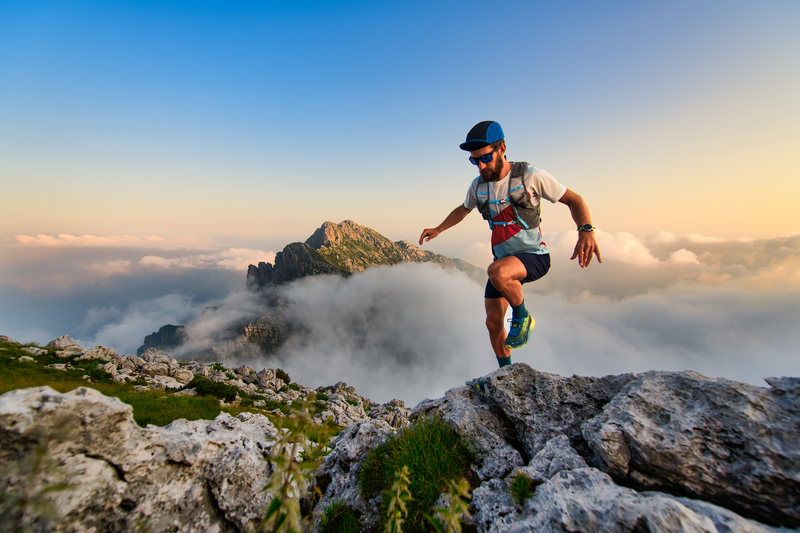
95% of researchers rate our articles as excellent or good
Learn more about the work of our research integrity team to safeguard the quality of each article we publish.
Find out more
SYSTEMATIC REVIEW article
Front. Aging Neurosci. , 26 July 2022
Sec. Neurocognitive Aging and Behavior
Volume 14 - 2022 | https://doi.org/10.3389/fnagi.2022.934844
This article is part of the Research Topic Physical Exercise for Age-Related Neuromusculoskeletal Disorders View all 36 articles
Background: The application of wearable sensor technology in an exercise intervention provides a new method for the standardization and accuracy of intervention. Considering that the deterioration of musculoskeletal conditions is of serious concern in patients with neurodegenerative diseases, it is worthwhile to clarify the effect of wearable sensor-based exercise on musculoskeletal disorders in such patients compared with traditional exercise.
Methods: Five health science-related databases, including PubMed, Cochrane Library, Embase, Web of Science, and Ebsco Cumulative Index to Nursing and Allied Health, were systematically searched. The protocol number of the study is PROSPERO CRD42022319763. Randomized controlled trials (RCTs) that were published up to March 2022 and written in English were included. Balance was the primary outcome measure, comprising questionnaires on postural stability and computerized dynamic posturography. The secondary outcome measures are motor symptoms, mobility ability, functional gait abilities, fall-associated self-efficacy, and adverse events. Stata version 16.0 was used for statistical analysis, and the weighted mean difference (WMD) was selected as the effect size with a 95% confidence interval (CI).
Results: Fifteen RCTs involving 488 participants with mean ages ranging from 58.6 to 81.6 years were included in this review, with 14 of them being pooled in a quantitative meta-analysis. Only five included studies showed a low risk of bias. The Berg balance scale (BBS) was used in nine studies, and the pooled data showed a significant improvement in the wearable sensor-based exercise group compared with the traditional exercise group after 3–12-week intervention (WMD = 1.43; 95% CI, 0.50 to 2.36, P = 0.003). A significant change in visual score was found both post-assessment and at 1-month follow-up assessment (WMD = 4.38; 95% CI, 1.69 to 7.07, P = 0.001; I2 = 0.0%). However, no significant differences were found between the two groups in the secondary outcome measures (all p > 0.05). No major adverse events were reported.
Conclusion: The wearable sensor-based exercise had advantages in improving balance in patients with neurodegenerative diseases, while there was a lack of evidence in motor symptoms, mobility, and functional gait ability enhancement. Future studies are recommended to construct a comprehensive rehabilitation treatment system for the improvement in both postural control and quality of life.
Systematic Review Registration: http://www.crd.york.ac.uk/prospero/, identifier CRD42022319763.
Neurodegenerative diseases (e.g., Alzheimer’s disease, Parkinson’s disease, motor neuron diseases, or amyotrophic lateral sclerosis) are caused by the progressive degeneration of neurons and/or their myelin sheaths and mainly characterized by the deposition of proteins showing altered physicochemical properties in the brain and in peripheral organs (Dugger and Dickson, 2017; Kovacs, 2017), such as degeneration and death of dopaminergic neurons in substantia nigra, or tangle of intracellular neurofibrillary. Symptoms of neurodegenerative diseases vary depending on the mechanism of degeneration and the corresponding brain region (Erkkinen et al., 2018). As degeneration increases, patients may develop different types of dysfunctions, including cognitive or motor impairments (Pender et al., 2020; Aarsland et al., 2021). Moreover, neurodegenerative diseases are among the most serious health problems affecting the life expectancy of millions of people worldwide (Kingwell, 2019; Dommershuijsen et al., 2020), with an estimated incidence rate from approximately 17 per 100,000 to 11.08 per 1000 person-years in Parkinson’s disease (PD) (Hirsch et al., 2016) and Alzheimer’s disease (AD) (Niu et al., 2017), respectively.
Previous study suggested that the main motor symptom, which was associated with neurodegeneration, increases gradually as the disease progresses (Ray and Agarwal, 2020), is the postural control abnormalities because the degeneration of the nervous system influences the integration of sensory information, the formation of motor patterns (which can be understood as the central nervous system generating the imagination of the movement), and the disorder of muscle control (Ryan et al., 2014). A growing body of research demonstrated that patients with neurodegenerative diseases may present with decreased balance, abnormal gait (Morel et al., 2020), a higher tendency to fall (Schell et al., 2019), and frailty (Swanson and Robinson, 2020; Waite et al., 2021), which brings about higher morbidity and mortality and also turns into significant healthcare concerns.
Cass et al. proposed that the ability to control postural balance is essential to perform most of the daily life activities, allowing people to maintain an active lifestyle, and avoiding falls (Cass, 2017). Motor intervention is a critical pathway for improving both balance and postural control in people with neurodegenerative diseases. The traditional exercise protocol consists of repeated balance training and gait relearning under the guidance of the therapist (Ni et al., 2018; Meng et al., 2020; Okada et al., 2021), while wearable systems are a promising solution to provide quantitative and meaningful clinical information about progress in a rehabilitation pathway, with personalized biofeedback or tele-therapy that can be administered in the comfort of settings for those with progressive neurological conditions (Porciuncula et al., 2018). Well-documented evidence suggested that the involvement of wearable sensor-based exercise, which means a cueing rehabilitation based on devices providing visual, auditory, or vibrotactile biofeedback (Carpinella et al., 2017), can provide patients with instant and sensitive biofeedback about the user’s performance and build an interactive environment for supporting motor learning (Argent et al., 2019). The sensors can accurately measure body motion and capture the tendency of incorrect action patterns to promote the learning of postural control (Carpinella et al., 2017; Jakob et al., 2021; Meng et al., 2021). Studies have supported that a wearable sensor-based intervention model is well suited to impact a movement disorder in people with mild cognitive impairment (Schwenk et al., 2016), as they can serve as a “sixth sense,” promote the central organization of multiple sensory inputs through external feedback (Gomez-Pinilla and Hillman, 2013; Gera et al., 2018), guide the formation of movement patterns in the brain (internal feedback) to control posture (Donath et al., 2016; Shih et al., 2016; Conradsson et al., 2017; Silva et al., 2017), and give a motivating effect due to game-based features (Horak et al., 2015).
Overview articles have discussed the potential of wearable sensor-based exercise for improving clinically relevant motor performances, such as postural stability or gait, which are important for safe ambulation and mobility-related quality of life (Horak et al., 2015), and a previous meta-analysis, including eight randomized controlled trials (RCTs), provided evidence for a positive effect of wearable sensor-based exercise on static steady-state balance of healthy and various patient populations in studies with usual care controls and studies with conventional balance training controls (Gordt et al., 2018). However, to our knowledge, it remains unclear whether the wearable sensor-based exercise can effectively improve musculoskeletal disorders in individuals with neurodegenerative diseases. Therefore, the purpose of this study was to clarify the effectiveness of wearable sensor-based exercise for musculoskeletal concerns in patients with neurodegenerative diseases.
The Preferred Reporting Item for Systematic Reviews and Meta-Analyses (PRISMA) guidelines were used to structure our review. PROSPERO was used to enroll the protocol for this meta-analysis (No. CRD42022319763).
Two reviewers (Xin Li and Zhengquan Chen) independently conducted an extensive search in five health science databases, including PubMed, Cochrane Library, Embase, Web of Science, and Ebsco Cumulative Index to Nursing and Allied Health, up to March 2022. The following search terms and their synonyms: “neurodegenerative diseases,” “Parkinson’s disease,” “Alzheimer’s disease,” “motor neuron diseases,” “amyotrophic lateral sclerosis,” “biofeedback,” “sensor,” “exercise,” and “postural control” (Table 1), were used. The search strategies are given in Supplementary Appendix 1.
We also searched the reference lists of the included studies and reviews of similar topics to identify additional eligible studies. This systematic review only included RCTs written in English. If there was a disagreement, the full text of the article was checked and discussed, if necessary, with third-party adjudication (Meiwen Zhu).
The following criteria that followed PICOS strategy were used to determine whether studies were eligible: (1) population: patients who were diagnosed with neurodegenerative diseases, including Parkinson’s disease, Alzheimer’s disease, motor neuron diseases, and amyotrophic lateral sclerosis; (2) intervention: wearable sensor-based exercise, including self-developed sensors or commercial sensors, which can provide visual, auditory, or vibrotactile biofeedback; (3) comparisons: therapeutic exercise without the use of sensors; (4) outcome measures: primary outcome being balance and secondary outcomes being motor symptoms, mobility ability, functional gait abilities, and fall-associated self-efficacy; and (5) study design: RCTs.
Two reviewers (Yiming Yue and Shuangyu Gu) independently screened the studies using the eligibility criteria. The titles and abstracts were initially screened, and then, the full texts of the remaining articles were extensively reviewed. Disagreements were resolved by discussion and rechecking the articles.
Considering the progression of neurodegenerative diseases, changes in the outcomes were compared between the wearable sensor-based exercise and controls. A change in balance was the primary outcome for extraction. Balance was assessed by evaluated by the Berg balance scale (BBS) or sensory organization test (SOT). A change in motor symptoms evaluated by the Unified Parkinson’s Disease Rating Scale-III (UPDRS-III), mobility ability evaluated by the timed “Up and Go” test (TUG), 10-meter walking test (10MWT), and 39-item Parkinson’s Disease Questionnaire (PDQ-39), functional gait abilities evaluated by the dynamic gait index (DGI), and fall-associated self-efficacy evaluated by the activities-specific balance confidence (ABC) scale were also extracted as the secondary outcomes.
Two reviewers (Qing Du and Haibin Guo) were paired up to retrieve the information and data from the included RCTs. Disagreements were resolved by discussion with a third reviewer (Xin Li). The following data were extracted: first author, published year, country, the number, gender, and age of participants; the duration, severity, and medication of the neurodegenerative diseases; sensor type; outcome measures; time points; and dropout rate. The details of the intervention methods (frequency, intensity, time, and type of exercise) in both the control group and sensor-based intervention group were also extracted. Adverse events were also collected to determine the safety of the wearable sensor-based exercise protocols.
Mean, standard deviation (SD), and the sample size were extracted for the outcome measures in each group (i.e., active and sham) for the pooled analysis. Published protocols were referenced and the corresponding authors were contacted for additional data when data were not directly available from the article.
The two reviewers (Xuan Zhou and Jing Tao) independently used the Cochrane risk of bias assessment tool (RoB 2.0) for RCTs to assess the methodological quality of the included studies. RoB 2.0 provides five domains and gives the overall risk of bias evaluation at the end. The five domains in RoB 2.0 are (1) the randomization process, (2) deviations from the intended interventions, (3) missing outcome data, (4) measurement of the outcome, and (5) selection of the reported result. For missing outcome data in individual studies, we stipulated a low risk of bias for loss to follow-up of less than 10% and a difference of less than 5% in missing data between intervention and control groups. Publication bias was assessed through visual inspection of funnel plots for each outcome in which 10 or more eligible studies were identified.
We used Stata version 16.0 (Stata Corp., College Station, TX, United States) to conduct this meta-analysis. The weighted mean difference (WMD) with 95 percent confidence intervals (Cls) was calculated to represent the effect size. The I2 test was used to estimate the heterogeneity. A random-effects model was adopted when there was a significant heterogeneity (I2 > 50%); otherwise, a fixed-effects model was used. Egger’s test was used to identify the publication bias of the main outcome measures. The p-value at the 0.05 level was considered statistically significant. Moreover, a sub-group analysis was performed on outcome measures of different follow-up time points according to the included studies.
The Grading of Recommendations Assessment, Development, and Evaluation (GRADE) approach was conducted in this systematic review and meta-analysis to determine the quality of the evidence provided by RCTs. Detailed GRADE guidance was used to assess the overall risk of bias, imprecision, inconsistency, indirectness, and publication bias and to summarize the results. We categorized each piece of evidence as high, medium, low, or very low quality (Atkins et al., 2004).
We found 1726 relevant articles from five health science databases. After removing duplicates, 1314 article titles and abstracts were screened for relevance. Eventually, 1299 articles were excluded due to not meeting the inclusion criteria or satisfying the exclusion criteria, and 15 articles were included. All these studies were randomized clinical trials. The flowchart of the study selection process is shown in Figure 1.
Figure 1. Flowchart of the process of literature search and extraction of studies meeting the inclusion criteria.
The demographic variables, type of sensors, time point, outcome measures, and dropout rate are given in Table 2. The frequency, intensity, time, and type of the exercise protocols in the intervention group and control group are presented in Table 3. A total of 15 articles involved 488 participants with mean ages ranging from 58.6 to 81.6 years, with 14 of them being pooled in a quantitative meta-analysis. The selected RCTs were performed in patients with PD (n = 13) (Yen et al., 2011; Nanhoe-Mahabier et al., 2012; Pompeu et al., 2012; van den Heuvel et al., 2014; Liao et al., 2015a,b; Yang et al., 2016; Carpinella et al., 2017; Gandolfi et al., 2017; Ribas et al., 2017; Cikajlo and Potisk, 2019; Santos et al., 2019; Kafle and Rizvi, 2021) and AD (n = 2) (Padala et al., 2012; Ugur and Sertel, 2020). Most studies included patients with mild-to-moderate Parkinson’s disease (Hoehn and Yahr stages I to III).
The included trials were carried out in China (n = 4), Brazil (n = 3), Italy (n = 2), Netherlands (n = 2), India (n = 1), Slovenia (n = 1), Turkey (n = 1), and the United States (n = 1) (Table 2). Nine studies used the Nintendo Wii Fit (Yen et al., 2011; Padala et al., 2012; Pompeu et al., 2012; Liao et al., 2015a,b; Gandolfi et al., 2017; Ribas et al., 2017; Ugur and Sertel, 2020; Kafle and Rizvi, 2021) connected to a large screen. Four studies (van den Heuvel et al., 2014; Yang et al., 2016; Cikajlo and Potisk, 2019; Santos et al., 2019) played sports games based on VR technology, one study (Carpinella et al., 2017) used a Gamepad system comprising six wearable inertial sensors, and one study (Nanhoe-Mahabier et al., 2012) conducted a balance training session with a real-time vibrotactile biofeedback system containing angular velocity sensors. One trial reported an immediate effect of one-time biofeedback program on trunk sway of PD (Nanhoe-Mahabier et al., 2012), while the rehabilitation process of other 14 trials was completed over 2 months with a minimum of 10 sessions and a maximum of 40 sessions. Besides, the dedicated time per training session ranged from 20 to 60 min; in three studies (Nanhoe-Mahabier et al., 2012; Santos et al., 2019; Kafle and Rizvi, 2021), the treatment time was not given. Regarding treatment frequency, most studies reported a frequency between two and five times a week, and only one study (Kafle and Rizvi, 2021) provided no information about intervention frequency. Two studies (Yang et al., 2016; Gandolfi et al., 2017) were carried out in the home circumstance, involving 99 participants.
The risk of bias is reported in Supplementary Table 1. Most of the studies (more than 75%) present an unclear risk of bias in deviations from the intended interventions (effect of assignment to intervention). More than 50% included studies presented a low risk of bias in the randomization process, missing outcome data, measurement of the outcome, and selection of the reported result. The quality of evidence appraisal is given in Supplementary Table 2, and the quality ranged from low to moderate.
The Berg balance scale is a comprehensive scale for postural stability assessment. Patients diagnosed with neurodegenerative diseases were assessed for static and dynamic postural control tasks using the BBS (Downs, 2015). The BBS was reported in nine studies (Padala et al., 2012; Pompeu et al., 2012; van den Heuvel et al., 2014; Yang et al., 2016; Carpinella et al., 2017; Gandolfi et al., 2017; Ribas et al., 2017; Santos et al., 2019; Kafle and Rizvi, 2021), with the duration from 3 to 12 weeks. When post-intervention data from nine randomized controlled studies were pooled, a significant improvement was found [WMD = 1.43; 95% CI, 0.50 to 2.36, P = 0.003; I2 = 0.0%, Figure 2(2.1)]. After 0.5–2 months of follow-up, no significant differences were found between the wearable sensor-based exercise and control [≤1-month follow-up, WMD = −0.26; 95% CI, −2.21 to 1.69, P = 0.79; >1-month follow-up, WMD = −0.14; 95% CI, −1.19 to 0.91, P = 0.80, Figures 2(2.2; 2.3)]. Besides, the overall result of this pooled analysis is not significant (WMD = 0.63; 95% CI, −0.03 to 1.28, P = 0.061; I2 = 0.0%, Figure 2). In addition, Egger’s test revealed no evidence of publication bias (P = 0.815).
The SOT is used to quantify sensory integration ability for balance using computerized dynamic posturography. It can provide information related to the integration of vision, somatosensory, and vestibular systems for maintaining postural stability (Gera et al., 2016). Only two studies (Yen et al., 2011; Liao et al., 2015b) from China with 78 participants used the SOT, and the results showed no significant effect of wearable sensor-based exercise on the somatosensory score (WMD = −0.63; 95% CI, −1.62 to 0.37, P = 0.216; I2 = 35.0%) (Figure 3A) or the vestibular score (WMD = −5.43; 95% CI, −24.58 to 13.73, P = 0.579; I2 = 93.3%) (Figure 3C). However, a significant change in visual score was found both post-assessment and at 1-month follow-up assessment (WMD = 4.38; 95% CI, 1.69 to 7.07, P = 0.001; I2 = 0.0%) (Figure 3B).
Figure 3. Forest plot of pooled results for sensory organization test. (A) Somatosensory scores. (B) Visual scores. (C) Vestibular scores.
The UPDRS is the most used comprehensive scale of PD severity. The scale has four sub-scales: I = Motivation, Behavior, and Mood; II = ADL; III = Motor Examination; and IV = Complications of Therapy (Goetz et al., 2008). The pooled results of four studies (van den Heuvel et al., 2014; Yang et al., 2016; Carpinella et al., 2017; Kafle and Rizvi, 2021) did not show a significant effect of wearable sensor-based exercise on the UPDRS-III either at the post-intervention or 0.5–1.5-month follow-up (WMD = −0.00; 95% CI, −2.79 to 2.78, P = 0.997; I2 = 46.9%) (Figure 4).
Five studies (Padala et al., 2012; Liao et al., 2015a; Yang et al., 2016; Carpinella et al., 2017; Santos et al., 2019) used the TUG to evaluate functional mobility. In the TUG, the participants were timed as they stood up from a backrest chair, walked with a normal gait 3 meters forward, and then sat down and leaned back (de Oliveira Silva et al., 2019; Yoo et al., 2020). The pooled analysis showed no significant effect of wearable sensor-based exercise on the TUG test (WMD = −1.06; 95% CI, −2.17 to 0.06, P = 0.589; I2 = 0%) (Figure 5). Three studies (van den Heuvel et al., 2014; Carpinella et al., 2017; Gandolfi et al., 2017) measured the effects on functional mobility by the 10MWT. Our analysis revealed no significant effect of wearable sensor-based exercise on the 10MWT (WMD = 0.01; 95% CI, −0.07 to 0.08, P = 0.481; I2 = 0%) (Figure 6).
Parkinson’s disease questionnaire-39 is a self-reported quality-of-life questionnaire evaluating mobility and social functions in people with Parkinson’s disease based on a five-point Likert scale (Neff et al., 2018). The PDQ-39 was reported in four studies (Liao et al., 2015a; Yang et al., 2016; Carpinella et al., 2017; Santos et al., 2019), and the pooled analysis showed no significant effect of wearable sensor-based exercise on PDQ-39 scores (WMD = 0.21; 95% CI, −4.24 to 4.66, P = 0.926; I2 = 0%, Figure 7A). The mobility scores of the PDQ-39 were reported in two studies (van den Heuvel et al., 2014; Ribas et al., 2017), and the pooled analysis did reveal no significant effect of wearable sensor-based exercise on these scores (WMD = −0.87; 95% CI, −6.43 to 4.70, P = 0.760; I2 = 0%, Figure 7B; Peto et al., 2001).
Figure 7. Forest plot of pooled results for (A) the total score of 39-item Parkinson’s disease questionnaire and (B) the mobility scores of the 39-item Parkinson’s disease questionnaire.
Dynamic gait index (DGI) has emerged as a valid indicator of functional gait abilities for people with balance and vestibular disorders. Scores on the DGI range from 0 to 24, with higher scores indicating better performance (Bloem et al., 2016). Three RCTs (Yang et al., 2016; Gandolfi et al., 2017; Santos et al., 2019) evaluated the effect of wearable sensor-based exercise on gait performance using the DGI. However, the pooled results did not reveal a significant effect (WMD = −0.55; 95% CI, −1.27 to 0.17, P = 0.135; I2 = 0%) (Figure 8).
Activities-specific balance confidence (ABC) scale measures balance confidence in particular postural control tasks. The ABC scale was employed in two studies (Carpinella et al., 2017; Gandolfi et al., 2017), and there was no significant difference between the wearable sensor-based exercise group and the control group (WMD = 0.40; 95% CI, −4.73 to 5.53, P = 0.879; I2 = 0%, Figure 9).
None of the included RCTs reported any adverse events, such as falling, during study duration. The dropout rate ranged from 0 to 23.5% (Tables 2, 3). One study evaluated the satisfaction of the subject with the wearable sensors, found most of the participants were positive about the device, and considered this a beneficial, reliable, easy to use, comfort, and safe technology (Carpinella et al., 2017).
This systematic review explored the effect of wearable sensor-based exercise on improving postural control in patients with neurodegenerative diseases compared with traditional exercise. The results of the meta-analysis including 14 studies indicated that the wearable sensor-based exercise could induce a significant short-term effect on balance, especially for an increased visual gain in the SOT. However, the 3–12-week wearable sensor-based exercise did not seem to improve mobility ability, functional gait abilities, and fall-associated self-efficacy, as the changes did not reach statistical significance. Overall, the wearable sensor-based exercise increases balance in patients with neurodegenerative diseases, but the evidence is lacking to improve postural control during daily activities, such as walking.
The movement disorders associated with neurodegeneration may be explained by various perspectives, such as oxidative stress or mitochondrial dysfunction (Borsche et al., 2021; Dionísio et al., 2021). Due to the complexity of neurodegeneration with many potential intervention targets, the research progress of drug treatment is slow, and the current drug treatment mainly focuses on improving motor symptoms (Armstrong and Okun, 2020; Dar et al., 2020). In contrast, an exercise intervention is a more direct protocol to improve motor symptoms, and the benefits of intervention can also delay the speed of neurodegeneration and improve the quality of life (Bonavita, 2020; Sujkowski et al., 2022). However, traditional exercise interventions often require the involvement of specialists, such as rehabilitation physicians and physical therapists. The quality of the intervention often depends on the experience of the specialists and the patient’s compliance (Botros et al., 2019; Schootemeijer et al., 2020). The involvement of sensor-based equipment brings new possibilities for an exercise intervention (Jahn et al., 2019). The instant biofeedback provided by sensors and the combination with exergame can form a standardized and interesting exercise protocol for patients with neurodegenerative movement disorders (Ribas et al., 2017; Ugur and Sertel, 2020; Kafle and Rizvi, 2021), such as avoiding obstacles or picking fruit.
The wearable sensors are directly attached to the patients to provide immediate and precise biofeedback on the patient’s movements (Albán-Cadena et al., 2021). Optical sensors often require a bright environment, and reflective clothing will affect the accuracy of their motion capture (Warmerdam et al., 2020). Optical sensors also need a wide living room or hospital treatment room to maximize the exercise experience, which may prevent some patients from using optical sensors. Compared with the use of optical sensors, such as cameras, the application environment of wearable sensors is rarely limited. In addition, patients can be trained in a home environment under the remote guidance of professionals with the emergence of commercialized sensor devices (Garcia-Agundez et al., 2019; Milosevic et al., 2020). It can be used as a feasible solution for an exercise intervention under epidemic conditions.
Although the neurodegenerative disease is progressive and the gray matter volume changes in the left inferior parietal cortex, middle temporal gyrus and right anterior precuneus were associated with the balance capacities (Sehm et al., 2014), a significant improvement in balance was found in the wearable sensor-based exercise group at the end of the intervention, which may be due to the real-time feedback and wrong action corrections in the intensive body control training in the protocol. This systematic review showed that most movement modalities in the interventional group used a center of gravity shifting training method, requiring patients to complete the directional and quantitative shifting of the center of gravity (Liao et al., 2015a,b; Yang et al., 2016; Santos et al., 2019). The inertial sensor placed on the waist can directly reflect the change in the center of gravity, while the pressure sensor placed on the sole can indirectly reflect the change in the center of gravity through the offset of the plantar pressure, thereby providing accurate and immediate biofeedback for the patient (Padala et al., 2012; Pompeu et al., 2012; van den Heuvel et al., 2014; Liao et al., 2015a,b; Carpinella et al., 2017; Ribas et al., 2017).
Otherwise, two studies in this systematic review used the SOT and demonstrated a significantly increased score in visual domain, which might owe to the contribution of visual input significantly increased during the wearable sensor-based exercise, compared with the traditional exercises (Yen et al., 2011; Liao et al., 2015b). The increased visual gain in the SOT conditions also reflects the importance of visual input for the control of balance. As balance perturbations tend to occur in dynamic tasks and in response to environmental constraints not present during the SOT, the SOT may provide additional information for clinical evaluation on neurodegenerative disease and deficient sensory processing (Chien et al., 2014).
Previous meta-analysis and systematic reviews concluded promising short- and long-term benefits of exercise on various meaningful outcomes, such as balance, gait, muscle strength, motor, and functional performance (Tomlinson et al., 2012; Shen et al., 2016; Mak et al., 2017). However, compared with the traditional exercise programs, the results for the secondary outcomes showed no significance in the mobility ability, functional gait abilities, and fall-associated self-efficacy in the wearable sensor-based exercise group. Impaired flexibility and muscle weakness are two common problems that may affect postural control and mobility. As compared to neurologically normal adults, people with neurodegenerative disease had a reduced range of trunk motion, which could partially be explained by axial muscle rigidity (Schenkman et al., 2001), with a reduction in muscle strength by 30–50% (Inkster et al., 2003).
Furthermore, limited by the technical conditions of the sensor as a piece of external equipment, the training protocol in the included studies mainly consisted of the control tasks with a small range of body sway. There was a lack of activities of daily living training that simulate daily scenes, such as walking (Carpinella et al., 2017; Cikajlo and Potisk, 2019). In this meta-analysis, the intervention duration was 3–12 weeks, and the negative results may be associated with overall short treatment durations with a relatively low frequency (an average of 6–7 weeks with two–three times a week). These treatment durations would be considered short relative to multimodal physical therapy for Parkinson’s disease (Tomlinson et al., 2012). On the other hand, although these measurements are the most frequently assessed in clinical practices, they might not have been sensitive enough to detect specific wearable sensor-based exercise training-related changes in dynamic postural control. Moreover, we did not find evidence that factors, such as control group (i.e., usual care vs. conventional training), sensor type, and type of training paradigm, were related to these negative findings, and the limited number of RCTs did not allow to draw definitive conclusions.
People with neurodegenerative diseases are nearly two times as likely to experience a fall as a healthy older person, often leading to debilitating effects on confidence, activity levels, and quality of life (Ashburn et al., 2019). A meta-analysis showed that performance confidence in overcoming barriers to exercise was best addressed with longer-term strategies that provided time for people to experience successfully conquering such barriers over a longer period (Higgins et al., 2014). With regard to the fall-associated self-efficacy in patients with neurodegenerative diseases, the results of our systematic review also showed that the 3–12-week wearable sensor-based exercise cannot improve fall-associated self-efficacy significantly, which might be due to the relatively short intervention duration. Moreover, people with neurodegenerative diseases may suffer from anxiety and depression, which could negatively impact self-efficacy (Stevens et al., 2020), and also, the community and societal factors may account for an important proportion of the improvement in self-efficacy (Bellou et al., 2016; Lee et al., 2016; Rosa Silva et al., 2020). Multidisciplinary teams, such as exercise specialists, occupational therapists, and psychologists, should take cognitive and psychological symptoms into account when working with these patients to maximize the potential effectiveness of treatment, and social workers may be needed to help patients with neurodegenerative diseases reintegrate into the community and society (Stożek et al., 2016; Homayoun, 2018; Ritter and Bonsaksen, 2019). Future research is recommended to construct a comprehensive intervention system for fall-associated self-efficacy.
The strength of this meta-analysis is that only RCTs were included. Furthermore, to the best of our knowledge, this is the first meta-analysis to focus on postural control ability in patients with neurodegenerative diseases compared with traditional therapeutic exercise. However, several limitations need to be highlighted in this systematic review. First, as an indicator of postural stability, the BBS has been significantly improved, but whether the degree of postural stability improvement can lead to effective clinical improvement in movement disorders is unclear. Second, due to the insufficient number of included studies, no significant improvement was found in indicators related to social life functions, such as activities of daily living and quality of life. Finally, the quality of the evidence in this article ranged from moderate to very low (Supplementary Table S2). Therefore, caution should be applied, however, to avoid overestimation of findings given the several methodological weaknesses in available studies, such as short follow-up (<12 months), small sample size (n < 100), and missed evaluation of facilitator.
Compared with traditional exercise interventions, the wearable sensor-based exercise can significantly improve balance in patients with neurodegenerative diseases. However, there was still a lack of evidence showing the superiority of wearable sensor-based exercise technology for other indicators of dynamic postural control, such as motor symptoms, mobility ability, functional gait abilities, and fall-associated self-efficacy. In future, it is necessary to conduct more research on the effect of wearable sensor-based exercise on sensory organization and try to build a comprehensive rehabilitation treatment system to improve both postural control and quality of life.
All raw data included in this study are available upon request by contact with the corresponding author.
XL and QD had the original idea. XL, ZC, and MZ performed the literature search. QD and HG screened the studies using the eligibility criteria and undertook the data collection. XZ and JT assessed the risk of bias. XL, YY, and SG analyzed, interpreted, and discussed the results. All authors contributed to the article and approved the submitted version.
The authors disclose receipt of the following financial support for the research, authorship, and/or publication of this article. This work was supported by the Advanced and Appropriate Technology Promotion Projects of Shanghai Municipal Health Commission (2019SY021), Action Plan for Sustainable Development of Science and Technology Innovation in Chongming District, Shanghai (CKY2021-50), and Chongming District Medical Key Specialty Project.
The authors thank Juping Liang for providing support and advice.
The authors declare that the research was conducted in the absence of any commercial or financial relationships that could be construed as a potential conflict of interest.
All claims expressed in this article are solely those of the authors and do not necessarily represent those of their affiliated organizations, or those of the publisher, the editors and the reviewers. Any product that may be evaluated in this article, or claim that may be made by its manufacturer, is not guaranteed or endorsed by the publisher.
The Supplementary Material for this article can be found online at: https://www.frontiersin.org/articles/10.3389/fnagi.2022.934844/full#supplementary-material
Aarsland, D., Batzu, L., Halliday, G. M., Geurtsen, G. J., Ballard, C., Ray Chaudhuri, K., et al. (2021). Parkinson disease-associated cognitive impairment. Nat. Rev. Dis. Primers 7:47. doi: 10.1038/s41572-021-00280-3
Albán-Cadena, A. C., Villalba-Meneses, F., Pila-Varela, K. O., Moreno-Calvo, A., Villalba-Meneses, C. P., and Almeida-Galárraga, D. A. (2021). Wearable sensors in the diagnosis and study of Parkinson’s disease symptoms: a systematic review. J. Med. Eng. Technol. 45, 532–545. doi: 10.1080/03091902.2021.1922528
Argent, R., Slevin, P., Bevilacqua, A., Neligan, M., Daly, A., and Caulfield, B. (2019). Wearable sensor-based exercise biofeedback for orthopaedic rehabilitation: a mixed methods user evaluation of a prototype system. Sensors 19:432. doi: 10.3390/s19020432
Armstrong, M. J., and Okun, M. S. (2020). Diagnosis and treatment of Parkinson disease: a review. JAMA 323, 548–560. doi: 10.1001/jama.2019.22360
Ashburn, A., Pickering, R., McIntosh, E., Hulbert, S., Rochester, L., Roberts, H. C., et al. (2019). Exercise- and strategy-based physiotherapy-delivered intervention for preventing repeat falls in people with Parkinson’s: the PDSAFE RCT. Health Technol. Assess. 23, 1–150. doi: 10.3310/hta23360
Atkins, D., Best, D., Briss, P. A., Eccles, M., Falck-Ytter, Y., Flottorp, S., et al. (2004). Grading quality of evidence and strength of recommendations. BMJ 328:1490. doi: 10.1136/bmj.328.7454.1490
Bellou, V., Belbasis, L., Tzoulaki, I., Evangelou, E., and Ioannidis, J. P. (2016). Environmental risk factors and Parkinson’s disease: an umbrella review of meta-analyses. Parkinsonism Relat. Disord. 23, 1–9. doi: 10.1016/j.parkreldis.2015.12.008
Bloem, B. R., Marinus, J., Almeida, Q., Dibble, L., Nieuwboer, A., Post, B., et al. (2016). Measurement instruments to assess posture, gait, and balance in Parkinson’s disease: critique and recommendations. Mov. Disord. 31, 1342–1355. doi: 10.1002/mds.26572
Bonavita, S. (2020). Exercise and Parkinson’s Disease. Adv. Exp. Med. Biol. 1228, 289–301. doi: 10.1007/978-981-15-1792-1_19
Borsche, M., Pereira, S. L., Klein, C., and Grünewald, A. (2021). Mitochondria and Parkinson’s disease: clinical, molecular, and translational aspects. J. Parkinsons Dis. 11, 45–60. doi: 10.3233/jpd-201981
Botros, A., Schütz, N., Camenzind, M., Urwyler, P., Bolliger, D., Vanbellingen, T., et al. (2019). Long-term home-monitoring sensor technology in patients with Parkinson’s disease-acceptance and adherence. Sensors 19:5169. doi: 10.3390/s19235169
Carpinella, I., Cattaneo, D., Bonora, G., Bowman, T., Martina, L., Montesano, A., et al. (2017). Wearable sensor-based biofeedback training for balance and gait in Parkinson disease: a pilot randomized controlled trial. Arch. Phys. Med. Rehabil. 98, 622–630.e3. doi: 10.1016/j.apmr.2016.11.003
Cass, S. P. (2017). Alzheimer’s disease and exercise: a literature review. Curr. Sports Med. Rep. 16, 19–22. doi: 10.1249/jsr.0000000000000332
Chien, J. H., Eikema, D. J., Mukherjee, M., and Stergiou, N. (2014). Locomotor sensory organization test: a novel paradigm for the assessment of sensory contributions in gait. Ann. Biomed. Eng. 42, 2512–2523. doi: 10.1007/s10439-014-1112-7
Cikajlo, I., and Potisk, K. P. (2019). Advantages of using 3D virtual reality based training in persons with Parkinson’s disease: a parallel study. J. Neuroeng. Rehabil. 16:14. doi: 10.1186/s12984-019-0601-1
Conradsson, D., Nero, H., Löfgren, N., Hagströmer, M., and Franzén, E. (2017). Monitoring training activity during gait-related balance exercise in individuals with Parkinson’s disease: a proof-of-concept-study. BMC Neurol. 17:19. doi: 10.1186/s12883-017-0804-7
Dar, K. B., Bhat, A. H., Amin, S., Reshi, B. A., Zargar, M. A., Masood, A., et al. (2020). Elucidating critical proteinopathic mechanisms and potential drug targets in neurodegeneration. Cell Mol. Neurobiol. 40, 313–345. doi: 10.1007/s10571-019-00741-0
de Oliveira Silva, F., Ferreira, J. V., Plácido, J., Chagas, D., Praxedes, J., Guimarães, C., et al. (2019). Stages of mild cognitive impairment and Alzheimer’s disease can be differentiated by declines in timed up and go test: a systematic review and meta-analysis. Arch. Gerontol. Geriatr. 85:103941. doi: 10.1016/j.archger.2019.103941
Dionísio, P. A., Amaral, J. D., and Rodrigues, C. M. P. (2021). Oxidative stress and regulated cell death in Parkinson’s disease. Ageing Res. Rev. 67:101263. doi: 10.1016/j.arr.2021.101263
Dommershuijsen, L. J., Heshmatollah, A., Darweesh, S. K., Koudstaal, P. J., Ikram, M. A., and Ikram, M. K. (2020). Life expectancy of parkinsonism patients in the general population. Parkinsonism Relat. Disord. 77, 94–99.
Donath, L., Rössler, R., and Faude, O. (2016). Effects of virtual reality training (exergaming) compared to alternative exercise training and passive control on standing balance and functional mobility in healthy community-dwelling seniors: a meta-analytical review. Sports Med. 46, 1293–1309. doi: 10.1007/s40279-016-0485-1
Dugger, B. N., and Dickson, D. W. (2017). Pathology of neurodegenerative diseases. Cold Spring Harb. Perspect. Biol. 9:a028035.
Erkkinen, M. G., Kim, M.-O., and Geschwind, M. D. (2018). Clinical neurology and epidemiology of the major neurodegenerative diseases. Cold Spring Harb. Perspect. Biol. 10:a033118.
Gandolfi, M., Geroin, C., Dimitrova, E., Boldrini, P., Waldner, A., Bonadiman, S., et al. (2017). Virtual reality telerehabilitation for postural instability in Parkinson’s disease: a multicenter, single-blind, randomized, controlled trial. Biomed. Res. Int. 2017:7962826. doi: 10.1155/2017/7962826
Garcia-Agundez, A., Folkerts, A. K., Konrad, R., Caserman, P., Tregel, T., Goosses, M., et al. (2019). Recent advances in rehabilitation for Parkinson’s disease with exergames: a systematic review. J. Neuroeng. Rehabil. 16:17. doi: 10.1186/s12984-019-0492-1
Gera, G., Chesnutt, J., Mancini, M., Horak, F. B., and King, L. A. (2018). Inertial sensor-based assessment of central sensory integration for balance after mild traumatic brain injury. Mil. Med. 183(Suppl._1), 327–332. doi: 10.1093/milmed/usx162
Gera, G., Freeman, D. L., Blackinton, M. T., Horak, F. B., and King, L. (2016). Identification of balance deficits in people with Parkinson disease; is the sensory organization test enough? Int. J. Phys. Med. Rehabil. 4:322. doi: 10.4172/2329-9096.1000322
Goetz, C. G., Tilley, B. C., Shaftman, S. R., Stebbins, G. T., Fahn, S., Martinez-Martin, P., et al. (2008). Movement Disorder Society-sponsored revision of the Unified Parkinson’s Disease Rating Scale (MDS-UPDRS): scale presentation and clinimetric testing results. Mov. Disord. 23, 2129–2170. doi: 10.1002/mds.22340
Gomez-Pinilla, F., and Hillman, C. (2013). The influence of exercise on cognitive abilities. Comprehens. Physiol. 3:403.
Gordt, K., Gerhardy, T., Najafi, B., and Schwenk, M. (2018). Effects of wearable sensor-based balance and gait training on balance, gait, and functional performance in healthy and patient populations: a systematic review and meta-analysis of randomized controlled trials. Gerontology 64, 74–89. doi: 10.1159/000481454
Higgins, T. J., Middleton, K. R., Winner, L., and Janelle, C. M. (2014). Physical activity interventions differentially affect exercise task and barrier self-efficacy: a meta-analysis. Health Psychol. 33, 891–903. doi: 10.1037/a0033864
Hirsch, L., Jette, N., Frolkis, A., Steeves, T., and Pringsheim, T. (2016). The incidence of Parkinson’s disease: a systematic review and meta-analysis. Neuroepidemiology 46, 292–300. doi: 10.1159/000445751
Homayoun, H. (2018). Parkinson disease. Ann. Intern. Med. 169, Itc33–Itc48. doi: 10.7326/aitc201809040
Horak, F., King, L., and Mancini, M. (2015). Role of body-worn movement monitor technology for balance and gait rehabilitation. Phys. Ther. 95, 461–470. doi: 10.2522/ptj.20140253
Inkster, L. M., Eng, J. J., MacIntyre, D. L., and Stoessl, A. J. (2003). Leg muscle strength is reduced in Parkinson’s disease and relates to the ability to rise from a chair. Mov. Disord. 18, 157–162. doi: 10.1002/mds.10299
Jahn, K., Freiberger, E., Eskofier, B. M., Bollheimer, C., and Klucken, J. (2019). Balance and mobility in geriatric patients : assessment and treatment of neurological aspects. Z. Gerontol. Geriatr. 52, 316–323. doi: 10.1007/s00391-019-01561-z
Jakob, V., Küderle, A., Kluge, F., Klucken, J., Eskofier, B. M., Winkler, J., et al. (2021). Validation of a sensor-based gait analysis system with a gold-standard motion capture system in patients with Parkinson’s disease. Sensors 21:7680. doi: 10.3390/s21227680
Kafle, A., and Rizvi, S. R. (2021). Effect of wii-based motor and cognitive training on activities of daily living in patients with Parkinson’s disease. Indian J. Physiother. Occupat. Ther. 15, 1–7. doi: 10.37506/ijpot.v15i3.16157
Kingwell, K. (2019). An exercise-linked mediator of memory protection. Nat. Rev. Drug Discov. 18, 97–98.
Kovacs, G. G. (2017). Concepts and classification of neurodegenerative diseases. Handb. Clin. Neurol. 145, 301–307. doi: 10.1016/b978-0-12-802395-2.00021-3
Lee, H. K., Altmann, L. J., McFarland, N., and Hass, C. J. (2016). The relationship between balance confidence and control in individuals with Parkinson’s disease. Parkinsonism Relat. Disord. 26, 24–28. doi: 10.1016/j.parkreldis.2016.02.015
Liao, Y.-Y., Yang, Y.-R., Cheng, S.-J., Wu, Y.-R., Fuh, J.-L., and Wang, R.-Y. (2015a). Virtual reality–based training to improve obstacle-crossing performance and dynamic balance in patients with Parkinson’s disease. Neurorehabil. Neural Repair 29, 658–667. doi: 10.1177/1545968314562111
Liao, Y. Y., Yang, Y. R., Wu, Y. R., and Wang, R. Y. (2015b). Virtual reality-based wii fit training in improving muscle strength, sensory integration ability, and walking abilities in patients with Parkinson’s disease: a randomized control trial. Int. J. Gerontol. 9, 190–195. doi: 10.1016/j.ijge.2014.06.007
Mak, M. K., Wong-Yu, I. S., Shen, X., and Chung, C. L. (2017). Long-term effects of exercise and physical therapy in people with Parkinson disease. Nat. Rev. Neurol. 13, 689–703. doi: 10.1038/nrneurol.2017.128
Meng, L., Huang, X., Yang, Y., Pang, J., Chen, L., and Ming, D. (2021). An inertial sensor-based algorithm for turning detection during gait. Annu. Int. Conf. IEEE Eng. Med. Biol. Soc. 2021, 6372–6375. doi: 10.1109/embc46164.2021.9630374
Meng, L., Li, X., Li, C., Tsang, R. C. C., Chen, Y., Ge, Y., et al. (2020). Effects of exercise in patients with amyotrophic lateral sclerosis: a systematic review and meta-analysis. Am. J. Phys. Med. Rehabil. 99, 801–810. doi: 10.1097/phm.0000000000001419
Milosevic, B., Leardini, A., and Farella, E. (2020). Kinect and wearable inertial sensors for motor rehabilitation programs at home: state of the art and an experimental comparison. Biomed. Eng. Online 19:25. doi: 10.1186/s12938-020-00762-7
Morel, E., Armand, S., Assal, F., and Allali, G. (2020). Parkinsonian gait in aging: a feature of Alzheimer’s pathology? Exp. Gerontol. 134:110905. doi: 10.1016/j.exger.2020.110905
Nanhoe-Mahabier, W., Allum, J. H., Pasman, E. P., Overeem, S., and Bloem, B. R. (2012). The effects of vibrotactile biofeedback training on trunk sway in Parkinson’s disease patients. Parkinsonism Relat. Disord. 18, 1017–1021. doi: 10.1016/j.parkreldis.2012.05.018
Neff, C., Wang, M. C., and Martel, H. (2018). Using the PDQ-39 in routine care for Parkinson’s disease. Parkinsonism Relat. Disord. 53, 105–107. doi: 10.1016/j.parkreldis.2018.05.019
Ni, M., Hazzard, J. B., Signorile, J. F., and Luca, C. (2018). Exercise guidelines for gait function in Parkinson’s disease: a systematic review and meta-analysis. Neurorehabil. Neural Repair 32, 872–886. doi: 10.1177/1545968318801558
Niu, H., Álvarez-Álvarez, I., Guillén-Grima, F., and Aguinaga-Ontoso, I. (2017). Prevalence and incidence of Alzheimer’s disease in Europe: a meta-analysis. Neurologia 32, 523–532. doi: 10.1016/j.nrl.2016.02.016
Okada, Y., Ohtsuka, H., Kamata, N., Yamamoto, S., Sawada, M., Nakamura, J., et al. (2021). Effectiveness of long-term physiotherapy in Parkinson’s disease: a systematic review and meta-analysis. J. Parkinsons Dis. 11, 1619–1630. doi: 10.3233/jpd-212782
Padala, K. P., Padala, P. R., Malloy, T. R., Geske, J. A., Dubbert, P. M., Dennis, R. A., et al. (2012). Wii-Fit for improving gait and balance in an assisted living facility: a pilot study. J. Aging Res. 2012:597573. doi: 10.1155/2012/597573
Pender, N., Pinto-Grau, M., and Hardiman, O. (2020). Cognitive and behavioural impairment in amyotrophic lateral sclerosis. Curr. Opin. Neurol. 33, 649–654.
Peto, V., Jenkinson, C., and Fitzpatrick, R. (2001). Determining minimally important differences for the PDQ-39 Parkinson’s disease questionnaire. Age Ageing 30, 299–302. doi: 10.1093/ageing/30.4.299
Pompeu, J. E., Mendes, F. A., Silva, K. G., Lobo, A. M., Oliveira Tde, P., Zomignani, A. P., et al. (2012). Effect of Nintendo Wii™-based motor and cognitive training on activities of daily living in patients with Parkinson’s disease: a randomised clinical trial. Physiotherapy 98, 196–204. doi: 10.1016/j.physio.2012.06.004
Porciuncula, F., Roto, A. V., Kumar, D., Davis, I., Roy, S., Walsh, C. J., et al. (2018). Wearable movement sensors for rehabilitation: a focused review of technological and clinical advances. PM R 10(9 Suppl. 2), S220–S232. doi: 10.1016/j.pmrj.2018.06.013
Ray, S., and Agarwal, P. (2020). Depression and anxiety in Parkinson disease. Clin. Geriatr. Med. 36, 93–104. doi: 10.1016/j.cger.2019.09.012
Ribas, C. G., da Silva, L. A., Correa, M. R., Teive, H. G., and Valderramas, S. (2017). Effectiveness of exergaming in improving functional balance, fatigue and quality of life in Parkinson’s disease: a pilot randomized controlled trial. Parkinsonism Relat. Disord. 38, 13–18. doi: 10.1016/j.parkreldis.2017.02.006
Ritter, V. C., and Bonsaksen, T. (2019). Improvement in quality of life following a multidisciplinary rehabilitation program for patients with Parkinson’s disease. J. Multidiscip. Healthc. 12, 219–227. doi: 10.2147/jmdh.S202827
Rosa Silva, J. P., Santiago Júnior, J. B., Dos Santos, E. L., de Carvalho, F. O., de França Costa, I. M. P., and Mendonça, D. M. F. (2020). Quality of life and functional independence in amyotrophic lateral sclerosis: a systematic review. Neurosci. Biobehav. Rev. 111, 1–11. doi: 10.1016/j.neubiorev.2019.12.032
Ryan, N. S., Shakespeare, T. J., Lehmann, M., Keihaninejad, S., Nicholas, J. M., Leung, K. K., et al. (2014). Motor features in posterior cortical atrophy and their imaging correlates. Neurobiol. Aging 35, 2845–2857.
Santos, P., Machado, T., Santos, L., Ribeiro, N., and Melo, A. (2019). Efficacy of the nintendo wii combination with conventional exercises in the rehabilitation of individuals with Parkinson’s disease: a randomized clinical trial. Neurorehabilitation 45, 255–263. doi: 10.3233/nre-192771
Schell, W. E., Mar, V. S., and Da Silva, C. P. (2019). Correlation of falls in patients with amyotrophic lateral sclerosis with objective measures of balance, strength, and spasticity. Neurorehabilitation 44, 85–93. doi: 10.3233/nre-182531
Schenkman, M. L., Clark, K., Xie, T., Kuchibhatla, M., Shinberg, M., and Ray, L. (2001). Spinal movement and performance of a standing reach task in participants with and without Parkinson disease. Phys. Ther. 81, 1400–1411. doi: 10.1093/ptj/81.8.1400
Schootemeijer, S., van der Kolk, N. M., Ellis, T., Mirelman, A., Nieuwboer, A., Nieuwhof, F., et al. (2020). Barriers and motivators to engage in exercise for persons with Parkinson’s disease. J. Parkinsons Dis. 10, 1293–1299. doi: 10.3233/jpd-202247
Schwenk, M., Sabbagh, M., Lin, I., Morgan, P., Grewal, G. S., Mohler, J., et al. (2016). Sensor-based balance training with motion feedback in people with mild cognitive impairment. J. Rehabil. Res. Dev. 53, 945–958. doi: 10.1682/jrrd.2015.05.0089
Sehm, B., Taubert, M., Conde, V., Weise, D., Classen, J., Dukart, J., et al. (2014). Structural brain plasticity in Parkinson’s disease induced by balance training. Neurobiol. Aging 35, 232–239. doi: 10.1016/j.neurobiolaging.2013.06.021
Shen, X., Wong-Yu, I. S., and Mak, M. K. (2016). Effects of exercise on falls, balance, and gait ability in Parkinson’s disease: a meta-analysis. Neurorehabil. Neural Repair 30, 512–527. doi: 10.1177/1545968315613447
Shih, M.-C., Wang, R.-Y., Cheng, S.-J., and Yang, Y.-R. (2016). Effects of a balance-based exergaming intervention using the Kinect sensor on posture stability in individuals with Parkinson’s disease: a single-blinded randomized controlled trial. J. Neuroeng. Rehabil. 13:78. doi: 10.1186/s12984-016-0185-y
Silva, K. G., De Freitas, T. B., Doná, F., Ganança, F. F., Ferraz, H. B., Torriani-Pasin, C., et al. (2017). Effects of virtual rehabilitation versus conventional physical therapy on postural control, gait, and cognition of patients with Parkinson’s disease: study protocol for a randomized controlled feasibility trial. Pilot Feasibility Stud. 3:68. doi: 10.1186/s40814-017-0210-3
Stevens, A., Stanton, R., and Rebar, A. L. (2020). Helping people with Parkinson disease build exercise self-efficacy. Phys. Ther. 100, 205–208. doi: 10.1093/ptj/pzz160
Stożek, J., Rudzińska, M., Pustułka-Piwnik, U., and Szczudlik, A. (2016). The effect of the rehabilitation program on balance, gait, physical performance and trunk rotation in Parkinson’s disease. Aging Clin. Exp. Res. 28, 1169–1177. doi: 10.1007/s40520-015-0506-1
Sujkowski, A., Hong, L., Wessells, R. J., and Todi, S. V. (2022). The protective role of exercise against age-related neurodegeneration. Ageing Res. Rev. 74, 101543. doi: 10.1016/j.arr.2021.101543
Swanson, R., and Robinson, K. M. (2020). Geriatric rehabilitation: gait in the elderly, fall prevention and Parkinson disease. Med. Clin. North Am. 104, 327–343. doi: 10.1016/j.mcna.2019.10.012
Tomlinson, C. L., Patel, S., Meek, C., Herd, C. P., Clarke, C. E., Stowe, R., et al. (2012). Physiotherapy intervention in Parkinson’s disease: systematic review and meta-analysis. BMJ 345:e5004. doi: 10.1136/bmj.e5004
Ugur, F., and Sertel, M. (2020). The effect of virtual reality applications on balance and gait speed in individuals with alzheimer dementia: a pilot study. Topics Geriatr. Rehabil. 36, 221–229. doi: 10.1097/TGR.0000000000000285
van den Heuvel, M. R. C., Kwakkel, G., Beek, P. J., Berendse, H. W., Daffertshofer, A., and van Wegen, E. E. H. (2014). Effects of augmented visual feedback during balance training in Parkinson’s disease: a pilot randomized clinical trial. Parkinsonism Relat. Disord. 20, 1352–1358. doi: 10.1016/j.parkreldis.2014.09.022
Waite, S. J., Maitland, S., Thomas, A., and Yarnall, A. J. (2021). Sarcopenia and frailty in individuals with dementia: a systematic review. Arch. Gerontol. Geriatr. 92:104268. doi: 10.1016/j.archger.2020.104268
Warmerdam, E., Romijnders, R., Welzel, J., Hansen, C., Schmidt, G., and Maetzler, W. (2020). Quantification of arm swing during walking in healthy adults and Parkinson’s disease patients: wearable sensor-based algorithm development and validation. Sensors 20:5963. doi: 10.3390/s20205963
Yang, W. C., Wang, H. K., Wu, R. M., Lo, C. S., and Lin, K. H. (2016). Home-based virtual reality balance training and conventional balance training in Parkinson’s disease: a randomized controlled trial. J. Formos. Med. Assoc. 115, 734–743. doi: 10.1016/j.jfma.2015.07.012
Yen, C. Y., Lin, K. H., Hu, M. H., Wu, R. M., Lu, T. W., and Lin, C. H. (2011). Effects of virtual reality-augmented balance training on sensory organization and attentional demand for postural control in people with Parkinson disease: a randomized controlled trial. Phys. Ther. 91, 862–874. doi: 10.2522/ptj.20100050
Keywords: neurodegenerative diseases, Parkinson’s disease, Alzheimer’s disease, wearable sensor-based exercise, musculoskeletal disorders, balance, dynamic postural control
Citation: Li X, Chen Z, Yue Y, Zhou X, Gu S, Tao J, Guo H, Zhu M and Du Q (2022) Effect of Wearable Sensor-Based Exercise on Musculoskeletal Disorders in Individuals With Neurodegenerative Diseases: A Systematic Review and Meta-Analysis. Front. Aging Neurosci. 14:934844. doi: 10.3389/fnagi.2022.934844
Received: 03 May 2022; Accepted: 23 June 2022;
Published: 26 July 2022.
Edited by:
Howe Liu, University of North Texas Health Science Center, United StatesReviewed by:
Xiao Bao, Yuebei People’s Hospital, ChinaCopyright © 2022 Li, Chen, Yue, Zhou, Gu, Tao, Guo, Zhu and Du. This is an open-access article distributed under the terms of the Creative Commons Attribution License (CC BY). The use, distribution or reproduction in other forums is permitted, provided the original author(s) and the copyright owner(s) are credited and that the original publication in this journal is cited, in accordance with accepted academic practice. No use, distribution or reproduction is permitted which does not comply with these terms.
*Correspondence: Meiwen Zhu, enh5eXptd0AxNjMuY29t; Qing Du, ZHVxaW5nQHhpbmh1YW1lZC5jb20uY24=
†These authors have contributed equally to this work and share first authorship
Disclaimer: All claims expressed in this article are solely those of the authors and do not necessarily represent those of their affiliated organizations, or those of the publisher, the editors and the reviewers. Any product that may be evaluated in this article or claim that may be made by its manufacturer is not guaranteed or endorsed by the publisher.
Research integrity at Frontiers
Learn more about the work of our research integrity team to safeguard the quality of each article we publish.