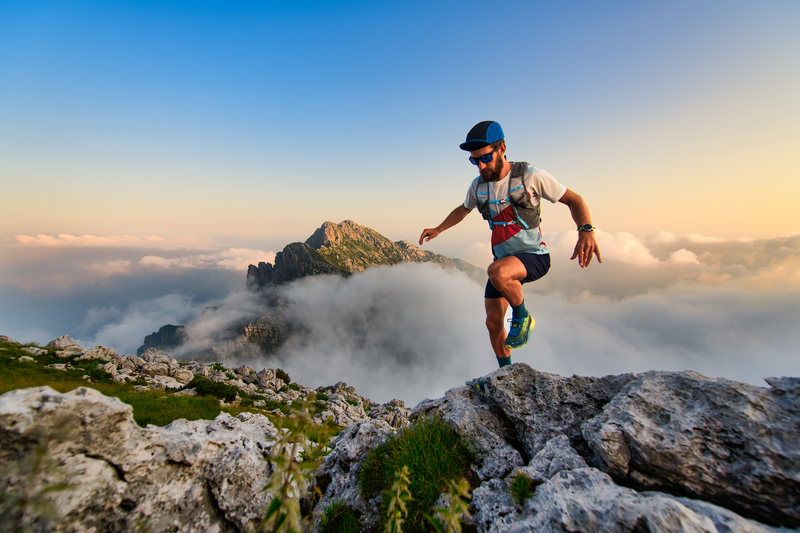
94% of researchers rate our articles as excellent or good
Learn more about the work of our research integrity team to safeguard the quality of each article we publish.
Find out more
ORIGINAL RESEARCH article
Front. Aging Neurosci. , 03 March 2022
Sec. Alzheimer's Disease and Related Dementias
Volume 14 - 2022 | https://doi.org/10.3389/fnagi.2022.811146
Introduction: Hippocampal atrophy is an established Alzheimer’s Disease (AD) biomarker. Volume loss in specific subregions as measurable with ultra-high field magnetic resonance imaging (MRI) may reflect earliest pathological alterations.
Methods: Data from positron emission tomography (PET) for estimation of cortical amyloid β (Aβ) and high-resolution 7 Tesla T1 MRI for assessment of hippocampal subfield volumes were analyzed in 61 non-demented elderly individuals who were divided into risk-categories as defined by high levels of cortical Aβ and low performance in standardized episodic memory tasks.
Results: High cortical Aβ and low episodic memory interactively predicted subicular volume [F(3,57) = 5.90, p = 0.018]. The combination of high cortical Aβ and low episodic memory was associated with significantly lower subicular volumes, when compared to participants with high episodic memory (p = 0.004).
Discussion: Our results suggest that low subicular volume is linked to established indicators of AD risk, such as increased cortical Aβ and low episodic memory. Our data support subicular volume as a marker of dementia-risk susceptibility in old-aged non-demented persons.
Cortical deposition of amyloid β (Aβ) plaques is a hallmark of Alzheimer’s Disease (AD), which is clinically characterized by deficits in episodic memory performance as the most typical and earliest clinical feature (Braak and Braak, 1991; Scheltens et al., 2016). The formation of Aβ plaques is believed to be one of the earliest changes in the hypothesized pathophysiological continuum of AD and to start decades before manifestation of the clinical syndrome (Braak and Braak, 1997; Jack et al., 2010; Sperling et al., 2011; Villemagne et al., 2013; Jansen et al., 2015; Parker et al., 2019). Several studies on healthy old-aged persons have consistently demonstrated an association between increased brain Aβ burden, reduced hippocampal volume, and increased risk for cognitive dysfunction (Rentz et al., 2010; Rodrigue et al., 2012; Vos et al., 2013; Hsu et al., 2015; Donohue et al., 2017; Bilgel et al., 2018; Fletcher et al., 2018; Haller et al., 2019; Parker et al., 2019; Svenningsson et al., 2019; Yassi et al., 2019). While the precise mechanism of how years of Aβ accumulation in the human brain may be linked to dementia is still not very well-understood, increased cerebral Aβ plaque load is nevertheless considered to represent a major risk factor for developing AD at old age (Tomlinson et al., 1968; Knopman et al., 2003; Bennett et al., 2006; Mintun et al., 2006; Rowe et al., 2007; Marchant et al., 2012; Jack et al., 2014). It is included in the criteria for the preclinical stages of AD and is increasingly used in clinical trials (Sperling et al., 2011; Sevigny et al., 2016). Yet, the predictive value of increased Aβ burden is compromised by a lack of specificity (Tomlinson et al., 1968; Mintun et al., 2006; Jack et al., 2014), and susceptibility of an individual at risk to eventually develop AD is known to be moderated by several factors (Prestia et al., 2015). Interestingly, risk for pathological cognitive decline at old age is significantly elevated when increased cerebral Aβ plaque load is associated with an already subtle cognitive dysfunction (Insel et al., 2016). Hence, further characterization of brain phenotypes (such as morphology) may contribute to a better understanding of the relationship between high cerebral Aβ plaque load in cognitively unimpaired subjects, and their individual risk for cognitive decline.
Subtle changes in episodic memory performance within the normal range may represent a prequel of the gradual progression of cognitive dysfunction in pre-clinical AD (Linn et al., 1995; Lange et al., 2002; Tierney et al., 2005; Albert, 2011; Bastin and Salmon, 2014; Pietrzak et al., 2015). Episodic memory dysfunction in old-aged persons is closely linked to hippocampal volume loss (Jack et al., 2000; Barnes et al., 2009; Dickerson and Eichenbaum, 2010; Gorbach et al., 2017; Nyberg, 2017). Considering a high degree of assumed functional specialization, atrophy of distinct hippocampal subfields may even better reflect earliest pathological changes of AD trajectories (de Flores et al., 2015a; Perrotin et al., 2015; Lindberg et al., 2017; Evans et al., 2018; Liang et al., 2018; Madusanka et al., 2019; Parker et al., 2019; Zhao et al., 2019; Dounavi et al., 2020; McKeever et al., 2020; Wong et al., 2020; Zhang et al., 2020).
Based on these earlier reports, we hypothesized that volume of distinct hippocampal subfields of cognitively normal old-aged adults may be associated with an individual’s risk-level for developing AD, as reflected by cortical Aβ-plaque deposition and episodic memory performance.
Participants were recruited in the cantone of Zurich, Switzerland, from ongoing studies at our center. All studies were conducted in compliance with local regulatory requirements, the Declaration of Helsinki and approved by the cantonal ethics committee of Zurich, Switzerland (World_Medical_Association., 1991).
Inclusion criteria for this project were age equal or above 50 years, written informed consent as approved by the local ethics authority, German language proficiency and unimpaired overall cognitive status as indicated by Mini−Mental State Examination (MMSE) ≥ 27/30. Exclusion criteria were presence of any condition possibly affecting cognition or study participation (e.g., severe hearing loss), any current medication or substance abuse with prompt effects on cognition, serious medical or psychiatric illness, any evidence of infarction or inflammation in the cranial MRI, contraindications to MRI or PET, or significant exposure to radiation. A total of 69 non-demented, cognitively normal old-aged persons completed both PET for brain Aβ-plaque burden (11C-PiB- or 18F-flutemetamol-PET) and high-field MRI at 7 T. Neuropsychological data was obtained for 68 participants. When stratifying the study sample by median split, seven participants with episodic memory or SUVR composite score equal to the median, were excluded.
All persons included in this analysis received standardized neuropsychological testing. Screening for cognitive impairment was performed by applying the MMSE (Folstein et al., 1975) and the Consortium to Establish a Registry for Alzheimer’s Disease (CERAD) neuropsychological battery (Sotaniemi et al., 2012). Episodic memory performance was assessed with the German Verbal Learning and Memory Test (VLMT) (Feurle et al., 1990; Helmstaedter et al., 2001), which is an adapted German language version of the Rey Auditory Verbal Learning Test (AVLT) (Muller et al., 1997; Lezak, 2004). The VLMT includes serial learning and recall of a 15-item word list over five trials. Recall performance was assessed immediately after reading the word list to the participant and after distraction (VLMT delayed recall measure). We created a composite measure of episodic memory by combining the z-standardized scores for immediate and delayed recall of the VLMT results for a more comprehensive assessment of episodic memory performance (Bastin and Salmon, 2014; Jonaitis et al., 2019).
All subjects were scanned using a Philips 7T Achieva whole-body scanner (Philips Healthcare, Best, Netherlands) equipped with a Nova Medical quadrature transmit head coil and 32-channel receive coil array, located at the Institute for Biomedical Engineering (IBT) at the Swiss Federal Institute of Technology at Zurich, Switzerland (ETH Zurich). A high-resolution T1-weighted 3D MP2RAGE image (TR/TE = 4.8 ms/2.1 ms, voxel size = 0.6 mm × 0.6 mm × 0.6 mm, SENSE-factor = 2 × 1 × 2, scan duration = 7:50 min) was acquired for anatomical referencing of brain structures and automated image segmentation.
Preprocessing of the T1 images was performed using the FreeSurfer software package Version 6.0 (Dale et al., 1999; Fischl et al., 1999, 2002, 2004a,b; Fischl and Dale, 2000; Han et al., 2006; Jovicich et al., 2006; Reuter et al., 2012). Pre-processing involved conversion from the three-dimensional nifti-format, motion correction and average of multiple volumetric T1-weighted images, transformation to Talairach space, intensity normalization, brain extraction, segmentation of the subcortical white and deep gray matter volumetric structures, tessellation of the gray and white matter boundary, automated topology correction, and surface deformation to optimally place the gray/white and gray/cerebrospinal fluid boundaries.
Hippocampal subfield segmentation was performed using algorithms implemented in FreeSurfer Version 6.01 (Iglesias et al., 2015). Bilateral hippocampi were segmented into 12 subfields each, representing across hemisphere averages as no lateralized hypothesis was followed: parasubiculum, presubiculum, subiculum, cornu ammonis (CA)1, CA2/3, CA4, granule cell layer of dentate gyrus (GC-DG), hippocampus-amygdala-transition-area (HATA), fimbria, molecular layer, hippocampal fissure, and hippocampal tail. Hippocampal segmentation quality was manually controlled for every participant. Intracranial volume (ICV), including brain tissues and other biological materials such as meninges and cerebrospinal fluid, was also estimated by applying FreeSurfer algorithms (Fischl and Dale, 2000).
Estimation of individual brain Aβ load was performed in 30 participants by applying 11C-labeled Pittsburgh Compound-B (PiB) positron emission tomography (PET) (Mathis et al., 2003; Klunk et al., 2004; Solbach et al., 2005). 11C-PiB PET scans were performed at the PET Center of the Division of Nuclear Medicine, Zurich University Hospital, as reported earlier by our group (Steininger et al., 2014; Gietl et al., 2015). In brief, an individual dose of approximately 350 MBq of 11C-labeled PiB was applied using intravenous access to the cubital vein. Images were corrected for attenuation (low-dose CT-based) and standard quantitative filtered back projection algorithm including necessary corrections for scatter, randoms and dead time were applied.
Cerebral amyloid deposition values were extracted from late-frame signals (minutes 50–70) using a standard routine as implemented in PMOD Brain Tool software-package (PNEURO, Version 3.4, PMOD Technologies Ltd., Zurich, Switzerland). As a single measure of individual cortical Aβ load, cortical PiB SUVR were calculated as reported earlier (Steininger et al., 2014; Gietl et al., 2015). In brief, a composite score based on cortical gray matter segmented regions of interest defined from Hammers maximum probability atlas (as implemented in PMOD Neuro Tool) were averaged and divided by cerebellar gray matter PIB Values. Regions of occipital lobe, insula and primary motor and sensorimotor were excluded. Gray matter segmentation as well as regions of interest analysis was performed on individual 3D MRI scans acquired close to the PET visit and visually controlled. We defined a PiB-measured “amyloid-positivity” by a mean cortical PiB-SUVR threshold of 1.4, as described earlier (Roberts et al., 2017).
Thirty one subjects were recruited from another ongoing study at our center, which used 18F-flutemetamol-PET to estimate individual cortical brain Aβ-plaque-load as described earlier by our group (van Bergen et al., 2018). The 18F-flutemetamol-PET was conducted at our research facilities at the Institute for Regenerative Medicine in Schlieren (IREM), Switzerland. An individual dose of 140 MBq of 18F-flutemetamol was injected into the cubital vein. Time-of-flight algorithms including necessary corrections were applied to reconstruct late frame PET-images (minutes 85–105). Standard MR imaging-based attenuation correction images were used to derive attenuation correction maps, which were generated using standard procedures implemented by the manufacturer. The resulting 3D-volumes of flutemetamol uptake (matrix = 256 × 256 × 89, voxel size = 1.2 mm × 1.2 mm × 2.78 mm) were also processed with PMOD Neuro Tool as the 11C-PiB-PET volumes. For defining 18F-flutemetamol measured “amyloid-positivity,” a SUVR threshold of 1.56 was used, which has been defined in earlier 18F-flutematamol studies on AD and healthy controls (Vandenberghe et al., 2010).
To be able to merge Aβ-plaque-load measures from the 11C-PiB-PET and 18F-flutemetamol-PET-groups, 18F- flutemetamol-, and 11C-PiB-based SUVRs were normalized with their respective established positivity thresholds (1.56 for 18F-flutemetamol and 1.4 for PiB). The yielded ratios of individual Aβ-plaque-load versus the positivity cut-off score of all subjects were then merged. The thus obtained individual Aβ-plaque-load ratios were then used for stratification of the study population.
A median split was performed on the episodic memory composite measure and the individual composite amyloid SUVR measure to divide the sample into four groups that reflect assumed individual risk for developing AD, as defined by earlier biomarkers studies on cognitive performance and brain Aβ-burden in old-aged, non-demented persons (Albert et al., 2001, 2011; Gomar et al., 2011; Jansen et al., 2015; Prestia et al., 2015; Elman et al., 2020; Papp et al., 2020). The four risk groups were defined as follows: (1) low-Aβ-high-episodic memory (lowest risk assumed), (2) low-Aβ-low-episodic memory, (3) high-Aβ-high-episodic memory, and (4) high-Aβ-low-episodic memory (highest risk assumed). To this effect, VLMT scores above the group median were categorized as “high episodic memory performance,” VLMT scores below the median as “low episodic memory performance.” Accordingly, composite SUVR scores above the group median of this study were categorized as “high-Aβ,” SUVR scores below the median as “low Aβ.”
Bilateral hippocampal subfield volumes were submitted to statistical analysis. Statistical analysis of volumes was designed to not include outliers, as defined by values of whole brain volume deviating more than 2.5 SD from the mean as this might point to a different pathology.
To test the effects of cortical Aβ and episodic memory on hippocampal subfield volumes, a multiple analysis of variance (MANOVA) was calculated with all 12 subfield volumes as dependent variables and group as independent variable. Following MANOVA, the group means of hippocampal subfield volumes were used for a cluster analysis (Mahalanobis distances) to identify AD risk subgroups with particular relevance for hippocampal subfield volumes. Mahalanobis distances is an established method to calculate statistic distances (independence) between data points (subgroups) (Mahalanobis, 1936; Kim, 2000; McLachlan, 2004). Statistic independence of data points is visualized in the resulting matrix of a hierarchichal binary clustertree (dendrogram), the graphical output of this function. Based on the results of this cluster analysis, the sample was then divided into a high-risk group with high cortical Aβ and low episodic memory performance and a low-risk group encompassing all other individuals. The ensuing one-way analyses of variance (ANOVA)s were calculated with all 12 subfield volumes as dependent variables and risk groups as independent variable. Post hoc analyses for each subfield were performed to compare their volumes between the high and the low-risk group and to test the interaction between cortical Aβ and episodic memory.
Multiple testing bias (false discovery rate, FDR) was allowed for using the Benjamini–Hochberg procedure (Benjamini and Hochberg, 1995). To this effect, all obtained p-values were FDR corrected, allowing estimation of test-significance at adjusted alpha = 5%.
The current analysis includes 61 cognitively normal old-aged study participants (Age 58–82 years; 22 females; Table 1).
Mean SUVR in study participants who received 11C-PiB-PET (n = 30) was 1.24 (SD = 0.25). In the group of study participants, who received 18F-flutemetamol-PET (n = 31), mean SUVR was 1.20 (SD = 0.32). Overall, 10 individuals were amyloid positive as defined by the established SUVR of ≥1.4 for PiB- and ≥1.56 for 18F-Flutemetamol-PET.
The average VLMT scores of all subjects were 9.59 (SD = 3.88) for the immediate recall and 9.18 (4.19) for the delayed recall.
Division of the sample by median split according to Aβ plaque load or episodic memory performance resulted in 30 subjects with low Aβ versus 31 subjects with high Aβ and 28 subjects with low episodic memory versus 33 subjects with high episodic memory. Allocation of the subjects to four groups representing AD risk generated group sizes of 13 subjects with high-Aβ-low-episodic memory, 17 subjects with high-Aβ-high-episodic memory, 15 subjects with low-Aβ-low-episodic memory, and 16 subjects with low-Aβ-high-episodic memory.
Hippocampal subfield segmentation resulted in accurate delineation of all 12 subfields in left and right hippocampi of all subjects (Figure 1). Accuracy of automatic subfield segmentation obtained by FreeSurfer 6.0 was consistent with hippocampal subfield tissue boundaries, which were visible in the high field strength 7T T1 images. The Anderson-Darling and the Jarque-Bera test for normality confirmed that data for none of the 12 subfields differed significantly from normal distribution (all p > 0.1), indicating that data were not skewed by over- or undersegmentation of any hippocampal subregion. Variability was assessed by standard deviation for each subfield. Coronal sections along the entire right-hemispheric hippocampus of one subject are shown in Figure 2.
Figure 1. Spatial definition of hippocampal subfields by 7 Tesla MP2RAGE. Coronal view of right-hemispheric hippocampal subfield segmentation (radiological convention) from one example subject, defined by using FreeSurfer V6.0 for segmentation of high resolution 7 Tesla MP2RAGE volumes. CA, cornu ammonis; GC-DG, granule cell layer of the dentate gyrus.
Figure 2. Additional views, hippocampal subfield delineation at 7 Tesla. Coronal view of hippocampal subfield segmentation (radiological display convention) at multiple locations of the same hippocampus. CA, cornu ammonis; GC-DG, granule cell layer of the dentate gyrus; HATA, hippocampus-amygdala-transition-area.
The MANOVA with the 12 subfields as dependent variables and the four aforementioned groups as independent variables showed a significant difference in volumes between groups, Wilk’s Lambda = 0.34, p = 0.039. The subsequent hierarchical clustering analysis revealed the lowest degree of statistical relatedness of the high-Aβ-low-episodic memory subgroup with the other subgroups, followed by the low-Aβ-high-episodic memory subgroup, while the other two subgroups (low-Aβ-low-episodic memory and high-Aβ-high-episodic memory) were closest related to all others. Cumulative distances (Mahalanobis) for each group were (SD), as defined by MANOVA statistic: Subgroup 1 (low-Aβ-low-episodic memory): 7.4 (1.3); subgroup 4 (high-Aβ-high-episodic memory): 8.6 (1.6); subgroup 2 (low-Aβ-high-episodic memory): 11.8 (2.5); subgroup 3 (high-Aβ-low-episodic memory, n = 13): 12.4 (2.5). Figure 3 depicts the distances between subgroups as obtained by Mahalanobis clustering, suggesting subgroup 3 as a separate entity. Based on this finding, we divided the sample into a high- and a low-risk group. The high-risk group corresponded to the high-Aβ-low-episodic memory group (n = 13) and the low-risk group (n = 48) was comprised of the other three groups. There were no significant differences in age, education and gender distributions between the thus defined high- and low-risk groups (all p > 0.05).
Figure 3. Mahalanobis distances of hippocampal subfield volumes indicate distinct effects conferred by the high-risk subgroup #3. In the Mahalanobis dendrogram statistic independence of data points is indicated by the length of the arms in the hierarchical binary cluster tree. The matrix shows that there is a low degree of statistical relatedness between effects of the high-risk subgroup #3 (high-Aβ-low-episodic memory group), with effects conferred by the other three subgroups: low-Aβ-low-episodic memory (subgroup 1), low-Aβ-high-episodic memory (subgroup 2), and high-Aβ-high-episodic memory (subgroup 4).
The one-way ANOVAs calculated to examine the differences in volumes of individual subfields between the high and the low-risk group (Figure 4) revealed most significant (both FDR-corrected p = 0.002) and strongest effects as indicated by eta squared effect sizes (0.222 and 0.2427, respectively) on subiculum and molecular layer (Table 2). Subiculum and molecular layer were thus further investigated for interactive effects of cortical Aβ and episodic memory performance. The effect sizes and FDR-corrected p-values for all subfields are listed in Table 2. The eta squared effect size for the volume reduction of CA1 (0.2045) was lower than for subiculum and molecular layer as was the level of significance (FDR-corrected p = 0.003).
Figure 4. Hippocampal subfield volumes by assumed Alzheimer’s disease (AD) risk. Mean subfield volumes of the high and low risk group with SEM and levels of significance for FDR-corrected p-values. SEM, standard error of mean; FDR, false discovery rate; *, FDR-corrected p < 0.05; **, FDR-corrected p < 0.01. CA, cornu ammonis; GC-DG, granule cell layer of the dentate gyrus; HATA, hippocampus-amygdala-transition-area.
The interaction between cortical Aβ and episodic memory performance was significant for subiculum, F(3,57) = 5.90, p = 0.018, but not molecular layer, F(3,57) = 3.66, p = 0.061. Secondary analysis using two-tailed, two-sample (unequal variance) t-tests within the high cortical Aβ subgroup revealed lower volumes of the subiculum [p = 0.004, mean mm3 left + right (SD) high episodic memory: 384.15(47.62); low episodic memory: 308.15(70.21)] and of the molecular layer [p = 0.003, mean mm3 left + right (SD) high episodic memory: 492.48(60.40); low episodic memory: 406.03(62.74)] in individuals with low versus individuals with high episodic memory performance. This effect was not observable for individuals within the low cortical Aβ subgroup [subiculum, p = 0.82, mean mm3 left + right (SD) high episodic memory: 392.17(50.52); low episodic memory: 398.27(87.48); molecular layer, p = 0.63, mean mm3 left + right (SD) high episodic memory: 516.79(71.71); low episodic memory: 502.85(84.34)] (Figure 5).
Figure 5. Subiculum and Molecular layer volumes by subgroup. Mean volumes of subiculum (A) and molecular layer (B) for low-Aβ-high-episodic memory, low-Aβ-low-episodic memory, high-Aβ-high-episodic memory and high-Aβ-low-episodic memory groups with SEM, and level of significance. SEM, standard error of measurement; *p < 0.05; **p < 0.01.
To our knowledge, this is the first report on an association of AD risk susceptibility, as defined by poorer episodic memory performance within the normal range and amyloid plaque load, with differential hippocampal subfield volume in cognitively normal elderly individuals.
Generally, with today’s clinically available methods, imaging biomarkers for downstream neurodegeneration become positive only after the manifestation of brain amyloidosis in the classical course of AD. Although hippocampal atrophy can be observed up to 10 years before disease onset, the decrease in the total volume of the hippocampus lacks specificity (Tondelli et al., 2012; Hatanpaa et al., 2014; Pini et al., 2016). Advances in structural imaging represent a promising approach for the development of more sensitive and specific methods in this field (Pini et al., 2016).
The mammalian hippocampus consists of several subfields, which have been reported to be variably affected by AD in both post-mortem histopathological and in vivo MRI investigations (Rossler et al., 2002; Zheng et al., 2018). Hippocampal subfield volumes might serve as a potential earlier and more specific biomarker for neurodegeneration due to AD (de Flores et al., 2015a; Pini et al., 2016). Histological studies of hippocampal pathology suggest pronounced neuronal loss in the CA1 and subiculum of persons with AD, when compared to healthy individuals (West et al., 1994; Price et al., 2001; Rossler et al., 2002; West et al., 2004).
Numerous in vivo MRI hippocampal subfield studies reported a significant volume loss of either almost all subfields with most pronounced effects or focal volume loss of CA1 or CA1 and subiculum in clinical manifest AD (Wang et al., 2006; Frisoni et al., 2008; Mueller and Weiner, 2009; Mueller et al., 2010; Apostolova et al., 2012; Lindberg et al., 2012; Wisse et al., 2014b; de Flores et al., 2015b; Perrotin et al., 2015; Tang et al., 2015; Madusanka et al., 2019). Moreover, subicular volume was found to be the single most predictive hippocampal subfield measure for AD diagnosis (Izzo et al., 2020). The findings of hippocampal subfield atrophy in AD could widely be replicated in patients with MCI, who generally had a more focal pattern of atrophy than AD patients (Chetelat et al., 2008; Mueller and Weiner, 2009; La Joie et al., 2013; de Flores et al., 2015b; Tang et al., 2015; Madusanka et al., 2019; Zhang et al., 2020). Between persons with MCI who converted to AD and non-converters most significant volume differences were reported for the subiculum and presubiculum in the Alzheimer’s Disease Neuroimaging Initiative cohort (Vasta et al., 2016). On the other hand, in MCI, also reports with no relevant atrophy in any hippocampal field have been reported, possibly due to low statistical power (Kerchner et al., 2013; Wisse et al., 2014b). Nevertheless, atrophy of subiculum and CA1 was also detected at very early stages, in cognitively healthy elders, who later developed MCI or AD as well as in elderly subjects with subjective cognitive decline (SCD) (Csernansky et al., 2005; Apostolova et al., 2010; Perrotin et al., 2015). In subjects at risk for AD, defined either by APOE4 carrier status or amyloidosis or positive family history, no conclusive subfield atrophy pattern is established yet (Donix et al., 2010; Dounavi et al., 2020; McKeever et al., 2020; Nadal et al., 2020). In healthy aging, no clear subfield volume evolution could be identified to date. While some studies found CA1 and subiculum to be most affected by age, others found these regions to be relatively preserved (de Flores et al., 2015a; Voineskos et al., 2015; Daugherty et al., 2016; Malykhin et al., 2017; Amaral et al., 2018). In a very recent study neither CA1 nor subiculum volumes expressed significant relationships with age (Bussy et al., 2021). Authors suggest, that the observed discrepancies in findings may be explained by different study designs (Bussy et al., 2021).
In our study we found distinct effects of subgroups as defined by amyloid load and episodic memory performance on hippocampal subfield volumes. Moreover, we observed a hierarchy of statistical independence of the subgroup’s effects ranging from the high-Aβ-low-episodic memory with the highest distance over the low-Aβ-high-episodic memory subgroup to the two other subgroups (low-Aβ-low-episodic memory and high-Aβ-high-episodic memory), that were closest related to all others. This might be consistent with the assumption that the four subgroups represent different stages in the risk spectrum for AD: the high-Aβ-low-episodic memory subgroup might represent the high-risk extreme, while the low-Aβ-high-episodic memory subgroup might be the low-risk extreme, and the low-Aβ-low-episodic memory and high-Aβ-high-episodic memory subgroups might constitute intermediate stages. We feel that this interpretation could be consistent with established concepts of biomarker-based assessment of aging-related brain pathology, where PET and cognitive performance represent independent, non-correlating, but complementary information (Jagust et al., 2009).
Our finding of differential hippocampal volume loss of the subiculum in the AD high-risk group compared to the low-risk group, comprised of the other three subgroups, is in line with the aforementioned results of subiculum atrophy in MCI and AD as well as a reported increased risk for cognitively healthy elders with low subicular volume to develop AD over time (Apostolova et al., 2010; Carlesimo et al., 2015). Moreover, to our knowledge, this is the first study to report a generalized difference in almost all subfields in cognitively normal elders according to AD risk profile, determined by Aβ load and episodic memory performance. While we found no significant difference in chronological age between the investigated groups, variation in Aβ and cognitive performance might reflect differences in biological age.
In contrast to other studies, we found profound but not most significant atrophy in the CA1. Partly, this might be explained by the fact, that atrophy in the CA1 has been suggested to be independent of Aβ plaque accumulation and our study sample was stratified by amyloidosis (La Joie et al., 2013; Ye et al., 2014). Also, it needs to be pointed out, that differing results might on the one hand also be explained by heterogenous methodology regarding hippocampus segmentation. On the other hand, differing results might partly be due to differing MRI resolution in the discussed reports. Except for the studies by Kerchner et al. and Wisse et al. all discussed studies were carried out at MRI field strengths below 7T. Previous MR studies performed at 7T often have investigated small sample sizes, which may limit power for detection of small effects (Kerchner et al., 2010, 2012, 2013, 2014; Boutet et al., 2014; Wisse et al., 2014b). Moreover, results pointing to atrophy in diverging subfields could possibly also be explained by different AD subtypes, that might have differing predilection atrophy sites not only in the neocortex, but also within the hippocampus.
Interestingly, lower subicular volume has already been reported to be associated with poorer performance in a test of immediate and delayed recall of a 15-word list (Carlesimo et al., 2015). Moreover, Zhao et al. (2019) recently reported that volume of left subiculum in comparison to other subfields is most strongly correlated with performance in AVLT measures in a mixed sample of persons with unimpaired cognition, SCD, amnestic MCI or AD. The fact that secondary analysis of our data revealed significant interactive effects of high Aβ and low episodic memory on subicular volume in a group of cognitively unimpaired persons is also in line with a recent report, that found a correlation of subicular volume with amyloid burden and memory decline in a group of subjects with MCI. In this group of MCI subjects, the correlation also existed for molecular layer and CA1 (Zhang et al., 2020). While we found most pronounced effects for the subiculum, the correlations described by Zhang et al. (2020) might represent a later stage of dementia-risk associated hippocampal subfield atrophy.
A major limitation of this study is the fact that it is cross-sectional. While 7T MRI provides very high contrast to noise ratio, longitudinal follow-up is complicated because of frequent changes in hardware and experimental MR-sequence setup. However, as outlined above, there are several other studies published at this point that corroborate subicular volume loss in later stages of AD (La Joie et al., 2013; Vasta et al., 2016; Madusanka et al., 2019; Zhao et al., 2019; Zhang et al., 2020). Another potential limitation lies in the used methodology for hippocampal subfield segmentation. Although the here employed FreeSurfer 6.0 software is the single one most applied software in this context and is increasingly recognized by experts in this field, there is an ongoing debate on the most proper method of segmentation (Wisse et al., 2014a,2021; Pini et al., 2016; Samann et al., 2020). The here used version 6.0 has overcome validation concerns of earlier versions with a novel statistical atlas of combined in vivo and ex vivo data for automatic, Bayesian segmentation (de Flores et al., 2015a; Iglesias et al., 2015). Moreover, there is a debate about the weighing of the source images. While FreeSurfer is able to segment T1 and T2 images as well as their combination, to date there is no clear demonstration of a superiority of either approach (Samann et al., 2020). The here used T1 MP2RAGE images are characterized by a particularly high gray matter/white matter contrast, which is crucial for valid subfield delineation. As longitudinal studies of hippocampal subfields are rare and inconclusive, results from long-term subfield volume observations as well as a harmonization of methods, which is currently undertaken by an international working group, would be of most interest for future studies (Pini et al., 2016).
To discuss another potential limitation of the present study, it has to be pointed out, that a switch in methodology for estimation of Aβ plaque load occurred after 31 subjects were recruited and had received 11C-PiB-PET due to the fact that subjects were recruited from two ongoing studies at our center. Accordingly, the latter 30 subjects were administered the 18F-flutemetamol PET tracer. As this might be a potential limitation, several studies verified the equivalency of 11C-PiB- and 18F-flutemetamol-PET as estimates for cerebral Aβ deposition, though, when both are standardized to cerebellar gray matter, as it was done in the present study (Vandenberghe et al., 2010; Hatashita et al., 2014; Adamczuk et al., 2016).
Taken together our data, as obtained by ultra-high field-strength MRI, indicate that hippocampal subfield atrophy in cognitively normal old-aged adults with higher-than-average cortical Aβ load and low episodic memory performance within the normal range, is a hallmark of increased risk for sporadic AD. The data also suggest that even subtle differences in episodic memory and amyloid load within normal variability can be meaningful as they correspond to significant structural changes as measurable with the sensitive and precise technique of ultra-high field 7TMRI. While our findings are consistent with many studies on brain change in old-aged persons with cognitive impairment, our data may support validity of subicular volume as a surrogate marker for Aβ burden related variation in episodic memory at high age. Thus, subicular atrophy might represent a critical neuroanatomical alteration in the prodrome of AD. Moreover, ultra-high field 7T MRI might be an appropriate technique to help to better identify subjects with a higher biological than chronological age as represented by stratification by biomarkers. Due to its small size, delineation of the entorhinal cortex is difficult even at field strenghts of 7T. In this study we did not suceed at delineating the entorhinal cortex. We hope that future developments in ultra-high field MRI will lead to valid measurement techniques of this region.
While the pathophysiological correlate of lower subicular volume in a context of AD-risk remains unclear at this point, earlier post-mortem studies in AD revealed the subiculum as a hippocampal subfield with particularly high levels of non-heme iron deposition and microglial activation (Zeineh et al., 2015; Madsen et al., 2020). This might accord with the notion of local non-heme iron deposition as a correlate of neurodegenerative brain pathology in AD (van Bergen et al., 2016; van Duijn et al., 2017; Ayton et al., 2019; Zhou et al., 2020). Longitudinal follow-up studies may clarify the temporal sequence of physiological changes, such as aggregation of pathological proteins, local iron deposition, neuronal disintegration, and tissue atrophy. Moreover, the future is believed to lie either in multimodal imaging approaches including Tau- and FDG-PET, fMRI or diffusion-tensor imaging, or even innovative imaging methods allowing for additional information on the underlying pathology that drives hippocampal subfield atrophy (de Flores et al., 2015a).
Last, it has to be mentioned, that these findings of ours also underline the pathogenic relevance of cerebral Aβ burden prior to significant cognitive impairment. Future therapeutic strategies aimed at prevention of AD dementia may allow for subtle Aβ related effects on brain integrity, as measurable by sensitive neuroimaging technology such as 7T MRI.
The raw data supporting the conclusions of this article will be made available by the authors upon reasonable request after evaluation by the authors and, if applicable, by the local ethics authority, without undue reservation.
The studies involving human participants were reviewed and approved by the cantonal ethics committee of Zurich, Switzerland. The patients/participants provided their written informed consent to participate in this study.
SMK: writing of the manuscript, subject recruitment, and statistical data analysis. CS: data processing, statistical analysis, and writing of the manuscript. JMGB: acquisition and processing of MRI data. SJS: acquisition of MRI data and neuropsychological workup of all subjects at time of MRI acquisition. RM and SCS: subject recruitment and extended neuropsychological workup of all participants. KPP and LV: assisted in acquiring data, quality control, and MR-sequence implementation at the 7 Tesla scanner at ETH Zürich. AFG: support of study design and conduct and acquisition of clinical data and PET data. VT and AB: acquisition and analysis of PET data. CH: sponsor of the study. PGU: study design and management, supervision of data processing and statistical analysis, final responsibility, and writing of the manuscript. All authors contributed to the article and approved the submitted version.
This project was supported by KFSP Molecular Imaging Network Zurich (MINZ), University of Zurich, the Mäxi Foundation, Swiss National Science Foundation, Synapsis Alzheimer Research Switzerland and institutional funding available to the Institute for Biomedical Engineering, University of Zurich, and ETH Zürich.
CH is an employee and a shareholder of Neurimmune.
The remaining authors declare that the research was conducted in the absence of any commercial or financial relationships that could be construed as a potential conflict of interest.
All claims expressed in this article are solely those of the authors and do not necessarily represent those of their affiliated organizations, or those of the publisher, the editors and the reviewers. Any product that may be evaluated in this article, or claim that may be made by its manufacturer, is not guaranteed or endorsed by the publisher.
We thank all participants for their study participation and all funding sources for making this study possible.
7T, 7 Tesla; A β, amyloid β; AD, Alzheimer’s disease; ANOVA, analysis of variance; AVLT, rey auditory verbal learning test; CA, cornu ammonis; CERAD, consortium to establish a registry for Alzheimer’s disease; GC-DG, granule cell layer of dentate gyrus; GCP, good clinical practice; HATA, hippocampus-amygdala-transition-area; ICV, intracranial volume; MMSE, mini − mental state examination; MANOVA, multiple analysis of variance; MRI, magnetic resonance imaging; PET, positron emission tomography; PiB, pittsburgh compound-B; SCD, subjective cognitive decline; SUVR, standardized uptake value ratio; VLMT, verbal learning and memory test.
Adamczuk, K., Schaeverbeke, J., Nelissen, N., Neyens, V., Vandenbulcke, M., Goffin, K., et al. (2016). Amyloid imaging in cognitively normal older adults: comparison between (18)F-flutemetamol and (11)C-Pittsburgh compound B. Eur. J. Nucl. Med. Mol. Imaging 43, 142–151. doi: 10.1007/s00259-015-3156-9
Albert, M. S. (2011). Changes in cognition. Neurobiol. Aging 32(Suppl. 1), S58–S63. doi: 10.1016/j.neurobiolaging.2011.09.010
Albert, M. S., DeKosky, S. T., Dickson, D., Dubois, B., Feldman, H. H., Fox, N. C., et al. (2011). The diagnosis of mild cognitive impairment due to Alzheimer’s disease: recommendations from the National Institute on Aging-Alzheimer’s Association workgroups on diagnostic guidelines for Alzheimer’s disease. Alzheimer’s Dement. 7, 270–279. doi: 10.1016/j.jalz.2011.03.008
Albert, M. S., Moss, M. B., Tanzi, R., and Jones, K. (2001). Preclinical prediction of AD using neuropsychological tests. J. Int. Neuropsychol. Soc. 7, 631–639. doi: 10.1017/s1355617701755105
Amaral, R. S. C., Park, M. T. M., Devenyi, G. A., Lynn, V., Pipitone, J., Winterburn, J., et al. (2018). Manual segmentation of the fornix, fimbria, and alveus on high-resolution 3T MRI: Application via fully-automated mapping of the human memory circuit white and grey matter in healthy and pathological aging. Neuroimage 170, 132–150. doi: 10.1016/j.neuroimage.2016.10.027
Apostolova, L. G., Green, A. E., Babakchanian, S., Hwang, K. S., Chou, Y. Y., Toga, A. W., et al. (2012). Hippocampal atrophy and ventricular enlargement in normal aging, mild cognitive impairment (MCI), and Alzheimer Disease. Alzheimer. Dis. Assoc. Disord. 26, 17–27. doi: 10.1097/WAD.0b013e3182163b62
Apostolova, L. G., Mosconi, L., Thompson, P. M., Green, A. E., Hwang, K. S., Ramirez, A., et al. (2010). Subregional hippocampal atrophy predicts Alzheimer’s dementia in the cognitively normal. Neurobiol. Aging 31, 1077–1088. doi: 10.1016/j.neurobiolaging.2008.08.008
Ayton, S., Wang, Y., Diouf, I., Schneider, J. A., Brockman, J., Morris, M. C., et al. (2019). Brain iron is associated with accelerated cognitive decline in people with Alzheimer pathology. Mol. Psychiatry 2019:7. doi: 10.1038/s41380-019-0375-7
Barnes, J., Bartlett, J. W., van de Pol, L. A., Loy, C. T., Scahill, R. I., Frost, C., et al. (2009). A meta-analysis of hippocampal atrophy rates in Alzheimer’s disease. Neurobiol. Aging 30, 1711–1723. doi: 10.1016/j.neurobiolaging.2008.01.010
Bastin, C., and Salmon, E. (2014). Early neuropsychological detection of Alzheimer’s disease. Eur. J. Clin. Nutr. 68, 1192–1199. doi: 10.1038/ejcn.2014.176
Benjamini, Y., and Hochberg, Y. (1995). Controlling the false discovery rate: a practical and powerful approach to multiple testing. J. R. Statist. Soc. Ser. B 57, 289–300.
Bennett, D. A., Schneider, J. A., Arvanitakis, Z., Kelly, J. F., Aggarwal, N. T., Shah, R. C., et al. (2006). Neuropathology of older persons without cognitive impairment from two community-based studies. Neurology 66, 1837–1844. doi: 10.1212/01.wnl.0000219668.47116.e6
Bilgel, M., An, Y., Helphrey, J., Elkins, W., Gomez, G., Wong, D. F., et al. (2018). Effects of amyloid pathology and neurodegeneration on cognitive change in cognitively normal adults. Brain 141, 2475–2485. doi: 10.1093/brain/awy150
Boutet, C., Chupin, M., Lehericy, S., Marrakchi-Kacem, L., Epelbaum, S., Poupon, C., et al. (2014). Detection of volume loss in hippocampal layers in Alzheimer’s disease using 7 T MRI: a feasibility study. Neuroimage Clin. 5, 341–348. doi: 10.1016/j.nicl.2014.07.011
Braak, H., and Braak, E. (1991). Neuropathological stageing of Alzheimer-related changes. Acta Neuropathol. 82, 239–259. doi: 10.1007/BF00308809
Braak, H., and Braak, E. (1997). Frequency of stages of Alzheimer-related lesions in different age categories. Neurobiol. Aging 18, 351–357.
Bussy, A., Plitman, E., Patel, R., Tullo, S., Salaciak, A., Bedford, S. A., et al. (2021). Hippocampal subfield volumes across the healthy lifespan and the effects of MR sequence on estimates. Neuroimage 233, 117931. doi: 10.1016/j.neuroimage.2021.117931
Carlesimo, G. A., Piras, F., Orfei, M. D., Iorio, M., Caltagirone, C., and Spalletta, G. (2015). Atrophy of presubiculum and subiculum is the earliest hippocampal anatomical marker of Alzheimer’s disease. Alzheimers Dement. 1, 24–32. doi: 10.1016/j.dadm.2014.12.001
Chetelat, G., Fouquet, M., Kalpouzos, G., Denghien, I., De la Sayette, V., Viader, F., et al. (2008). Three-dimensional surface mapping of hippocampal atrophy progression from MCI to AD and over normal aging as assessed using voxel-based morphometry. Neuropsychologia 46, 1721–1731. doi: 10.1016/j.neuropsychologia.2007.11.037
Csernansky, J. G., Wang, L., Swank, J., Miller, J. P., Gado, M., McKeel, D., et al. (2005). Preclinical detection of Alzheimer’s disease: hippocampal shape and volume predict dementia onset in the elderly. Neuroimage 25, 783–792. doi: 10.1016/j.neuroimage.2004.12.036
Dale, A. M., Fischl, B., and Sereno, M. I. (1999). Cortical surface-based analysis. I. Segmentation and surface reconstruction. Neuroimage 9, 179–194. doi: 10.1006/nimg.1998.0395
Daugherty, A. M., Bender, A. R., Raz, N., and Ofen, N. (2016). Age differences in hippocampal subfield volumes from childhood to late adulthood. Hippocampus 26, 220–228. doi: 10.1002/hipo.22517
de Flores, R., La Joie, R., and Chetelat, G. (2015a). Structural imaging of hippocampal subfields in healthy aging and Alzheimer’s disease. Neuroscience 309, 29–50. doi: 10.1016/j.neuroscience.2015.08.033
de Flores, R., La Joie, R., Landeau, B., Perrotin, A., Mezenge, F., de La Sayette, V., et al. (2015b). Effects of age and Alzheimer’s disease on hippocampal subfields: comparison between manual and FreeSurfer volumetry. Hum. Brain Mapp. 36, 463–474. doi: 10.1002/hbm.22640
Dickerson, B. C., and Eichenbaum, H. (2010). The episodic memory system: neurocircuitry and disorders. Neuropsychopharmacology 35, 86–104. doi: 10.1038/npp.2009.126
Donix, M., Burggren, A. C., Suthana, N. A., Siddarth, P., Ekstrom, A. D., Krupa, A. K., et al. (2010). Family history of Alzheimer’s disease and hippocampal structure in healthy people. Am. J. Psychiatry 167, 1399–1406. doi: 10.1176/appi.ajp.2010.09111575
Donohue, M. C., Sperling, R. A., Petersen, R., Sun, C. K., Weiner, M. W., Aisen, P. S., et al. (2017). Association Between Elevated Brain Amyloid and Subsequent Cognitive Decline Among Cognitively Normal Persons. JAMA 317, 2305–2316. doi: 10.1001/jama.2017.6669
Dounavi, M. E., Mak, E., Wells, K., Ritchie, K., Ritchie, C. W., Su, L., et al. (2020). Volumetric alterations in the hippocampal subfields of subjects at increased risk of dementia. Neurobiol. Aging 91, 36–44. doi: 10.1016/j.neurobiolaging.2020.03.006
Elman, J. A., Panizzon, M. S., Gustavson, D. E., Franz, C. E., Sanderson-Cimino, M. E., Lyons, M. J., et al. (2020). Amyloid-beta Positivity Predicts Cognitive Decline but Cognition Predicts Progression to Amyloid-beta Positivity. Biol. Psychiatry 87, 819–828. doi: 10.1016/j.biopsych.2019.12.021
Evans, T. E., Adams, H. H. H., Licher, S., Wolters, F. J., van der Lugt, A., Ikram, M. K., et al. (2018). Subregional volumes of the hippocampus in relation to cognitive function and risk of dementia. Neuroimage 178, 129–135. doi: 10.1016/j.neuroimage.2018.05.041
Feurle, G. E., Helmstaedter, V., Buehring, A., Bettendorf, U., and Eckardt, V. F. (1990). Distinct immunohistochemical findings in columnar epithelium of esophageal inlet patch and of Barrett’s esophagus. Dig. Dis. Sci. 35, 86–92. doi: 10.1007/BF01537228
Fischl, B., and Dale, A. M. (2000). Measuring the thickness of the human cerebral cortex from magnetic resonance images. Proc. Natl. Acad. Sci. U. S. A. 97, 11050–11055. doi: 10.1073/pnas.200033797
Fischl, B., Salat, D. H., Busa, E., Albert, M., Dieterich, M., Haselgrove, C., et al. (2002). Whole brain segmentation: automated labeling of neuroanatomical structures in the human brain. Neuron 33, 341–355. doi: 10.1016/s0896-6273(02)00569-x
Fischl, B., Salat, D. H., van der Kouwe, A. J., Makris, N., Segonne, F., Quinn, B. T., et al. (2004a). Sequence-independent segmentation of magnetic resonance images. Neuroimage 23(Suppl. 1), S69–S84. doi: 10.1016/j.neuroimage.2004.07.016
Fischl, B., Sereno, M. I., and Dale, A. M. (1999). Cortical surface-based analysis. II: Inflation, flattening, and a surface-based coordinate system. Neuroimage 9, 195–207. doi: 10.1006/nimg.1998.0396
Fischl, B., van der Kouwe, A., Destrieux, C., Halgren, E., Segonne, F., Salat, D. H., et al. (2004b). Automatically parcellating the human cerebral cortex. Cereb. Cortex 14, 11–22. doi: 10.1093/cercor/bhg087
Fletcher, E., Filshtein, T. J., Harvey, D., Renaud, A., Mungas, D., and DeCarli, C. (2018). Staging of amyloid beta, t-tau, regional atrophy rates, and cognitive change in a nondemented cohort: Results of serial mediation analyses. Alzheimers Dement. 10, 382–393. doi: 10.1016/j.dadm.2018.04.001
Folstein, M. F., Folstein, S. E., and McHugh, P. R. (1975). “Mini-mental state”. A practical method for grading the cognitive state of patients for the clinician. J. Psychiatr. Res. 12, 189–198.
Frisoni, G. B., Ganzola, R., Canu, E., Rub, U., Pizzini, F. B., Alessandrini, F., et al. (2008). Mapping local hippocampal changes in Alzheimer’s disease and normal ageing with MRI at 3 Tesla. Brain 131(Pt 12), 3266–3276. doi: 10.1093/brain/awn280
Gietl, A. F., Warnock, G., Riese, F., Kalin, A. M., Saake, A., Gruber, E., et al. (2015). Regional cerebral blood flow estimated by early PiB uptake is reduced in mild cognitive impairment and associated with age in an amyloid-dependent manner. Neurobiol. Aging 36, 1619–1628. doi: 10.1016/j.neurobiolaging.2014.12.036
Gomar, J. J., Bobes-Bascaran, M. T., Conejero-Goldberg, C., Davies, P., Goldberg, T. E., Alzheimer’s Disease, et al. (2011). Utility of combinations of biomarkers, cognitive markers, and risk factors to predict conversion from mild cognitive impairment to Alzheimer disease in patients in the Alzheimer’s disease neuroimaging initiative. Arch. Gen. Psychiatry 68, 961–969. doi: 10.1001/archgenpsychiatry.2011.96
Gorbach, T., Pudas, S., Lundquist, A., Oradd, G., Josefsson, M., Salami, A., et al. (2017). Longitudinal association between hippocampus atrophy and episodic-memory decline. Neurobiol. Aging 51, 167–176. doi: 10.1016/j.neurobiolaging.2016.12.002
Haller, S., Montandon, M. L., Rodriguez, C., Garibotto, V., Herrmann, F. R., and Giannakopoulos, P. (2019). Hippocampal Volume Loss, Brain Amyloid Accumulation, and APOE Status in Cognitively Intact Elderly Subjects. Neurodegener. Dis. 19, 139–147. doi: 10.1159/000504302
Han, X., Jovicich, J., Salat, D., van der Kouwe, A., Quinn, B., Czanner, S., et al. (2006). Reliability of MRI-derived measurements of human cerebral cortical thickness: the effects of field strength, scanner upgrade and manufacturer. Neuroimage 32, 180–194. doi: 10.1016/j.neuroimage.2006.02.051
Hatanpaa, K. J., Raisanen, J. M., Herndon, E., Burns, D. K., Foong, C., Habib, A. A., et al. (2014). Hippocampal sclerosis in dementia, epilepsy, and ischemic injury: differential vulnerability of hippocampal subfields. J. Neuropathol. Exp. Neurol. 73, 136–142. doi: 10.1097/OPX.0000000000000170
Hatashita, S., Yamasaki, H., Suzuki, Y., Tanaka, K., Wakebe, D., and Hayakawa, H. (2014). [18F]Flutemetamol amyloid-beta PET imaging compared with [11C]PIB across the spectrum of Alzheimer’s disease. Eur. J. Nucl. Med. Mol. Imaging 41, 290–300. doi: 10.1007/s00259-013-2564-y
Helmstaedter, C., Lendt, M., and Lux, S. (2001). Verbaler Lern- und Merkfähigkeitstest. Göttingen: Beltz Test GmbH.
Hsu, P. J., Shou, H., Benzinger, T., Marcus, D., Durbin, T., Morris, J. C., et al. (2015). Amyloid burden in cognitively normal elderly is associated with preferential hippocampal subfield volume loss. J. Alzheimers Dis. 45, 27–33. doi: 10.3233/JAD-141743
Iglesias, J. E., Augustinack, J. C., Nguyen, K., Player, C. M., Player, A., Wright, M., et al. (2015). A computational atlas of the hippocampal formation using ex vivo, ultra-high resolution MRI: Application to adaptive segmentation of in vivo MRI. Neuroimage 115, 117–137. doi: 10.1016/j.neuroimage.2015.04.042
Insel, P. S., Donohue, M. C., Mackin, R. S., Aisen, P. S., Hansson, O., Weiner, M. W., et al. (2016). Cognitive and functional changes associated with Abeta pathology and the progression to mild cognitive impairment. Neurobiol. Aging 48, 172–181. doi: 10.1016/j.neurobiolaging.2016.08.017
Izzo, J., Andreassen, O. A., Westlye, L. T., and van der Meer, D. (2020). The association between hippocampal subfield volumes in mild cognitive impairment and conversion to Alzheimer’s disease. Brain Res. 1728:146591. doi: 10.1016/j.brainres.2019.146591
Jack, C. R. Jr., Knopman, D. S., Jagust, W. J., Shaw, L. M., Aisen, P. S., Weiner, M. W., et al. (2010). Hypothetical model of dynamic biomarkers of the Alzheimer’s pathological cascade. Lancet Neurol. 9, 119–128. doi: 10.1016/S1474-4422(09)70299-6
Jack, C. R. Jr., Petersen, R. C., Xu, Y., O’Brien, P. C., Smith, G. E., Ivnik, R. J., et al. (2000). Rates of hippocampal atrophy correlate with change in clinical status in aging and AD. Neurology 55, 484–489. doi: 10.1212/wnl.55.4.484
Jack, C. R. Jr., Wiste, H. J., Weigand, S. D., Rocca, W. A., Knopman, D. S., Mielke, M. M., et al. (2014). Age-specific population frequencies of cerebral beta-amyloidosis and neurodegeneration among people with normal cognitive function aged 50-89 years: a cross-sectional study. Lancet Neurol. 13, 997–1005. doi: 10.1016/S1474-4422(14)70194-2
Jagust, W. J., Landau, S. M., Shaw, L. M., Trojanowski, J. Q., Koeppe, R. A., Reiman, E. M., et al. (2009). Relationships between biomarkers in aging and dementia. Neurology 73, 1193–1199. doi: 10.1212/WNL.0b013e3181bc010c
Jansen, W. J., Ossenkoppele, R., Knol, D. L., Tijms, B. M., Scheltens, P., Verhey, F. R., et al. (2015). Prevalence of cerebral amyloid pathology in persons without dementia: a meta-analysis. JAMA 313, 1924–1938. doi: 10.1001/jama.2015.4668
Jonaitis, E. M., Koscik, R. L., Clark, L. R., Ma, Y., Betthauser, T. J., Berman, S. E., et al. (2019). Measuring longitudinal cognition: Individual tests versus composites. Alzheimers Dement. 11, 74–84. doi: 10.1016/j.dadm.2018.11.006
Jovicich, J., Czanner, S., Greve, D., Haley, E., van der Kouwe, A., Gollub, R., et al. (2006). Reliability in multi-site structural MRI studies: effects of gradient non-linearity correction on phantom and human data. Neuroimage 30, 436–443. doi: 10.1016/j.neuroimage.2005.09.046
Kerchner, G. A., Berdnik, D., Shen, J. C., Bernstein, J. D., Fenesy, M. C., Deutsch, G. K., et al. (2014). APOE epsilon4 worsens hippocampal CA1 apical neuropil atrophy and episodic memory. Neurology 82, 691–697. doi: 10.1212/WNL.0000000000000154
Kerchner, G. A., Bernstein, J. D., Fenesy, M. C., Deutsch, G. K., Saranathan, M., Zeineh, M. M., et al. (2013). Shared vulnerability of two synaptically-connected medial temporal lobe areas to age and cognitive decline: a seven tesla magnetic resonance imaging study. J. Neurosci. 33, 16666–16672. doi: 10.1523/JNEUROSCI.1915-13.2013
Kerchner, G. A., Deutsch, G. K., Zeineh, M., Dougherty, R. F., Saranathan, M., and Rutt, B. K. (2012). Hippocampal CA1 apical neuropil atrophy and memory performance in Alzheimer’s disease. Neuroimage 63, 194–202. doi: 10.1016/j.neuroimage.2012.06.048
Kerchner, G. A., Hess, C. P., Hammond-Rosenbluth, K. E., Xu, D., Rabinovici, G. D., Kelley, D. A., et al. (2010). Hippocampal CA1 apical neuropil atrophy in mild Alzheimer disease visualized with 7-T MRI. Neurology 75, 1381–1387. doi: 10.1212/WNL.0b013e3181f736a1
Kim, M. G. (2000). Multivariate outliers and decompositions of Mahalanobis distance. Commun. Statist. Theory Methods 29, 1511–1526.
Klunk, W. E., Engler, H., Nordberg, A., Wang, Y., Blomqvist, G., Holt, D. P., et al. (2004). Imaging brain amyloid in Alzheimer’s disease with Pittsburgh Compound-B. Ann. Neurol. 55, 306–319. doi: 10.1002/ana.20009
Knopman, D. S., Parisi, J. E., Salviati, A., Floriach-Robert, M., Boeve, B. F., Ivnik, R. J., et al. (2003). Neuropathology of cognitively normal elderly. J. Neuropathol. Exp. Neurol. 62, 1087–1095. doi: 10.1093/jnen/62.11.1087
La Joie, R., Perrotin, A., de La Sayette, V., Egret, S., Doeuvre, L., Belliard, S., et al. (2013). Hippocampal subfield volumetry in mild cognitive impairment, Alzheimer’s disease and semantic dementia. Neuroimage Clin. 3, 155–162. doi: 10.1016/j.nicl.2013.08.007
Lange, K. L., Bondi, M. W., Salmon, D. P., Galasko, D., Delis, D. C., Thomas, R. G., et al. (2002). Decline in verbal memory during preclinical Alzheimer’s disease: examination of the effect of APOE genotype. J. Int. Neuropsychol. Soc. 8, 943–955. doi: 10.1017/s1355617702870096
Liang, X., Yin, Z., Liu, R., Zhao, H., Wu, S., Lu, J., et al. (2018). The Role of MRI Biomarkers and Their Interactions with Cognitive Status and APOE epsilon4 in Nondemented Elderly Subjects. Neurodegener. Dis. 18, 270–280. doi: 10.1159/000495754
Lindberg, O., Martensson, G., Stomrud, E., Palmqvist, S., Wahlund, L. O., Westman, E., et al. (2017). Atrophy of the Posterior Subiculum Is Associated with Memory Impairment, Tau- and Abeta Pathology in Non-demented Individuals. Front. Aging Neurosci. 9:306. doi: 10.3389/fnagi.2017.00306
Lindberg, O., Walterfang, M., Looi, J. C., Malykhin, N., Ostberg, P., Zandbelt, B., et al. (2012). Hippocampal shape analysis in Alzheimer’s disease and frontotemporal lobar degeneration subtypes. J. Alzheimers Dis. 30, 355–365. doi: 10.3233/JAD-2012-112210
Linn, R. T., Wolf, P. A., Bachman, D. L., Knoefel, J. E., Cobb, J. L., Belanger, A. J., et al. (1995). The ‘preclinical phase’ of probable Alzheimer’s disease. A 13-year prospective study of the Framingham cohort. Arch. Neurol. 52, 485–490. doi: 10.1001/archneur.1995.00540290075020
Madsen, S. J., DiGiacomo, P. S., Zeng, Y., Goubran, M., Chen, Y., Rutt, B. K., et al. (2020). Correlative Microscopy to Localize and Characterize Iron Deposition in Alzheimer’s Disease. J. Alzheimers Dis. Rep. 4, 525–536. doi: 10.3233/ADR-200234
Madusanka, N., Choi, H. K., So, J. H., Choi, B. K., and Park, H. G. (2019). One-year Follow-up Study of Hippocampal Subfield Atrophy in Alzheimer’s Disease and Normal Aging. Curr. Med. Imaging Rev. 15, 699–709. doi: 10.2174/1573405615666190327102052
Mahalanobis, P. C. (1936). On the generalised distance in statistics. Proc. Natl. Instit. Sci. India 2, 49–55.
Malykhin, N. V., Huang, Y., Hrybouski, S., and Olsen, F. (2017). Differential vulnerability of hippocampal subfields and anteroposterior hippocampal subregions in healthy cognitive aging. Neurobiol. Aging 59, 121–134. doi: 10.1016/j.neurobiolaging.2017.08.001
Marchant, N. L., Reed, B. R., DeCarli, C. S., Madison, C. M., Weiner, M. W., Chui, H. C., et al. (2012). Cerebrovascular disease, beta-amyloid, and cognition in aging. Neurobiol. Aging 33, e1025–e1036. doi: 10.1016/j.neurobiolaging.2011.10.001
Mathis, C. A., Wang, Y., Holt, D. P., Huang, G. F., Debnath, M. L., and Klunk, W. E. (2003). Synthesis and evaluation of 11C-labeled 6-substituted 2-arylbenzothiazoles as amyloid imaging agents. J. Med. Chem. 46, 2740–2754. doi: 10.1021/jm030026b
McKeever, A., Paris, A. F., Cullen, J., Hayes, L., Ritchie, C. W., Ritchie, K., et al. (2020). Hippocampal Subfield Volumes in Middle-Aged Adults at Risk of Dementia. J. Alzheimers Dis. 75, 1211–1218. doi: 10.3233/JAD-200238
McLachlan, G. (2004). Discriminant Analysis and Statistical Pattern Recognition. New Jersey, NJ: John Wiley & Sons.
Mintun, M. A., Larossa, G. N., Sheline, Y. I., Dence, C. S., Lee, S. Y., Mach, R. H., et al. (2006). [11C]PIB in a nondemented population: potential antecedent marker of Alzheimer disease. Neurology 67, 446–452. doi: 10.1212/01.wnl.0000228230.26044.a4
Mueller, S. G., Schuff, N., Yaffe, K., Madison, C., Miller, B., and Weiner, M. W. (2010). Hippocampal atrophy patterns in mild cognitive impairment and Alzheimer’s disease. Hum. Brain Mapp. 31, 1339–1347. doi: 10.1002/hbm.20934
Mueller, S. G., and Weiner, M. W. (2009). Selective effect of age, Apo e4, and Alzheimer’s disease on hippocampal subfields. Hippocampus 19, 558–564. doi: 10.1002/hipo.20614
Muller, H., Hasse-Sander, I., Horn, R., Helmstaedter, C., and Elger, C. E. (1997). Rey Auditory-Verbal Learning Test: structure of a modified German version. J. Clin. Psychol. 53, 663–671. doi: 10.1002/(sici)1097-4679(199711)53:7<663::aid-jclp4>3.0.co;2-j
Nadal, L., Coupe, P., Helmer, C., Manjon, J. V., Amieva, H., Tison, F., et al. (2020). Differential annualized rates of hippocampal subfields atrophy in aging and future Alzheimer’s clinical syndrome. Neurobiol. Aging 90, 75–83. doi: 10.1016/j.neurobiolaging.2020.01.011
Nyberg, L. (2017). Functional brain imaging of episodic memory decline in ageing. J. Intern. Med. 281, 65–74. doi: 10.1111/joim.12533
Papp, K. V., Buckley, R., Mormino, E., Maruff, P., Villemagne, V. L., Masters, C. L., et al. (2020). Clinical meaningfulness of subtle cognitive decline on longitudinal testing in preclinical AD. Alzheimers Dement. 16, 552–560. doi: 10.1016/j.jalz.2019.09.074
Parker, T. D., Cash, D. M., Lane, C. A. S., Lu, K., Malone, I. B., Nicholas, J. M., et al. (2019). Hippocampal subfield volumes and pre-clinical Alzheimer’s disease in 408 cognitively normal adults born in 1946. PLoS One 14:e0224030. doi: 10.1371/journal.pone.0224030
Perrotin, A., de Flores, R., Lamberton, F., Poisnel, G., La Joie, R., de la Sayette, V., et al. (2015). Hippocampal Subfield Volumetry and 3D Surface Mapping in Subjective Cognitive Decline. J. Alzheimers Dis. 48(Suppl. 1), S141–S150. doi: 10.3233/JAD-150087
Pietrzak, R. H., Lim, Y. Y., Ames, D., Harrington, K., Restrepo, C., Martins, R. N., et al. (2015). Trajectories of memory decline in preclinical Alzheimer’s disease: results from the Australian Imaging, Biomarkers and Lifestyle Flagship Study of ageing. Neurobiol. Aging 36, 1231–1238. doi: 10.1016/j.neurobiolaging.2014.12.015
Pini, L., Pievani, M., Bocchetta, M., Altomare, D., Bosco, P., Cavedo, E., et al. (2016). Brain atrophy in Alzheimer’s Disease and aging. Ageing Res. Rev. 30, 25–48. doi: 10.1016/j.arr.2016.01.002
Prestia, A., Caroli, A., Wade, S. K., van der Flier, W. M., Ossenkoppele, R., Van Berckel, B., et al. (2015). Prediction of AD dementia by biomarkers following the NIA-AA and IWG diagnostic criteria in MCI patients from three European memory clinics. Alzheimers Dement. 11, 1191–1201. doi: 10.1016/j.jalz.2014.12.001
Price, J. L., Ko, A. I., Wade, M. J., Tsou, S. K., McKeel, D. W., and Morris, J. C. (2001). Neuron number in the entorhinal cortex and CA1 in preclinical Alzheimer disease. Arch. Neurol. 58, 1395–1402. doi: 10.1001/archneur.58.9.1395
Rentz, D. M., Locascio, J. J., Becker, J. A., Moran, E. K., Eng, E., Buckner, R. L., et al. (2010). Cognition, reserve, and amyloid deposition in normal aging. Ann. Neurol. 67, 353–364. doi: 10.1002/ana.21904
Reuter, M., Schmansky, N. J., Rosas, H. D., and Fischl, B. (2012). Within-subject template estimation for unbiased longitudinal image analysis. Neuroimage 61, 1402–1418. doi: 10.1016/j.neuroimage.2012.02.084
Roberts, B. R., Lind, M., Wagen, A. Z., Rembach, A., Frugier, T., Li, Q. X., et al. (2017). Biochemically-defined pools of amyloid-beta in sporadic Alzheimer’s disease: correlation with amyloid PET. Brain 140, 1486–1498. doi: 10.1093/brain/awx057
Rodrigue, K. M., Kennedy, K. M., Devous, M. D. Sr., Rieck, J. R., Hebrank, A. C., Diaz-Arrastia, R., et al. (2012). beta-Amyloid burden in healthy aging: regional distribution and cognitive consequences. Neurology 78, 387–395. doi: 10.1212/WNL.0b013e318245d295
Rossler, M., Zarski, R., Bohl, J., and Ohm, T. G. (2002). Stage-dependent and sector-specific neuronal loss in hippocampus during Alzheimer’s disease. Acta Neuropathol. 103, 363–369. doi: 10.1007/s00401-001-0475-7
Rowe, C. C., Ng, S., Ackermann, U., Gong, S. J., Pike, K., Savage, G., et al. (2007). Imaging beta-amyloid burden in aging and dementia. Neurology 68, 1718–1725. doi: 10.1212/01.wnl.0000261919.22630.ea
Samann, P. G., Iglesias, J. E., Gutman, B., Grotegerd, D., Leenings, R., Flint, C., et al. (2020). FreeSurfer-based segmentation of hippocampal subfields: A review of methods and applications, with a novel quality control procedure for ENIGMA studies and other collaborative efforts. Hum. Brain Mapp. 2020:25326. doi: 10.1002/hbm.25326
Scheltens, P., Blennow, K., Breteler, M. M., de Strooper, B., Frisoni, G. B., Salloway, S., et al. (2016). Alzheimer’s disease. Lancet 388, 505–517. doi: 10.1016/S0140-6736(15)01124-1
Sevigny, J., Chiao, P., Bussiere, T., Weinreb, P. H., Williams, L., Maier, M., et al. (2016). The antibody aducanumab reduces Abeta plaques in Alzheimer’s disease. Nature 537, 50–56. doi: 10.1038/nature19323
Solbach, C., Uebele, M., Reischl, G., and Machulla, H. J. (2005). Efficient radiosynthesis of carbon-11 labelled uncharged Thioflavin T derivatives using [11C]methyl triflate for beta-amyloid imaging in Alzheimer’s Disease with PET. Appl. Radiat. Isot. 62, 591–595. doi: 10.1016/j.apradiso.2004.09.003
Sotaniemi, M., Pulliainen, V., Hokkanen, L., Pirttila, T., Hallikainen, I., Soininen, H., et al. (2012). CERAD-neuropsychological battery in screening mild Alzheimer’s disease. Acta Neurol. Scand. 125, 16–23. doi: 10.1111/j.1600-0404.2010.01459.x
Sperling, R. A., Aisen, P. S., Beckett, L. A., Bennett, D. A., Craft, S., Fagan, A. M., et al. (2011). Toward defining the preclinical stages of Alzheimer’s disease: recommendations from the National Institute on Aging-Alzheimer’s Association workgroups on diagnostic guidelines for Alzheimer’s disease. Alzheimers Dement. 7, 280–292. doi: 10.1016/j.jalz.2011.03.003
Steininger, S. C., Liu, X., Gietl, A., Wyss, M., Schreiner, S., Gruber, E., et al. (2014). Cortical Amyloid Beta in Cognitively Normal Elderly Adults is Associated with Decreased Network Efficiency within the Cerebro-Cerebellar System. Front. Aging Neurosci. 6:52. doi: 10.3389/fnagi.2014.00052
Svenningsson, A. L., Stomrud, E., Insel, P. S., Mattsson, N., Palmqvist, S., and Hansson, O. (2019). beta-amyloid pathology and hippocampal atrophy are independently associated with memory function in cognitively healthy elderly. Sci. Rep. 9:11180. doi: 10.1038/s41598-019-47638-y
Tang, X., Holland, D., Dale, A. M., Younes, L., and Miller, M. I. Alzheimer’s Disease et al. (2015). The diffeomorphometry of regional shape change rates and its relevance to cognitive deterioration in mild cognitive impairment and Alzheimer’s disease. Hum. Brain Mapp. 36, 2093–2117. doi: 10.1002/hbm.22758
Tierney, M. C., Yao, C., Kiss, A., and McDowell, I. (2005). Neuropsychological tests accurately predict incident Alzheimer disease after 5 and 10 years. Neurology 64, 1853–1859. doi: 10.1212/01.WNL.0000163773.21794.0B
Tomlinson, B. E., Blessed, G., and Roth, M. (1968). Observations on the brains of non-demented old people. J. Neurol. Sci. 7, 331–356. doi: 10.1016/0022-510x(68)90154-8
Tondelli, M., Wilcock, G. K., Nichelli, P., De Jager, C. A., Jenkinson, M., and Zamboni, G. (2012). Structural MRI changes detectable up to ten years before clinical Alzheimer’s disease. Neurobiol. Aging 33, e825–e836. doi: 10.1016/j.neurobiolaging.2011.05.018
van Bergen, J. M., Li, X., Hua, J., Schreiner, S. J., Steininger, S. C., Quevenco, F. C., et al. (2016). Colocalization of cerebral iron with Amyloid beta in Mild Cognitive Impairment. Sci. Rep. 6:35514. doi: 10.1038/srep35514
van Bergen, J. M. G., Li, X., Quevenco, F. C., Gietl, A. F., Treyer, V., Leh, S. E., et al. (2018). Low cortical iron and high entorhinal cortex volume promote cognitive functioning in the oldest-old. Neurobiol. Aging 64, 68–75. doi: 10.1016/j.neurobiolaging.2017.12.014
van Duijn, S., Bulk, M., van Duinen, S. G., Nabuurs, R. J. A., van Buchem, M. A., van der Weerd, L., et al. (2017). Cortical Iron Reflects Severity of Alzheimer’s Disease. J. Alzheimers Dis. 60, 1533–1545. doi: 10.3233/JAD-161143
Vandenberghe, R., Van Laere, K., Ivanoiu, A., Salmon, E., Bastin, C., Triau, E., et al. (2010). 18F-flutemetamol amyloid imaging in Alzheimer disease and mild cognitive impairment: a phase 2 trial. Ann. Neurol. 68, 319–329. doi: 10.1002/ana.22068
Vasta, R., Augimeri, A., Cerasa, A., Nigro, S., Gramigna, V., Nonnis, M., et al. (2016). Hippocampal Subfield Atrophies in Converted and Not-Converted Mild Cognitive Impairments Patients by a Markov Random Fields Algorithm. Curr. Alzheimer. Res. 13, 566–574. doi: 10.2174/1567205013666160120151457
Villemagne, V. L., Burnham, S., Bourgeat, P., Brown, B., Ellis, K. A., Salvado, O., et al. (2013). Amyloid beta deposition, neurodegeneration, and cognitive decline in sporadic Alzheimer’s disease: a prospective cohort study. Lancet Neurol. 12, 357–367. doi: 10.1016/S1474-4422(13)70044-9
Voineskos, A. N., Winterburn, J. L., Felsky, D., Pipitone, J., Rajji, T. K., Mulsant, B. H., et al. (2015). Hippocampal (subfield) volume and shape in relation to cognitive performance across the adult lifespan. Hum. Brain Mapp. 36, 3020–3037. doi: 10.1002/hbm.22825
Vos, S. J., Xiong, C., Visser, P. J., Jasielec, M. S., Hassenstab, J., Grant, E. A., et al. (2013). Preclinical Alzheimer’s disease and its outcome: a longitudinal cohort study. Lancet Neurol. 12, 957–965. doi: 10.1016/S1474-4422(13)70194-7
Wang, L., Miller, J. P., Gado, M. H., McKeel, D. W., Rothermich, M., Miller, M. I., et al. (2006). Abnormalities of hippocampal surface structure in very mild dementia of the Alzheimer type. Neuroimage 30, 52–60. doi: 10.1016/j.neuroimage.2005.09.017
West, M. J., Coleman, P. D., Flood, D. G., and Troncoso, J. C. (1994). Differences in the pattern of hippocampal neuronal loss in normal ageing and Alzheimer’s disease. Lancet 344, 769–772. doi: 10.1016/s0140-6736(94)92338-8
West, M. J., Kawas, C. H., Stewart, W. F., Rudow, G. L., and Troncoso, J. C. (2004). Hippocampal neurons in pre-clinical Alzheimer’s disease. Neurobiol. Aging 25, 1205–1212. doi: 10.1016/j.neurobiolaging.2003.12.005
Wisse, L. E., Biessels, G. J., and Geerlings, M. I. (2014a). A Critical Appraisal of the Hippocampal Subfield Segmentation Package in FreeSurfer. Front. Aging Neurosci. 6:261. doi: 10.3389/fnagi.2014.00261
Wisse, L. E., Biessels, G. J., Heringa, S. M., Kuijf, H. J., Koek, D. H., Luijten, P. R., et al. (2014b). Hippocampal subfield volumes at 7T in early Alzheimer’s disease and normal aging. Neurobiol. Aging 35, 2039–2045. doi: 10.1016/j.neurobiolaging.2014.02.021
Wisse, L. E. M., Chetelat, G., Daugherty, A. M., de Flores, R., la Joie, R., Mueller, S. G., et al. (2021). Hippocampal subfield volumetry from structural isotropic 1 mm(3) MRI scans: A note of caution. Hum. Brain Mapp. 42, 539–550. doi: 10.1002/hbm.25234
Wong, F. C. C., Yatawara, C., Low, A., Foo, H., Wong, B. Y. X., Lim, L., et al. (2020). Cerebral Small Vessel Disease Influences Hippocampal Subfield Atrophy in Mild Cognitive Impairment. Transl. Stroke Res. 2020:4. doi: 10.1007/s12975-020-00847-4
World_Medical_Association. (1991). “Declaration of Helsinki,” in Law Med Health Care (Ferney-Voltaire: World_Medical_Association).
Yassi, N., Hilal, S., Xia, Y., Lim, Y. Y., Watson, R., Kuijf, H., et al. (2019). Influence of Comorbidity of Cerebrovascular Disease and Amyloid-beta on Alzheimer’s Disease. J. Alzheimers Dis. 2019:191028. doi: 10.3233/JAD-191028
Ye, B. S., Seo, S. W., Kim, C. H., Jeon, S., Kim, G. H., Noh, Y., et al. (2014). Hippocampal and cortical atrophy in amyloid-negative mild cognitive impairments: comparison with amyloid-positive mild cognitive impairment. Neurobiol. Aging 35, 291–300. doi: 10.1016/j.neurobiolaging.2013.08.017
Zeineh, M. M., Chen, Y., Kitzler, H. H., Hammond, R., Vogel, H., and Rutt, B. K. (2015). Activated iron-containing microglia in the human hippocampus identified by magnetic resonance imaging in Alzheimer disease. Neurobiol. Aging 36, 2483–2500. doi: 10.1016/j.neurobiolaging.2015.05.022
Zhang, L., Mak, E., Reilhac, A., Shim, H. Y., Ng, K. K., Ong, M. Q. W., et al. (2020). Longitudinal trajectory of Amyloid-related hippocampal subfield atrophy in nondemented elderly. Hum. Brain Mapp. 41, 2037–2047. doi: 10.1002/hbm.24928
Zhao, W., Wang, X., Yin, C., He, M., Li, S., and Han, Y. (2019). Trajectories of the Hippocampal Subfields Atrophy in the Alzheimer’s Disease: A Structural Imaging Study. Front. Neuroinform. 13:13. doi: 10.3389/fninf.2019.00013
Zheng, F., Cui, D., Zhang, L., Zhang, S., Zhao, Y., Liu, X., et al. (2018). The Volume of Hippocampal Subfields in Relation to Decline of Memory Recall Across the Adult Lifespan. Front. Aging Neurosci. 10:320. doi: 10.3389/fnagi.2018.00320
Keywords: ultra-high field MRI, 7 Tesla, hippocampus subfield segmentation, prodromal AD, episodic memory, iron, subiculum, Alzheimer’s disease
Citation: Kagerer SM, Schroeder C, van Bergen JMG, Schreiner SJ, Meyer R, Steininger SC, Vionnet L, Gietl AF, Treyer V, Buck A, Pruessmann KP, Hock C and Unschuld PG (2022) Low Subicular Volume as an Indicator of Dementia-Risk Susceptibility in Old Age. Front. Aging Neurosci. 14:811146. doi: 10.3389/fnagi.2022.811146
Received: 08 November 2021; Accepted: 31 January 2022;
Published: 03 March 2022.
Edited by:
Stephen D. Ginsberg, Nathan Kline Institute for Psychiatric Research, United StatesReviewed by:
Emilio Artacho-Perula, University of Castilla-La Mancha, SpainCopyright © 2022 Kagerer, Schroeder, van Bergen, Schreiner, Meyer, Steininger, Vionnet, Gietl, Treyer, Buck, Pruessmann, Hock and Unschuld. This is an open-access article distributed under the terms of the Creative Commons Attribution License (CC BY). The use, distribution or reproduction in other forums is permitted, provided the original author(s) and the copyright owner(s) are credited and that the original publication in this journal is cited, in accordance with accepted academic practice. No use, distribution or reproduction is permitted which does not comply with these terms.
*Correspondence: Paul G. Unschuld, cGF1bC51bnNjaHVsZEBoY3VnZS5jaA==
†These authors have contributed equally to this work and share first authorship
Disclaimer: All claims expressed in this article are solely those of the authors and do not necessarily represent those of their affiliated organizations, or those of the publisher, the editors and the reviewers. Any product that may be evaluated in this article or claim that may be made by its manufacturer is not guaranteed or endorsed by the publisher.
Research integrity at Frontiers
Learn more about the work of our research integrity team to safeguard the quality of each article we publish.