- 1Center for Innovation in Brain Science, University of Arizona, Tucson, AZ, United States
- 2Department of Pharmacology, University of Arizona College of Medicine, Tucson, AZ, United States
- 3MD-PhD Training Program, University of Arizona College of Medicine, Tucson, AZ, United States
- 4Department of Neurology, University of Arizona College of Medicine, Tucson, AZ, United States
Objective: We sought to determine the impact of Type 2 Diabetes Mellitus (T2D) anti-hyperglycemic medications (A-HgM) on risk of Alzheimer’s disease (AD) and related dementias (ADRD) outcomes including vascular dementia, and non-AD dementia such as frontotemporal, Lewy body, and mixed etiology dementias.
Research Design and Methods: This retrospective cohort study used the US-based Mariner claims dataset. 1,815,032 T2D participants 45 years and older with records 6 months prior and at least 3 years after the diagnosis of T2D were included. Claims were surveyed for a diagnosis of AD and ADRD 12 months post T2D diagnosis. A propensity score approach was used to minimize selection bias. Analyses were conducted between January 1st and February 28th, 2021.
Results: In this cohort study A-HgM exposure was associated with decreased diagnosis of AD (RR, 0.61; 95% CI, 0.59–0.62; p < 0.001), vascular dementia (RR, 0.72; 95% CI, 0.69–0.74; p < 0.001) and non-AD dementia (RR, 0.67; 95% CI, 0.66–0.68; p < 0.001). Metformin was associated with the greatest risk reduction and insulin with the least reduction in risk compared to patients not receiving A-HgM for ADRD risk. Of interest, patients with a diagnosis of AD, while either on metformin or insulin, were older in age and predominately female, than individuals on these drugs that did not develop AD. Mean (SD) follow-up was 6.2 (1.8) years.
Conclusion: After controlling for age, sex, and comorbidities, A-HgM in patients with T2D was associated with a reduced risk of AD and ADRD. These findings provide evidence in support of T2D as a risk factor for AD and ADRD and the beneficial impact of early and effective control of hyperglycemia to mitigate risk.
Introduction
Type 2 diabetes (T2D) is a lifestyle-associated disease with widespread prevalence (International Diabetes Federation, 2019). The hallmark of T2D is dysregulation of glucose metabolism resulting in hyperglycemia. According to a recent report by International Diabetes Federation (2019), the number of patients with T2D worldwide was 463 million in 2019, indicating that one in eleven adults are diabetic. However, this statistic is likely an underestimate of the burden of this disease as it is predicted that, worldwide, one in two patients with diabetes are undiagnosed in most under-developed and developing countries (Cho et al., 2018).
Multiple population-based studies have reported an association between T2D and cognitive impairment (Hassing et al., 2004; Strachan, 2011), Alzheimer’s disease (AD) (Li et al., 2015; Sun et al., 2020) and vascular dementia (VasD) (Cholerton et al., 2016; Teixeira et al., 2020). Factors that contribute to this increased risk include cardiovascular disease, hyperglycemia, inflammation, and oxidative stress (Rosales-Corral et al., 2015; Willette et al., 2015; Ferreira et al., 2018). Further, middle-aged and older adults with T2D experience a more rapid cognitive decline than those without T2D (McCrimmon et al., 2012).
Dementia, AD and VasD are progressive neurodegenerative disorders that manifest in impaired cognition, executive function, psychiatric symptoms and loss of independent living (2020). Dementia affects approximately 50 million people globally and is rapidly increasing in prevalence with AD and VasD as the most prevalent forms of dementia (2020). Late-onset AD (LOAD) primarily affects persons 65 years of age and older (2020). Risk factors of LOAD include APOE4 genotype, mid-life hypertension, mid-life metabolic dysregulation (metabolic syndrome and T2D), stroke and brain trauma (Edwards III et al., 2019). VasD is primarily caused by impaired cerebral blood flow or cerebrovascular disease, including stroke (Smith, 2017).
Medications that restore glucose control in T2D act through multiple mechanisms (Inzucchi et al., 2012). Broadly, anti-hyperglycemic medications (A-HgM) are divided into four main categories based on mechanism of action and include: (1) insulin sensitizers (biguanides and glitazones), (2) insulin secretagogues (sulfonylureas and meglitinides), (3) incretin mimetics (GLP1 agonists and DPP4 inhibitors) and (4) insulin. Insulin is indicated to patients who fail to meet glycemic targets on multiple non-insulin therapeutics, patients with catabolic symptoms suggestive of insulin deficiency or patients with advanced disease who have lost significant endogenous insulin secretory capacity.
Multiple studies have evaluated the effect of metformin on cognitive decline with mixed results (Moore et al., 2013; Herath et al., 2016; Orkaby et al., 2017; Scherrer et al., 2019; Samaras et al., 2020). Metformin, which is first line therapy for the treatment of T2D, is a biguanide in the insulin sensitizer class (Nasri and Rafieian-Kopaei, 2014). Metformin concentrates in the mitochondria due to its positive charge (Nasri and Rafieian-Kopaei, 2014). Mitochondrial dysfunction is well documented as a potential phenotype during the prodromal phase of AD making metformin an interesting target in AD treatment (Chakravorty et al., 2019; Wang et al., 2020). Studies have shown a benefit of metformin, particularly in comparison to the sulfonylurea medication class in reducing the risk of AD such that many studies use metformin as the control group for comparison with other A-HgM agents (Rizzo et al., 2014; Orkaby et al., 2017). In a recent study involving approximately 2,000 patients with T2D, metformin use was associated with better memory performance over time and dipeptidyl peptidase-4 (DDP-4) inhibitors slowed the rate of memory decline with the greatest benefit in APOE4 carriers (Wu et al., 2020).
This study sought to determine the impact of A-HgM overall and as individual mechanistic drug classes on the incidence of newly diagnosed AD, VasD or non-AD dementia outcomes in patients with T2D over the age 45. The comparator group used for this study was patients with T2D without exposure to A-HgM agents and control their diabetes with lifestyle modifications. Analyses were conducted using a US-based population insurance claims dataset and a substantially larger number of patients than previously reported (Moore et al., 2013; Orkaby et al., 2017; Samaras et al., 2020). The majority of studies involved small number of patients (from 80 to 1455). The two larger studies involving tens of thousands of patients involved the evaluation of a largely male population (veterans) (Orkaby et al., 2017; Scherrer et al., 2019). Herein, we report the association of individual A-HgM agents and their drug class families with reduced risk of development of age-associated AD, VasD and Non-AD dementia. Further, we conducted a responder’s analysis to identify and describe characteristics of patient groups who exhibited benefit vs. no benefit for AD outcomes with respect to A-HgM exposure.
Research Design and Methods
Data Source
The Mariner database is an insurance claims dataset that serves the United States with patient populations from all U.S. states and territories. Pearl Diver is for-fee research software that facilitates interaction with individual commercial, state-based Medicaid, Medicare stand-alone prescription drug plan, group Medicare Advantage, and individual Medicare Advantage data. The Mariner dataset contains patient demographic characteristics, prescription records, and numerous other data points for patients with Current Procedural Terminology, International Classification of Diseases, Ninth Revision (ICD-9), and International Statistical Classification of Diseases and Related Health Problems, Tenth Revision (ICD-10) codes. As of October 2020, Mariner encompasses all indications and represents 122 million patients throughout the duration of the set with claims from 2010 through the second quarter of 2018.
This report follows the Strengthening the Reporting of Observational Studies in Epidemiology (STROBE) reporting guideline. This study was approved by the University of Arizona Institutional Review Board. Requirements for informed consent were waived as the data were deidentified.
Study Design and Variables
The subset of 5,283,017 patients with Type 2 Diabetes Mellitus (T2D) were selected from the available Mariner dataset based on the presence of greater than one ICD code for T2D (Table 1). The outcome variable was defined as the occurrence of the first diagnosis of AD, VasD, or non-AD dementia based on ICD-9 and ICD-10 codes in the patient’s medical claims data (Table 2). An index date one year after the diagnosis of T2D was selected to allow for onboarding to therapy and to focus on long-term impact on disease progression given the prodromal nature of these neurodegenerative diseases. Participants younger than 45 years old, with a diagnosis of Type 1 Diabetes, or with a history of neurosurgery, brain cancer, or neurodegenerative disease prior to the index date were excluded from the study. An enrollment criterion in the claims dataset of at least 6 months prior to and 3 years after the diagnosis of T2D was applied. The enrollment criteria was required before any analysis of exposure to A-HgM for development of AD and ADRD for all patients to account for patients that may be leaving, dying, or switching the insurance provider (Figure 1). Additionally, the 3-year follow-up is not with respect to A-HgM exposure but instead with respect to T2D diagnosis. Patient groups were defined according to the therapeutic intervention used. The treatment group is defined as patients having at least one medication charge for an anti-hyperglycemic agent occurring after the diagnosis of T2D. This group was then stratified based on the mechanism of action of each therapeutic intervention for T2D (Tables 1, 3). The A-HgM group was defined as patients with one or more codes for insulin, metformin, glitazones, sulfonylureas, sodium-glucose cotransporter-2 (SGLT2) inhibitors, glucagon-like peptide (GLP-1) agonists, DPP4 inhibitors, glinides, or combination therapies (e.g., metformin and sulfonylureas) (Table 3). Drug groups with a low number of patients were not included in the sensitivity analysis evaluating the association between AD and ADRD and individual drug groups but were included in the relative risk analysis of overall A-HgM. Median adherence rates for each drug as well as the duration of treatment are reported in Table 1. Age in the study is defined by the age at diagnosis of T2D. Following the analysis in Branigan et al. (2020) and Torrandell-Haro et al. (2020), an analysis of comorbidities known to be associated with AD and ADRD outcomes was conducted (Table 2) which was used to generate a logistic regression-based propensity score matched cohort for treatment and control populations.
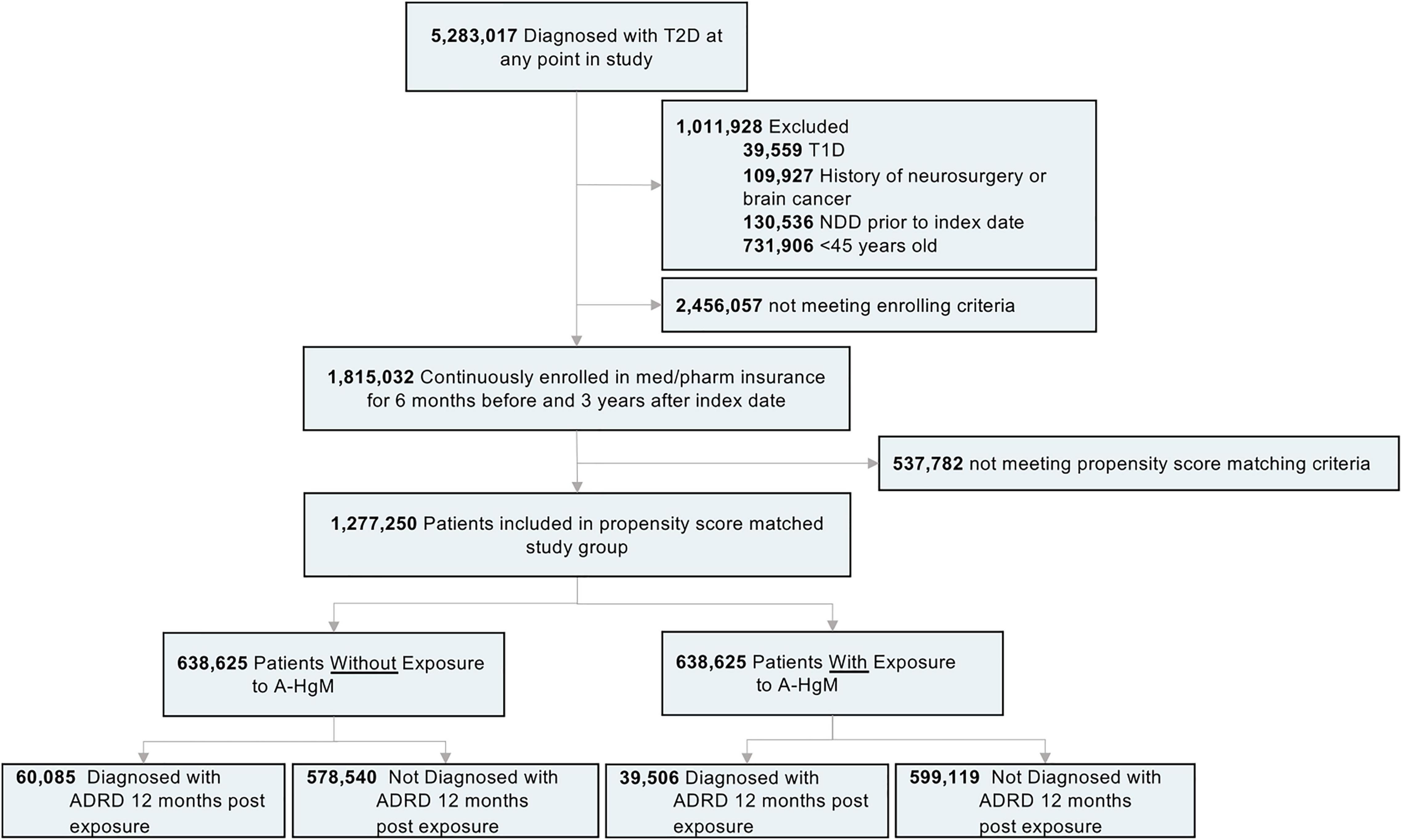
Figure 1. Study design and patient breakdown. T2D, type 2 diabetes; T1D, type 1 diabetes; NDD, neurodegenerative diseases; A-HgM, anti-hyperglycemic medication; ADRD, Alzheimer’s disease related dementias.
Statistical Analysis
Statistical analyses were conducted between January 1st and February 28th, 2021. Patient demographic statistics and incidence statistics were analyzed using unpaired two-tailed t-tests or χ2 tests, as appropriate, to test the significance of the differences between continuous and categorical variables. In all analyses, a two-sided P < 0.05 was considered statistically significant.
A propensity score-matched population was generated by using a logistic regression to identify confounding factors for therapeutic treatment of T2D as outcome between the treatment and control groups as previously reported (Branigan et al., 2020; Torrandell-Haro et al., 2020). In brief, the resulting factors included age, region, gender, Charlson Comorbidity Index (CCI) rank as well as variable comorbidities including Asthma, Chronic Obstructive Pulmonary Disease (COPD), Chronic Kidney Disease, Congestive Heart Failure, Coronary Artery Disease, Hypertension, Obesity, Pulmonary Heart Disease, Ischemic Heart Disease, Tobacco Use, Osteoarthritis, Rheumatoid Arthritis, Stroke and Tobacco Use (Tables 2, 4). These factors were then used to match patients in the treatment group to patients in the control group to minimize confounding variables in the patient populations. The matching was assessed by standardized mean difference with percentage balance improvement (Table 5). Kaplan–Meier survival curves for AD-Free Survival were created using the propensity score-matched population in the Bellwether-PearlDiver interface.
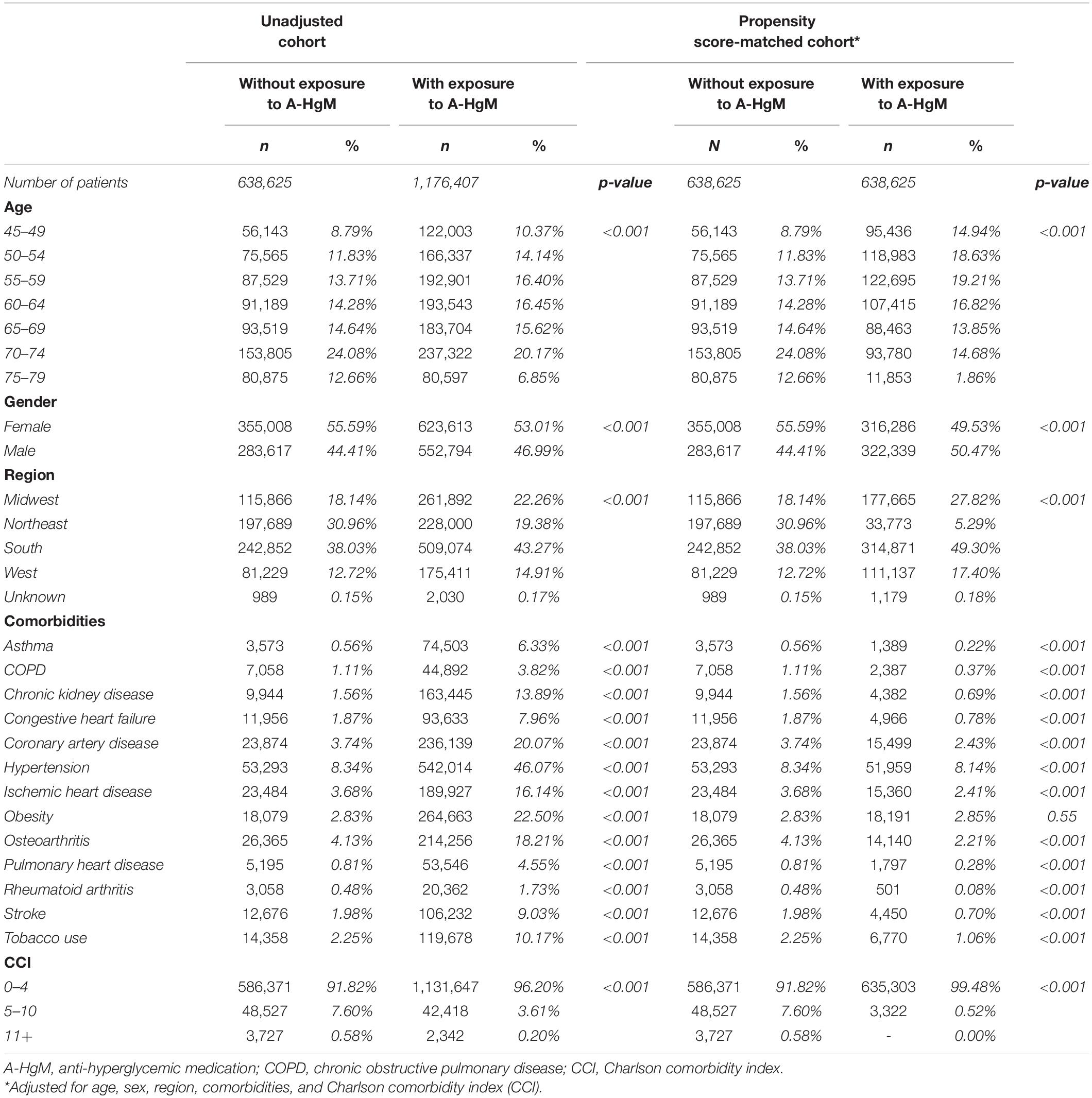
Table 4. Baseline characteristics for unadjusted and propensity score-matched patients with or without exposure to anti-hyperglycemic medication.
Results
In the Mariner dataset available for analysis, 5,283,017 patients were identified with a diagnosis of Type 2 Diabetes (Figure 1). The exclusion and enrollment criteria for the study was met by 1,815,032 patients (Figure 1). After propensity score matching for age, gender, and comorbidities as previously described, 638,625 patients (mean age [SD]: 61.85 [6.2] years) were not exposed to A-HgM and controlled their diabetes through lifestyle whereas 638,625 patients (57.37 [5.6] years) were exposed to at least one therapeutic option (Figure 1). Mean (SD) follow-up was 6.2 (1.8) years.
In the unadjusted cohort, patients that did not receive medication for the treatment of T2D were older (51.38% vs. 43.18% patients 65 and older) and had lower comorbidity incidence at baseline (3.6 [Rheumatoid arthritis] to 11.3 [asthma] fold reduction when compared to drug exposed group) (Table 4). Cardiovascular disease (approximately 4–5 fold reduction), chronic kidney disease (8.9 fold reduction) and obesity (7.9 fold reduction), all risk factors that affect the incidence of dementia, were reduced in the population with diabetes controlled through lifestyle interventions (Table 4). In contrast, after adjusting for age, gender, and comorbidities, those patients who were not exposed to A-HgM had a slightly higher incidence of comorbidities than those who were treated with A-HgM (Table 4). To address the severity of T2D, the number of A-HgM drugs in the treated group was determined: 342,783 (56.68%) of patients were treated with 2 or less A-HgM drugs, 273,574 (42.84%) were exposed to 3 drugs, and 22,268 (3.49%) were exposed to 4.
In the population receiving A-HgM, there was an overall decreased risk of a newly diagnosed AD (2.18% vs. 2.68%, RR: 0.81; 95% CI: 0.80–0.83, p < 0.001), VasD (1.14% vs. 1.28%, RR: 0.89; 95% CI: 0.87–0.91, p < 0.001) or non-AD dementia (4.62% vs. 5.45%, RR: 0.85; 95% CI: 0.84–0.86, p < 0.001) (Table 6). After propensity score matching, exposure to A-HgM agents was associated with a decreased incidence of AD (1.63% vs. 2.68%, RR: 0.61; 95% CI: 0.59–0.62, p < 0.001), VasD (0.91% vs. 1.28%, RR: 0.72; 95% CI: 0.69–0.74, p < 0.001) and non-AD dementia (3.64% vs. 5.45%, RR: 0.67; 95% CI: 0.66–0.68, p < 0.001) compared to control patients (lifestyle controlled/non-A-HgM exposure) (Table 6 and Figure 2). The number of patients needed to treat to reduce risk of AD, VasD, and non-AD dementia was 95.32, 274.9 and 55.26 respectively in the adjusted population (Table 6). Additional analyses indicated no significant sex differences in AD and ADRD risk reduction profiles between males and females when evaluated separately (Figure 3).
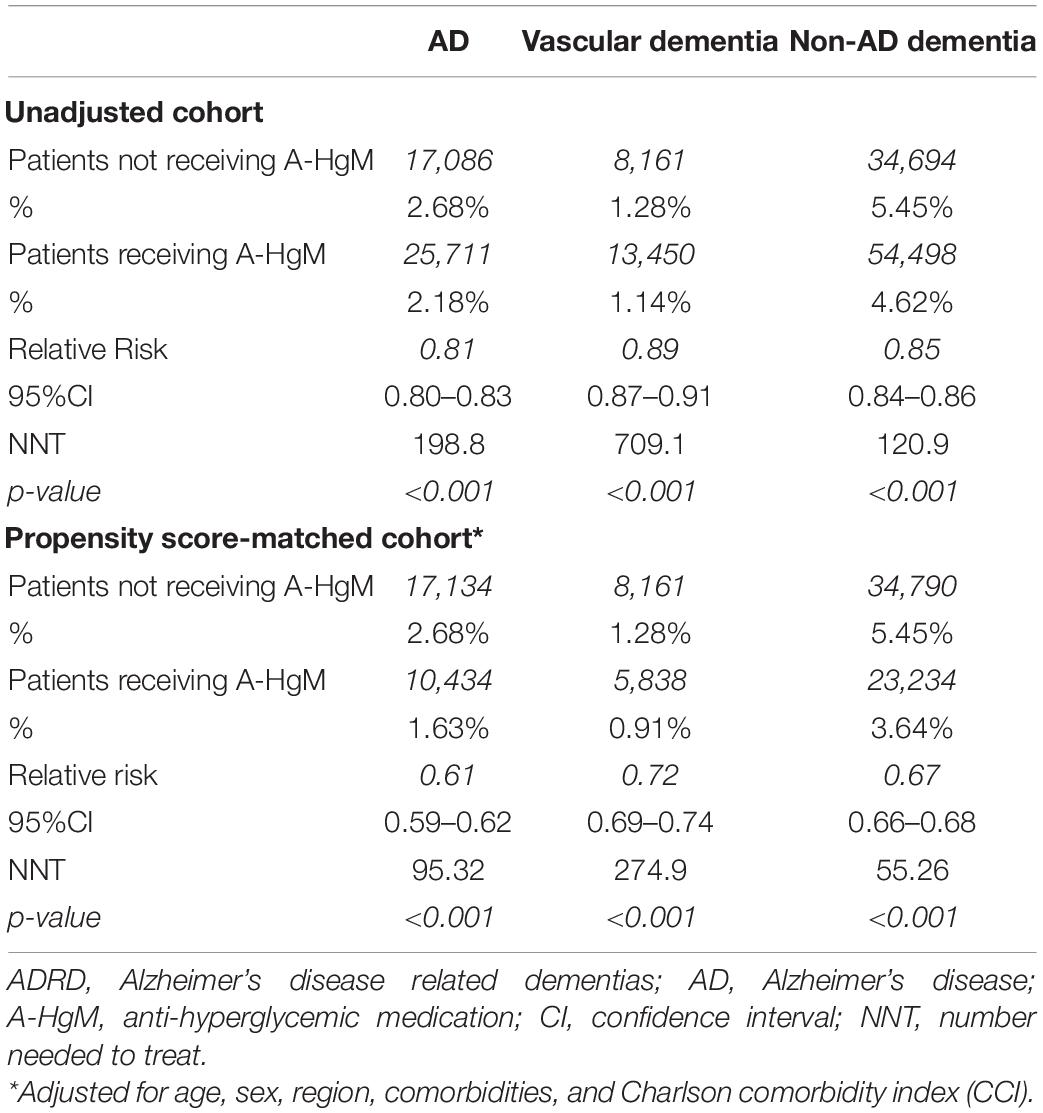
Table 6. Incidence and relative risk of patients taking anti-hyperglycemic medication to develop AD and ADRD.
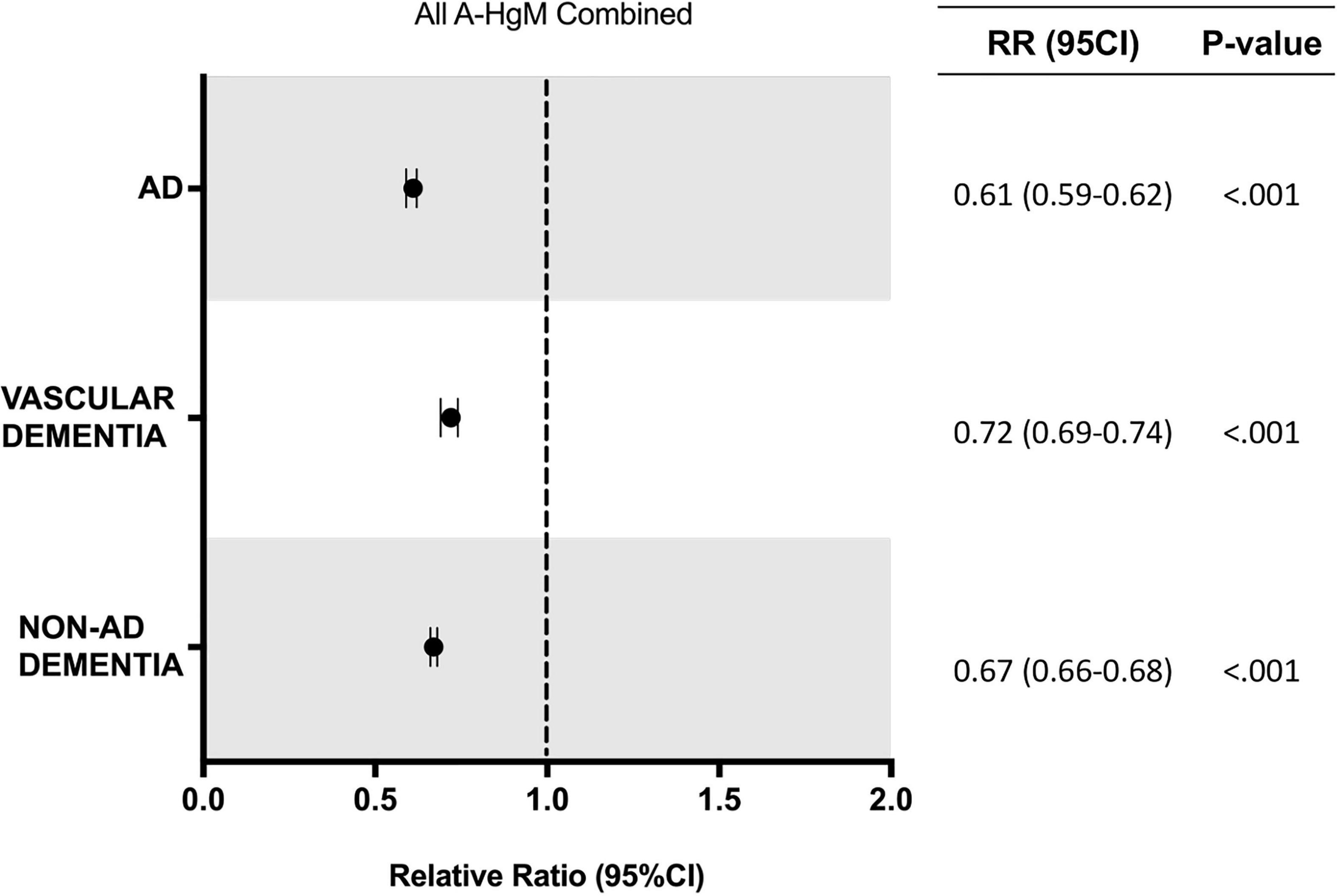
Figure 2. Relative risk of developing AD and ADRD for T2D patients with exposure to anti-hyperglycemic medication. AD, Alzheimer’s disease; RR, relative risk; CI, confidence interval.
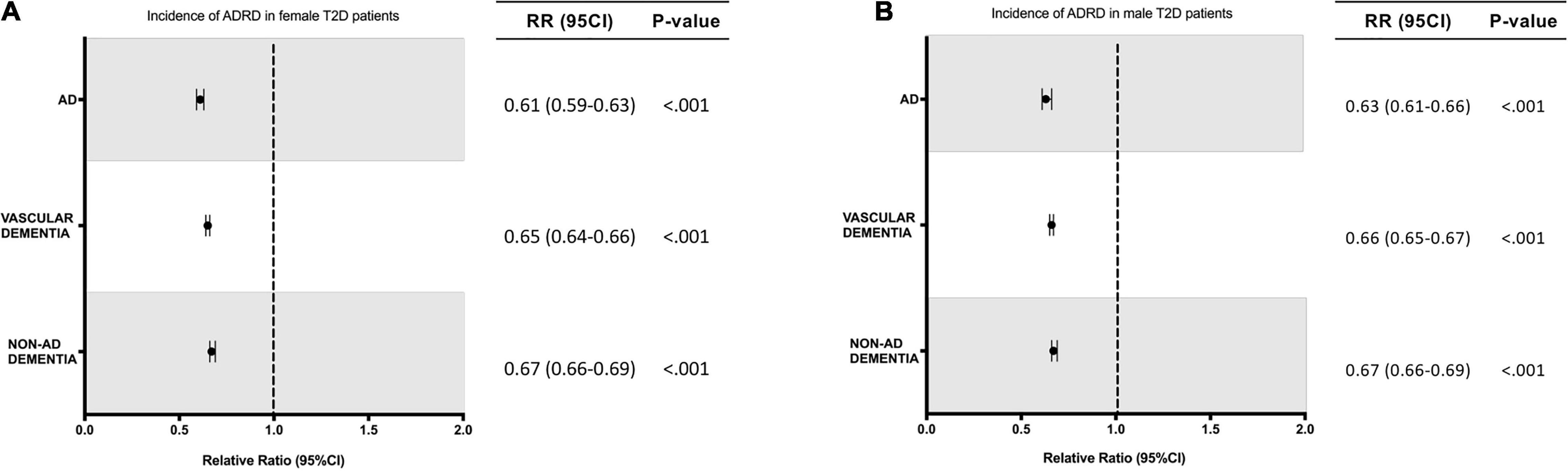
Figure 3. Relative risk of developing AD or ADRD for T2D women and men with exposure to anti-hyperglycemic medication. (A) Relative risk for T2D female patients. (B) Relative risk for T2D male patients. ADRD, Alzheimer’s disease related dementias; AD, Alzheimer’s disease; RR, relative risk; CI, confidence interval.
Within the propensity score matched population, the association of individual classes of drugs on AD, VasD and non-AD dementia outcomes was evaluated (Figure 4). Metformin was associated with the greatest reduction in risk of AD (1.44% vs. 2.68%, RR: 0.54; 95% CI: 0.52-0.55, p < 0.001) VasD (0.80% vs. 1.28%, RR: 0.63; 95% CI: 0.61–0.65, p < 0.001) and non-AD dementia (3.16% vs. 5.45%, RR: 0.58; 95% CI: 0.57–0.59, p < 0.001). The association between metformin and reduced ADRD risk was significantly greater than all other drug categories and showed non-overlapping 95% confidence intervals within a disease outcome (Figure 4). For incidence of non-AD dementia, insulin (4.76% vs. 5.45%, RR: 0.87; CI: 0.86–0.89, p-value < 0.001) use was associated with a significantly lower risk reduction than all other drug categories. For VasD, insulin was not associated with a change in risk (1.26%, vs. 1.28%, RR: 0.99; 95% CI: 0-95–1.03, p = 0.53) compared to control. For a new diagnosis of AD, insulin showed a smaller relative risk reduction than metformin, DDP4 inhibitors and metformin combined with sulfonylureas or glitazones.
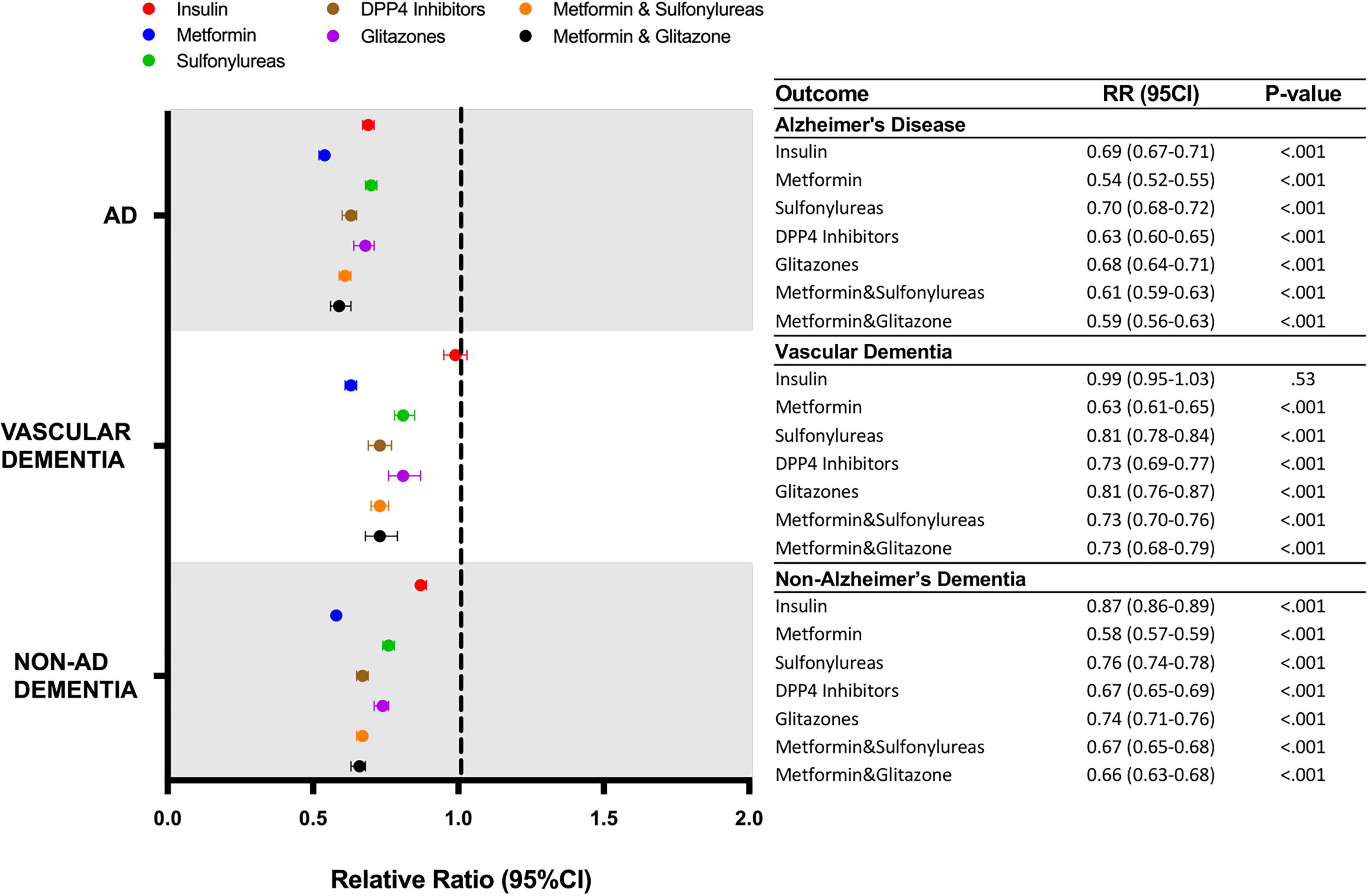
Figure 4. Relative risk of developing AD or ADRD for T2D patients receiving different classes of anti-hyperglycemic medication. AD, Alzheimer’s disease; DPP4, dipeptidyl-peptidase 4; RR, relative risk; CI, confidence interval.
Differences in the baseline characteristics of patients exposed to insulin and those exposed to metformin were determined (Table 7). The population exposed to insulin were slightly older and had a higher incidence of comorbidities. Specifically, they had a higher incidence of chronic kidney disease, congestive heart failure, coronary artery disease, pulmonary heart disease, and stroke (Table 7). Based on these differences, determination of baseline comorbidities of patients that developed AD while using insulin or metformin was investigated (Table 8). Patients who developed AD in both insulin and metformin exposed groups were older and predominantly female compared to patients who did not develop AD (Table 8). Patients who developed AD had an overall higher incidence of comorbidities but surprisingly, had a significantly lower incidence of obesity in both metformin and insulin populations compared to patients who did not develop AD. When comparing patients who developed AD with exposure to metformin versus exposure to insulin, there were significant differences in age and comorbidity rates. Patients who developed AD after metformin exposure were older than those who developed AD after insulin exposure. However, patients who developed AD after being exposed to insulin had a higher incidence of COPD, chronic kidney disease, chronic heart failure, coronary artery disease, obesity and stroke but had less incidence of hypertension (Table 8).
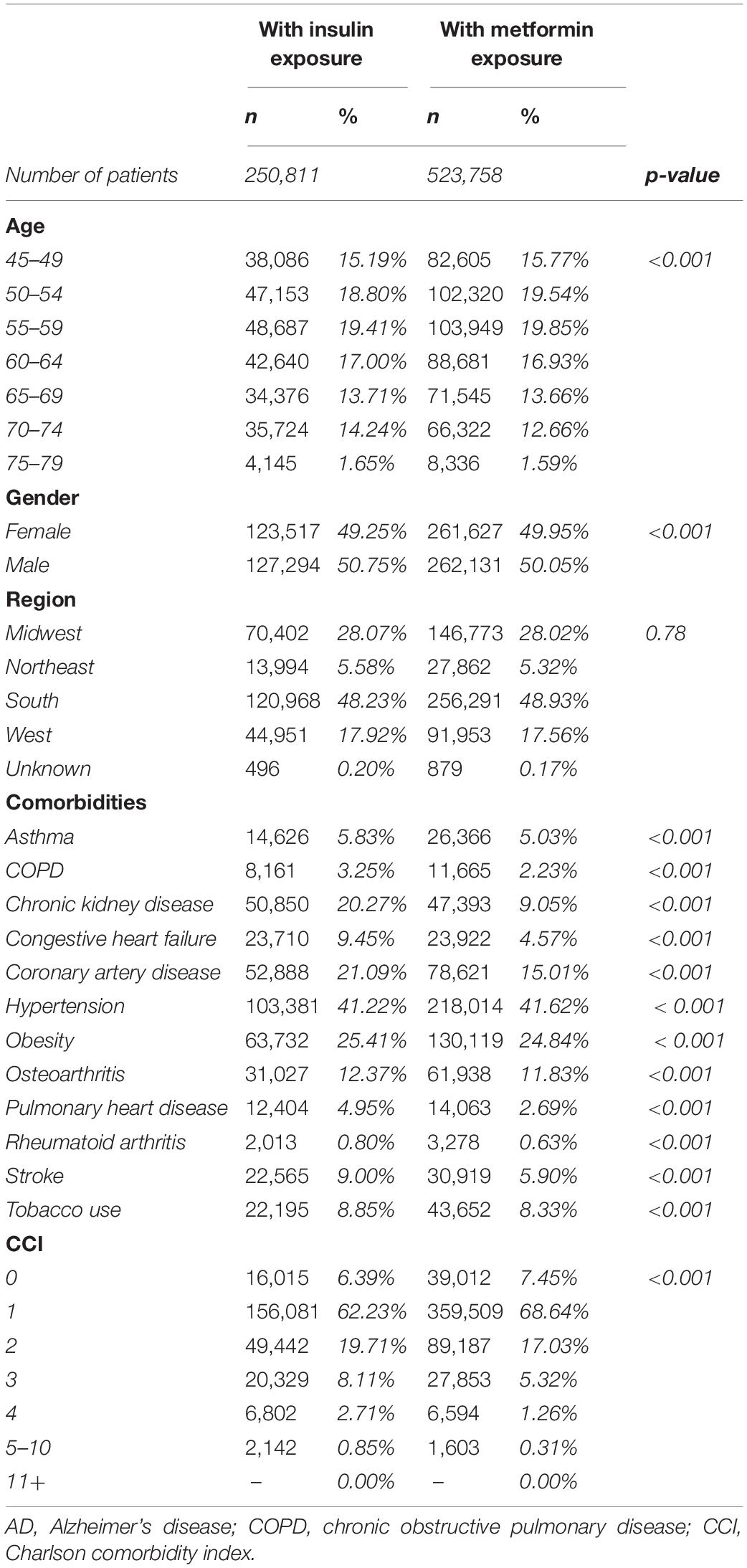
Table 7. Patient demographics and comorbidities in patients treated with insulin in comparison with patients treated with metformin.
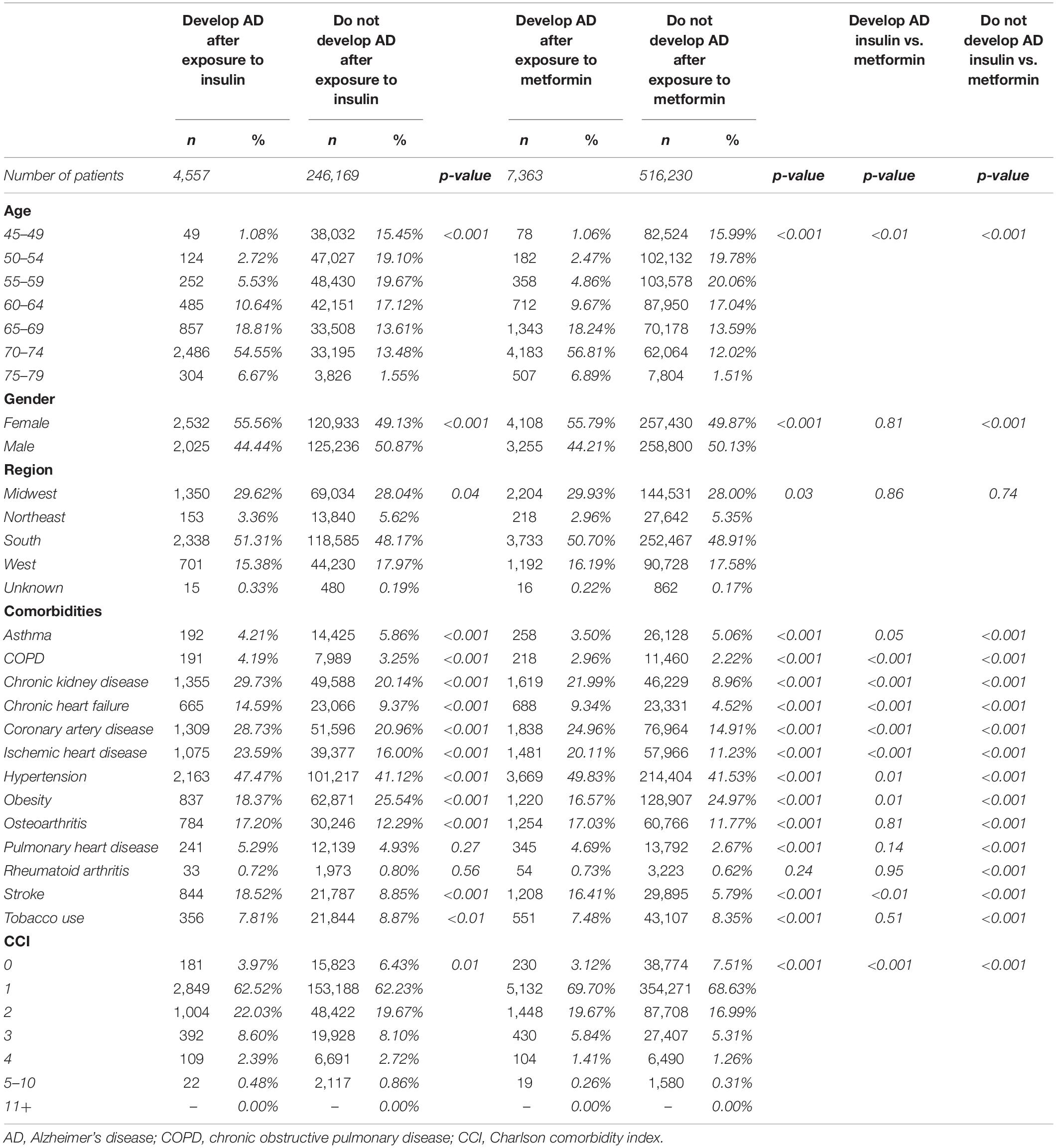
Table 8. Patient demographics and comorbidities in patients who develop and who do not develop AD treated with insulin in comparison with patients treated with metformin.
In Kaplan–Meier survival curves, depicting disease development over time, exposure to A-HgM was associated with a reduced conversion to AD and ADRD with the greatest risk reduction occurring in non-AD dementia outcomes followed by AD with A-HgM exposure showing the smallest risk reduction profile in VasD (Figure 5).
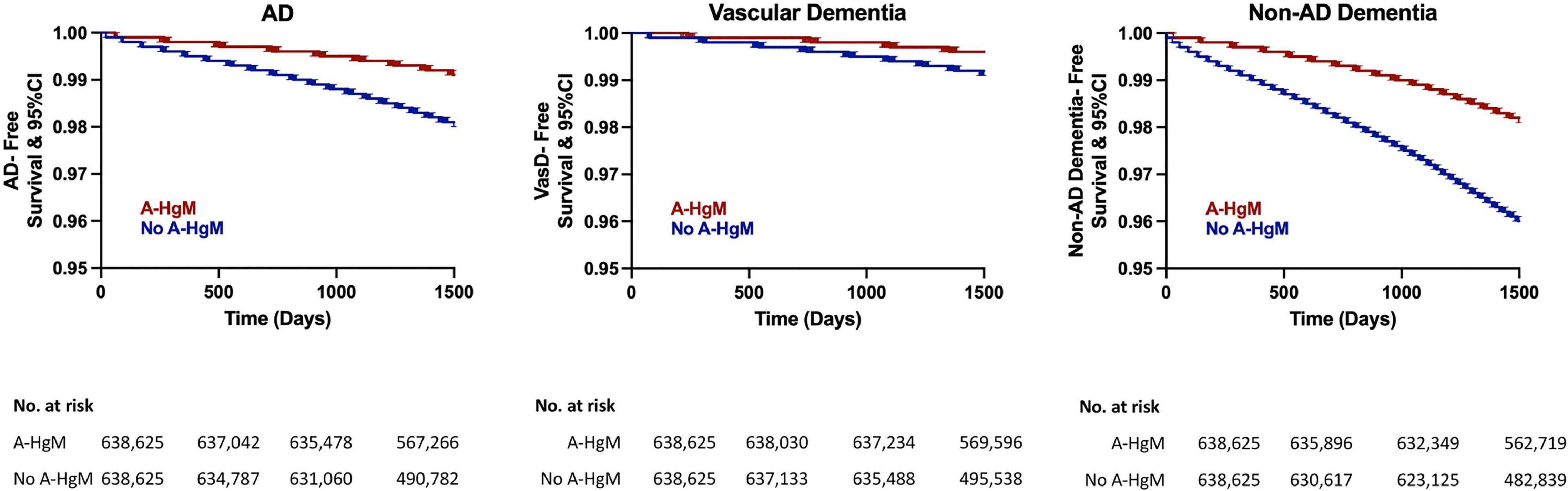
Figure 5. Kaplan–Meier survival curves for disease-free survival for AD and ADRD subtype (vascular dementia and non-AD dementia). AD, Alzheimer’s disease; A-HgM, anti-hyperglycemic medication; CI, confidence interval.
Discussion
There is growing evidence that use of A-HgM medications has the potential to reduce AD and ADRD risk (Bohlken et al., 2018; Boccardi et al., 2019). Based on this body of evidence, clinical trials to test the effects of A-HgM in patients with AD were conducted (Geldmacher et al., 2011; Koenig et al., 2017; Craft et al., 2020). In a small trial in 20 non-diabetic patients with MCI or mild dementia due to AD, metformin improved executive function (Trails-B test) (Koenig et al., 2017) which has led to an ongoing Phase II/III trial assessing metformin in a comparable population (NCT01965756). DPP4 inhibitors also have been reported to protect against cognitive decline in diabetic patients (Rizzo et al., 2014). Intranasal insulin administered over 12 months to patients with MCI or mild dementia due to AD did not show cognitive or functional improvement (Craft et al., 2020). Inconsistent results have been observed in clinical studies testing the effects of pioglitazone in AD patients (Geldmacher et al., 2011; Sato et al., 2011). However, these studies were small and evaluated the effects of A-HgM therapeutics in patients, not all T2D, with memory decline. The results from this study involve the evaluation of A-HgM exposure for prevention of AD and ADRD, not treatment, in patients with a risk factor for AD, T2D.
Overall, results of analyses reported herein indicated a positive association of anti-hyperglycemic exposure in T2D patients with reduced risk of AD and AD related dementias (ADRD) outcomes. In our initial non-adjusted analysis, patients without therapeutic intervention for diabetes were older, but had reduced incidence of several comorbidities (pulmonary and cardiovascular disease, arthritis, and obesity) and reduced tobacco use compared to control. These observations are consistent with clinical practice in which patients that control their diabetes with lifestyle changes tend to be healthier overall (Colberg et al., 2016). As many of these comorbidities are risk factors for the development of AD and ADRD, propensity score matching of the populations was conducted to isolate the contribution of A-HgM exposure alone. The association between anti-hyperglycemic agents and reduced risk was observed in both the unadjusted and adjusted populations. After matching for covariates of interest (Table 4), the association for reduced risk with A-HgM exposure in the propensity score matched population was greater than that observed in the unadjusted population suggesting that the comorbidities of interest can impact the efficacy of these therapies to reduce risk of AD and ADRD (Table 6 and Figure 2).
Metformin was associated with the greatest risk reduction in AD, VasD and non-AD dementia outcomes whereas insulin was associated with a smaller reduction in risk and was not associated with any change in VasD risk. This is consistent with a recent publication (Weinstein et al., 2019) that analyzed data pooled from five longitudinal cohorts where insulin use was associated with increased risk of developing dementia and decline in global cognitive function which led the authors to hypothesize that the increase in cognitive decline could be due to increased incidence of hypoglycemia. Of interest, T2D patients who developed AD were older and predominantly female compared to patients who did not develop AD. This is consistent with AD epidemiological data where age and female sex are the primary risk factors for the development of AD. These finding warrant further studies to determine potential subsets of responders and non-responders to these therapeutics. Taken together, our results support the hypothesis that early and effective control of hyperglycemia has the potential to reduce risk for AD and ADRD in the setting of T2D. Anti-hyperglycemic therapies can affect specific pathways of action of glucose metabolism and thus result in differential risk profiles based on the systems of biology affected by each therapeutic.
Limitations
This analysis has several limitations. First, as this study is a retrospective analysis of a claims database patients included may have obtained services outside of those included in this database for which we would not be able to address. Second, there could be factors, known and unknown, that even with propensity matching may not be adequately addressed. Thus, as with all observational studies, there are opportunities for bias in the interpretation of these data. We have attempted to address these through the use of propensity score matching and in our study design wherever possible. Third, lifestyle modifications, which are commonly used in the early stages of disease, could not be addressed in this study. Additionally, this dataset does not include information on glycemic index of the patients Fourth, this dataset does not contain data on ethnicity or race and therefore, those were not assessed in the analysis. Fifth, diagnosis of disease relied on the physician diagnosis and the ICD code assigned to each patient presentation. As the diagnosis of AD and ADRD is clinical, there may be overlap between AD and dementia codes given similar presentations despite different underlying pathophysiology. Furthermore, there could be biases in the prescribing trends for A-HgM that cannot be controlled in the model. Lastly, we did not address switching of A-HgM between therapeutic groups in our analysis.
Conclusion
Analysis of specific classes of A-HgM therapeutics advance a precision medicine approach for the prevention of Alzheimer’s Disease and related dementias. Exposure to anti-hyperglycemic agents was associated with reduced incidence of AD and ADRD. Further, individual therapies within A-HgM exert different risk reduction profiles with metformin exerting the greatest risk reduction. As T2D affects more than 400 million persons world-wide and is a risk factor for AD and ADRD, targeted A-HgM therapeutics to reduce risk of AD and ADRD could substantially impact long-term neurological health outcomes.
Data Availability Statement
The data analyzed in this study is subject to the following licenses/restrictions: Restrictions apply to the availability of some or all data generated or analyzed during this study to preserve patient confidentiality or because they were used under license. The corresponding author will on request detail the restrictions and any conditions under which access to some data may be provided. Requests to access these datasets should be directed to info@pearldiverinc.com.
Author Contributions
GT-H, GB, KR, and RB: full access to all the data in the study and take responsibility for the integrity of the data and the accuracy of the data analysis. RB and KR: obtain funding, administrative, technical, material support, supervision, and guarantor. GT-H and GB: statistical analysis. All authors: concept and design, acquisition, analysis, interpretation of data, drafting of the manuscript, and critical revision of the manuscript for important intellectual content.
Funding
This study was supported by grants from the National Institute on Aging grants P01AG026572 (Perimenopause in Brain Aging and Alzheimer’s Disease), T32AG061897 (Translational Research in Alzheimer’s Disease and Related Dementias [TRADD]), and R37AG053589 (Aging and Estrogenic Control of the Bioenergetic System in Brain) and the Women’s Alzheimer’s Movement to RB. R25 NS107185 (Undergraduate Readying for Burgeoning Research for American Indian Neuroscientists) and P01AG026572 (Peripheral Immune Activation on the Road to Development of Alzheimer’s Disease) to KR. The National Institutes of Health and the Women’s Alzheimer’s Movement Foundation had no role in the design and conduct of the study; collection, management, analysis, and interpretation of the data; preparation, review, or approval of the manuscript; and decision to submit the manuscript for publication.
Conflict of Interest
RB reported receiving grants from the Women’s Alzheimer’s Movement and the National Institute on Aging during the conduct of the study. KR reported receiving grants from the National Institute on Neurological Disease and Stroke during the conduct of the study.
The remaining authors declare that the research was conducted in the absence of any commercial or financial relationships that could be construed as a potential conflict of interest.
Publisher’s Note
All claims expressed in this article are solely those of the authors and do not necessarily represent those of their affiliated organizations, or those of the publisher, the editors and the reviewers. Any product that may be evaluated in this article, or claim that may be made by its manufacturer, is not guaranteed or endorsed by the publisher.
Acknowledgments
We would like to thank Lawrence Mandarino, for his insights regarding type-2 diabetes treatment and medical management and for his comments during the drafting of this manuscript.
References
Alzheimer’s Association (2020). 2020 Alzheimer’s disease facts and figures. Alzheimers Dement. 17, 327–406. doi: 10.1002/alz.12068
Boccardi, V., Murasecco, I., and Mecocci, P. (2019). Diabetes drugs in the fight against Alzheimer’s disease. Ageing Res. Rev. 54:100936. doi: 10.1016/j.arr.2019.100936
Bohlken, J., Jacob, L., and Kostev, K. (2018). Association Between the Use of Antihyperglycemic Drugs and Dementia Risk: a Case-Control Study. J. Alzheimer’s Dis. 66, 725–732. doi: 10.3233/JAD-180808
Branigan, G. L., Soto, M., Neumayer, L., Rodgers, K., and Brinton, R. D. (2020). Association Between Hormone-Modulating Breast Cancer Therapies and Incidence of Neurodegenerative Outcomes for Women With Breast Cancer. JAMA Netw. Open 3:e201541. doi: 10.1001/jamanetworkopen.2020.1541
Chakravorty, A., Jetto, C. T., and Manjithaya, R. (2019). Dysfunctional Mitochondria and Mitophagy as Drivers of Alzheimer’s Disease Pathogenesis. Front. Aging Neurosci. 11:311. doi: 10.3389/fnagi.2019.00311
Cho, N. H., Shaw, J. E., Karuranga, S., Huang, Y., da Rocha Fernandes, J. D., Ohlrogge, A. W., et al. (2018). IDF Diabetes Atlas: global estimates of diabetes prevalence for 2017 and projections for 2045. Diabetes Res. Clin. Pract. 138, 271–281. doi: 10.1016/j.diabres.2018.02.023
Cholerton, B., Baker, L. D., Montine, T. J., and Craft, S. (2016). Type 2 Diabetes, Cognition, and Dementia in Older Adults: toward a Precision Health Approach. Diabetes Spectr. 29, 210–219. doi: 10.2337/ds16-0041
Colberg, S. R., Sigal, R. J., Yardley, J. E., Riddell, M. C., Dunstan, D. W., Dempsey, P. C., et al. (2016). Physical Activity/Exercise and Diabetes: a Position Statement of the American Diabetes Association. Diabetes Care 39, 2065–2079. doi: 10.2337/dc16-1728
Craft, S., Raman, R., Chow, T. W., Rafii, M. S., Sun, C. K., Rissman, R. A., et al. (2020). Safety, Efficacy, and Feasibility of Intranasal Insulin for the Treatment of Mild Cognitive Impairment and Alzheimer Disease Dementia: a Randomized Clinical Trial. JAMA Neurol. 77, 1099–1109. doi: 10.1001/jamaneurol.2020.1840
Edwards, G. A. III, Gamez, N., Escobedo, G. Jr., Calderon, O., and Moreno-Gonzalez, I. (2019). Modifiable Risk Factors for Alzheimer’s Disease. Front. Aging Neurosci. 11:146. doi: 10.3389/fnagi.2019.00146
Ferreira, L. S. S., Fernandes, C. S., Vieira, M. N. N., and De Felice, F. G. (2018). Insulin Resistance in Alzheimer’s Disease. Front. Neurosci. 12:830. doi: 10.3389/fnins.2018.00830
Geldmacher, D. S., Fritsch, T., McClendon, M. J., and Landreth, G. (2011). A randomized pilot clinical trial of the safety of pioglitazone in treatment of patients with Alzheimer disease. Arch. Neurol. 68, 45–50. doi: 10.1001/archneurol.2010.229
Hassing, L. B., Grant, M. D., Hofer, S. M., Pedersen, N. L., Nilsson, S. E., Berg, S., et al. (2004). Type 2 diabetes mellitus contributes to cognitive decline in old age: a longitudinal population-based study. J. Int. Neuropsychol. Soc. 10, 599–607. doi: 10.1017/s1355617704104165
Herath, P. M., Cherbuin, N., Eramudugolla, R., and Anstey, K. J. (2016). The Effect of Diabetes Medication on Cognitive Function: evidence from the PATH Through Life Study. Biomed. Res. Int. 2016:7208429. doi: 10.1155/2016/7208429
International Diabetes Federation (2019). IDF Diabetes Atlas Ninth Edition 2019. Belgium: International Diabetes Federation.
Inzucchi, S. E., Bergenstal, R. M., Buse, J. B., Diamant, M., Ferrannini, E., Nauck, M., et al. (2012). Management of hyperglycaemia in type 2 diabetes: a patient-centered approach. Position statement of the American Diabetes Association (ADA) and the European Association for the Study of Diabetes (EASD). Diabetologia 55, 1577–1596. doi: 10.1007/s00125-012-2534-0
Koenig, A. M., Mechanic-Hamilton, D., Xie, S. X., Combs, M. F., Cappola, A. R., Xie, L., et al. (2017). Effects of the Insulin Sensitizer Metformin in Alzheimer Disease: pilot Data From a Randomized Placebo-controlled Crossover Study. Alzheimer Dis. Assoc. Disord. 31, 107–113. doi: 10.1097/wad.0000000000000202
Li, X., Song, D., and Leng, S. X. (2015). Link between type 2 diabetes and Alzheimer’s disease: from epidemiology to mechanism and treatment. Clin. Interv. Aging 10, 549–560. doi: 10.2147/CIA.S74042
McCrimmon, R. J., Ryan, C. M., and Frier, B. M. (2012). Diabetes and cognitive dysfunction. Lancet 379, 2291–2299. doi: 10.1016/s0140-6736(12)60360-2
Moore, E. M., Mander, A. G., Ames, D., Kotowicz, M. A., Carne, R. P., Brodaty, H., et al. (2013). Increased risk of cognitive impairment in patients with diabetes is associated with metformin. Diabetes Care 36, 2981–2987. doi: 10.2337/dc13-0229
Nasri, H., and Rafieian-Kopaei, M. (2014). Metformin: current knowledge. J. Res. Med. Sci. 19, 658–664.
Orkaby, A. R., Cho, K., Cormack, J., Gagnon, D. R., and Driver, J. A. (2017). Metformin vs sulfonylurea use and risk of dementia in US veterans aged ≥65 years with diabetes. Neurology 89, 1877–1885. doi: 10.1212/wnl.0000000000004586
Rizzo, M. R., Barbieri, M., Boccardi, V., Angellotti, E., Marfella, R., and Paolisso, G. (2014). Dipeptidyl peptidase-4 inhibitors have protective effect on cognitive impairment in aged diabetic patients with mild cognitive impairment. J. Gerontol. A Biol. Sci. Med. Sci. 69, 1122–1131. doi: 10.1093/gerona/glu032
Rosales-Corral, S., Tan, D. X., Manchester, L., and Reiter, R. J. (2015). Diabetes and Alzheimer disease, two overlapping pathologies with the same background: oxidative stress. Oxid. Med. Cell Longev. 2015:985845. doi: 10.1155/2015/985845
Samaras, K., Makkar, S., Crawford, J. D., Kochan, N. A., Wen, W., Draper, B., et al. (2020). Metformin Use Is Associated With Slowed Cognitive Decline and Reduced Incident Dementia in Older Adults With Type 2 Diabetes: the Sydney Memory and Ageing Study. Diabetes Care 43, 2691–2701. doi: 10.2337/dc20-0892
Sato, T., Hanyu, H., Hirao, K., Kanetaka, H., Sakurai, H., and Iwamoto, T. (2011). Efficacy of PPAR-γ agonist pioglitazone in mild Alzheimer disease. Neurobiol. Aging 32, 1626–1633. doi: 10.1016/j.neurobiolaging.2009.10.009
Scherrer, J. F., Morley, J. E., Salas, J., Floyd, J. S., Farr, S. A., and Dublin, S. (2019). Association Between Metformin Initiation and Incident Dementia Among African American and White Veterans Health Administration Patients. Ann. Fam. Med. 17, 352–362. doi: 10.1370/afm.2415
Smith, E. E. (2017). Clinical presentations and epidemiology of vascular dementia. Clin. Sci. 131, 1059–1068. doi: 10.1042/cs20160607
Strachan, M. W. J. (2011). R D Lawrence Lecture 2010^. The brain as a target organ in Type 2 diabetes: exploring the links with cognitive impairment and dementia. Diabet. Med. 28, 141–147. doi: 10.1111/j.1464-5491.2010.03199.x
Sun, Y., Ma, C., Sun, H., Wang, H., Peng, W., Zhou, Z., et al. (2020). Metabolism: a Novel Shared Link between Diabetes Mellitus and Alzheimer’s Disease. J. Diabetes Res. 2020:4981814. doi: 10.1155/2020/4981814
Teixeira, M. M., Passos, V. M. A., Barreto, S. M., Schmidt, M. I., Duncan, B. B., Beleigoli, A. M. R., et al. (2020). Association between diabetes and cognitive function at baseline in the Brazilian Longitudinal Study of Adult Health (ELSA- Brasil). Sci. Rep. 10:1596. doi: 10.1038/s41598-020-58332-9
Torrandell-Haro, G., Branigan, G. L., Vitali, F., Geifman, N., Zissimopoulos, J. M., and Brinton, R. D. (2020). Statin therapy and risk of Alzheimer’s and age-related neurodegenerative diseases. Alzheimer’s Dement. 6:e12108. doi: 10.1002/trc2.12108
Wang, W., Zhao, F., Ma, X., Perry, G., and Zhu, X. (2020). Mitochondria dysfunction in the pathogenesis of Alzheimer’s disease: recent advances. Mol. Neurodegener. 15:30. doi: 10.1186/s13024-020-00376-6
Weinstein, G., Davis-Plourde, K. L., Conner, S., Himali, J. J., Beiser, A. S., Lee, A., et al. (2019). Association of metformin, sulfonylurea and insulin use with brain structure and function and risk of dementia and Alzheimer’s disease: pooled analysis from 5 cohorts. PLoS One 14:e0212293. doi: 10.1371/journal.pone.0212293
Willette, A. A., Bendlin, B. B., Starks, E. J., Birdsill, A. C., Johnson, S. C., Christian, B. T., et al. (2015). Association of Insulin Resistance With Cerebral Glucose Uptake in Late Middle-Aged Adults at Risk for Alzheimer Disease. JAMA Neurol. 72, 1013–1020. doi: 10.1001/jamaneurol.2015.0613
Wu, C.-Y., Ouk, M., Wong, Y. Y., Anita, N. Z., Edwards, J. D., Yang, P., et al. (2020). Relationships between memory decline and the use of metformin or DPP4 inhibitors in people with type 2 diabetes with normal cognition or Alzheimer’s disease, and the role APOE carrier status. Alzheimer’s Dement. 16, 1663–1673. doi: 10.1002/alz.12161
Keywords: type 2 diabetes, Alzheimer’s disease, vascular dementia, anti-hyperglycemic agents, risk analyses
Citation: Torrandell-Haro G, Branigan GL, Brinton RD and Rodgers KE (2022) Association Between Specific Type 2 Diabetes Therapies and Risk of Alzheimer’s Disease and Related Dementias in Propensity-Score Matched Type 2 Diabetic Patients. Front. Aging Neurosci. 14:878304. doi: 10.3389/fnagi.2022.878304
Received: 17 February 2022; Accepted: 15 April 2022;
Published: 06 May 2022.
Edited by:
Ramesh Kandimalla, Indian Institute of Chemical Technology (CSIR), IndiaReviewed by:
Akila Prashant, JSS Medical College & Hospital, JSS Academy of Higher Education and Research, IndiaBrendon Pearce, University of the Western Cape, South Africa
Robert Rusina, Charles University, Czechia
Copyright © 2022 Torrandell-Haro, Branigan, Brinton and Rodgers. This is an open-access article distributed under the terms of the Creative Commons Attribution License (CC BY). The use, distribution or reproduction in other forums is permitted, provided the original author(s) and the copyright owner(s) are credited and that the original publication in this journal is cited, in accordance with accepted academic practice. No use, distribution or reproduction is permitted which does not comply with these terms.
*Correspondence: Kathleen E. Rodgers, krodgers@arizona.edu
†These authors share first authorship
‡These authors share senior authorship