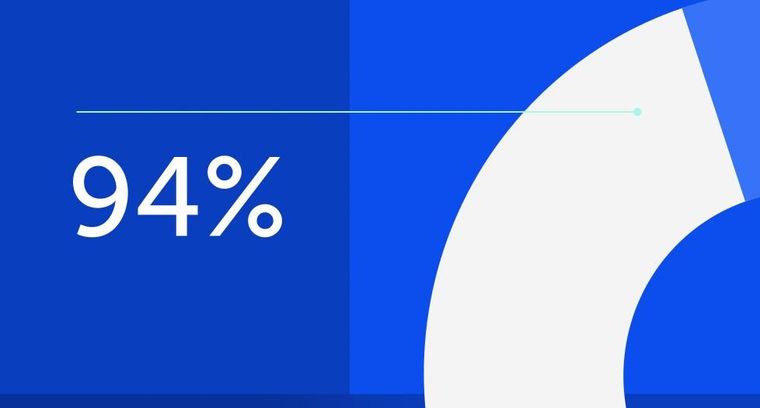
94% of researchers rate our articles as excellent or good
Learn more about the work of our research integrity team to safeguard the quality of each article we publish.
Find out more
ORIGINAL RESEARCH article
Front. Aging Neurosci., 03 August 2022
Sec. Cellular and Molecular Mechanisms of Brain-aging
Volume 14 - 2022 | https://doi.org/10.3389/fnagi.2022.938117
This article is part of the Research TopicSystem Approaches to Advance Drug Discovery Against Aging and Age-Related DiseasesView all 6 articles
Protein structure is determined by the amino acid sequence and a variety of post-translational modifications, and provides the basis for physiological properties. Not all proteins in the proteome attain a stable conformation; roughly one third of human proteins are unstructured or contain intrinsically disordered regions exceeding 40% of their length. Proteins comprising or containing extensive unstructured regions are termed intrinsically disordered proteins (IDPs). IDPs are known to be overrepresented in protein aggregates of diverse neurodegenerative diseases. We evaluated the importance of disordered proteins in the nematode Caenorhabditis elegans, by RNAi-mediated knockdown of IDPs in disease-model strains that mimic aggregation associated with neurodegenerative pathologies. Not all disordered proteins are sequestered into aggregates, and most of the tested aggregate-protein IDPs contribute to important physiological functions such as stress resistance or reproduction. Despite decades of research, we still do not understand what properties of a disordered protein determine its entry into aggregates. We have employed machine-learning models to identify factors that predict whether a disordered protein is found in sarkosyl-insoluble aggregates isolated from neurodegenerative-disease brains (both AD and PD). Machine-learning predictions, coupled with principal component analysis (PCA), enabled us to identify the physiochemical properties that determine whether a disordered protein will be enriched in neuropathic aggregates.
Proteins play critical and significant roles in every regulatory network that governs an organism’s cellular and physiological functions. Protein folding is a critical step in achieving a functional state; each protein transitions under physiological conditions to attain the conformation with the lowest possible free energy (Rose et al., 2006). Proteins that never attain a stable folded conformation, and that lack rigid tertiary or quaternary three-dimensional structures, are termed intrinsically disordered proteins (IDPs). IDP conformations are not fixed by the thermodynamics of single native proteins, but are able to vary due to protein-protein or protein-ligand interactions, and/or as a result of post-translational modifications (PTMs). Nearly a third of all proteins in the human proteome have been classified as unstructured or intrinsically disordered proteins (Ali and Ivarsson, 2018; Deiana et al., 2019). These IDPs play important roles in multiple physiological processes such as vesicular transport and signal transduction, and are also prominent in neurodegenerative pathology. Protein folding to attain minimal free energy is assisted by a variety of chaperone proteins. Any aberration in the protein folding process may lead to accumulation of unfolded/misfolded proteins, resulting in “Endoplasmic Reticulum stress” (ER stress).
Like most proteins, IDPs are susceptible to multiple PTMs; in neurological diseases, excessive PTMs may alter protein structure, favor binding to novel partners, and promote aggregation. Neurons have extensive protein-repair capacity that helps them to detect and salvage misfolded proteins, thereby preventing or reducing ER stress and thus ameliorating neurological damage (Jellinger, 2010; Cristofani et al., 2020). The accumulation of misfolded or intrinsically disordered proteins is recognized as a common characteristic of many neurodegenerative diseases (Uversky, 2015; Ayyadevara et al., 2021). IDPs have unique plasticity, conformational adaptability, and ability to bind to multiple partners — conferred by diverse properties such as structural malleability, low hydrophobicity, high solvent-accessible surface area, and high abundance of charged and polar residues (Wright and Dyson, 2015; Salvi et al., 2019).
Misfolded proteins are also known to cause cytotoxicity through toxic gain of function (Soto and Pritzkow, 2018). Many misfolded proteins/peptides such as Aβ1–42, hyper-phosphorylated tau (hP-tau), α-synuclein, and others, are involved in synaptic signaling pathways (Ashraf et al., 2014). For example, α-synuclein is a presynaptic protein that can relocate to mitochondria where it disrupts protein import; whereas mutated or hyperphosphorylated tau disrupts microtubule function (Stefanis, 2012; Melo et al., 2018). Liquid-liquid phase separation is an early event in the formation of aggregates featuring key neuropathology-associated proteins such as α-synuclein in Parkinson’s disease (Ray et al., 2020). Microtubule-associated protein tau normally stabilizes neuronal microtubules, but over time, and especially when hyperphosphorylated, tau can undergo liquid-liquid phase separation leading to microtubule nucleation and irreversible aggregation (Wheeler, 2020). Numerous misfolded proteins appear among the constituents of aggregates associated with Alzheimer’s disease (AD) (Ayyadevara et al., 2016a). Although specific aggregate components distinguish among different neurological diseases, such as AD, Parkinson’s disease (PD), and Amyotrophic Lateral Sclerosis (ALS), these pathologies all involve similar processes of protein misfolding and aggregate accrual. Although disease-associated aggregate proteins exhibit considerable diversity in sequence, size, structure, and function, after misfolding most form intermolecular β-sheet-rich structures ranging from small oligomers to large aggregates. Since not all disordered proteins end up in aggregates, we sought to identify properties that distinguish disordered proteins that are destined for aggregation, from those that are not associated with neuropathology.
The DisProt database1 is a manually curated database of intrinsically disordered proteins. DisProt has been updated over the last 14 years, including addition of attributes such as structural/functional aspects of protein domains. DisProt has its own set of descriptors for each protein, including state(s), state transitions, and “disorder ontology.” Twenty three disordered proteins were selected from DisProt to test the effects of their knockdowns on aggregation and aggregation-dependent traits in C. elegans models of neuropathogenic aggregation.
All C. elegans strains were grown under standard conditions at 20°C unless otherwise noted. Four transgenic strains were used in this study. (i.) CL2355 [pCL45 (snb-1:Aβ1–42: 3’ UTR (long) + mtl-2:gfp], a strain with pan-neuronal expression of a human Aβ1–42 transgene, causing deficits in chemotaxis, associative learning, and thrashing in liquid media. (ii.) CL4176 [dvIs27; myo-3p:Aβ1–42:let-851 3’ UTR) + rol-6(su1006)], a strain with muscle expression of human Aβ1–42. CL2355 and CL4176 produce low levels of Aβ1–42 at 20°C but progress to chemotaxis or paralysis, respectively, with age or after upshift to 25.5°C (Balasubramaniam et al., 2019; Ayyadevara et al., 2021). (iii.) NL5901 [unc-54p:α-synuclein:yfp + unc-119(+)] expresses YFP-tagged human α-synuclein in muscle, resulting in progressive paralysis. (iv.) AM141 [unc-54/q40:yfp] expresses Q40:YFP in muscle, leading to adult accumulation of YFP-fluorescent foci and late paralysis. All strains were obtained from the Caenorhabditis Genetics Center. Escherichia coli strain OP50 was replaced as the bacterial food source for RNAi exposure by E. coli HT115, harboring a vector that expresses RNAi constructs as dsRNA; these substrains were selected from the Ahringer RNAi Library (Kamath and Ahringer, 2003).
Selected genes, encoding IDPs listed in DisProt that were also implicated by proteomics showing enrichment in both Parkinson’s and Alzheimer’s aggregates (Balasubramaniam et al., 2019), were subjected to RNAi-mediated knockdown by feeding them target-specific RNAi bacteria from the Ahringer library (Kamath and Ahringer, 2003). Synchronously harvested eggs were transferred to plates seeded with selected sublines of E. coli strain HT115 (DE3) that transcribe double-stranded RNA corresponding to an exonic segment of each targeted gene, cloned into the L4440 plasmid multiple-cloning site (Kamath and Ahringer, 2003). Control worms were fed bacteria carrying L4440 without an exonic insert (“feeding vector” or “FV” controls).
Synchronous cohorts of the CL4176 strain, expressing Aβ1–42 in body-wall muscle, were initiated by lysing adult worms in alkaline sodium hypochlorite solution (Ayyadevara et al., 2001, 2008). Unlaid eggs recovered from lysed worms were transferred onto 60-mm agar plates seeded with bacteria expressing dsRNAs against targeted genes (see preceding section). Worms in all groups were upshifted from 20° to 25.5°C at the L3/L4 transition (47–49 h after lysis of parental worms) to induce expression of Aβ1–42 (Ayyadevara et al., 2016a). Paralysis of worms (defined as loss of touch-response motility) was scored at 19, 27, and 42 h post-upshift, until the longest-surviving group exceeded 50% mortality. To slow development of progeny in synchronized populations, 5-fluoro-2’-deoxyuridine (FUdR) was added to RNAi plates and control (FV) plates, at a final concentration of 2 μM, each containing worms from pre-gravid (L4/adult molt, day 2.5 post-hatch) through post-gravid ages (beyond 6–7 days post-hatch).
Aggregates in strain AM141, expressing Q40:YFP in muscle which forms punctate aggregates in adult worms, were analyzed for number and intensity of aggregates using FIJI (ImageJ2) (Schneider et al., 2012). Parameters and exposure were kept constant for each experiment to avoid bias. To visualize GFP aggregates, worms were collected and immobilized using sodium azide, and their images captured at 10× magnification using a Keyance fluorescence microscope. Counts of Q40:YFP aggregates per worm, and total punctate fluorescence per worm, were calculated for 4–8 worms per field, and 5–6 fields per group.
Alpha synuclein inclusions in body-wall muscle of strain NL5901 were analyzed for the average intensity of YFP expression, using FIJI (ImageJ2) while maintaining uniform conditions. Very similar results were obtained with strain OW13 (a genetically identical construct created independently; data not shown). Each experiment was initiated with synchronized eggs from well-fed worms, lysed with alkaline hypochlorite; eggs were transferred to NGM plates seeded with OP50 for maintenance, or HT115 for knockdown experiments. RNAi KD worms were grown on different RNAi-expressing HT115 clones, at 20°C. Images of worms were captured at adult days 1 and 5 (24 h or 5 days after the L4/adult molt) to quantify α-synuclein aggregation based on punctate YFP signal.
Parkinson’s disease (PD) aggregates were processed to separate sarkosyl-soluble from sarkosyl-insoluble aggregates. Recovered aggregates were digested with trypsin for proteomic analyses by LC-MS/MS as previously described (Ayyadevara et al., 2016b). Mass spectrometry data were collected, along with protein-sequence-based analyses, to compile descriptors (Table 3), and to calculate physiochemical properties and disorder scores, etc. using PONDR2, ESpritz Version 1.33, and Aggrescan4. PONDR derives 5 predictions from the FASTA sequence of each protein: VLXT, XL1_XT, CAN_XT, VL3-BA, and VLS2 (Xue et al., 2010). ESpritz produces protein disorder scores based on a choice of prediction tools (e.g., X-Ray, NMR, and Disprot) (Walsh et al., 2012), from which we selected NMR. Both PONDR and ESpritz were set to thresholds of 40% to predict disorder, but numerical output values were used as inputs to NN and SVM. These packages were augmented with Python code to calculate hydrophobicity, aromaticity, percent of individual amino acids, percent basic and acidic amino acids, etc. PSPredictor5, a second-generation, sequence-based tool to predict the potential of each protein for liquid-liquid phase-separation (Chu et al., 2022), was set to ≥ 0.5 threshold; actual numerical output values were used as inputs to NN and SVM.
Algorithms for Neural Network (NN), Support Vector Machine (SVM), and Principal Component Analysis (PCA) were implemented, trained and tested using Orange™ software (Demsar et al., 2013) to generate and visualize the outputs.
For Neural Networks, Orange™ employs a multilayer perceptron algorithm with back-propagation, splitting the dataset randomly 80:20 into training and testing sets. NN was assessed with a range of input parameters (Supplementary Table 1). The configuration with highest AUC had 300 hidden layers and 1350 iterations; activation method was set to “ReLu,” solver selected as “SGD” and numerical tolerance was set to 0.0005 (Deng et al., 2015).
Support Vector Machine (SVM) is a machine-learning method used for classification, regression and outlier detection, in which linear regression is performed in a high-dimension feature space. Where possible, SVM imputes missing values as means of existing values; otherwise, SVM removes instances with unknown target values and empty columns. SVM was assessed with a variety of input parameters (Supplementary Table 2), selecting those producing the maximal AUC (cost = 0.30, regression loss = 0.40, kernel = 0.01, numerical tolerance = 0.00011, iterations = 100).
Principal component analysis (PCA) is a stepwise, forward/reverse multivariate linear regression performed within Orange™ (Demsar et al., 2013) to identify orthogonal clusters of input parameters. These clusters collapse highly correlated predictors into a minimal set of uncorrelated (“orthogonal”) predictor dimensions computed from network graphs for the nodes of interest (Jolliffe and Cadima, 2016). PCA applies linear transformations to fit all 49 predictors into a coordinate system in which the most significant variance component is represented by the first component (PC1), and each successive component is orthogonal to all others and accounts for a smaller fraction (%) of total variance (Giuseppe et al., 2013). The first six PCs accounted for >88% of total variance.
Simulations were performed using the GROMACS simulation package implemented via the WebGRO server6 developed in-house. Each target protein was immersed in a triclinic box containing simple point charge (SPC) water. GROMOS96 43A force field was added evenly to the simulation system. The simulation system was neutralized by adding NaCl as counterions, and NaCl was supplemented to 0.15 M to approximate the physiological salt concentration. The whole system was energy minimized using Steepest Descent method for 5000 steps, and then equilibrated using the NVT/NPT method for 300 picoseconds. Each MD run used the leap-frog integrator for 200 ns; simulation trajectories were identified with the GROMACS trajectory analysis package and plotted using XMGRACE.
Equal numbers of synchronized L1 worms (strain AM141, expressing unc54/q40:yfp in muscle) were placed on 100-mm agar plates and maintained at 20°C. Worms matured into gravid adults in 2.5 days, and the number of progeny produced during days 5 and 6 post-hatch were counted for triplicate plates, calculating the average number of progeny per plate per day. Significance was determined by a 2-tailed heteroscedastic t-test.
To investigate the roles of disordered proteins in aggregation, we assessed 23 high-confidence IDPs from DisProt, a manually curated IDP database. The selected IDPs were tested in C. elegans strain CL2355, after induction of human Aβ1–42 peptide synthesis in all neurons. RNAi-mediated knockdown of each gene was initiated at hatch, and day-5 post-hatch worms were assayed for chemotaxis toward n-butanol, a behavior that deteriorates gradually with age and acutely upon induced neuronal expression of the Aβ1–42 peptide (Balasubramaniam et al., 2019). Of 23 tested IDP knockdowns, 12 (53%) conferred significant protection against loss of chemotaxis following Aβ aggregate formation, relative to controls, whereas 5 knockdowns had no effect or may have slightly worsened the trait (Figure 1A). For the 12 protective knockdowns, chemotaxis rescued 15–51% of the deficit attributed to neuronal Aβ1–42 expression, implying that each protein contributes to neuronal Aβ1–42 aggregation and the associated loss of chemotaxis. It is of particular interest that knockdown of 11 other genes encoding disordered proteins (RPAB3, GRB14, ATP7A, ITF2, PO2F1, CALR, P53, APEX, ESR1, VAMP, and TNNI3) did not significantly improve chemotaxis, suggesting that their encoded proteins contribute little to chemosensory deficit caused by Aβ1–42 aggregation, or are functionally redundant with other genes or pathways.
Figure 1. Aggregate abatement by knockdown of intrinsically disordered proteins (IDPs) from Disprot. (A) Histogram showing means ± SEM for calculated average chemotaxis of Caenorhabditis elegans strain CL2355 [Aβ1–42:3’UTR(long)], a model featuring pan-neuronal expression of human Aβ1–42 peptide, which leads to a 50 – 60% deficit in chemotaxis relative to young-adult wild-type worms (N = 100–150 worms per group). (B) Histogram show mean ± SEM for paralysis of C. elegans strain CL4176, an induced-Aβ1–42 model of AD-like amyloid deposition after muscle expression of human Aβ1–42 (N = 200–250 worms per group). Significances of differences from controls by heteroscedastic, 2-tailed t tests are *P ≤ 0.05; **P ≤ 0.01; ***P ≤ 0.0001; ****P ≤ 0.00001. Red asterisks indicate values significantly below controls.
We next evaluated the roles of these disordered proteins in a C. elegans model of muscle Aβ1–42 aggregation. We knocked down expression of the same disordered proteins in C. elegans strain CL4176, which expresses Aβ1–42 peptide in muscle, leading to Aβ1–42 aggregation and ensuing paralysis. The fraction of paralyzed worms was assayed in day-4.5 adults (i.e., 47 hr. after the L4/adult molt; Figure 1B). Sixteen of 23 knockdowns (70%) elicited significant rescue, restoring 23 – 78% of the motility lost due to Aβ1–42-mediated aggregation in muscle. However, five knockdowns did not cause a significant shift in paralysis, and two (P53 and APEX) significantly decreased motility (red asterisks in Figure 1B).
Although the two assays evaluated disparate effects of Aβ1–42 aggregation in different tissues, the Pearson correlation between these results is significant (RP = 0.59; P < 0.0025). We recognize that the efficiency of RNAi knockdown may be reduced for some neuronal genes. The 23 IDPs listed in the paper include 18 with documented neuronal expression (see WormBase.org), of which 16 (89%) were successfully suppressed by RNAi, whereas for the 5 gene targets with no detectable neuronal expression, only one (20%) impacted the chemotaxis phenotype upon knockdown. As summarized in Table 1, RNAi knockdown of neuronally expressed genes was nearly 6-fold more effective in disrupting a behavioral trait (chemotaxis) than was KD of neuronally silent genes (P < 0.004). In contrast, there was a 2.4-fold change in KD efficacy (not significant) for paralysis, a trait mediated by genes expressed in both muscle cells and neurons (Table 1). Together, these data imply that disordered proteins play crucial roles in aggregate formation across diverse neurodegenerative-disease models, impacting motility and chemosensory behavior, along with other physiological processes.
Table 1. RNAi knockdowns have far greater effects on a neuronally-mediated trait (chemotaxis) for KD targets with documented neuronal expression.
We observed a substantial number of intrinsically disordered proteins in AD hippocampal aggregates (Ayyadevara et al., 2016a), many of which had also been previously identified in aggregates isolated from other neurodegenerative diseases (Vaquer-Alicea and Diamond, 2019) and also found in aging human skeletal muscle, and hearts and brains from aged or AD-model mice (Ayyadevara et al., 2016b,2019; Kakraba et al., 2019). These commonalities suggested that similar processes may be involved in a diverse array of age-associated pathologies, possibly involving a conserved set of IDPs. To pursue that possibility, we asked whether PD brain aggregates are also enriched for disordered proteins. We predicted the disordered fraction of each protein identified by proteomic analysis of Parkinson’s disease tissue, in both soluble and detergent-insoluble aggregates, using the PONDR and ESpritz on-line servers (Xue et al., 2010; Walsh et al., 2012). Results were similar; only PONDR outputs are shown here.
These servers predicted that 53% of the proteins identified in Parkinson’s aggregates have at least 40% disorder, an enrichment >1.7-fold above the 31–32% predicted for human proteins overall (Ali and Ivarsson, 2018; Deiana et al., 2019) (Chi-squared P < 10–4). Of 845 IDP proteins enriched in insoluble aggregates from PD, 632 (75%) were also significantly enriched in AD aggregates (each relative to similar aggregates from age-matched controls) (Ayyadevara et al., 2016a). A subset of 197 aggregate-enriched proteins shared by AD and PD, selected for a broad range of disorder scores (Table 2), indicates very little correlation between AGGRESCAN-predicted aggregation propensity and estimated disorder (RP = 0.095).
Table 2. Selected proteins shared by Alzheimer’s disease (AD) and Parkinson’s disease (PD) aggregates, with a range of disorder scores.
The above data on IDPs led us to re-evaluate the relationship between the disorder level and other properties of a protein, and its tendency to enter into aggregates. To understand which physiochemical properties might favor aggregation of disordered proteins, we selected 400 disordered proteins enriched in both PD and AD aggregates, and compiled the numbers of spectral hits for each such protein in the detergent-soluble and -insoluble fractions. The resulting dataset also listed 49 distinct physiochemical properties for each protein, including disorder score, hydrophobicity, aggregation score, aromaticity, and percentage of several other key amino acids. Machine-learning software then randomly partitioned the list 80:20, into sets used for training and testing respectively; this process (partitioning, training and testing) was repeated for 50 permutations. We then categorized the proteins based on their PD-aggregate spectral hits into four groups: “INSOL,” where the protein is only found in insoluble aggregates; “SOL,” where the protein is found only in soluble aggregates; “BOTH,” where the protein is found in both soluble and insoluble aggregates; and “NOAGG” for proteins not found in any aggregates.
We trained Principal Component Analysis (PCA), neural-network (NN), and support-vector-machine (SVM) algorithms to predict a protein’s probability of entering aggregates. The neural-network and SVM predictions resulted in 79.5 and 80% accuracy, respectively, for the testing groups — indicating equivalent performance. This suggests that, among 49 input descriptors, there may be a subset of properties that determine aggregation propensity of disordered proteins, supporting our hypothesis that disorder alone does not dictate aggregation. The PCA, NN, and SVM algorithms used physiochemical properties, including net hydrophobicity, % acidic residues, % basic residues, % charged and uncharged residues, total aggregation-prone expanse, total hot-spot aggregation expanse, total number of disordered segments, longest disordered region, and overall% disorder, augmented by in silico predictors of protein disorder (Table 3).
To identify a minimal set of descriptors or properties required for prediction accuracy, we used Principal Component Analysis (PCA) to compare predictive models. Disorder scores generated by the PONDR program, which itself uses neural networks to identify disordered protein regions, was the most influential predictor of aggregate inclusion (PC1 in Table 3), followed by spectral counts in α-synuclein insoluble aggregates (PC2). The first 6 principal components accounted for > 88% of dataset variance. Limiting descriptor inputs to the top 3 PCA components reduced SVM and NN accuracy (AUC) by a further 4.6% (see Table 4). A 2-dimensional partitioning of proteins by aggregation propensity, based on the first two components, is illustrated in Figure 2A. Predictions using both PC1 and PC2 show a correlation coefficient R of 0.895, and R2 = 0.80, with actual detection in PD and AD aggregates. Receiver-Operating Characteristic (ROC) analyses, plotting sensitivity vs. specificity, are displayed for neural network and support vector machine predictions for each of the classes BOTH, INSOL, and NOAGG (Figures 2B–D, respectively). The SOL category was poorly resolved from other classes (Supplementary Table 3). We also predicted potential liquid-liquid phase separation for each protein in the dataset using PSPredictor. PSPredictor scores correlated fairly well with NN and SVM predictions, producing linear (Pearson) correlation coefficients of 0.75 and 0.85 with NN and SVM predictions, respectively. Spearman rank-order correlations were slightly higher at 0.81 for NN and 0.90 for SVM predictions (Supplementary Table 4, last line).
Figure 2. Data partitioning based on the first two principal component analysis (PCA) components. (A) Scatter-plot illustrating 2-dimensional sorting of intrinsically disordered proteins (IDPs) based on the first two components, PC1 and PC2, into categories INSOL (sarkosyl-insoluble aggregates), NOAGG (not aggregated), and BOTH (included in both soluble and insoluble aggregates). (B–D) Receiver-Operating Characteristic (ROC) analysis of the 3 classes, INSOL, NOAGG, and BOTH, showing similar curves for NN and SVM, for total positives (TP, y axis) vs. false positives (FP, x axis) — representing sensitivity and specificity, respectively.
Since the majority of disordered proteins are predicted to be structurally unstable, and are thus likely to unfold more readily than other proteins (Uversky, 2019), we analyzed the structural dynamics of several IDPs that were enriched in both PD and AD insoluble aggregates. We selected tubulin beta-4A chain (TUBB4A), a disordered monomer that polymerizes into the highly ordered and stable microtubule structure; glial fibrillary acidic protein (GFAP), an intermediate filament protein with a disorder score of ∼86%; RAP2A, a small GTP-binding protein related to Ras, which forms a signaling complex with NEDD4 and TNIK regulating neuronal dendrite morphogenesis; and three 14-3-3 paralogs (α, γ, and σ), members of a small family of conserved signaling molecules responsive to protein phosphorylation. Each of these aggregate-enriched proteins was consistently abundant (50–485 spectral counts) in each of the immunopurified aggregate types (sarkosyl-insoluble aggregates isolated by antibody affinity for α-synuclein, Aβ1–42, or tau), with the exception of RAP2A, which was substantially less abundant (25 total hits). Spectral counts for individual proteins in each aggregate class were roughly in proportion to total sarkosyl-insoluble aggregate protein (see percentages in Table 5).
We predicted structural dynamics of these six proteins using atomistic molecular-dynamic simulations of the monomeric forms, conducted for 200 ns in triplicate (Figure 3). All six proteins are predicted to show RMSD instability, based on average tracings of three 200-ns simulations for each protein. Tubulin β chain 4B is the most stable of these, but nevertheless undergoes RMSD fluctuations of 10–20% for at least 200 ns (Figure 3A). The RMSD of GFAP continued to expand throughout the 200-ns simulations, indicative of progressive unfolding (Figure 3B). RAP2A showed RMSD fluctuations of > 50%, and beyond ∼70 ns it appeared to oscillate between two or more metastable conformations (Figure 3C). The three 14-3-3 paralogs (Figures 3D–F) were predicted to expand progressively over the course of the simulations.
Figure 3. RMSD plots of disordered proteins. Intrinsically disordered protein (IDP) structures were simulated for 200 ns: (A) Tubulin 4B; (B) glial fibrillary acidic protein (GFAP); (C) RAP2A; (D) 14-3-3α (alpha); (E) 14-3-3γ (gamma); (F) 14-3-3σ (sigma). RMSD instability reflects random structural perturbations over time.
We previously reported that RNAi knockdowns that suppress expression of orthologs of AD aggregate-enriched proteins conferred significant protection from pathology-associated outcomes in C. elegans models of neurodegenerative aggregation (Ayyadevara et al., 2015, 2016a,2017, 2021). To assess whether knockdowns are similarly protective for target IDPs implicated by our SVM and NN algorithms, we quantified aggregate formation and progeny production after RNAi-mediated knockdown of C. elegans orthologs of six representative IDP genes with ≥ 40% disorder, enriched in both AD and PD aggregates (DHX9, PLEC, FABPH, TUBB4, GFAP, and MPPA).
We first assessed the effects of knockdowns targeting orthologs of these IDPs in a C. elegans model of α-synuclein aggregation, characteristic of PD. To visually monitor the consequences of each knockdown, we employed C. elegans strain NL5901, expressing α-synuclein fused to yellow fluorescent protein [unc-54p:alpha-synuclein:yfp + unc-119 (+)] in body-wall muscle. We quantified YFP inclusions in muscle of control worms, vs. worms subjected to RNAi knockdowns targeting C. elegans orthologs of the 6 IDP genes that encode proteins enriched in AD and PD aggregates. RNAi exposure extended from hatch until aggregate assessment 5 days later. Based on mean YFP intensity per worm, these IDP knockdowns decreased α-synuclein aggregate load by 15–43% (Figure 4), with GFAP KD exerting the greatest effect, followed by PLEC.
Figure 4. Aggregation of human α-synuclein after KD of Alzheimer’s disease (AD)/ Parkinson’s disease (PD)-aggregate Intrinsically disordered proteins (IDPs). (A) Histograms for worms exposed to RNAis of IDPs at 20°C, imaged as day-5 post-hatch adults. Six IDP knockdowns were exposed continuously from hatch, to RNAi targeting IDPs shared by AD and PD aggregates. (B) Images are shown of Caenorhabditis elegans day-5 adults of strain NL5901 [unc-54p:alpha-synuclein:YFP + unc-119(+)], a model of PD-like α-synuclein aggregation, with YFP fluorescent foci appearing in body-wall muscle. Experimental groups differ from controls with significance based on heteroscedastic, 1-tailed t tests: *P ≤ 0.05; **P ≤ 0.005. FV-A and other images are shown at 4× magnification; FV-B is shown at 20× magnification.
We next assessed these same six IDPs in a C. elegans model of age-progressive, huntingtin-like aggregate formation (distinct fluorescent foci arising from YFP-tagged Q40 expressed in muscle). RNAi suppression of these IDPs decreased Q40:YFP punctate fluorescence per worm by 12–73% (Figures 5A,B). Both the number and intensity of aggregates were reduced by suppression of these IDPs, with GFAP and PLEC again exerting the greatest effect, followed closely by TUBB4A (tubulin β chain 4A) (Figure 5A). We noticed fewer progeny issuing from these KD groups, leading us to quantify fecundity (Figure 5C). Intriguingly, each IDP knockdown significantly lowered the average number of eggs laid on days 5 and 6 post-hatch, by 40–60% relative to progeny of feeding-vector control worms (P ≤ 0.001 to P ≤ 0.0001). This observation implies that each IDP contributes to fertility and/or development; that is, each gene product serves a positive function early in life, although most become deleterious subsequently.
Figure 5. Polyglutamine aggregation and fertility after KD of Alzheimer’s disease (AD)/Parkinson’s disease (PD)-aggregate IDPs. (A) Average aggregate fluorescence per worm in Caenorhabditis elegans strain AM141 (expressing q40:yfp in body-wall muscle), a model of HD huntingtin-like aggregation. Six IDPs were assessed following exposure to RNAi for the indicated IDPs, shared by both AD and PD. (B) Images are shown (10× magnification) of C. elegans AM141 adults, at 5 days post-hatch after 4 days exposure to RNAi targeting β-tubulin or GFAP. (C) Average number of eggs laid during days 5 and 6 post-hatch following knockdown with the indicated RNAi. Differences from controls are significant by heteroscedastic, 1-tailed t tests at *P ≤ 0.05; **P ≤ 0.01; ***P ≤ 0.0001.
By far the most influential descriptor, for prediction of aggregate inclusion, was the PONDR disorder score (PC1 in Table 3). This confirms that disorder is the predominant feature determining protein accretion into aggregates – which has been widely assumed and was strongly supported by our observation of highly significant IDP enrichment in protein aggregates. PC2 is simply the total insoluble-aggregate content of α-synuclein, which Table 5 reveals to be a reasonably good surrogate for the sarkosyl-insoluble protein content of any aggregate class. This supports our hypothesis that common processes of accrual mediate the formation of all aggregate varieties. Additional increments, although of ever-diminishing importance, are provided by PC3–PC6. It is noteworthy that all PCA dimensions are deemed to be independent of one another, meaning that there is no discernable correlation between disorder score (PC1), insoluble aggregate burden (PC2), the number of extensive disordered regions (PC3) or the% amphipathic, acidic, aromatic, or basic residues (PC3, PC5, PC6). AGGRESCAN provides a sequence-based prediction of aggregation propensity, which appears as PC4. At this level, we cannot be certain that this descriptor is truly orthogonal to all other input variables, but it is clear that AGGRESCAN provides less valuable information than PONDR (PC1) or aggregate burden (PC2).
Protein folding is obligatory for generation of functional proteins (Diaz-Villanueva et al., 2015). Most newly synthesized proteins will reach a native conformation upon completion of synthesis; proteins that are initially misfolded are assisted by chaperones to assume stable conformations (Hwang and Qi, 2018). Multiple mechanisms provide redundancy, and help to minimize the loss of functionally robust structures (Hwang and Qi, 2018); nevertheless, it is estimated that over half of newly synthesized proteins may be degraded co-translationally (Turner and Varshavsky, 2000). IDPs have been reported to be involved as key regulators of diverse and essential physiological processes including transcription, translation and cell signal transduction (Wright and Dyson, 2015). In a recent study, Cuevas-Velazques et al. demonstrated that IDPs coupled to fluorescent tags can serve as biosensors of osmotic stress (Cuevas-Velazquez et al., 2021). We here add the novel observation that IDPs contribute to reproductive potential (Figure 5C).
Multiple proteins, and especially IDPs, coalesce into aggregates, which also contain specific RNA and DNA sequences to which many IDPs bind (Shmookler Reis et al., 2021). Disease-associated aggregate components serve as diagnostic biomarkers for diverse neurodegenerative pathologies (Balasubramaniam et al., 2019; Ayyadevara et al., 2021). To evaluate the importance of these IDPs for aggregate formation and associated traits, we performed an initial screen in which IDPs from the DisProt database were individually knocked down in C. elegans by RNA interference. Most KDs conferred substantial protection against aggregate formation, and/or protected against age-progressive traits used as end-points in C. elegans models of diverse neurodegenerative diseases. We observed similar protection in studies of the human SERF2 protein (Balasubramaniam et al., 2018) and its C. elegans ortholog CRAM-1 (Ayyadevara et al., 2015). Each IDP KD conferred similar protection against aggregate accrual, a hallmark feature of aging and age-associated diseases (van Ham et al., 2010).
Numerous IDPs play key functional roles during high energy-demand states such as reproduction and response to stresses encountered during development. Nevertheless, the age-progressive increase in protein aggregation, which is largely post-reproductive, will be exacerbated by the tendency of these sticky proteins to interact non-randomly with other protein partners, or with RNA or DNA (Marcelo et al., 2021; Shmookler Reis et al., 2021), and in response to stressors in the cell environment (Balasubramaniam et al., 2019).
Individual IDPs may qualify as instances of “antagonistic pleiotropy” (Williams, 1957), wherein allele-specific survival and/or reproductive value drive natural selection early in life, unhindered by detrimental effects arising later. We found that 6 out of 6 IDP knockdowns reduce C. elegans reproductive fitness, evidenced by reduced fecundity (Figure 5C), despite decreasing protein aggregation (Figure 5A) and its deleterious sequelae that reduce long-term survival (Ayyadevara et al., 2016a,b). Antagonistic pleiotropy is not an obligatory property of natural gene variants, but is observed for a subset of longevity-associated alleles (Ayyadevara et al., 2001). We expect IDPs to play important roles in reproduction, perhaps due to their ability to bind multiple partners and thus coordinate multiple pathways. With aging, however, IDPs may become increasingly sensitive to progressive changes arising from oxidation and inflammation, ultimately impairing proteostasis (Kikis et al., 2010; Bektas et al., 2018). Non-random interactions of these IDPs with protein and nucleic-acid partners may contribute to aggregate initiation and progression (Stefanis, 2012; Ayyadevara et al., 2017, 2021; Uversky, 2019; Shmookler Reis et al., 2021). Examples of dysregulated IDPs include tau and Aβ1–42 in AD, TDP-43 in ALS and other diseases, and α-synuclein in PD (Irwin et al., 2013; Burre et al., 2018). We found many IDPs enriched in aggregates from human-AD hippocampus and in diverse C. elegans models of human neuropathic aggregation (Ayyadevara et al., 2015).
Not all IDPs are enriched in aggregates, and so we sought to identify properties that determine whether proteins are incorporated into aggregates or excluded from them. We utilized tools developed previously to predict protein disorder and aggregation propensity (Conchillo-Sole et al., 2007; Xue et al., 2010), but tailored our approach to allow us to infer which IDP properties favor or disfavor their entry into PD and AD aggregates. We combined 3 machine-learning methods and 49 predictors (several of which were scores from other machine-learning algorithms) to predict whether an IDP will enter into detergent-insoluble or detergent-soluble aggregates.
This strategy has the important benefit of providing insights into the most influential factors used by NN or SVM algorithms. Our NN predictions suggested that a combination of crucial physiochemical properties of a disordered protein are, at least in part, responsible for entry of a disordered protein into aggregates. Properties such as the abundance of basic or aromatic residues are predicted to be among the crucial factors in determining a disordered protein’s aggregation propensity (Table 3). Some of our predictions are supported by real-world examples, including tau, TDP-43 and α-synuclein (Deckert et al., 2016). We used principal component analysis to reduce the number of orthogonal inputs (“dimensionality”) for neural-network and SVM algorithms, and thus to define a minimal set of non-redundant determinants necessary to predict IDP aggregation. This approach was successful, in that restricting inputs to the first 3 PCA components only reduced the accuracy of SVM and NN by <5% (Table 4), enabling us to conclude that expected disorder is the most influential predictor for aggregation of specific proteins, followed by relative aggregate burden (i.e., the overall protein content of any of 5 aggregate subtypes). Disorder appears to be, by far, the most influential factor, enhanced somewhat by aggregate abundance. These predictions benefited only rather modestly from a variety of sequence-based determinants of aggregation propensity.
It is intriguing that the predicted susceptibility of proteins to liquid-liquid phase separation (Deckert et al., 2016) correlated fairly well (RP = 0.746) with neural-network prediction of entry into observed AD and PD aggregates, and a bit better (RP = 0.853) with SVM predictions (Figure 6 and Supplementary Table 1). This suggests that the underlying “logic” employed by NN and SVM to predict aggregate inclusion, to some extent employs features also used by PSPredictor to assign likelihood of a liquid-liquid phase separation.
Figure 6. Scatter plots illustrate Spearman and Pearson correlations. (A,B) PSPredictor scores (Chu et al., 2022) (y axes) are plotted against aggregation propensities (x axes) predicted for 197 proteins, based on (A) neural network (NN), or (B) support vector machine (SVM). (C,D) Rank orders of PSPredictor scores (y axes) are plotted against rank orders of aggregation propensities (x axes) predicted for (C) neural network (NN), or (D) support vector machine (SVM). RP is the Pearson (linear) correlation coefficient; RS is the Spearman rank-order correlation coefficient.
The raw data supporting the conclusions of this article will be made available by the authors, without undue reservation.
Experiments were planned and interpreted by AG, MB, SA, and RJSR. Data were analyzed by AG and MB. The manuscript was written by AG, SA, and RJSR with input from MB on computational matters. All authors contributed to the article and approved the submitted version.
This research was supported by an R01 grants to SA and RJSR (AG062254) and a Merit Award to RJSR (I01 BX001655).
The authors declare that the research was conducted in the absence of any commercial or financial relationships that could be construed as a potential conflict of interest.
All claims expressed in this article are solely those of the authors and do not necessarily represent those of their affiliated organizations, or those of the publisher, the editors and the reviewers. Any product that may be evaluated in this article, or claim that may be made by its manufacturer, is not guaranteed or endorsed by the publisher.
The Supplementary Material for this article can be found online at: https://www.frontiersin.org/articles/10.3389/fnagi.2022.938117/full#supplementary-material
Ali, M., and Ivarsson, Y. (2018). High-throughput discovery of functional disordered regions. Mol. Syst. Biol. 14:e8377. doi: 10.15252/msb.20188377
Ashraf, G. M., Greig, N. H., Khan, T. A., Hassan, I., Tabrez, S., Shakil, S., et al. (2014). Protein misfolding and aggregation in Alzheimer’s disease and type 2 diabetes mellitus. CNS Neurol. Disord. Drug Targets 13, 1280–1293. doi: 10.2174/1871527313666140917095514
Ayyadevara, S., Alla, R., Thaden, J. J., and Shmookler Reis, R. J. (2008). Remarkable longevity and stress resistance of nematode PI3K-null mutants. Aging Cell 7, 13–22. doi: 10.1111/j.1474-9726.2007.00348.x
Ayyadevara, S., Ayyadevara, R., Hou, S., Thaden, J. J., and Shmookler Reis, R. J. (2001). Genetic mapping of quantitative trait loci governing longevity of Caenorhabditis elegans in recombinant-inbred progeny of a Bergerac-BO x RC301 interstrain cross. Genetics 157, 655–666. doi: 10.1093/genetics/157.2.655
Ayyadevara, S., Balasubramaniam, M., Gao, Y., Yu, L. R., Alla, R., and Shmookler Reis, R. J. (2015). Proteins in aggregates functionally impact multiple neurodegenerative disease models by forming proteasome-blocking complexes. Aging Cell 14, 35–48. doi: 10.1111/acel.12296
Ayyadevara, S., Balasubramaniam, M., Kakraba, S., Alla, R., Mehta, J. L., and Shmookler Reis, R. J. (2017). Aspirin-mediated acetylation protects against multiple neurodegenerative pathologies by impeding protein aggregation. Antioxid. Redox Signal. 27, 1383–1396. doi: 10.1089/ars.2016.6978
Ayyadevara, S., Balasubramaniam, M., Parcon, P. A., Barger, S. W., Griffin, W. S., Alla, R., et al. (2016a). Proteins that mediate protein aggregation and cytotoxicity distinguish Alzheimer’s hippocampus from normal controls. Aging Cell 15, 924–939. doi: 10.1111/acel.12501
Ayyadevara, S., Ganne, A., Balasubramaniam, M., and Shmookler Reis, R. J. (2021). Intrinsically disordered proteins identified in the aggregate proteome serve as biomarkers of neurodegeneration. Metab. Brain Dis. 37, 147–152, doi: 10.1007/s11011-021-00791-8
Ayyadevara, S., Ganne, A., Hendrix, R. D., Balasubramaniam, M. S., Shmookler Reis, R. J., and Barger, S. W. (2019). Functional assessments through novel proteomics approaches: application to insulin/IGF signaling in neurodegenerative disease. J. Neurosci. Methods 319, 40–46. doi: 10.1016/j.neumeth.2018.11.005
Ayyadevara, S., Mercanti, F., Wang, X., Mackintosh, S. G., Tackett, A. J., Prayaga, S. V., et al. (2016b). Age- and hypertension-associated protein aggregates in mouse heart have similar proteomic profiles. Hypertension 67, 1006–1013. doi: 10.1161/HYPERTENSIONAHA.115.06849
Balasubramaniam, M., Ayyadevara, S., and Shmookler Reis, R. J. (2018). Structural insights into pro-aggregation effects of C. elegans CRAM-1 and its human ortholog SERF2. Sci. Rep. 8:14891. doi: 10.1038/s41598-018-33143-1
Balasubramaniam, M., Ayyadevara, S., Ganne, A., Kakraba, S., Penthala, N. R., Du, X., et al. (2019). Aggregate interactome based on protein cross-linking interfaces predicts drug targets to limit aggregation in neurodegenerative diseases. iScience 20, 248–264. doi: 10.1016/j.isci.2019.09.026
Bektas, A., Schurman, S. H., Sen, R., and Ferrucci, L. (2018). Aging, inflammation and the environment. Exp. Gerontol. 105, 10–18. doi: 10.1016/j.exger.2017.12.015
Burre, J., Sharma, M., and Sudhof, T. C. (2018). Cell biology and pathophysiology of alpha-synuclein. Cold Spring Harb. Perspect. Med. 8:a024091. doi: 10.1101/cshperspect.a024091
Chu, X., Sun, T., Li, Q., Xu, Y., Zhang, Z., Lai, L., et al. (2022). Prediction of liquid-liquid phase separating proteins using machine learning. BMC Bioinform. 23:72. doi: 10.1186/s12859-022-04599-w
Conchillo-Sole, O., de Groot, N. S., Aviles, F. X., Vendrell, J., Daura, X., and Ventura, S. (2007). Aggrescan: a server for the prediction and evaluation of “hot spots” of aggregation in polypeptides. BMC Bioinform. 8:65. doi: 10.1186/1471-2105-8-65
Cristofani, R., Crippa, V., Cicardi, M. E., Tedesco, B., Ferrari, V., Chierichetti, M., et al. (2020). A crucial role for the protein quality control system in motor neuron diseases. Front. Aging Neurosci. 12:191. doi: 10.3389/fnagi.2020.00191
Cuevas-Velazquez, C. L., Vellosillo, T., Guadalupe, K., Schmidt, H. B., Yu, F., Moses, D., et al. (2021). Intrinsically disordered protein biosensor tracks the physical-chemical effects of osmotic stress on cells. Nat. Commun. 12:5438. doi: 10.1038/s41467-021-25736-8
Deckert, A., Waudby, C. A., Wlodarski, T., Wentink, A. S., Wang, X., Kirkpatrick, J. P., et al. (2016). Structural characterization of the interaction of alpha-synuclein nascent chains with the ribosomal surface and trigger factor. Proc. Natl. Acad. Sci. U.S.A. 113, 5012–5017. doi: 10.1073/pnas.1519124113
Deiana, A., Forcelloni, S., Porrello, A., and Giansanti, A. (2019). Intrinsically disordered proteins and structured proteins with intrinsically disordered regions have different functional roles in the cell. PLoS One 14:e0217889. doi: 10.1371/journal.pone.0217889
Demsar, J., Curk, T., Erjavec, A., Gorup, C., Hocevar, T., Milutinovic, M., et al. (2013). Orange: data mining toolbox in Python. J. Mach. Learn. Res. 14, 2349–2353.
Deng, X., Gumm, J., Karki, S., Eickholt, J., and Cheng, J. (2015). An overview of practical applications of protein disorder prediction and drive for faster, more accurate predictions. Int. J. Mol. Sci. 16, 15384–15404. doi: 10.3390/ijms160715384
Diaz-Villanueva, J. F., Diaz-Molina, R., and Garcia-Gonzalez, V. (2015). Protein folding and mechanisms of proteostasis. Int. J. Mol. Sci. 16, 17193–17230. doi: 10.3390/ijms160817193
Giuseppe, P., Marano, B., and Biganzoli, E. M. (2013). Estimation of the piecewise exponential model by Bayesian P-splines via gibbs sampling: robustness and reliability of posterior estimates. Open J. Stat. 6, 451–468. doi: 10.4236/ojs.2016.63041
Hwang, J., and Qi, L. (2018). Quality control in the endoplasmic reticulum: crosstalk between ERAD and UPR pathways. Trends Biochem. Sci. 43, 593–605. doi: 10.1016/j.tibs.2018.06.005
Irwin, D. J., Lee, V. M., and Trojanowski, J. Q. (2013). Parkinson’s disease dementia: convergence of alpha-synuclein, tau and amyloid-beta pathologies. Nat. Rev. Neurosci. 14, 626–636. doi: 10.1038/nrn3549
Jellinger, K. A. (2010). Basic mechanisms of neurodegeneration: a critical update. J. Cell Mol. Med. 14, 457–487. doi: 10.1111/j.1582-4934.2010.01010.x
Jolliffe, I. T., and Cadima, J. (2016). Principal component analysis: a review and recent developments. Philos. Trans. A Math. Phys. Eng. Sci. 374:20150202. doi: 10.1098/rsta.2015.0202
Kakraba, S., Ayyadevara, S., Penthala, N. R., Balasubramaniam, M., Ganne, A., Liu, L., et al. (2019). A novel microtubule-binding drug attenuates and reverses protein aggregation in animal models of Alzheimer’s Disease. Front. Mol. Neurosci. 12:310. doi: 10.3389/fnmol.2019.00310
Kamath, R. S., and Ahringer, J. (2003). Genome-wide RNAi screening in Caenorhabditis elegans. Methods 30, 313–321. doi: 10.1016/S1046-2023(03)00050-1
Kikis, E. A., Gidalevitz, T., and Morimoto, R. I. (2010). Protein homeostasis in models of aging and age-related conformational disease. Adv. Exp. Med. Biol. 694, 138–159. doi: 10.1007/978-1-4419-7002-2_11
Marcelo, A., Koppenol, R., de Almeida, L. P., Matos, C. A., and Nobrega, C. (2021). Stress granules, RNA-binding proteins and polyglutamine diseases: too much aggregation? Cell Death Dis. 12:592. doi: 10.1038/s41419-021-03873-8
Melo, T. Q., Copray, S., and Ferrari, M. F. R. (2018). Alpha-Synuclein toxicity on protein quality control, mitochondria and endoplasmic reticulum. Neurochem. Res. 43, 2212–2223. doi: 10.1007/s11064-018-2673-x
Ray, S., Singh, N., Kumar, R., Patel, K., Pandey, S., Datta, D., et al. (2020). Alpha-Synuclein aggregation nucleates through liquid-liquid phase separation. Nat. Chem. 12, 705–716. doi: 10.1038/s41557-020-0465-9
Rose, G. D., Fleming, P. J., Banavar, J. R., and Maritan, A. (2006). A backbone-based theory of protein folding. Proc. Natl. Acad. Sci. U.S.A. 103, 16623–16633. doi: 10.1073/pnas.0606843103
Salvi, N., Abyzov, A., and Blackledge, M. (2019). Solvent-dependent segmental dynamics in intrinsically disordered proteins. Sci. Adv. 5:eaax2348. doi: 10.1126/sciadv.aax2348
Schneider, C. A., Rasband, W. S., and Eliceiri, K. W. (2012). NIH Image to ImageJ: 25 years of image analysis. Nat. Methods 9, 671–675. doi: 10.1038/nmeth.2089
Shmookler Reis, R. J., Atluri, R., Balasubramaniam, M., Johnson, J., Ganne, A., and Ayyadevara, S. (2021). “Protein aggregates” contain RNA and DNA, entrapped by misfolded proteins but largely rescued by slowing translational elongation. Aging Cell 20:e13326. doi: 10.1111/acel.13326
Soto, C., and Pritzkow, S. (2018). Protein misfolding, aggregation, and conformational strains in neurodegenerative diseases. Nat. Neurosci. 21, 1332–1340. doi: 10.1038/s41593-018-0235-9
Stefanis, L. (2012). Alpha-Synuclein in Parkinson’s disease. Cold Spring Harb. Perspect. Med. 2:a009399. doi: 10.1101/cshperspect.a009399
Turner, G. C., and Varshavsky, A. (2000). Detecting and measuring cotranslational protein degradation in vivo. Science 289, 2117–2120. doi: 10.1126/science.289.5487.2117
Uversky, V. N. (2015). Intrinsically disordered proteins and their (disordered) proteomes in neurodegenerative disorders. Front. Aging Neurosci. 7:18. doi: 10.3389/fnagi.2015.00018
Uversky, V. N. (2019). Intrinsically disordered proteins and their “Mysterious” (Meta)Physics. Front. Phys. 7:10. doi: 10.3389/fphy.2019.00010
van Ham, T. J., Holmberg, M. A., van der Goot, A. T., Teuling, E., Garcia-Arencibia, M., Kim, H. E., et al. (2010). Identification of MOAG-4/SERF as a regulator of age-related proteotoxicity. Cell 142, 601–612. doi: 10.1016/j.cell.2010.07.020
Vaquer-Alicea, J., and Diamond, M. I. (2019). Propagation of protein aggregation in neurodegenerative diseases. Annu. Rev. Biochem. 88, 785–810. doi: 10.1146/annurev-biochem-061516-045049
Walsh, I., Martin, A. J., Di Domenico, T., and Tosatto, S. C. (2012). ESpritz: accurate and fast prediction of protein disorder. Bioinformatics 28, 503–509. doi: 10.1093/bioinformatics/btr682
Wheeler, R. J. (2020). Therapeutics-how to treat phase separation-associated diseases. Emerg. Top. Life Sci. 4, 307–318. doi: 10.1042/ETLS20190176
Williams, G. C. (1957). Pleiotropy, natural selection, and the evolution of senescence. Evolution 11, 398–411. doi: 10.2307/2406060
Wright, P. E., and Dyson, H. J. (2015). Intrinsically disordered proteins in cellular signalling and regulation. Nat. Rev. Mol. Cell Biol. 16, 18–29. doi: 10.1038/nrm3920
Keywords: proteostasis, misfolding and aggregation, Alzheimer’s disease, Parkinson’s disease, drug screening and discovery, intrinsically disordered proteins (IDPs), neural network, support vector machine
Citation: Ganne A, Balasubramaniam M, Ayyadevara S and Shmookler Reis RJ (2022) Machine-learning analysis of intrinsically disordered proteins identifies key factors that contribute to neurodegeneration-related aggregation. Front. Aging Neurosci. 14:938117. doi: 10.3389/fnagi.2022.938117
Received: 07 May 2022; Accepted: 04 July 2022;
Published: 03 August 2022.
Edited by:
Alexey Moskalev, Komi Scientific Center (RAS), RussiaReviewed by:
Mali Jiang, Johns Hopkins University, United StatesCopyright © 2022 Ganne, Balasubramaniam, Ayyadevara and Shmookler Reis. This is an open-access article distributed under the terms of the Creative Commons Attribution License (CC BY). The use, distribution or reproduction in other forums is permitted, provided the original author(s) and the copyright owner(s) are credited and that the original publication in this journal is cited, in accordance with accepted academic practice. No use, distribution or reproduction is permitted which does not comply with these terms.
*Correspondence: Srinivas Ayyadevara, QXl5YWRldmFyYVNyaW5pdmFzQHVhbXMuZWR1; Robert J. Shmookler Reis, cmpzckB1YW1zLmVkdQ==
Disclaimer: All claims expressed in this article are solely those of the authors and do not necessarily represent those of their affiliated organizations, or those of the publisher, the editors and the reviewers. Any product that may be evaluated in this article or claim that may be made by its manufacturer is not guaranteed or endorsed by the publisher.
Research integrity at Frontiers
Learn more about the work of our research integrity team to safeguard the quality of each article we publish.