51–64. doi: 10.4155/fmc.09.140
Moretti, M., and Rodrigues, A. L. S. (2021). Functional role of ascorbic acid in the central nervous system: a focus on neurogenic and synaptogenic processes. Nutr. Neurosci. 2021, 1–11. doi: 10.1080/1028415X.2021.1956848
Mou, Y., Zhang, Y., Wu, J., Hu, B., Zhang, C., Duan, C., et al. (2020). The landscape of iron metabolism-related and methylated genes in the prognosis prediction of clear cell renal cell carcinoma. Front. Oncol. 10:788. doi: 10.3389/fonc.2020.00788
Mulder, R., Banete, A., and Basta, S. (2014). Spleen-derived macrophages are readily polarized into classically activated (M1) or alternatively activated (M2) states. Immunobiology 219, 737–745. doi: 10.1016/j.imbio.2014.05.005
Mullard, A (2021). Landmark Alzheimer's drug approval confounds research community. Nature 594, 309–310. doi: 10.1038/d41586-021-01546-2
Murray, P. J., Allen, J. E., Biswas, S. K., Fisher, E. A., Gilroy, D. W., Goerdt, S., et al. (2014). Macrophage activation and polarization: nomenclature and experimental guidelines. Immunity 41, 14–20. doi: 10.1016/j.immuni.2014.06.008
Owen, D. R., Gunn, R. N., Rabiner, E. A., Bennacef, I., Fujita, M., Kreisl, W. C., et al. (2011). Mixed-affinity binding in humans with 18-kDa translocator protein ligands. J. Nucl. Med. 52, 24–32. doi: 10.2967/jnumed.110.079459
Pal, A., Rani, I., Pawar, A., Picozza, M., Rongioletti, M., and Squitti, R. (2021). Microglia and astrocytes in Alzheimer's disease in the context of the aberrant copper homeostasis hypothesis. Biomolecules 11:111598. doi: 10.3390/biom11111598
Pantopoulos, K., Porwal, S. K., Tartakoff, A., and Devireddy, L. (2012). Mechanisms of mammalian iron homeostasis. Biochemistry 51, 5705–5724. doi: 10.1021/bi300752r
Patrick, G. N (2006). Synapse formation and plasticity: recent insights from the perspective of the ubiquitin proteasome system. Curr. Opin. Neurobiol. 16, 90–94. doi: 10.1016/j.conb.2006.01.007
Peng, Y., Chang, X., and Lang, M. (2021). Iron homeostasis disorder and Alzheimer's disease. Int. J. Mol. Sci. 22:12442. doi: 10.3390/ijms222212442
Perea, J. R., Lleó, A., Alcolea, D., Fortea, J., Ávila, J., and Bolós, M. (2018). Decreased CX3CL1 levels in the cerebrospinal fluid of patients with Alzheimer's disease. Front. Neurosci. 12:609. doi: 10.3389/fnins.2018.00609
Perez-Nievas, B. G., Johnson, L., Beltran-Lobo, P., Hughes, M. M., Gammallieri, L., Tarsitano, F., et al. (2021). Astrocytic C-X-C motif chemokine ligand-1 mediates β-amyloid-induced synaptotoxicity. J. Neuroinflam. 18:306. doi: 10.1186/s12974-021-02371-0
Quintana, C., and Gutiérrez, L. (2010). Could a dysfunction of ferritin be a determinant factor in the aetiology of some neurodegenerative diseases? Biochim. Biophys. Acta 1800, 770–782. doi: 10.1016/j.bbagen.2010.04.012
Rahman, M. H., Akter, R., Bhattacharya, T., Abdel-Daim, M. M., Alkahtani, S., Arafah, M. W., et al. (2020). Resveratrol and neuroprotection: impact and its therapeutic potential in Alzheimer's disease. Front. Pharmacol. 11:619024. doi: 10.3389/fphar.2020.619024
Randle, S. J., and Laman, H. (2016). F-box protein interactions with the hallmark pathways in cancer. Semin. Cancer Biol. 36, 3–17. doi: 10.1016/j.semcancer.2015.09.013
Raven, E. P., Lu, P. H., Tishler, T. A., Heydari, P., and Bartzokis, G. (2013). Increased iron levels and decreased tissue integrity in hippocampus of Alzheimer's disease detected in vivo with magnetic resonance imaging. J. Alzheimer's Dis. 37, 127–136. doi: 10.3233/JAD-130209
Readnower, R. D., Sauerbeck, A. D., and Sullivan, P. G. (2011). Mitochondria, amyloid β, and Alzheimer's disease. Int. J. Alzheimer's Dis. 2011:104545. doi: 10.4061/2011/104545
Reginster, J. Y., Jeugmans-Huynen, A. M., Albert, A., Denis, D., Deroisy, R., Lecart, M. P., et al. (1988). Biological and clinical assessment of a new bisphosphonate, (chloro-4 phenyl) thiomethylene bisphosphonate, in the treatment of Paget's disease of bone. Bone 9, 349–354. doi: 10.1016/8756-3282(88)90115-9
Richardson, K., Savva, G. M., Boyd, P. J., Aldus, C., Maidment, I., Pakpahan, E., et al. (2021). Non-benzodiazepine hypnotic use for sleep disturbance in people aged over 55 years living with dementia: a series of cohort studies. Health Technol. Assess. 25, 1–202. doi: 10.3310/hta25010
Ru, B., Wong, C. N., Tong, Y., Zhong, J. Y., Zhong, S. S. W., Wu, W. C., et al. (2019). TISIDB: an integrated repository portal for tumor-immune system interactions. Bioinformatics 35, 4200–4202. doi: 10.1093/bioinformatics/btz210
Rupprecht, R., Papadopoulos, V., Rammes, G., Baghai, T. C., Fan, J., Akula, N., et al. (2010). Translocator protein (18 kDa) (TSPO) as a therapeutic target for neurological and psychiatric disorders. Nat. Rev. Drug Discov. 9, 971–988. doi: 10.1038/nrd3295
Sanz, M., Marco Del Castillo, A., Jepsen, S., Gonzalez-Juanatey, J. R., D'Aiuto, F., Bouchard, P., et al. (2020). Periodontitis and cardiovascular diseases: consensus report. J. Clin. Periodontol. 47, 268–288. doi: 10.1111/jcpe.13189
Selvaraj, V., and Stocco, D. M. (2015). The changing landscape in translocator protein (TSPO) function. Trends Endocrinol. Metabol. 26, 341–348. doi: 10.1016/j.tem.2015.02.007
Sepe, S., Nardacci, R., Fanelli, F., Rosso, P., Bernardi, C., Cecconi, F., et al. (2014). Expression of Ambra1 in mouse brain during physiological and Alzheimer type aging. Neurobiol. Aging 35, 96–108. doi: 10.1016/j.neurobiolaging.2013.07.001
Shichita, T., Sugiyama, Y., Ooboshi, H., Sugimori, H., Nakagawa, R., Takada, I., et al. (2009). Pivotal role of cerebral interleukin-17-producing gammadeltaT cells in the delayed phase of ischemic brain injury. Nat. Med. 15, 946–950. doi: 10.1038/nm.1999
Sun, C., Qiu, X., Wang, Y., Liu, J., Li, Q., Jiang, H., et al. (2020). Long-term oral melatonin alleviates memory deficits, reduces amyloid-β deposition associated with downregulation of BACE1 and mitophagy in APP/PS1 transgenic mice. Neurosci. Lett. 735:135192. doi: 10.1016/j.neulet.2020.135192
Sun, P., Zhou, W., Yue, H., Zhang, C., Ou, Y., Yang, Z., et al. (2020). Compound AD110 acts as therapeutic management for Alzheimer's disease and stroke in mouse and rat models. ACS Chem. Neurosci. 11, 929–938. doi: 10.1021/acschemneuro.9b00651
Suresh, P., Phasuk, S., and Liu, I. Y. (2021). Modulation of microglia activation and Alzheimer's disease: CX3 chemokine ligand 1/CX3CR and P2X(7)R signaling. Tzu chi Med. J. 33, 1–6. doi: 10.4103/tcmj.tcmj_144_20
Sutton, C. E., Lalor, S. J., Sweeney, C. M., Brereton, C. F., Lavelle, E. C., and Mills, K. H. (2009). Interleukin-1 and IL-23 induce innate IL-17 production from gammadelta T cells, amplifying Th17 responses and autoimmunity. Immunity 31, 331–341. doi: 10.1016/j.immuni.2009.08.001
Tan, Z., Sun, X., Hou, F. S., Oh, H. W., Hilgenberg, L. G., Hol, E. M., et al. (2007). Mutant ubiquitin found in Alzheimer's disease causes neuritic beading of mitochondria in association with neuronal degeneration. Cell Death Different. 14, 1721–1732. doi: 10.1038/sj.cdd.4402180
Thirupathi, A., and Chang, Y. Z. (2019). Brain iron metabolism and CNS diseases. Adv. Exp. Med. Biol. 1173, 1–19. doi: 10.1007/978-981-13-9589-5_1
Tournier, B. B., Tsartsalis, S., Ceyzériat, K., Garibotto, V., and Millet, P. (2020). In vivo TSPO signal and neuroinflammation in Alzheimer's disease. Cells 9:1941. doi: 10.3390/cells9091941
Vahlensieck, C., Thiel, C. S., Adelmann, J., Lauber, B. A., Polzer, J., and Ullrich, O. (2021). Rapid transient transcriptional adaptation to hypergravity in jurkat T cells revealed by comparative analysis of microarray and RNA-seq data. Int. J. Mol. Sci. 22:168451. doi: 10.3390/ijms22168451
van Tijn, P., Hobo, B., Verhage, M. C., Oitzl, M. S., van Leeuwen, F. W., and Fischer, D. F. (2011). Alzheimer-associated mutant ubiquitin impairs spatial reference memory. Physiol. Behav. 102, 193–200. doi: 10.1016/j.physbeh.2010.11.001
Venneti, S., Wiley, C. A., and Kofler, J. (2009). Imaging microglial activation during neuroinflammation and Alzheimer's disease. J. Neuroimmune Pharmacol. 4, 227–243. doi: 10.1007/s11481-008-9142-2
Wang, H., Jiang, T., Li, W., Gao, N., and Zhang, T. (2018). Resveratrol attenuates oxidative damage through activating mitophagy in an in vitro model of Alzheimer's disease. Toxicol. Lett. 282, 100–108. doi: 10.1016/j.toxlet.2017.10.021
Ward, R. J., Zucca, F. A., Duyn, J. H., Crichton, R. R., and Zecca, L. (2014). The role of iron in brain ageing and neurodegenerative disorders. Lancet Neurol. 13, 1045–1060. doi: 10.1016/S1474-4422(14)70117-6
Weisser, S. B., McLarren, K. W., Kuroda, E., and Sly, L. M. (2013). Generation and characterization of murine alternatively activated macrophages. Methods Mol. Biol. 946, 225–239. doi: 10.1007/978-1-62703-128-8_14
Williamson, W. R., and Hiesinger, P. R. (2010). On the role of v-ATPase V0a1-dependent degradation in Alzheimer disease. Commun. Integr. Biol. 3, 604–607. doi: 10.4161/cib.3.6.13364
Wishart, D. S., Feunang, Y. D., Guo, A. C., Lo, E. J., Marcu, A., Grant, J. R., et al. (2018). DrugBank 5.0: a major update to the DrugBank database for 2018. Nucl. Acids Res. 46, D1074–d1082. doi: 10.1093/nar/gkx1037
Wyatt-Johnson, S. K., and Brutkiewicz, R. R. (2020). The complexity of microglial interactions with innate and adaptive immune cells in Alzheimer's disease. Front. Aging Neurosci. 12:592359. doi: 10.3389/fnagi.2020.592359
Yan, N., and Zhang, J. (2019). Iron metabolism, ferroptosis, and the links with Alzheimer's disease. Front. Neurosci. 13:1443. doi: 10.3389/fnins.2019.01443
Yan, Y., Yang, H., Xie, Y., Ding, Y., Kong, D., and Yu, H. (2020). Research progress on Alzheimer's disease and resveratrol. Neurochem. Res. 45, 989–1006. doi: 10.1007/s11064-020-03007-0
Yang, R., Duan, J., Luo, F., Tao, P., and Hu, C. (2021). IL-6, IL-8 and IL-10 polymorphisms may impact predisposition of Alzheimer's disease: a meta-analysis. Acta Neurol. Belgica 121, 1505–1512. doi: 10.1007/s13760-020-01369-4
Zhang, H., Wang, H., Gao, F., Yang, J., Xu, Y., Fu, Y., et al. (2021). TSPO deficiency accelerates amyloid pathology and neuroinflammation by impairing microglial phagocytosis. Neurobiol. Aging 106, 292–303. doi: 10.1016/j.neurobiolaging.2021.06.020
Zhang, T., Liu, N., Wei, W., Zhang, Z., and Li, H. (2021). Integrated analysis of weighted gene co-expression network analysis identifying six genes as novel biomarkers for Alzheimer's disease. Oxid. Med. Cell. Longevity 2021:9918498. doi: 10.1155/2021/9918498
Zhao, Y., Hegde, A. N., and Martin, K. C. (2003). The ubiquitin proteasome system functions as an inhibitory constraint on synaptic strengthening. Current biology : CB. 13, 887–898. doi: 10.1016/S0960-9822(03)00332-4
Zhu, X., Victor, T. W., Ambi, A., Sullivan, J. K., Hatfield, J., Xu, F., et al. (2020). Copper accumulation and the effect of chelation treatment on cerebral amyloid angiopathy compared to parenchymal amyloid plaques. Metallomics : integrated biometal science. 12, 539–546. doi: 10.1039/c9mt00306a
Keywords: Alzheimer's disease, iron metabolism, hub gene, diagnostic, drug, immune
Citation: Gu X, Lai D, Liu S, Chen K, Zhang P, Chen B, Huang G, Cheng X and Lu C (2022) Hub Genes, Diagnostic Model, and Predicted Drugs Related to Iron Metabolism in Alzheimer's Disease. Front. Aging Neurosci. 14:949083. doi: 10.3389/fnagi.2022.949083
Received: 23 May 2022; Accepted: 14 June 2022;
Published: 07 July 2022.
Edited by:
Tao Huang, Shanghai Institute of Nutrition and Health (CAS), ChinaReviewed by:
Meijing Li, Shanghai Maritime University, ChinaCopyright © 2022 Gu, Lai, Liu, Chen, Zhang, Chen, Huang, Cheng and Lu. This is an open-access article distributed under the terms of the Creative Commons Attribution License (CC BY). The use, distribution or reproduction in other forums is permitted, provided the original author(s) and the copyright owner(s) are credited and that the original publication in this journal is cited, in accordance with accepted academic practice. No use, distribution or reproduction is permitted which does not comply with these terms.
*Correspondence: Changlian Lu, bHZjbEBzdW1ocy5lZHUuY24=; Xiaoqin Cheng, Y3hxMTk4NTA0MjlAYWxpeXVuLmNvbQ==; Gang Huang, aHVhbmdnQHN1bWhzLmVkdS5jbg==; Xuefeng Gu, Z3V4ZkBzdW1ocy5lZHUuY24=
†These authors have contributed equally to this work
Disclaimer: All claims expressed in this article are solely those of the authors and do not necessarily represent those of their affiliated organizations, or those of the publisher, the editors and the reviewers. Any product that may be evaluated in this article or claim that may be made by its manufacturer is not guaranteed or endorsed by the publisher.
Research integrity at Frontiers
Learn more about the work of our research integrity team to safeguard the quality of each article we publish.