- Aging Institute, School of Medicine, University of Pittsburgh, Pittsburgh, PA, United States
Continually emerging data indicate that sub-clinical, non-convulsive epileptiform activity is not only prevalent in Alzheimer’s disease (AD) but is detectable early in the course of the disease and predicts cognitive decline in both humans and animal models. Epileptiform activity and other electroencephalographic (EEG) measures may hold powerful, untapped potential to improve the translational validity of AD-related biomarkers in model animals ranging from mice, to rats, and non-human primates. In this review, we will focus on studies of epileptiform activity, EEG slowing, and theta-gamma coupling in preclinical models, with particular focus on its role in cognitive decline and relevance to AD. Here, each biomarker is described in the context of the contemporary literature and recent findings in AD relevant animal models are discussed.
Introduction
Alzheimer’s disease (AD) is the foremost cause of dementia (NIA Alzheimer’s Disease Fact Sheet, 2020) and the fifth leading cause of death worldwide. Over 6 million people 65 or older currently live with AD (WHO, 2020). As global life expectancy continues to increase this number of patients is expected to double every 20 years and reach 131.5 million by 2050 (Alzheimers.net, 2020). The global cost of dementia is expected to exceed $2 trillion United States by 2030, topping the gross domestic product of all but 17 countries and the total market values of Apple, Google, and Exxon. At this rate, AD will place increasingly severe burdens on global healthcare markets over the next 30 years. People living with dementia also require caregiver assistance, especially in the later stages of the disease. Frequently, these caregivers are close relatives (World Alzheimer Report, 2015). Global cost estimates will never fully capture the physical and emotional toll imposed on these individuals who love and care for someone suffering from AD.
It is now over 115 years since the first clinical report describing AD in 1906 (Alzheimer, 1906), but the first purported “disease-modifying” treatment for AD, Aduhelm was only recently approved in June 2021 (Yang and Sun, 2021). Time will tell whether this new treatment can make an impact. To date, the search for a cure has largely focused on clearance and prevention of histopathological aggregates such as amyloid plaques (Haass and Selkoe, 2007; Mucke and Selkoe, 2012; Selkoe and Hardy, 2016) and neurofibrillary inclusions. More recently, investigation has broadened to strategies for minimizing chronic inflammation, mediating neurovascular risk-factors (NIA, What Happens to the Brain in Alzheimer’s Disease, 2020), vaccination (Novak et al., 2017; Kabir et al., 2020), and development of exogenous antibodies (Sevigny et al., 2016; Uhlmann et al., 2020). These interventions continue to fall short of meeting the most important endpoint of AD, which is improvement or prevention of cognitive decline in AD. In conjunction with symptom modifying drugs such as acetylcholinesterase inhibitors or low-affinity NMDA blockers such as memantine (Galimberti and Scarpini, 2011), prevention and early intervention remain the most viable current treatment strategies.
Early detection and treatment at the very earliest stages of amyloid seeding may hold critical potential for the treatment of AD (Uhlmann et al., 2020). Owing to its widespread accessibility, data-rich non-invasive methods, and low cost relative to expensive diagnostic neuroimaging, electroencephalography (EEG) is a promising approach for detection and development of early biomarkers related to neurological dysfunction resulting from AD. In particular, seizure and sub-clinical epileptiform activity related to AD is readily identifiable with EEG (Horváth et al., 2016; Palop and Mucke, 2016; Lam et al., 2017; Horvath, 2018; Smailovic et al., 2018). Recent reports indicate that sub-clinical, non-convulsive epileptiform activity is not only prevalent in AD (Palop and Mucke, 2016; Vossel et al., 2016, 2017; Horváth et al., 2017; Lam et al., 2017), but is detectable early in the course of the disease (Vossel et al., 2013), and predicts cognitive decline (Vossel et al., 2016). Given that preclinical translational studies focusing on behavioral outcomes for cognition have repeatedly failed to translate to cognitive benefits in humans, there is an urgent need to develop improved translational approaches. To this end, advances in measuring epileptiform activity and other EEG biomarkers may hold powerful, untapped potential to improve the translational validity of AD-related biomarkers in model animals and enable improved preclinical to clinical translation for understanding cognitive deficits and potential therapeutic interventions for AD.
Mechanisms Underlying Abnormal Epileptiform Activity
Epileptiform activity is a broad term to describe sporadic discharges or trains of spiking activity resulting from highly synchronous oscillatory activity in the brain. The activity captured in a surface EEG is largely generated from reciprocal excitatory-inhibitory connections throughout thalamocortical circuits and is not necessarily equated to classical full body seizure type events; although severe events of this nature may result in such. In short, hyperactivity in layer VI pyramidal cells triggers an inhibitory response from GABAergic neurons within the thalamic reticular nucleus. During this inhibitory response, low-threshold calcium channels in thalamocortical neurons recover from initial inactivation. Consequently, as thalamocortical neurons recover from inhibitory post-synaptic currents generated by the thalamic reticular nucleus, this available pool of calcium channels trigger another burst of excitatory activity. This cycle produces reverberating oscillatory activity detectable as spike trains in EEG (Blumenfeld, 2005). Perturbations in this excitatory-inhibitory (E-I) balance, particularly within cortico-hippocampal circuitry could arise from innumerable gene mutations or physical and environmental insults in the polygenetic or degenerative patterns in late onset AD (LOAD). In LOAD, constellations of genetic mutations converge to produce synapse deterioration and loss, amyloidosis, neurotoxicity, and neuroinflammation that can all contribute to shifting the intrinsic E-I balance.
Current theories hypothesize that progressive age-related decline of synaptic function in the earliest stages of AD alters firing properties of neurons within broad networks mediating genome, immune, and metabolic homeostasis, among other functions. Dysregulation within these networks feeds forward resulting in loss of function and cognitive decline as in AD (Frere and Slutsky, 2018). This understanding of homeostatic dysfunction resulting from age-related degradation of synaptic function across broad regulatory networks captures the diversity of genetic and environmental factors at play in sporadic AD, while also speaking to mechanisms of autosomal dominant familial AD (FAD) mutations.
Incidence and Prevalence
Despite broad acceptance of its occurrence, accurate coincidence of abnormal epileptiform activity in AD has proven difficult to estimate. Given that these neural perturbations are often subclinical and not presented with any overt motoric type of seizure events, awareness of seizure activity may be limited. Indeed, previous reviews have reported incidence ranging from 0.5 to 64% (Horváth et al., 2016; Palop and Mucke, 2016). However, two separate studies recently reported incidence rates of 13.4 and 14.0% in LOAD patients (Beagle et al., 2017; Lyou et al., 2018). This broad range may result from the diversity of seizure types assessed in each study. Seizure recurrence is frequent, increases with disease duration, and is associated with poorer cognitive performance (Vöglein et al., 2020). While convulsive, tonic-clonic seizures which are easily detected by overt motor phenomena has largely comprised a bulk of the literature related to reports of seizures in AD to date (Horváth et al., 2016). By comparison, a recent study reported 42.4% of patients meeting the National Institute of Aging – Alzheimer’s Association criteria for probable AD (McKhann et al., 2011) demonstrated epileptiform activity. Interestingly, all patients in this study also showed evidence of cortical atrophy assessed using voxel-based morphometry with amyloidosis confirmed in 61% of these patients by PET/MR. Of those not exhibiting positive amyloid PET, several tested positive for CSF biomarkers Aβ42, t-Tau, p-Tau, or Aβ42-Tau index. Interestingly, at the time of initial EEG assessment patients presenting with epileptiform activity did not differ in global cognitive ability assessed by the Mini-Mental State Examination. Longitudinal monitoring of these patients over the course of 1–5 years revealed a more precipitous decline in global cognition and executive function (Vossel et al., 2016). These data therefore implicate epileptiform activity as an early indicator of AD pathology and future cognitive decline. Separately, another study comparing MCI and AD patients with epilepsy to AD patients with subclinical epileptiform activity reported that the latter group began experiencing cognitive decline 5.5–6 years sooner (Vossel et al., 2013). Implanted electrodes in two patients targeting the medial temporal lobe detected hyperexcitability in the region of the hippocampus that was not readily detectable on scalp electrodes. This activity was especially prominent during sleep (Lam et al., 2017). Continuous EEG monitoring over a 24 h period in 5 AD patients with epilepsy, revealed 82% of epileptiform discharges occurred during sleep, with 55% occurring during non-rapid eye movement (NREM) sleep (Horváth et al., 2017). Interference with memory consolidation that takes place during this period likely contributes to continued cognitive decline in AD patients. These finding are not without challenges, however, as analysis of seizure activity using more strictly defined parameters has failed to detect differences in subclinical seizure incidence between cases and controls (Brunetti et al., 2020). Further study should seek to define specific criteria for subclinical epileptiform activity that drives differences between cases and controls. Taken together, these clinical data indicate that epileptiform activity may indeed serve as an early biomarker for cognitive decline and early pathological events in AD.
Translational Electroencephalographic Biomarkers for Preclinical Investigation in Animal Models
Recent literature highlights the utility of EEG studies in animal models as demonstrating translational potential for AD (Born, 2015; Horváth et al., 2016; Palop and Mucke, 2016; Vossel et al., 2017). More specifically, four distinct phenotypes have emerged that include (1) ectopic cortical spiking activity, especially during NREM sleep, absent overt physical symptoms of acute seizure, (2) hippocampal slowing evidenced by leftward shifts in the power distribution of EEG oscillatory activity at baseline, (3) hyperexcitability of hippocampal neurons, particularly in response to cortical inputs, and (4) impaired theta-gamma coupling. In patient populations, this activity is not only highly predictive of disease course (Vöglein et al., 2020), but may precede the earliest cognitive symptoms. Here we review these outcome measures, identifying reports from relevant animal models expressing these phenotypes and their translational utility for AD. While previous publications have discussed epileptiform and other abnormalities in EEG activity in AD animal models generally focusing on FAD alleles which can be comprehensively reviewed elsewhere (Born, 2015; Horváth et al., 2016; Palop and Mucke, 2016; Vossel et al., 2017; Kitchigina, 2018), the present review provides a contemporary update that highlights subsequent and more recent findings in these and newer mouse models with a focus on distinct phenotypes of EEG outcome measures.
In animal models, epileptiform activity is used broadly to refer to ectopic discharges that may present with (interictal/seizure) or without (subclinical) motor features. Applications of EEG in animal models generally consists of the identification of spike wave discharges (SWD) or single spikes predominantly during NREM. SWDs are typically characterized by surface-negative spikes alternating with 7–10 Hz surface positive waves (Jin et al., 2021). Single spikes, as their name would imply are sharp deflections in the EEG trace that do not cluster with other spikes as in an SWD. This type of spike has no clear definition, owing to the complexity and heterogeneity of this type of activity. These spikes are generally quantified as events crossing a predefined threshold relative to baseline activity of the EEG trace. These criteria vary widely, but general guidelines have recently been developed for mice which will greatly facilitate improved rigor and reproducibility going forward (Jin et al., 2021).
Electroencephalographic slowing is a key biomarker in AD and AD related dementias (ADRD) (Jeong, 2004; Horvath, 2018). In the EEG trace, EEG slowing generally manifests as a reduction in the distribution of alpha (8–12 Hz) and beta (12–30 Hz) waves and a commensurate increase in the distribution of delta (0.5–4 Hz) and theta (4–8 Hz) waves (Brenner et al., 1986; Jeong, 2004). Patients with MCI exhibit increases in theta activity and a decrease in beta activity. Severe dementia, on the other hand, is related to decreases in alpha and an increase in delta frequency activity (Coben et al., 1985). Lower overall mean frequency is also well correlated to dementia severity (Brenner et al., 1986). EEG slowing is likely attributable to degeneration of acetylcholine neurons during the course of AD (Bartus et al., 1982). This is supported by multiple clinical and preclinical studies using the muscarinic antagonist scopolamine (Ebert and Kirch, 1998). In rats administration of scopolamine increases the power in the delta and theta range (Buzsáki et al., 1988). In healthy human subjects scopolamine administration increased delta and theta activity while decreasing alpha and beta power (Ebert et al., 2001). Further, scopolamine is well known for inducing memory impairment in healthy subjects, akin to impairment measured in MCI (Ebert and Kirch, 1998).
Hyperexcitability of hippocampal neurons has been used in animal models as a surrogate to hippocampal hyperactivation observed in AD patients during fMRI (Zott et al., 2018). In an fMRI, memory tasks increase hippocampal activation with hyperactivity reported in AD patients reported prior to and throughout initial impairments in cognitive performance and mild memory loss (Dickerson et al., 2005; Huijbers et al., 2015). Later in the progression of AD as cognitive impairment becomes more severe, hippocampal activity is diminished (O’Brien et al., 2010). This pattern may suggest an initial overcompensation by the hippocampus followed by a loss of activation later in the course of AD progression (Sperling et al., 2010).
Theta-gamma coupling (TGC) is another electrophysiological biomarker with emerging relevance to AD (Goodman et al., 2018; Kitchigina, 2018). TGC refers to the modulation of gamma oscillations (30–100 Hz) by slower theta oscillations (4–7 Hz) This relationship was first characterized in the study of place cells in the hippocampus. Specifically, place cells were shown to fire at different theta phases according to a specific temporal pattern coinciding with sequential spatial position on a walking track. Therefore, theta activity encodes information along a temporal sequence, termed temporal sweeps. Gamma oscillations “nested” within each temporal sweep are thought to represent units of events in order. In other words, theta represents the time interval during which items of information encoded by gamma are held in memory. The extent which gamma varies as a function of theta is quantified as TGC (Lisman and Buzsáki, 2008; Lisman and Jensen, 2013). Breakdowns in this encoding would lead to degradation of representations of events in order. Indeed, this type of cross-frequency coupling is important for both long-term (Tort et al., 2009) and working memory (Lisman, 2010). Patients with AD show significantly lower TGC compared to healthy controls, which is associated with impaired performance on an N-back task (Goodman et al., 2018).
Epileptiform Activity in Alzheimer’s Disease-Relevant Mouse Models
Much of the preclinical AD research to date has utilized mouse models expressing FAD mutations, specifically amyloid precursor protein (APP) and presenilin 1 (PS1). Despite the causal mechanisms of these mutations in early-onset AD, FAD only comprises between 1 and 5.5% of all AD cases (Sassi et al., 2014; Zhu et al., 2015). Moreover, severe neuropathology and behavioral phenotypes are detectable in young FAD mice, a result of transgenic overexpression that is well beyond physiologically relevant changes (Oddo et al., 2003; Richard et al., 2015) limiting translational value for the majority of AD cases who do not experience rapid decline in early adulthood. New approaches seek to incorporate LOAD risk-factor genes in tandem with FAD mutations, other risk alleles, and environmental variables to begin understand the complex age-dependent etiology of AD that has remained elusive to date (Onos et al., 2016; Castillo et al., 2017; Pandey et al., 2019; Preuss et al., 2019; Oblak et al., 2020). Understanding how these newly emerging risk-factors play a role in the induction of epileptiform activity and network dysfunction could provide key insights to earlier sub-clinical manifestations of LOAD.
Amyloid precursor protein is a transmembrane protein with yet unknown endogenous functions, though it has been implicated in a broad array of roles such as synapse formation (Priller et al., 2006), plasticity (Montagna et al., 2017), and metallic ion chelation (Cuajungco et al., 2005). Proteolysis of APP is accomplished through two pathways. Initial cleavage is executed by α- or β-secretase. Cleavage by β-secretase, also known as Beta APP Cleaving Enzyme 1 (BACE1) produces β-amyloid (Aβ) fragments after final cleavage by γ-secretase. These fragments are the primary component of amyloid plaques in AD (Nunan and Small, 2000). Many FAD mutations of APP have been characterized, which has led to the generation of equally diverse APP mouse models.
One of the earliest APP mouse models is the Tg2576, overexpresses a mutant form of APP with the Swedish (APP KN670/671NL) mutation resulting in significant and early onset amyloid pathology. This is a double mutation directly adjacent to the β-cleavage site of APP, which facilitates β-secretase cleavage. Owing to its name, the Swedish mutation was first characterized in two Swedish families with a high degree of genealogical overlap. Affected members of these families experienced progressive memory loss consistent with AD (Mullan et al., 1992). Late in the disease course, myoclonic jerks and seizures are frequent in these families (Axelman et al., 1994). Aβ plaque aggregation is present in aged Tg2576 mice (>11 months), though diminished LTP (Jacobsen et al., 2006) and synapse loss (Lanz et al., 2003) has been described in 4–5 months old mice before plaques are detected (Table 1). Cognitive impairments in spatial learning and fear conditioning were initially thought to be progressive, only seen in 12 months or older mice (Hsiao et al., 1996). Though subsequent reports have describe behavioral deficits as early as 6 months (Jacobsen et al., 2006). Recent publications have characterized large, synchronous spikes consistent with interictal spikes seen in epilepsy. These spikes are present in 5 weeks old mice, long before any of the pathology discussed. These spikes progressed into adulthood (7 months) and were predominantly detected during REM sleep (Kam et al., 2016). Though it is unclear if the exogenous transgene hamster prion protein promotor itself be disrupting neuronal signaling and contributing to the epileptiform activity in these animals. It should be noted that Tg2576 mice are maintained on a hybrid C57BL6/SJL background. Lower seizure thresholds have been reported for the SJL mouse (Golden et al., 2001) and depending on C57 or SJL substrain used, this may contribute to SWD phenotype as well (Letts et al., 2014) which underscores the need for specific documentation of background strain given the heterogeneity of seizure phenotypes across AD transgenic lines and mouse strains and substrains (Schauwecker, 2011; Letts et al., 2014; Copping et al., 2019).
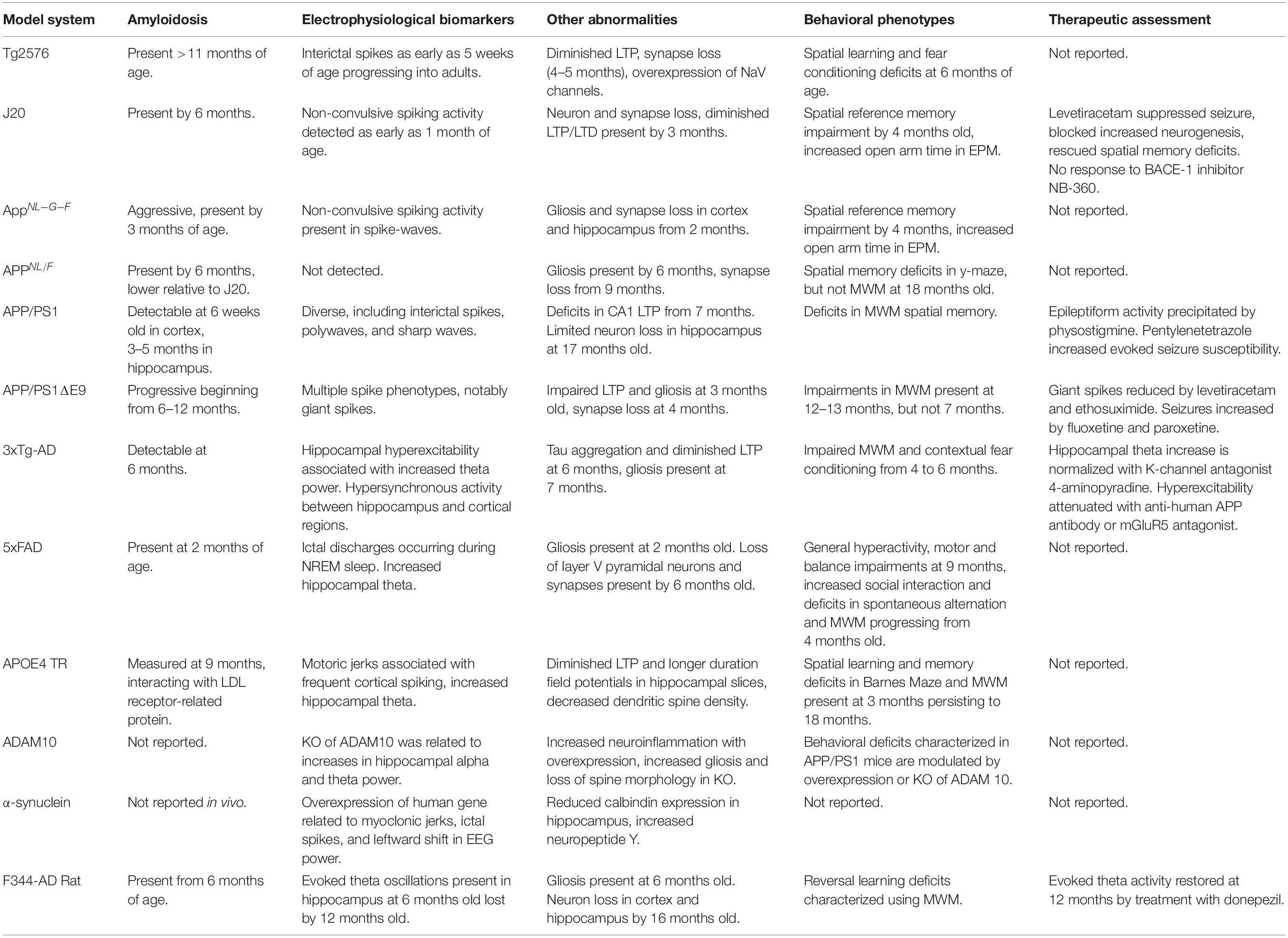
Table 1. Summary of rodent models, Alzheimer’s disease relevant pathology and reported behavioral and EEG phenotypes.
A recent paper by Johnson et al. (2020) directly compared epileptiform activity across three APP mouse models. hAPP-I5 (I5) mice carry a transgene overexpressing human neuronal APP (hAPP). This mouse serves as a comparison strain for FAD-mutant hAPP mice (J20), which overexpress APP with Swedish (KN670/671NL) and Indiana (V717F) mutations (Mucke et al., 2000). The third strain, AppNL–G–F, does not overexpress APP, but instead express fully humanized Aβ fragments from cleavage of Swedish (KM670/671NL), Arctic (E693G), and Beyreuther/Iberian (I716F) mutant APP (Saito et al., 2014). As expected, I5 and J20, but not APPNL–G–F, overexpress APP in cortex and hippocampus at 6–8 months of age. Though, compared to I5 and J20 strains, APPNL–G–F express more Aβ overall with a higher ratio of Aβ1–42 in hippocampus and cortex. EEG recordings in all three of these mouse models at 8–12 months of age revealed non-convulsive epileptiform activity compared to their WT littermates. J20 mice expressed the most spikes over the entire 12 h recording, while the APPNL–G–F mice experienced the highest number of spike wave discharges. Behaviorally, all three strains displayed deficits in active place avoidance. However, given that J20 and APPNL–G–F mice also spent more time in the open arms of an elevated plus maze (EPM) indicative of an anxiolytic-like phenotype, it may be difficult to distinguish between spatial memory deficits in an avoidance paradigm or an overall loss of inhibition/aversion to risk in these mice. Subsequent experiments showed that J20 mice do exhibit spatial memory deficits in the Morris Water Maze (MWM) (Johnson et al., 2020), although the MWM phenotype in APPNL–G–F mice has been inconsistent (Mehla et al., 2019; Saifullah et al., 2020). However, deficits in location discrimination, in the absence of MWM deficits have been detailed (Saifullah et al., 2020). Spatial memory deficits (Cheng et al., 2007; Wright et al., 2013), neuron loss (Wright et al., 2013), and LTP deficits (Saganich et al., 2006) have been reported in J20 mice as early as 4 months of age. Thus, it remains to be determined if epileptiform activity in J20 mice is a cause or effect of the early pathology seen in this strain, or if promoter driven transgene expression may be playing a role on its own. Notably, treatment with the BACE1 inhibitor NB-360 did not modify any of the behavioral or synaptic abnormalities measured in the J20 mice despite lowering levels of Aβ (Johnson et al., 2020). This particular result is notable as BACE1 inhibitors have failed to translate to improved cognition in the clinic, despite AB lowering (Mullard, 2018). Overall, these results indicate that APP overexpression is not necessary for inducing non-convulsive epileptiform activity or behavioral abnormalities induced by FAD mutations (Johnson et al., 2020). In a separate study comparing J20 to APPNL/F mice that carry the Swedish and Iberian APP mutations (Saito et al., 2014; Brown et al., 2018), epileptiform activity in the form of inter-ictal spikes was identified in J20 mice, especially during REM sleep. These events were not detected in APPNL/F mice. Interestingly, APPNL/F mice express more physiologically relevant levels of Aβ compared to overexpression in J20 and APPNL–G–F strains, which may diminish their seizure susceptibility although it remains unclear any direct correlations of Aβ levels with seizure susceptibility in humans or mouse models. Importantly, these findings during REM sleep in APP models contradict the predominance of epileptiform activity in NREM sleep in humans, suggesting key differences in seizure pathology across species not yet understood (Kam et al., 2016; Brown et al., 2018). Additional recent studies have begun to shed light on seizure-related pathogenesis in J20 mice. Increased spike wave discharges and increased latency of field responses in reticular thalamus following stimulation in deep cortical layers have been characterized from 4 to 6 months (Hazra et al., 2016). Reports that amyloid deposition leads to large increases of A2a expression on astrocytes suggests that in these J20 mice, astrocytic adenosine A2a receptors may play a role in AD like pathology. The A2a blocker istradefylline remediated MWM deficits following 1–3 weeks treatment administered in drinking water (Orr et al., 2018). Activation of A2a is proconvulsant and decreases seizure thresholds in kindling procedures (Tescarollo et al., 2020). These preclinical data open interesting avenues for studying the relationship of A2a receptors related to spontaneous seizure incidence in AD.
Over 70 mutations of the presenilin (PSEN) gene have been characterized (Jankowsky et al., 2004), accounting for roughly 90% of all FAD cases (Watanabe and Shen, 2017). The PS genes, PS1 and PS2, encode the catalytic subunit of γ-secretase, the final enzyme involved in APP proteolysis. When APP is initially cleaved by β-secretase, final cleavage by γ-secretase produces amyloid fragments typically between 38 and 42 residues in length, with Aβ40 comprising the bulk of amyloid fragments produced by APP proteolysis through the β-secretase pathway. Mutations in PSEN can result in greater production of long amyloid fragments, particularly Aβ42 that has a higher liability for deposition and, therefore produces more amyloid plaques compared to shorter fragments. This greater plaque burden is key in the pathogenesis of PSEN related FAD (Chow et al., 2010).
The APP/PS1 mouse combines a transgene carrying both hAPP and PSEN mutations, though the specific mutations may vary per each strain. Mice expressing APP Swedish and PSEN L166P mutations are most common. These mice express the APP transgene at threefold higher levels compared to murine APP and human Aβ42 is expressed in higher concentrations over Aβ40. Amyloid deposition is detectable in 6 weeks old mice, beginning in the neocortex. Amyloid deposits in the hippocampus, striatum, thalamus and brainstem appear in young adult mice 3–5 months old, though tau inclusions have not been identified in this strain (Radde et al., 2006; Maia et al., 2013). Deficits in spatial reversal learning, MWM spatial memory, and CA1 LTP have been observed as early as 7 months of age (Radde et al., 2006; Serneels et al., 2009; Gengler et al., 2010). Neuron loss is minimal in APP/PS1 mice and is generally constrained to the dentate gyrus as measured in 17-month old mice (Rupp et al., 2011). Spontaneous epileptiform discharges including interictal spikes, polywaves, and sharp waves have been described in 4–9 months old APP/PS1 mice, which were precipitated by the acetylcholinesterase inhibitor physostigmine (Reyes-Marin and Nuñez, 2017). These mice also have a greater susceptibility to seizures evoked using the pro-convulsant pentylenetetrazole, which was strongly correlated to the number of Aβ plaques measured in the cortex (Reyes-Marin and Nuñez, 2017).
Another study comparing mice carrying a deletion of PSEN1 exon 9 (APP/PS1ΔE9) also reported a seizure phenotype exhibiting multiple forms of spontaneous epileptiform events. This is particularly interesting because indeed patients with the PSEN1 exon 9 mutation also demonstrate seizure activity (Cortini et al., 2018). Utilizing electrodes implanted in cortical and subcortical structures, both strain dependency and structural dynamics of 8 specific epileptiform events could be identified. Three types of cortical spikes were identified. Cortical spikes were recorded when a discharge was detected across only cortical electrodes. These cortical spikes could co-occur with muscle twitches in the neck electromyogram and/or hippocampal discharges. Cortical spiking activity was also detected in sleep-spindles as well as higher frequency, low amplitude spindles (fast spindles). Spike wave discharges and cortico-hippocampal spikes with after-hyperpolarization were also characterized. The eighth type of discharge, termed a “giant spike” by the authors, was characterized using four criteria: (1) detection across all recording channels, (2) presence of spike complexes across multiple channels, (3) voltage changes ≥10 mV in hippocampal channel, and (4) a long after-hyperpolarization ≥10 ms. These giant spikes only occurred during sleep. APP/PS1ΔE9 mice experienced more cortical spikes either with or without hippocampal spikes, fast-spindles, cortico-hippocampal spikes, and giant spikes. In each case, these events were suppressed by treatment with the anticonvulsant levetiracetam or ethosuximide, a drug developed to suppress absence seizures. Notably, it was the presence of giant spikes that predicted seizure incidence in APP/PS1ΔE9 mice, demonstrating high utility of pairing cortical EEG with depth recording in hippocampus (Gureviciene et al., 2019). This is a particularly noteworthy translational phenotype given recent advances of foramen ovale recordings in distinguishing silent hippocampal seizures in AD (Lam et al., 2017).
Interestingly, a similar strain carrying the APP Arctic mutation (E693G) with the dE9 deletion have a comparatively mild seizure phenotype compared to the Swedish (APP KN670/671NL) mutation of APP. This mutation of APP also does not produce amyloidosis (Saito et al., 2014). This would suggest that seizure related activity is closely linked to the overproduction of amyloid, as in Swedish (APP KN670/671NL), whereas the APP Arctic mutation (E693G) may be more closely tied in with the pro-inflammatory phenotypes in AD.
Two separate papers have recently described a startling phenomenon in APP/PS1ΔE9 mice regarding the use of selective serotonin reuptake inhibitors (SSRIs) and the induction of AD-related seizure activity. Treatment with fluoxetine (Sierksma et al., 2016) and paroxetine (Severino et al., 2018) both led to increased age-related seizure incidence and premature death in these mice compared to their untreated controls. This effect is believed to arise from the anticholinergic properties of these drugs. Modern SSRIs seek to minimize their anticholinergic properties, which are associated with the most poorly tolerated side effects of modern anti-depressants, such as sedation, dry mouth, constipation, loss of libido and sexual dysfunction (Ferguson, 2001). Exposure to antidepressant, urological, or antiparkinsonian drugs with anticholinergic effects has been shown to induce anticholinergic cognitive burden that was related to increased incidence of dementia. This is particularly concerning considering the renewed interest in the anti-cholinergic mechanisms of rapid-acting antidepressants such as scopolamine (Drevets et al., 2013) and the increasing rates of antidepressant use over the last 20 years (Pratt et al., 2017). These data in mouse models provide further support for the use of EEG to identify potential adverse properties of therapeutic interventions.
The 5xFAD mouse has rapidly gained widespread use in the study of AD. These mice express 5 human transgenes linked to familial AD: three APP mutations [Swedish (K670N/M671L), Florida (I716V) and London (V717I) and two PSEN1 mutations (M146L and L286V)]. AD-relevant pathology in these mice progresses aggressively. Amyloid plaques and gliosis have been characterized in as early as 2 months old mice (Oakley et al., 2006). At 6 months, these mice are already experiencing neuron loss around areas with the highest plaque burden (Devi and Ohno, 2010). Despite prominent neuropathology, behavioral abnormalities have been inconsistent (Oblak et al., 2021). The most robust phenotypes to emerge are motor (hyperactivity) and balance impairments at 9 months (O’Leary et al., 2020), aggressive social interaction (Flanigan et al., 2014), spatial working memory deficits in spontaneous alternation at 4–5 months (Oakley et al., 2006; Devi and Ohno, 2010), and MWM deficits progressively declining from 6 months (Flanigan et al., 2014; Xiao et al., 2015; O’Leary et al., 2020). Though it is unclear how much of these motor and cognitive dysfunctions may be attributable to a general hyperactive behavioral phenotype (Flanigan et al., 2014; Lee et al., 2019; O’Leary et al., 2020). Neurophysiology impairments include degeneration of CA1 LTP (Kimura and Ohno, 2009) and loss of synaptic transmission in layer V cortex beginning at 6 months (Crouzin et al., 2013).
Siwek et al. (2015) characterized AD-relevant epileptiform activity, concluding that 18 months old 5XFAD mice exhibit ictal discharges in surface cortical EEG. However, EMG recordings were not included in this publication, making it difficult to distinguish these discharges from epochs of normal NREM sleep using the identification parameters reported (Siwek et al., 2015). As discussed, seizure activity in NREM is in fact typical in patients with AD (Horváth et al., 2016, 2017; Horvath, 2018). So while it is likely ictal discharges would occur in NREM, further characterization of sleep staging would help distinguish these events from normal sleep. Further, these mice were on a C57BL6/SJL background, which, as has been discussed previously, may alter seizure susceptibility (Golden et al., 2001; Letts et al., 2014). More recently, a rigorous comprehensive phenotypic characterization of 5XFAD on a congenic C57BL/6J background strain revealed a lack of robust EEG phenotypes in 5XFAD mice relevant to age and sex matched controls despite significant amyloid deposition in this model (Oblak et al., 2021). Single spikes have been identified as early as 4 months of age in 5xFAD mice. Interestingly in this paper it was found that spiking activity was exacerbated in an aquaporin KO 5xFAD mouse (Abe et al., 2020).
The ε4 allele of apolipoprotein E protein (APOE) is the strongest known risk factor for the development of non-familial LOAD. Three allelic variants of APOE are common in humans: ε2, ε3, and ε4. APOE is synthesized in the liver and the brain and mainly functions in the transport and homeostasis of cholesterol and other lipids throughout the body. With particular relevance to AD, APOE binds Aβ in the extracellular space and plays a role in clearing Aβ from the CNS through the blood–brain barrier (BBB). Of the three APOE isoforms, ApoE4 is poorest at binding Aβ and clearing it across the BBB; while APOE2 is the most effective. APOE2 is also known to be protective against AD. Thus, risk for LOAD is dose dependent on the APOE4 allele (APOE4/APOE4 > APOE3/APOE4 > APOE2/APOE4) (Kim et al., 2009; Holtzman et al., 2012; Safieh et al., 2019). APOE4 carriers have a higher risk for temporal lobe epilepsy (Najm et al., 2019), though it’s links to seizure in AD are still unclear.
An age-dependent seizure phenotype has been described in aged mice with a targeted replacement of the endogenous murine APOE allele with the humanized APOE4 allele (APOE4 TR). Female APOE4 TR mice were the first to develop a seizure phenotype of motoric jerks without arrest or clonus at six months of age. This same phenotype was present in males, albeit emerging later at 9 months. Cortical EEG reveled frequent spiking in the cortex of the APOE4 TR mice (Rodriguez et al., 2013).
Triggering receptor expressed on myeloid cells 2 (TREM2) is a frequent mutation related to AD. TREM2 is a transmembrane receptor involved in the immune and compliment systems. AD related mutations in TREM2 result in loss of function and are thought to mediate immunogenicity and inflammation that is enhanced in AD (Carmona et al., 2018). Myoclonus and seizures have been reported in a small cohort of carriers of the common TREM2 mutation R47H (Luis et al., 2014). Viral overexpression of TREM 2 was found to decrease pilocarpine induced seizures, while also reducing oxidative stress and increasing cell survival. TREM2s direct physiological role in AD has proven difficult to define, however, despite much investigation (Ulrich et al., 2017; Carmona et al., 2018). Likewise, its role in the induction of seizure pathology is yet to be detailed.
Electroencephalographic Slowing and Hippocampal Hyperexcitability
The 3xTG mouse combines the APP Swedish mutation with the PSEN M146V mutation and the P301L mutation of Microtubule Associated Protein Tau (MAPT). The PSEN M146V mutation impairs γ-secretase cleavage of APP resulting in greater secretion of Aβ42 and increased Aβ42/Aβ40 ratio (Li et al., 2016). Individuals carrying this PSEN1 mutation have experienced onset of familial AD between 35 and 40 years with death in their early 50s (Clark et al., 1995; Riudavets et al., 2013). The MAPT P301L mutation is a missense point mutation in a conserved region of the gene encoding the microtubule protein tau. This mutation induces aggregation of tau protein in neurons and microglia, particularly in the hippocampus, neocortex, and substantia nigra leading to neuron loss and gliosis resulting in dementia and Parkinsonism, but notably this mutation is not specific to AD per se (Dumanchin et al., 1998; Hutton et al., 1998). When combined in the 3xTg mouse, these mutations lead to Aβ deposits by 6 months of age, age related tau aggregation after 6 months of age, and decreased LTP (Oddo et al., 2003). Gliosis has been detected at 7 months of age (Caruso et al., 2013) and impaired MWM and deficits in contextual fear conditioning emerges from 4 to 6 months (Billings et al., 2005).
Resting state functional magnetic imaging (re-fMRI) performed in 3xTg mice has revealed that, although tau immunoreactivity was present in 6–8 and 18–24 months old groups, the older cohort displays a higher degree of synchronous activity between multiple brain regions including hippocampus, auditory association cortex, temporal association cortex, and lateral entorhinal cortex. Thus, although development of tauopathy was not age-dependent in these mice, development of hypersynchronous activity in 3xTg is an age-dependent phenotype (Liu et al., 2018).
EEG slowing and hippocampal hyperexcitability are also AD-seizure relevant phenotypes in 3xTg mice. Juvenile 3xTg mice (postnatal day 30) present with increased hippocampal theta activity that is associated with increased tau phosphorylation. In these mice, this effect can be reversed by the potassium channel antagonist 4-aminopyradine (Mondragón-Rodríguez et al., 2018b). In vivo anesthetized recordings in older 4–6 and 17–18 months 3xTg mice comparing evoked responses in CA1 and DG of hippocampus following performant path stimulation revealed that short-term plasticity in response to brief high frequency stimulation is facilitated in both young and old 3xTg mice. Though responses to single or low frequency paired-pulses indicate that synapse integrity is nominal (Davis et al., 2014). This hippocampal hyperexcitability has been associated with pilocarpine-evoked (Yan et al., 2012), audiogenic (Kazim et al., 2017), and corneal kindled seizures (Vyver et al., 2020). This seizure susceptibility could be attenuated by treatment with an anti-human APP antibody or with an mGluR5 antagonist (Kazim et al., 2017). Though it remains to be determined if spontaneous seizure activity is present in 3xTg mice. Important to note are reports of inconsistent phenotypes related to behavioral and pathological changes in 3xTG mice thus again emphasizing the need for rigorous reporting of source and background strain (Onos et al., 2016).
Patch clamp recordings in hippocampal slices from 3 months old Tg2576 have revealed that overexpression of voltage gated sodium channels (NaV) leads to resting membrane depolarization and increased spike frequency. Interestingly, exposure of Tg2576 embryos to Aβ1–42 induced a selective upregulation of NaV 1.6 channels (Ciccone et al., 2019). In each case, it appears that neuronal hyperexcitability is present in Tg2576 mice at a very early age. This is coincident with an increase in theta power during REM (Kam et al., 2016), which is likely involved in the progressive cognitive impairments in Tg2576 mice.
Fu et al. (2019) demonstrated that epileptiform activity is detectable in J20 mice as early as 1 month of age and is associated with neuronal hyperexcitability. At 2 months of age these mice begin experiencing seizures that coincide with increases in hippocampal neurogenesis. This increased neurogenesis was not detected at 1 month of age. Decreased neurogenesis was then detected at 3 months of age compared to non-transgenic mice, which continued to decline over the lifespan of the mouse. These findings may indicate that neuronal hyperexcitability in the hippocampus drives early epileptiform activity, spurring an initial increase in neurogenesis in J20 mice. This neurogenesis feeds forward to produce seizures and deplete neural stem cells, leading to the advanced neurodegeneration in the J20 line. Treatment with levetiracetam was effective at blocking the increases in neurogenesis, rescue spatial discrimination deficits, and suppress seizures in J20 mice (Sanchez et al., 2012; Corbett et al., 2017). Notably, phase II clinical trials are currently underway to investigate the degree to which suppression of seizure related activity by levetiracetam can improve outcomes in AD (Levetiracetam for Alzheimer’s Disease-Associated Epileptiform Activity, 2020).
Seizure activity and the resulting cognitive deficits in J20 mice may be related to a deficient expression of calcium binding protein calbindin-D28k (You et al., 2017). Calbindin-D28k is a critical regulator of calcium signaling in the dentate gyrus (Brini et al., 2014) and expression of calbindin is inversely related to seizure activity in patients with temporal lobe epilepsy (Karádi et al., 2012). Calbindin expression was found to be decreased in both AD and non-AD dementia patients (McLachlan et al., 1987), is decreased in J20 mice (You et al., 2017), and knockout of calbindin-D28k results in deficient synaptic plasticity and spatial working memory impairment (Molinari et al., 1996). Transcription of the calbindin allele in the dentate gyrus is suppressed by promoter acetylation mediated by the activity driven expression of the immediate early gene ΔFosB. Seizure activity in J20 mice has been reported to be related to increased ΔFosB activity and reduced calbindin expression in the hippocampus (You et al., 2017). These markers coincide with decreased theta power and decreased time spent asleep, which predicted MWM deficits (Hazra et al., 2016).
A study in APP/PS1 mice compared induction of LTP in hippocampal slices of 1.5 and 8 months old mice following high frequency stimulation (HFS) of Schaffer collaterals. Small, but statistically non-significant differences in post HFS LTP were measured in 1.5 months old mice. At 8-months LTP was significantly diminished compared to younger mice. Smaller field excitatory post-synaptic potential (fEPSP) output in response to stimulation and increases in paired-pulse ratio at shorter inter-pulse intervals were also described. Treatment with the GABA agonist diazepam was sufficient to reduce paired-pulse ratios in 8 months old WT littermates, but did not produce a significant decrease in the APP/PS1 mice. Taken together, these studies indicate a loss of function in GABA signaling that produces dysfunction in hippocampal LTP (Oyelami et al., 2016), which may lead to hyperexcitability and age-dependent seizure and epileptiform activity in this strain (Reyes-Marin and Nuñez, 2017). In line with this observation, reductions in GABA currents, changes in GABA subunit expression, and a loss in sensitivity to GABA have been observed in post-mortem AD brains (Limon et al., 2012).
Multiple reports have characterized alterations in EEG power in 5xFAD mice. Siwek et al. (2015) reported an increase in hippocampal theta activity, but an overall decrease in mean theta frequency within that range. Both effects would be consistent with an EEG slowing phenotype. A separate report documented reductions in power across the delta-gamma range, but a substantial increase in power within the subdelta range (0.1–0.5 Hz) in 6 months old 5xFAD mice. This range of activity is not as frequently reported, but suggests a strong EEG slowing phenotype in 5xFAD (Schneider et al., 2014). Anesthetized field recordings in 8 months old 5xFAD have identified decreases in theta and gamma power elicited by brainstem stimulation (Stoiljkovic et al., 2016). Taken together, these data indicate neurophysiological deficiencies, especially within the theta frequency range, that are consistent with an EEG slowing phenotype in 5xFAD mice.
APOE4 mice were recently shown to have greater glucose metabolism in the entorhinal cortex measured by fMRI, increased activity of excitatory neurons in awake and freely moving mice, and longer duration extracellular field potentials with diminished LTP in hippocampal slices (Nuriel et al., 2017). These results provide strong evidence for an APOE4 related hyperexcitability phenotype in AD. Increased activity within the theta range has also been reported in APOE4 mice (Hunter et al., 2012). Though AD like progression associated with behavioral phenotypes are limited as deficits in spatial learning and memory are present in 3 months old mice (Rodriguez et al., 2013).
Rare gene variants of the disinterring and metalloproteinase domain-containing protein 10 (ADAM10) confer a low to moderate risk of AD. ADAM10 is an α-secretase that cleaves APP into non-toxic products preventing the production of Aβ fragments. Mutation or loss of function of ADAM10 will favor BACE cleavage and production of plaque forming Aβ fragments. Overexpression of ADAM10 in the hippocampus of C57BL/6J mice reduced markers of neuroinflammation (iNOS, COX-2, IL1-β, and TNF-α) and protected against pilocarpine-induced seizures (Zhu et al., 2018). On the other hand, ADAM10 knockout mice show increased activation of microglia, gliosis, and loss of spine morphology that coincided with a leftward shift of hippocampal EEG power in theta and gamma ranges, indicative of a hippocampal slowing phenotype (Prox et al., 2013). A direct relationship between ADAM10 mutation and seizure has yet to be described in humans but speaks to the utility of EEG findings in animal models that may be explored clinically.
Although not specific to AD, alpha-synuclein mutant mice used in the study of Dementia with Lewy Bodies (DLB) and other synucleinopathies may also be instructive to mechanisms related to seizure activity and hippocampal dysfunction. In DLB, inclusions of α-synuclein form when the presynaptic membrane is mislocalized and begins to aggregate (Morris et al., 2015). EEG slowing is a key biomarker shared by DLB and AD. In AD, EEG slowing is attributable to loss of acetylcholine neurons into hippocampus. This contributes to breakdowns in hippocampal plasticity and dysregulation of recurrent excitatory/inhibitory circuitry between the cortex. Intermittent seizure activity with myoclonic jerks and EEG featuring prominent ictal spikes were evident in mice overexpressing human α-synuclein. These events coincided with a leftward shift in baseline EEG power reflecting a greater proportion of low frequency activity (Morris et al., 2015). When overexpression of a mutant transgene of α-synuclein (A53S) is combined in a mouse lacking endogenous tau, EEG-slowing induced by overexpression of α-synuclein is markedly attenuated, suggesting a clear link between these two proteins in seizure pathology witnessed in DLB (Peters et al., 2020).
The AD-relevant neural activity has also been identified in some rat models as well. F344-AD rats express two transgenes: Swedish (APP KN670/671NL) and human presenilin PS1ΔE9. Urethane anesthetized and freely moving F344-AD rats exhibit diminished activity within alpha frequencies. Though, diminished activity was also observed in the theta frequency range, opposite of what is typically seen for EEG slowing. In both WT and F344-AD anesthetized rats, stimulation of the tegmental nucleus pontis oralis at 6 months of age strongly entrains a theta oscillation in the hippocampus. At 12 months, however, this evoked theta oscillation is diminished (Stoiljkovic et al., 2019). Treatment with the acetylcholinesterase inhibitor donepezil was able to restore evoked and freely-moving theta oscillations in F344-AD rats, while augmenting this activity in WT counterparts (Stoiljkovic et al., 2018). In patients with mild dementia, however, long-term treatment with donepezil reduces absolute-theta activity. So it is likely the beneficial effects of donepezil in patients reflects a long-term response to treatment (Kogan et al., 2001).
While the majority of EEG phenotypes in AD animal models have been reported in rodent species including in this present review, despite the utility of EEG in rodents for translational studies, it should not be dismissed that neurophysiological characteristics of rodents are largely different from those of humans. Thus, most pharmaceutical interventions that have been developed in rodents and reported as efficacious for improving cognitive impairment did not show any significant cognitive improvement in clinical trials (Silverman et al., 2020). From this perspective, the use of NHPs as animal models for AD have been of growing interest because of the maturity and anatomical similarity of the primate brain to those of humans (Kaiser and Feng, 2015; Van Essen et al., 2019). It has been demonstrated that aggregation of Aβ plaques which is similar in sequence to humans were naturally found in aged animals accompanied by declining cognitive function (Geula et al., 2002; Sani et al., 2003; Oikawa et al., 2010). In NHPs, some AD models have already been published including rhesus monkeys, cynomolgus monkeys, vervet monkeys, and marmosets (Geula et al., 1998, 2002; Lemere et al., 2004; Forny-Germano et al., 2014; Yang et al., 2014; Melamed et al., 2017; Seita et al., 2020). Details of each model were described elsewhere but it is noteworthy that recent technological advances have made it possible to generate genetically engineered animal models in NHPs (Sasaki et al., 2009; De Felice and Munoz, 2016; Li et al., 2019; Seita et al., 2020). There are still a number of limitations when utilizing NHPs as AD models such as their relatively long lifespans compared to rodents as well as ethical and technical issues. While the utility for conducting EEG across NHP species has been demonstrated (Muggleton et al., 2005; Authier et al., 2014; Cheng et al., 2015; Nakazawa et al., 2015; Ishikawa et al., 2017; Uslaner et al., 2018), to the best our knowledge, only one comprehensive study investigating epileptiform activity in an AD relevant NHP model has been reported; reporting intriguing results of EEG signatures with corresponding pathology and cognitive decline in mouse lemurs (Gary et al., 2019). Further studies with NHPs could reveal the roles of epileptiform activity of neurons in AD and accelerate the understanding of the etiology and progressive mechanism of this disease, as well as provide primate specific translational outcomes for AD.
Theta-Gamma Interactions
TGC is impaired in AppNL–F mice. Local field potentials were recorded in 5 months old homozygous male and female AppNL–F and five non-litter male and female C57BL/6 mice using tetrodes implanted and advanced through the entorhinal cortex. TGC was measured as the phase at the time of gamma oscillation maxima. Prominent gamma oscillations were characterized during theta states in both strains. Further the power of gamma oscillations did not differ between strains. However, though the number of gamma oscillations were not significantly affected in AppNL–F mice the temporal structure of gamma oscillations was impaired. Specifically, the peak of gamma oscillations observed in the falling phase to trough of theta was reduced in magnitude compared to C57BL/6. So, while epileptiform activity may be absent in AppNL–F mice, they are not spared in terms of EEG abnormalities (Nakazono et al., 2017).
Alterations in spike-gamma coupling have been observed in AppNL–G–F mice. Spike-gamma coupling refers to the generation of gamma oscillations generated by rhythmic synchronous activity of fast spiking interneurons. A loss of spike-gamma coupling could lead to impairments in theta-gamma coupling as temporal associations breakdown across oscillatory frequencies. Patch clamp recordings of fast spiking interneurons and pyramidal cells in 1, 2, 4, and 6 months old. AppNL–G–F mice revealed impairment of spike-gamma coherence as early as 2 months of age, preceding amyloid plaque formation. Gamma oscillations also become degraded with age in AppNL–G–F mice, which is correlated to Aβ1–42 concentration in the brain (Arroyo-García et al., 2021). This result suggests that the breakdown of gamma oscillatory activity and coupling to fast-spiking interneurons in AppNL–G–F mice may contribute to breakdown of theta-gamma interactions, though this remains to be demonstrated.
J20 mice also exhibit breakdowns in cross frequency coupling. In an in vitro prep combining patch and field recordings with optogenetics in hippocampal tissue from 30 to 39 day old mice, a number of circuit-level abnormalities were characterized. First, theta oscillations displayed less rhythmicity compared to non-transgenic mice. TG mice also exhibited reduced theta-gamma CFC. Therefore it is clear that these circuit level disruptions are detectable in very young J20 mice, preceding any signs of Aβ accumulation (Mondragón-Rodríguez et al., 2018a). J20 mice expressing re-recombinase (PVJ20) to enable optogenetic interventions exhibit decreased slow gamma (30–60 Hz) coupling to theta. Gamma oscillation power was also reduced in the PVJ20 mice compared to PVJ20- littermates. Optogenetically mediated 40 Hz stimulation was effective in increasing slow gamma phase-amplitude coupling to theta. However, 80 Hz stimulation did not increase coupling, possibly because fast-gamma coupling was not impaired. Further, 40 Hz stimulation rescued a deficit in discrimination in a novel object place recognition paradigm (Etter et al., 2019). These results suggest that optogenetic stimulation in the slow gamma frequency range is capable of restoring gamma oscillations and improving deficient memory recall.
3xTg mice also exhibit deficient coordination between theta, slow gamma, and place cells in the hippocampus at 8–9 months of age. Mice were trained to run unidirectionally around a circular track to receive a reward that was always placed in the same location. Tetrodes aimed at the CA1 region of hippocampus recorded single units and continuous sampled local field potentials. Theta phase coupled increases in slow gamma power were diminished in 3xTG mice compared to WTs, suggesting that hippocampal slow gamma modulation is impaired in the 3xTg mouse (Mably et al., 2017). Though it is noteworthy that amyloid deposition was not detected in the 8–9 month 3xTg mice in this study, which contradicts earlier findings that amyloid is detectable in this strain as early as 4 months (Oddo et al., 2003).
Transgenic rats also exhibit impaired CFC. Anesthetized in vivo recordings in the hippocampus and mPFC of 9-month old F344-AD rats assessed phase-amplitude coupling, specifically the ability of low frequency theta oscillations to modulate high frequency gamma oscillations. This analysis showed a decrease in phase-amplitude CFC in both regions (Bazzigaluppi et al., 2018). These results suggest poor temporal coordination within these networks in this strain.
Discussion
Subclinical seizure and epileptiform activity are potentially very powerful pre-diagnostic biomarkers of prodromal dementia and LOAD (Vossel et al., 2013, 2016, 2017; Horváth et al., 2016; Palop and Mucke, 2016; Vöglein et al., 2020). Four distinct phenotypes have repeatedly emerged in the course of this and other reviews, which could potentially be assessed both in patient populations and mouse models using relatively inexpensive and accessible tools. These emergent phenotypes are (1) ectopic cortical spiking activity, especially during NREM sleep, absent overt physical symptoms of acute seizure, (2) hippocampal slowing evidenced by leftward shifts in the power distribution of EEG oscillatory activity at baseline, (3) hyperexcitability of hippocampal neurons, particularly in response to cortical inputs, and (4) theta-gamma interactions. In patient populations, this activity is not only highly predictive of disease course (Vöglein et al., 2020), but may precede the earliest cognitive symptoms in LOAD by >5 years (Vossel et al., 2013, 2017). Increased preventative screening could push this boundary even earlier and allow for aggressive intervention and cognitive therapy while also aiding family and caretakers in the preparation and planning of disease course. Absent any further advancement, these factors alone would dramatically improve quality of life of patients, loved ones, and caretakers, as well as the general population as a whole. With continued definition, methodological advancement, and standardization, regular EEG assessment of these phenotypes in conjunction with genetic screening for risk alleles could become a standard of preventative care in individuals over the age of 40.
Aside from clinical value, these EEG biomarkers have high potential to provide insights into the etiology and progression of AD in ways that go beyond amyloid and tau hypotheses that have failed to produce tenable, disease-modifying treatments for AD. In rodents, EEG and EMG can be recorded by wireless telemetric transmitters using skull surface electrodes that can be implanted with a relatively simple surgical preparation (Vogel et al., 2002; Kramer and Kinter, 2003; Lundt et al., 2015). This can be combined with depth electrodes throughout related circuitry (Gureviciene et al., 2019). Analysis of these data sets can also be largely automated. This preparation allows for minimally invasive, continual assessment of epileptiform activity. There is also value added in sleep analysis in the same animal. As has been noted in this and earlier reviews (Born, 2015; Palop and Mucke, 2016; Vossel et al., 2017; Horvath, 2018), nearly equivalent measures of human seizure and epileptiform activity are detectible in model mice for AD. These measures are sensitive to acetylcholinesterase inhibitors (Reyes-Marin and Nuñez, 2017; Stoiljkovic et al., 2018) and anti-seizure (Sanchez et al., 2012) medications utilized in the treatment of AD. Taken together, this is evidence of high construct, face, and predictive validity of these biomarkers. Understanding of the origins and physiology of epileptiform activity in model animals has the potential to broaden horizons for novel treatments in AD.
There is plenty of room for improvement in this arena. First, there is no animal model that fully recapitulates all aspects of AD; an important caveat that has been discussed extensively elsewhere (see Jankowsky and Zheng, 2017; Oblak et al., 2020; Mckean et al., 2021; Vitek et al., 2021). Although these animal models continue to be powerful tools for forming our current understanding of AD and for translational studies evaluating potential therapeutic interventions, most of the rodent models evaluated for EEG phenotypes to date are primarily transgenic mouse models based on familial, early onset mutations. The models present with aggressive amyloid or tau overexpression that manifests at an early age (e.g., 6 months). Presentation of disease in 6–12 months aged animals is merely equivalent to that of a 20–50 years old human and therefore does not capture physiological changes related to aging which are critical for the study of neurodegenerative diseases including AD. While minimizing aging costs for research colonies of aging animal models may be a benefit in the short term to researchers, incorporating the biological construct of aging is important for improving preclinical to clinical translation and represents a major pitfall which has plagued the AD animal model field. Relatedly, the lack of applying standardized nomenclature of these mouse lines further complicates an already dense literature. Mouse models are frequently referred to with shorthanded nomenclature which do not necessarily include the specific mutation or background strain information (Sukoff Rizzo et al., 2020), Examples include whether the strain is overexpressing a transgene, if sequence of alleles are humanized, and if the mice are congenic or a hybrid cross of multiple strains. Importantly, the background strains themselves have divergent EEG signatures, which may contribute to the phenotype of the mutation and are often under-reported (Copping et al., 2019). These pitfalls may lead to misidentification of similarities between different strains or under appreciation of overlap where it does exist. Ensuring that standardized nomenclature is applied when reporting data from mouse models continues to be critical and requires much consideration by researchers.
Second, understanding of the genetic mechanisms and environmental interactions contributing to LOAD are still poorly understood. Third, understanding of the timecourse of progressive degeneration and normal aging related changes in EEG in animal models has been limited. Finally, putative disease modifying drugs developed using these mice have all thus far failed to translate (Silverman et al., 2020). If the field is to realize the power of EEG as an important translational biomarker there is an onus on researchers to begin incorporating the key risk factors for AD, of aging and LOAD risk genes (Onos et al., 2016; Oblak et al., 2020).
Ideally, these EEG measures will begin to inform the onset of pathological changes in AD and predict cognitive decline, which has thus far eluded researchers to date. To date, EEG as an early biomarker has not yet fully realized its potential with respect to cognitive changes. Importantly, detection of spontaneous sub-clinical seizures may be difficult in a clinical setting where it may not be possible to record for extensive periods of time, which could limit its potential. Optimally, future investigations can begin incorporating EEG in animals performing cognitive tasks with discrete stimulus presentation and high temporal fidelity with recordings, such as the touchscreen batteries that are rapidly becoming increasingly commonplace in behavioral neuroscience (Horner et al., 2013; Marquardt et al., 2017). These active tasks can provide finer-grain analysis of pathophysiology in AD, potentially across a shorter period. Indeed, touchscreen tasks have already been validated in AppNL–G–F/NL–G–F mice (Saifullah et al., 2020) and methods to pair these tasks with EEG have already been detailed (Marquardt et al., 2017). These methods also have the potential to improve translation between rodents and humans by putatively equalizing stimulus detection and response strategies across species to eliminate potential confounds.
The specificity of EEG signatures as biomarkers continues to remain under speculation across the field and requires more research. Measures of electroencephalographic hyperexcitability have been reported in several CNS disorders including in multiple sclerosis (Zipser et al., 2018), autism spectrum disorders (Boutros et al., 2015), and Parkinson’s disease (Yu et al., 2007), among others. Indeed more work is required in order to determine the distinct contributions of AD related pathology in the pathogenesis of these EEG biomarkers and specific AD related signatures. In particular, it will be very important that future endeavors understand time course of these markers. In animal models, many have been characterized very early on, even before significant amyloid deposition. Therefore, prospective, longitudinal studies would be key in parsing the specific contributions of these EEG biomarkers to the pathogenesis of age-related cognitive decline in AD.
Overall, pre-clinical AD research is in need of new approaches. Decades of research focused on behavioral testing in FAD mutant mouse models have not appreciably moved the needle on our ability to prevent degeneration or extend lifespan in patients. EEG assessment of epileptiform activity in diverse model systems including NHPs and new models genetically engineered with mutations relevant to LOAD risk has the potential to push this horizon in new ways and ultimately enable accelerating the pace of bringing effective therapeutics to patients.
Author Contributions
All authors listed have made a substantial, direct, and intellectual contribution to the work, and approved it for publication.
Funding
Funding for this work was supported by NIH U54 AG05434503 and NIH 1R01AG067289-01.
Conflict of Interest
The authors declare that the research was conducted in the absence of any commercial or financial relationships that could be construed as a potential conflict of interest.
Publisher’s Note
All claims expressed in this article are solely those of the authors and do not necessarily represent those of their affiliated organizations, or those of the publisher, the editors and the reviewers. Any product that may be evaluated in this article, or claim that may be made by its manufacturer, is not guaranteed or endorsed by the publisher.
References
Abe, Y., Ikegawa, N., Yoshida, K., Muramatsu, K., Hattori, S., Kawai, K., et al. (2020). Behavioral and electrophysiological evidence for a neuroprotective role of aquaporin-4 in the 5xFAD transgenic mice model. Acta Neuropathol. Commun. 8:67. doi: 10.1186/s40478-020-00936-3
Alzheimer, A. (1906). Uber einen eigenartigen schweren Erkrankungsprozess der Hirninde. Neurol. Cent. 25:1134.
Alzheimer’s Disease Fact Sheet (2020). Alzheimer’s Disease Fact Sheet. Available online at: http://www.nia.nih.gov/health/alzheimers-disease-fact-sheet (accessed November 16, 2020).
Alzheimers.net (2020). Alzheimer’s Statistics Alzheimers.net. Available online at: https://www.alzheimers.net/resources/alzheimers-statistics (accessed November 16, 2020).
Arroyo-García, L. E., Isla, A. G., Andrade-Talavera, Y., Balleza-Tapia, H., Loera-Valencia, R., Alvarez-Jimenez, L., et al. (2021). Impaired spike-gamma coupling of area CA3 fast-spiking interneurons as the earliest functional impairment in the AppNL-G-F mouse model of Alzheimer’s disease. Mol. Psychiatry 26, 5557–5567. doi: 10.1038/s41380-021-01257-0
Authier, S., Bassett, L., Pouliot, M., Rachalski, A., Troncy, E., Paquette, D., et al. (2014). Effects of amphetamine, diazepam and caffeine on polysomnography (EEG, EMG, EOG)-derived variables measured using telemetry in Cynomolgus monkeys. J. Pharmacol. Toxicol. Methods 70, 287–294. doi: 10.1016/j.vascn.2014.10.004
Axelman, K., Basun, H., Winblad, B., and Lannfelt, L. (1994). A large Swedish family with Alzheimer’s disease with a codon 670/671 amyloid precursor protein mutation. A clinical and genealogical investigation. Arch. Neurol. 51, 1193–1197. doi: 10.1001/archneur.1994.00540240037013
Bartus, R. T., Dean, R. L., Beer, B., and Lippa, A. S. (1982). The cholinergic hypothesis of geriatric memory dysfunction. Science 217, 408–414. doi: 10.1126/science.7046051
Bazzigaluppi, P., Beckett, T. L., Koletar, M. M., Lai, A. Y., Joo, I. L., Brown, M. E., et al. (2018). Early-stage attenuation of phase-amplitude coupling in the hippocampus and medial prefrontal cortex in a transgenic rat model of Alzheimer’s disease. J. Neurochem. 144, 669–679. doi: 10.1111/jnc.14136
Beagle, A. J., Darwish, S. M., Ranasinghe, K. G., La, A. L., Karageorgiou, E., and Vossel, K. A. (2017). Relative incidence of seizures and myoclonus in Alzheimer’s disease, dementia with lewy bodies, and frontotemporal dementia. J. Alzheimers Dis. 60, 211–223. doi: 10.3233/JAD-170031
Billings, L. M., Oddo, S., Green, K. N., McGaugh, J. L., and LaFerla, F. M. (2005). Intraneuronal Aβ causes the onset of early alzheimer’s disease-related cognitive deficits in transgenic mice. Neuron 45, 675–688. doi: 10.1016/j.neuron.2005.01.040
Blumenfeld, H. (2005). Cellular and network mechanisms of spike-wave seizures. Epilepsia 46, 21–33. doi: 10.1111/j.1528-1167.2005.00311.x
Born, H. A. (2015). Seizures in Alzheimer’s disease. Neuroscience 286, 251–263. doi: 10.1016/j.neuroscience.2014.11.051
Boutros, N. N., Lajiness-O’Neill, R., Zillgitt, A., Richard, A. E., and Bowyer, S. M. (2015). EEG changes associated with autistic spectrum disorders. Neuropsychiatr. Electrophysiol. 1:3. doi: 10.1186/s40810-014-0001-5
Brenner, R. P., Ulrich, R. F., Spiker, D. G., Sclabassi, R. J., Reynolds, C. F., Marin, R. S., et al. (1986). Computerized EEG spectral analysis in elderly normal, demented and depressed subjects. Electroencephalogr. Clin. Neurophysiol. 64, 483–492. doi: 10.1016/0013-4694(86)90184-7
Brini, M., Calì, T., Ottolini, D., and Carafoli, E. (2014). Neuronal calcium signaling: function and dysfunction. Cell. Mol. Life Sci. 71, 2787–2814. doi: 10.1007/s00018-013-1550-7
Brown, R., Lam, A. D., Gonzalez-Sulser, A., Ying, A., Jones, M., Chou, R. C.-C., et al. (2018). Circadian and brain state modulation of network hyperexcitability in alzheimer’s disease. eNeuro 5:ENEURO.0426-17.2018. doi: 10.1523/ENEURO.0426-17.2018
Brunetti, V., D’Atri, A., Della Marca, G., Vollono, C., Marra, C., Vita, M. G., et al. (2020). Subclinical epileptiform activity during sleep in Alzheimer’s disease and mild cognitive impairment. Clin. Neurophysiol. 131, 1011–1018. doi: 10.1016/j.clinph.2020.02.015
Buzsáki, G., Bickford, R. G., Armstrong, D. M., Ponomareff, G., Chen, K. S., Ruiz, R., et al. (1988). Electric activity in the neocortex of freely moving young and aged rats. Neuroscience 26, 735–744. doi: 10.1016/0306-4522(88)90095-4
Carmona, S., Zahs, K., Wu, E., Dakin, K., Bras, J., and Guerreiro, R. (2018). The role of TREM2 in Alzheimer’s disease and other neurodegenerative disorders. Lancet Neurol. 17, 721–730. doi: 10.1016/S1474-4422(18)30232-1
Caruso, D., Barron, A. M., Brown, M. A., Abbiati, F., Carrero, P., Pike, C. J., et al. (2013). Age-related changes in neuroactive steroid levels in 3xTg-AD mice. Neurobiol. Aging 34, 1080–1089. doi: 10.1016/j.neurobiolaging.2012.10.007
Castillo, E., Leon, J., Mazzei, G., Abolhassani, N., Haruyama, N., Saito, T., et al. (2017). Comparative profiling of cortical gene expression in Alzheimer’s disease patients and mouse models demonstrates a link between amyloidosis and neuroinflammation. Sci. Rep. 7:17762. doi: 10.1038/s41598-017-17999-3
Cheng, I. H., Scearce-Levie, K., Legleiter, J., Palop, J. J., Gerstein, H., Bien-Ly, N., et al. (2007). Accelerating Amyloid-β Fibrillization reduces oligomer levels and functional deficits in Alzheimer disease mouse models *. J. Biol. Chem. 282, 23818–23828. doi: 10.1074/jbc.M701078200
Cheng, L., Lei, S., Chen, S.-H., Hong, Z., Yang, T.-H., Li, L., et al. (2015). Pretreatment with intravenous levetiracetam in the rhesus monkey Coriaria Lactone-induced status epilepticus model. J. Neurol. Sci. 348, 111–120. doi: 10.1016/j.jns.2014.11.017
Chow, V. W., Mattson, M. P., Wong, P. C., and Gleichmann, M. (2010). An overview of APP processing enzymes and products. Neuromol. Med. 12, 1–12. doi: 10.1007/s12017-009-8104-z
Ciccone, R., Franco, C., Piccialli, I., Boscia, F., Casamassa, A., de Rosa, V., et al. (2019). Amyloid β-induced upregulation of Na v 1.6 underlies neuronal hyperactivity in Tg2576 Alzheimer’s disease mouse model. Sci. Rep. 9:13592. doi: 10.1038/s41598-019-50018-1
Clark, R. F., Hutton, M., Fuldner, M., Froelich, S., Karran, E., Talbot, C., et al. (1995). The structure of the presenilin 1 (S182) gene and identification of six novel mutations in early onset AD families. Nat. Genet. 11, 219–222. doi: 10.1038/ng1095-219
Coben, L. A., Danziger, W., and Storandt, M. (1985). A longitudinal EEG study of mild senile dementia of Alzheimer type: changes at 1 year and at 2.5 years. Electroencephalogr. Clin. Neurophysiol. 61, 101–112. doi: 10.1016/0013-4694(85)91048-X
Copping, N., Adhikari, A., Petkova, S., and Silverman, J. (2019). Genetic Backgrounds have unique seizure response profiles and behavioral outcomes following convulsant administration. Epilepsy Behav. EB 101:106547. doi: 10.1016/j.yebeh.2019.106547
Corbett, B. F., You, J. C., Zhang, X., Pyfer, M. S., Tosi, U., Iascone, D. M., et al. (2017). ΔFosB regulates gene expression and cognitive dysfunction in a mouse model of Alzheimer’s disease. Cell Rep. 20, 344–355. doi: 10.1016/j.celrep.2017.06.040
Cortini, F., Cantoni, C., and Villa, C. (2018). Epileptic seizures in autosomal dominant forms of Alzheimer’s disease. Seizure 61, 4–7. doi: 10.1016/j.seizure.2018.07.015
Crouzin, N., Baranger, K., Cavalier, M., Marchalant, Y., Cohen-Solal, C., Roman, F. S., et al. (2013). Area-specific alterations of synaptic plasticity in the 5XFAD mouse model of Alzheimer’s disease: dissociation between somatosensory cortex and hippocampus. PLoS One 8:e74667. doi: 10.1371/journal.pone.0074667
Cuajungco, M. P., Frederickson, C. J., and Bush, A. I. (2005). “Amyloid-β metal interaction and metal chelation,” in Alzheimer’s Disease: Cellular and Molecular Aspects of Amyloid β Subcellular Biochemistry, eds J. R. Harris and F. Fahrenholz (Boston, MA: Springer US), 235–254. doi: 10.1007/0-387-23226-5_12
Davis, K. E., Fox, S., and Gigg, J. (2014). Increased hippocampal excitability in the 3xTgAD mouse model for Alzheimer’s disease in vivo. PLoS One 9:e91203. doi: 10.1371/journal.pone.0091203
De Felice, F. G., and Munoz, D. P. (2016). Opportunities and challenges in developing relevant animal models for Alzheimer’s disease. Ageing Res. Rev. 26, 112–114. doi: 10.1016/j.arr.2016.01.006
Devi, L., and Ohno, M. (2010). Phospho-eIF2α level is important for determining abilities of BACE1 reduction to rescue cholinergic neurodegeneration and memory defects in 5XFAD mice. PLoS One 5:e12974. doi: 10.1371/journal.pone.0012974
Dickerson, B. C., Salat, D. H., Greve, D. N., Chua, E. F., Rand-Giovannetti, E., Rentz, D. M., et al. (2005). Increased hippocampal activation in mild cognitive impairment compared to normal aging and AD. Neurology 65, 404–411. doi: 10.1212/01.wnl.0000171450.97464.49
Drevets, W. C., Zarate, C. A., and Furey, M. L. (2013). Antidepressant effects of the muscarinic cholinergic receptor antagonist scopolamine: a review. Biol. Psychiatry 73, 1156–1163. doi: 10.1016/j.biopsych.2012.09.031
Dumanchin, C., Camuzat, A., Campion, D., Verpillat, P., Hannequin, D., Dubois, B., et al. (1998). Segregation of a missense mutation in the microtubule-associated protein tau gene with familial frontotemporal dementia and Parkinsonism. Hum. Mol. Genet. 7, 1825–1829. doi: 10.1093/hmg/7.11.1825
Ebert Kirch. (1998). Scopolamine model of dementia: electroencephalogram findings and cognitive performance. Eur. J. Clin. Invest. 28, 944–949. doi: 10.1046/j.1365-2362.1998.00393.x
Ebert, U., Grossmann, M., Oertel, R., Gramatté, T., and Kirch, W. (2001). Pharmacokinetic-pharmacodynamic modeling of the electroencephalogram effects of scopolamine in healthy volunteers. J. Clin. Pharmacol. 41, 51–60. doi: 10.1177/00912700122009836
Etter, G., van der Veldt, S., Manseau, F., Zarrinkoub, I., Trillaud-Doppia, E., and Williams, S. (2019). Optogenetic gamma stimulation rescues memory impairments in an Alzheimer’s disease mouse model. Nat. Commun. 10:5322. doi: 10.1038/s41467-019-13260-9
Ferguson, J. M. (2001). SSRI antidepressant medications: adverse effects and tolerability. Prim. Care Companion J. Clin. Psychiatry 3, 22–27. doi: 10.4088/pcc.v03n0105
Flanigan, T. J., Xue, Y., Kishan Rao, S., Dhanushkodi, A., and McDonald, M. P. (2014). Abnormal vibrissa-related behavior and loss of barrel field inhibitory neurons in 5xFAD transgenics. Genes Brain Behav. 13, 488–500. doi: 10.1111/gbb.12133
Forny-Germano, L., Lyra e Silva, N. M., Batista, A. F., Brito-Moreira, J., Gralle, M., Boehnke, S. E., et al. (2014). Alzheimer’s disease-like pathology induced by Amyloid-β oligomers in nonhuman primates. J. Neurosci. 34, 13629–13643. doi: 10.1523/JNEUROSCI.1353-14.2014
Frere, S., and Slutsky, I. (2018). Alzheimer’s disease: from firing instability to homeostasis network collapse. Neuron 97, 32–58. doi: 10.1016/j.neuron.2017.11.028
Fu, C.-H., Iascone, D. M., Petrof, I., Hazra, A., Zhang, X., Pyfer, M. S., et al. (2019). Early seizure activity accelerates depletion of hippocampal neural stem cells and impairs spatial discrimination in an Alzheimer’s disease model. Cell Rep. 27, 3741–3751.e4. doi: 10.1016/j.celrep.2019.05.101
Galimberti, D., and Scarpini, E. (2011). Disease-modifying treatments for Alzheimer’s disease. Ther. Adv. Neurol. Disord. 4, 203–216. doi: 10.1177/1756285611404470
Gary, C., Lam, S., Hérard, A.-S., Koch, J. E., Petit, F., Gipchtein, P., et al. (2019). Encephalopathy induced by Alzheimer brain inoculation in a non-human primate. Acta Neuropathol. Commun. 7:126. doi: 10.1186/s40478-019-0771-x
Gengler, S., Hamilton, A., and Hölscher, C. (2010). Synaptic plasticity in the hippocampus of a APP/PS1 mouse model of Alzheimer’s disease is impaired in old but not young mice. PLoS One 5:e9764. doi: 10.1371/journal.pone.0009764
Geula, C., Nagykery, N., and Wu, C.-K. (2002). Amyloid-beta deposits in the cerebral cortex of the aged common marmoset (Callithrix jacchus): incidence and chemical composition. Acta Neuropathol. (Berl.) 103, 48–58. doi: 10.1007/s004010100429
Geula, C., Wu, C. K., Saroff, D., Lorenzo, A., Yuan, M., and Yankner, B. A. (1998). Aging renders the brain vulnerable to amyloid beta-protein neurotoxicity. Nat. Med. 4, 827–831. doi: 10.1038/nm0798-827
Golden, G. T., Ferraro, T. N., Smith, G. G., Snyder, R. L., Jones, N. L., and Berrettini, W. H. (2001). Acute cocaine-induced seizures: differential sensitivity of six inbred mouse strains. Neuropsychopharmacology 24, 291–299. doi: 10.1016/S0893-133X(00)00204-9
Goodman, M. S., Kumar, S., Zomorrodi, R., Ghazala, Z., Cheam, A. S. M., Barr, M. S., et al. (2018). Theta-gamma coupling and working memory in Alzheimer’s dementia and mild cognitive impairment. Front. Aging Neurosci. 10:101. doi: 10.3389/fnagi.2018.00101
Gureviciene, I., Ishchenko, I., Ziyatdinova, S., Jin, N., Lipponen, A., Gurevicius, K., et al. (2019). Characterization of epileptic spiking associated with brain amyloidosis in APP/PS1 mice. Front. Neurol. 10:1151. doi: 10.3389/fneur.2019.01151
Haass, C., and Selkoe, D. J. (2007). Soluble protein oligomers in neurodegeneration: lessons from the Alzheimer’s amyloid β-peptide. Nat. Rev. Mol. Cell Biol. 8, 101–112. doi: 10.1038/nrm2101
Hazra, A., Corbett, B. F., You, J. C., Aschmies, S., Zhao, L., Li, K., et al. (2016). Corticothalamic network dysfunction and behavioral deficits in a mouse model of Alzheimer’s disease. Neurobiol. Aging 44, 96–107. doi: 10.1016/j.neurobiolaging.2016.04.016
Holtzman, D. M., Herz, J., and Bu, G. (2012). Apolipoprotein E and apolipoprotein E receptors: normal biology and roles in Alzheimer disease. Cold Spring Harb. Perspect. Med. 2:a006312. doi: 10.1101/cshperspect.a006312
Horner, A. E., Heath, C. J., Hvoslef-Eide, M., Kent, B. A., Kim, C. H., Nilsson, S. R. O., et al. (2013). The touchscreen operant platform for testing learning and memory in rats and mice. Nat. Protoc. 8, 1961–1984. doi: 10.1038/nprot.2013.122
Horvath, A. (2018). EEG and ERP biomarkers of Alzheimer disease: a critical review. Front. Biosci. 23:183–220. doi: 10.2741/4587
Horváth, A., Szûcs, A., Barcs, G., and Kamondi, A. (2017). Sleep EEG detects epileptiform activity in Alzheimer’s disease with high sensitivity. J. Alzheimers Dis. 56, 1175–1183. doi: 10.3233/JAD-160994
Horváth, A., Szucs, A., Barcs, G., Noebels, J. L., and Kamondi, A. (2016). Epileptic Seizures in Alzheimer disease: a review. Alzheimer Dis. Assoc. Disord. 30, 186–192. doi: 10.1097/WAD.0000000000000134
Hsiao, K., Chapman, P., Nilsen, S., Eckman, C., Harigaya, Y., Younkin, S., et al. (1996). Correlative memory deficits, Abeta elevation, and amyloid plaques in transgenic mice. Science 274, 99–102. doi: 10.1126/science.274.5284.99
Huijbers, W., Mormino, E. C., Schultz, A. P., Wigman, S., Ward, A. M., Larvie, M., et al. (2015). Amyloid-β deposition in mild cognitive impairment is associated with increased hippocampal activity, atrophy and clinical progression. Brain J. Neurol. 138, 1023–1035. doi: 10.1093/brain/awv007
Hunter, J. M., Cirrito, J. R., Restivo, J. L., Kinley, R. D., Sullivan, P. M., Holtzman, D. M., et al. (2012). Emergence of a seizure phenotype in aged apolipoprotein epsilon 4 targeted replacement mice. Brain Res. 1467, 120–132. doi: 10.1016/j.brainres.2012.05.048
Hutton, M., Lendon, C. L., Rizzu, P., Baker, M., Froelich, S., Houlden, H., et al. (1998). Association of missense and 5’-splice-site mutations in tau with the inherited dementia FTDP-17. Nature 393, 702–705. doi: 10.1038/31508
Ishikawa, A., Sakai, K., Maki, T., Mizuno, Y., Niimi, K., Oda, Y., et al. (2017). Investigation of sleep-wake rhythm in non-human primates without restraint during data collection. Exp. Anim. 66, 51–60. doi: 10.1538/expanim.16-0073
Jacobsen, J. S., Wu, C.-C., Redwine, J. M., Comery, T. A., Arias, R., Bowlby, M., et al. (2006). Early-onset behavioral and synaptic deficits in a mouse model of Alzheimer’s disease. Proc. Natl. Acad. Sci. U.S.A. 103, 5161–5166. doi: 10.1073/pnas.0600948103
Jankowsky, J. L., and Zheng, H. (2017). Practical considerations for choosing a mouse model of Alzheimer’s disease. Mol. Neurodegener. 12:89. doi: 10.1186/s13024-017-0231-7
Jankowsky, J. L., Slunt, H. H., Gonzales, V., Jenkins, N. A., Copeland, N. G., and Borchelt, D. R. (2004). APP processing and amyloid deposition in mice haplo-insufficient for presenilin 1. Neurobiol. Aging 25, 885–892. doi: 10.1016/j.neurobiolaging.2003.09.008
Jeong, J. (2004). EEG dynamics in patients with Alzheimer’s disease. Clin. Neurophysiol. 115, 1490–1505. doi: 10.1016/j.clinph.2004.01.001
Jin, N., Babiloni, C., Drinkenburg, W. H., Hajós, M., Nygaard, H. B., and Tanila, H. (2021). Recommendations for preclinical testing of treatments against Alzheimer’s disease-related epileptiform spikes in transgenic rodent models. J. Alzheimers Dis. 1–16. doi: 10.3233/JAD-210209 [Epub ahead of print].
Johnson, E. C. B., Ho, K., Yu, G.-Q., Das, M., Sanchez, P. E., Djukic, B., et al. (2020). Behavioral and neural network abnormalities in human APP transgenic mice resemble those of App knock-in mice and are modulated by familial Alzheimer’s disease mutations but not by inhibition of BACE1. Mol. Neurodegener. 15:53. doi: 10.1186/s13024-020-00393-5
Kabir, M. T., Uddin, M. S., Mathew, B., Das, P. K., Perveen, A., and Ashraf, G. M. (2020). Emerging promise of immunotherapy for Alzheimer’s disease: a new hope for the development of Alzheimer’s vaccine. Curr. Top. Med. Chem. 20, 1214–1234. doi: 10.2174/1568026620666200422105156
Kaiser, T., and Feng, G. (2015). Modeling psychiatric disorders for developing effective treatments. Nat. Med. 21, 979–988. doi: 10.1038/nm.3935
Kam, K., Duffy, ÁM., Moretto, J., LaFrancois, J. J., and Scharfman, H. E. (2016). Interictal spikes during sleep are an early defect in the Tg2576 mouse model of β-amyloid neuropathology. Sci. Rep. 6:20119. doi: 10.1038/srep20119
Karádi, K., Janszky, J., Gyimesi, C., Horváth, Z., Lucza, T., Dóczi, T., et al. (2012). Correlation between calbindin expression in granule cells of the resected hippocampal dentate gyrus and verbal memory in temporal lobe epilepsy. Epilepsy Behav. 25, 110–119. doi: 10.1016/j.yebeh.2012.06.007
Kazim, S. F., Chuang, S.-C., Zhao, W., Wong, R. K. S., Bianchi, R., and Iqbal, K. (2017). Early-onset network hyperexcitability in presymptomatic Alzheimer’s disease transgenic mice is suppressed by passive immunization with anti-human APP/Aβ antibody and by mGluR5 blockade. Front. Aging Neurosci. 9:71. doi: 10.3389/fnagi.2017.00071
Kim, J., Basak, J. M., and Holtzman, D. M. (2009). The role of Apolipoprotein E in Alzheimer’s disease. Neuron 63, 287–303. doi: 10.1016/j.neuron.2009.06.026
Kimura, R., and Ohno, M. (2009). Impairments in remote memory stabilization precede hippocampal synaptic and cognitive failures in 5XFAD Alzheimer mouse model. Neurobiol. Dis. 33, 229–235. doi: 10.1016/j.nbd.2008.10.006
Kitchigina, V. F. (2018). Alterations of coherent theta and gamma network oscillations as an early biomarker of temporal lobe epilepsy and Alzheimer’s disease. Front. Integr. Neurosci. 12:36. doi: 10.3389/fnint.2018.00036
Kogan, E. A., Korczyn, A. D., Virchovsky, R. G., Klimovizky, S. Sh, Treves, T. A., and Neufeld, M. Y. (2001). EEG changes during long-term treatment with donepezil in Alzheimer’s disease patients. J. Neural Transm. 108, 1167–1173. doi: 10.1007/s007020170006
Kramer, K., and Kinter, L. B. (2003). Evaluation and applications of radiotelemetry in small laboratory animals. Physiol. Genomics 13, 197–205. doi: 10.1152/physiolgenomics.00164.2002
Lam, A. D., Deck, G., Goldman, A., Eskandar, E. N., Noebels, J., and Cole, A. J. (2017). Silent hippocampal seizures and spikes identified by foramen ovale electrodes in Alzheimer’s disease. Nat. Med. 23, 678–680. doi: 10.1038/nm.4330
Lanz, T. A., Carter, D. B., and Merchant, K. M. (2003). Dendritic spine loss in the hippocampus of young PDAPP and Tg2576 mice and its prevention by the ApoE2 genotype. Neurobiol. Dis. 13, 246–253. doi: 10.1016/S0969-9961(03)00079-2
Lee, M., Lee, H.-J., Jeong, Y. J., Oh, S. J., Kang, K. J., Han, S. J., et al. (2019). Age dependency of mGluR5 availability in 5xFAD mice measured by PET. Neurobiol. Aging 84, 208–216. doi: 10.1016/j.neurobiolaging.2019.08.006
Lemere, C. A., Beierschmitt, A., Iglesias, M., Spooner, E. T., Bloom, J. K., Leverone, J. F., et al. (2004). Alzheimer’s disease abeta vaccine reduces central nervous system abeta levels in a non-human primate, the Caribbean vervet. Am. J. Pathol. 165, 283–297. doi: 10.1016/s0002-9440(10)63296-8
Letts, V. A., Beyer, B. J., and Frankel, W. N. (2014). Hidden in plain sight: spike-wave discharges in mouse inbred strains. Genes Brain Behav. 13, 519–526. doi: 10.1111/gbb.12142
Levetiracetam for Alzheimer’s Disease-Associated Epileptiform Activity (2020). Levetiracetam for Alzheimer’s Disease-Associated Epileptiform Activity Natl. Inst. Aging. Available online at: http://www.ni a.nih.gov/alzheimers/clinical-trials/levetiracetam-alzheimers-disease-associated-epileptiform-activity (accessed December 9, 2020).
Li, H.-W., Zhang, L., and Qin, C. (2019). Current state of research on non-human primate models of Alzheimer’s disease. Anim. Model. Exp. Med. 2, 227–238. doi: 10.1002/ame2.12092
Li, N., Liu, K., Qiu, Y., Ren, Z., Dai, R., Deng, Y., et al. (2016). Effect of presenilin mutations on APP cleavage; insights into the pathogenesis of FAD. Front. Aging Neurosci. 8:51. doi: 10.3389/fnagi.2016.00051
Limon, A., Reyes-Ruiz, J. M., and Miledi, R. (2012). Loss of functional GABAA receptors in the Alzheimer diseased brain. Proc. Natl. Acad. Sci. U.S.A. 109, 10071–10076. doi: 10.1073/pnas.1204606109
Lisman, J. (2010). Working memory: the importance of theta and gamma oscillations. Curr. Biol. 20, R490–R492. doi: 10.1016/j.cub.2010.04.011
Lisman, J. E., and Jensen, O. (2013). The theta-gamma neural code. Neuron 77, 1002–1016. doi: 10.1016/j.neuron.2013.03.007
Lisman, J., and Buzsáki, G. (2008). A neural coding scheme formed by the combined function of gamma and theta oscillations. Schizophr. Bull. 34, 974–980. doi: 10.1093/schbul/sbn060
Liu, D., Lu, H., Stein, E., Zhou, Z., Yang, Y., and Mattson, M. P. (2018). Brain regional synchronous activity predicts tauopathy in 3×TgAD mice. Neurobiol. Aging 70, 160–169. doi: 10.1016/j.neurobiolaging.2018.06.016
Luis, E. O., Ortega-Cubero, S., Lamet, I., Razquin, C., Cruchaga, C., Benitez, B. A., et al. (2014). Frontobasal gray matter loss is associated with the TREM2 p.R47H variant. Neurobiol. Aging 35, 2681–2690. doi: 10.1016/j.neurobiolaging.2014.06.007
Lundt, A., Wormuth, C., Siwek, M. E., Müller, R., Ehninger, D., Henseler, C., et al. (2015). EEG radiotelemetry in small laboratory rodents: a powerful state-of-the art approach in neuropsychiatric, neurodegenerative, and epilepsy research. Neural Plast. 2016:e8213878. doi: 10.1155/2016/8213878
Lyou, H. J., Seo, K.-D., Lee, J. E., Pak, H. Y., and Lee, J. H. (2018). Association of Alzheimer’s disease with the risk of developing epilepsy: a 10-year nationwide Cohort study. Dement. Neurocogn. Disord. 17, 156–162. doi: 10.12779/dnd.2018.17.4.156
Mably, A. J., Gereke, B. J., Jones, D. T., and Colgin, L. L. (2017). Impairments in spatial representations and rhythmic coordination of place cells in the 3xTg mouse model of Alzheimer’s disease. Hippocampus 27, 378–392. doi: 10.1002/hipo.22697
Maia, L. F., Kaeser, S. A., Reichwald, J., Hruscha, M., Martus, P., Staufenbiel, M., et al. (2013). Changes in Amyloid-β and Tau in the cerebrospinal fluid of transgenic mice overexpressing amyloid precursor protein. Sci. Transl. Med. 5:194re2. doi: 10.1126/scitranslmed.3006446
Marquardt, K., Sigdel, R., and Brigman, J. L. (2017). Touch-screen visual reversal learning is mediated by value encoding and signal propagation in the orbitofrontal cortex. Neurobiol. Learn. Mem. 139, 179–188. doi: 10.1016/j.nlm.2017.01.006
Mckean, N. E., Handley, R. R., and Snell, R. G. (2021). A review of the current mammalian models of Alzheimer’s disease and challenges that need to be overcome. Int. J. Mol. Sci. 22:13168. doi: 10.3390/ijms222313168
McKhann, G. M., Knopman, D. S., Chertkow, H., Hyman, B. T., Jack, C. R., Kawas, C. H., et al. (2011). The diagnosis of dementia due to Alzheimer’s disease: recommendations from the National Institute on Aging-Alzheimer’s association workgroups on diagnostic guidelines for Alzheimer’s disease. Alzheimers Dement. J. Alzheimers Assoc. 7, 263–269. doi: 10.1016/j.jalz.2011.03.005
McLachlan, D. R. C., Wong, L., Bergeron, C., and Baimbridge, K. G. (1987). Calmodulin and calbindin D28K in Alzheimer disease. Alzheimer Dis. Assoc. Disord. 1, 171–179. doi: 10.1097/00002093-198701030-00009
Mehla, J., Lacoursiere, S. G., Lapointe, V., McNaughton, B. L., Sutherland, R. J., McDonald, R. J., et al. (2019). Age-dependent behavioral and biochemical characterization of single APP knock-in mouse (APPNL-G-F/NL-G-F) model of Alzheimer’s disease. Neurobiol. Aging 75, 25–37. doi: 10.1016/j.neurobiolaging.2018.10.026
Melamed, J. L., de Jesus, F. M., Maior, R. S., and Barros, M. (2017). scopolamine induces deficits in spontaneous object-location recognition and fear-learning in marmoset monkeys. Front. Pharmacol. 8:395. doi: 10.3389/fphar.2017.00395
Molinari, S., Battini, R., Ferrari, S., Pozzi, L., Killcross, A. S., Robbins, T. W., et al. (1996). Deficits in memory and hippocampal long-term potentiation in mice with reduced calbindin D28K expression. Proc. Natl. Acad. Sci. U.S.A. 93, 8028–8033. doi: 10.1073/pnas.93.15.8028
Mondragón-Rodríguez, S., Salas-Gallardo, A., González-Pereyra, P., Macías, M., Ordaz, B., Peña-Ortega, F., et al. (2018b). Phosphorylation of Tau protein correlates with changes in hippocampal theta oscillations and reduces hippocampal excitability in Alzheimer’s model. J. Biol. Chem. 293, 8462–8472. doi: 10.1074/jbc.RA117.001187
Mondragón-Rodríguez, S., Gu, N., Manseau, F., and Williams, S. (2018a). Alzheimer’s transgenic model is characterized by very early brain network alterations and β-CTF fragment accumulation: reversal by β-secretase inhibition. Front. Cell. Neurosci. 12:121. doi: 10.3389/fncel.2018.00121
Montagna, E., Dorostkar, M. M., and Herms, J. (2017). The role of APP in structural spine plasticity. Front. Mol. Neurosci. 10:136. doi: 10.3389/fnmol.2017.00136
Morris, M., Sanchez, P. E., Verret, L., Beagle, A. J., Guo, W., Dubal, D., et al. (2015). Network dysfunction in α−synuclein transgenic mice and human Lewy body dementia. Ann. Clin. Transl. Neurol. 2, 1012–1028. doi: 10.1002/acn3.257
Mucke, L., and Selkoe, D. J. (2012). Neurotoxicity of Amyloid β-protein: synaptic and network dysfunction. Cold Spring Harb. Perspect. Med. 2:a006338. doi: 10.1101/cshperspect.a006338
Mucke, L., Masliah, E., Yu, G.-Q., Mallory, M., Rockenstein, E. M., Tatsuno, G., et al. (2000). High-level neuronal expression of Aβ1–42 in wild-type human amyloid protein precursor transgenic mice: synaptotoxicity without plaque formation. J. Neurosci. 20, 4050–4058. doi: 10.1523/JNEUROSCI.20-11-04050.2000
Muggleton, N. G., Smith, A. J., Scott, E. A. M., Wilson, S. J., and Pearce, P. C. (2005). A long-term study of the effects of diazinon on sleep, the electrocorticogram and cognitive behaviour in common marmosets. J. Psychopharmacol. 19, 455–466. doi: 10.1177/0269881105056521
Mullan, M., Crawford, F., Axelman, K., Houlden, H., Lilius, L., Winblad, B., et al. (1992). A pathogenic mutation for probable Alzheimer’s disease in the APP gene at the N-terminus of beta-amyloid. Nat. Genet. 1, 345–347. doi: 10.1038/ng0892-345
Mullard, A. (2018). BACE failures lower AD expectations, again. Nat. Rev. Drug Discov. 17, 385–385. doi: 10.1038/nrd.2018.94
Najm, R., Jones, E. A., and Huang, Y. (2019). Apolipoprotein E4, inhibitory network dysfunction, and Alzheimer’s disease. Mol. Neurodegener. 14:24. doi: 10.1186/s13024-019-0324-6
Nakazawa, S., Murai, T., Miyauchi, M., Kotani, M., and Ikeda, K. (2015). Behavioral and neurophysiological effects of Ro 10-5824, a dopamine D4 receptor partial agonist, in common marmosets. Psychopharmacology 232, 3287–3295. doi: 10.1007/s00213-015-3978-y
Nakazono, T., Lam, T. N., Patel, A. Y., Kitazawa, M., Saito, T., Saido, T. C., et al. (2017). Impaired in vivo gamma oscillations in the medial entorhinal cortex of knock-in Alzheimer model. Front. Syst. Neurosci. 11:48. doi: 10.3389/fnsys.2017.00048
Novak, P., Schmidt, R., Kontsekova, E., Zilka, N., Kovacech, B., Skrabana, R., et al. (2017). Safety and immunogenicity of the tau vaccine AADvac1 in patients with Alzheimer’s disease: a randomised, double-blind, placebo-controlled, phase 1 trial. Lancet Neurol. 16, 123–134. doi: 10.1016/S1474-4422(16)30331-3
Nunan, J., and Small, D. H. (2000). Regulation of APP cleavage by α-, β- and γ-secretases. FEBS Lett. 483, 6–10. doi: 10.1016/S0014-5793(00)02076-7
Nuriel, T., Angulo, S. L., Khan, U., Ashok, A., Chen, Q., Figueroa, H. Y., et al. (2017). Neuronal hyperactivity due to loss of inhibitory tone in APOE4 mice lacking Alzheimer’s disease-like pathology. Nat. Commun. 8:1464. doi: 10.1038/s41467-017-01444-0
O’Brien, J. L., O’Keefe, K. M., LaViolette, P. S., DeLuca, A. N., Blacker, D., Dickerson, B. C., et al. (2010). Longitudinal fMRI in elderly reveals loss of hippocampal activation with clinical decline. Neurology 74, 1969–1976. doi: 10.1212/WNL.0b013e3181e3966e
O’Leary, T. P., Mantolino, H. M., Stover, K. R., and Brown, R. E. (2020). Age-related deterioration of motor function in male and female 5xFAD mice from 3 to 16 months of age. Genes Brain Behav. 19:e12538. doi: 10.1111/gbb.12538
Oakley, H., Cole, S. L., Logan, S., Maus, E., Shao, P., Craft, J., et al. (2006). Intraneuronal beta-amyloid aggregates, neurodegeneration, and neuron loss in transgenic mice with five familial Alzheimer’s disease mutations: potential factors in amyloid plaque formation. J. Neurosci. Off. J. Soc. Neurosci. 26, 10129–10140. doi: 10.1523/JNEUROSCI.1202-06.2006
Oblak, A. L., Forner, S., Territo, P. R., Sasner, M., Carter, G. W., Howell, G. R., et al. (2020). Model organism development and evaluation for late-onset Alzheimer’s disease: MODEL-AD. Alzheimers Dement. Transl. Res. Clin. Interv. 6:e12110. doi: 10.1002/trc2.12110
Oblak, A. L., Lin, P. B., Kotredes, K. P., Pandey, R. S., Garceau, D., Williams, H. M., et al. (2021). Comprehensive evaluation of the 5XFAD mouse model for preclinical testing applications: a MODEL-AD study. Front. Aging Neurosci. 13:431. doi: 10.3389/fnagi.2021.713726
Oddo, S., Caccamo, A., Shepherd, J. D., Murphy, M. P., Golde, T. E., Kayed, R., et al. (2003). Triple-transgenic model of Alzheimer’s disease with plaques and tangles: intracellular Abeta and synaptic dysfunction. Neuron 39, 409–421. doi: 10.1016/s0896-6273(03)00434-3
Oikawa, N., Kimura, N., and Yanagisawa, K. (2010). Alzheimer-type tau pathology in advanced aged nonhuman primate brains harboring substantial amyloid deposition. Brain Res. 1315, 137–149. doi: 10.1016/j.brainres.2009.12.005
Onos, K. D., Sukoff Rizzo, S. J., Howell, G. R., and Sasner, M. (2016). Toward more predictive genetic mouse models of Alzheimer’s disease. Brain Res. Bull. 122, 1–11. doi: 10.1016/j.brainresbull.2015.12.003
Orr, A. G., Lo, I., Schumacher, H., Ho, K., Gill, M., Guo, W., et al. (2018). Istradefylline reduces memory deficits in aging mice with amyloid pathology. Neurobiol. Dis. 110, 29–36. doi: 10.1016/j.nbd.2017.10.014
Oyelami, T., Bondt, A. D., den Wyngaert, I. V., Hoorde, K. V., Hoskens, L., Shaban, H., et al. (2016). Age-dependent concomitant changes in synaptic dysfunction and GABAergic pathway in the APP/PS1 mouse model. Acta Neurobiol. Exp. (Warsz.) 76, 282–293. doi: 10.21307/ane-2017-027
Palop, J. J., and Mucke, L. (2016). Network abnormalities and interneuron dysfunction in Alzheimer disease. Nat. Rev. Neurosci. 17, 777–792. doi: 10.1038/nrn.2016.141
Pandey, R. S., Graham, L., Uyar, A., Preuss, C., Howell, G. R., and Carter, G. W. (2019). Genetic perturbations of disease risk genes in mice capture transcriptomic signatures of late-onset Alzheimer’s disease. Mol. Neurodegener. 14:50. doi: 10.1186/s13024-019-0351-3
Peters, S. T., Fahrenkopf, A., Choquette, J. M., Vermilyea, S. C., Lee, M. K., and Vossel, K. (2020). Ablating Tau reduces hyperexcitability and moderates electroencephalographic slowing in transgenic mice expressing A53T human α-Synuclein. Front. Neurol. 11:563. doi: 10.3389/fneur.2020.00563
Pratt, L. A., Brody, D. J., and Gu, Q. (2017). Antidepressant Use Among Persons Aged 12 and Over: United States, 2011–2014. NCHS Data Brief, No. 283. Hyattsville, MD: National Center for Health Statistics.
Preuss, C., Pandey, R., Piazza, E., Fine, A., Uyar, A., Perumal, T., et al. (2019). A novel systems biology approach to evaluate mouse models of late-onset Alzheimer’s disease. Neuroscience doi: 10.1101/682856
Priller, C., Bauer, T., Mitteregger, G., Krebs, B., Kretzschmar, H. A., and Herms, J. (2006). Synapse formation and function is modulated by the Amyloid precursor protein. J. Neurosci. 26, 7212–7221. doi: 10.1523/JNEUROSCI.1450-06.2006
Prox, J., Bernreuther, C., Altmeppen, H., Grendel, J., Glatzel, M., D’Hooge, R., et al. (2013). Postnatal disruption of the Disintegrin/metalloproteinase ADAM10 in brain causes epileptic seizures, learning deficits, altered spine morphology, and defective synaptic functions. J. Neurosci. 33, 12915–12928. doi: 10.1523/JNEUROSCI.5910-12.2013
Radde, R., Bolmont, T., Kaeser, S. A., Coomaraswamy, J., Lindau, D., Stoltze, L., et al. (2006). Aβ42-driven cerebral amyloidosis in transgenic mice reveals early and robust pathology. EMBO Rep. 7, 940–946. doi: 10.1038/sj.embor.7400784
Reyes-Marin, K. E., and Nuñez, A. (2017). Seizure susceptibility in the APP/PS1 mouse model of Alzheimer’s disease and relationship with amyloid β plaques. Brain Res. 1677, 93–100. doi: 10.1016/j.brainres.2017.09.026
Richard, B. C., Kurdakova, A., Baches, S., Bayer, T. A., Weggen, S., and Wirths, O. (2015). Gene dosage dependent aggravation of the neurological phenotype in the 5XFAD mouse model of Alzheimer’s disease. J. Alzheimers Dis. 45, 1223–1236. doi: 10.3233/JAD-143120
Riudavets, M. A., Bartoloni, L., Troncoso, J. C., Pletnikova, O., St George-Hyslop, P., Schultz, M., et al. (2013). Familial dementia with frontotemporal features associated with M146V Presenilin-1 mutation. Brain Pathol. Zurich Switz. 23, 595–600. doi: 10.1111/bpa.12051
Rodriguez, G. A., Burns, M. P., Weeber, E. J., and Rebeck, G. W. (2013). Young APOE4 targeted replacement mice exhibit poor spatial learning and memory, with reduced dendritic spine density in the medial entorhinal cortex. Learn. Mem. 20, 256–266. doi: 10.1101/lm.030031.112
Rupp, N. J., Wegenast-Braun, B. M., Radde, R., Calhoun, M. E., and Jucker, M. (2011). Early onset amyloid lesions lead to severe neuritic abnormalities and local, but not global neuron loss in APPPS1 transgenic mice. Neurobiol. Aging 32, 2324.e1–2324.e6. doi: 10.1016/j.neurobiolaging.2010.08.014
Safieh, M., Korczyn, A. D., and Michaelson, D. M. (2019). ApoE4: an emerging therapeutic target for Alzheimer’s disease. BMC Med. 17:64. doi: 10.1186/s12916-019-1299-4
Saganich, M. J., Schroeder, B. E., Galvan, V., Bredesen, D. E., Koo, E. H., and Heinemann, S. F. (2006). Deficits in synaptic transmission and learning in amyloid precursor protein (APP) transgenic mice require C-terminal cleavage of APP. J. Neurosci. 26, 13428–13436. doi: 10.1523/JNEUROSCI.4180-06.2006
Saifullah, M. A. B., Komine, O., Dong, Y., Fukumoto, K., Sobue, A., Endo, F., et al. (2020). Touchscreen-based location discrimination and paired associate learning tasks detect cognitive impairment at an early stage in an App knock-in mouse model of Alzheimer’s disease. Mol. Brain 13:147. doi: 10.1186/s13041-020-00690-6
Saito, T., Matsuba, Y., Mihira, N., Takano, J., Nilsson, P., Itohara, S., et al. (2014). Single App knock-in mouse models of Alzheimer’s disease. Nat. Neurosci. 17, 661–663. doi: 10.1038/nn.3697
Sanchez, P. E., Zhu, L., Verret, L., Vossel, K. A., Orr, A. G., Cirrito, J. R., et al. (2012). Levetiracetam suppresses neuronal network dysfunction and reverses synaptic and cognitive deficits in an Alzheimer’s disease model. Proc. Natl. Acad. Sci. U.S.A. 109, E2895–E2903. doi: 10.1073/pnas.1121081109
Sani, S., Traul, D., Klink, A., Niaraki, N., Gonzalo-Ruiz, A., Wu, C.-K., et al. (2003). Distribution, progression and chemical composition of cortical amyloid-β deposits in aged rhesus monkeys: similarities to the human. Acta Neuropathol. (Berl.) 105, 145–156. doi: 10.1007/s00401-002-0626-5
Sasaki, E., Suemizu, H., Shimada, A., Hanazawa, K., Oiwa, R., Kamioka, M., et al. (2009). Generation of transgenic non-human primates with germline transmission. Nature 459, 523–527. doi: 10.1038/nature08090
Sassi, C., Guerreiro, R., Gibbs, R., Ding, J., Lupton, M. K., Troakes, C., et al. (2014). Exome sequencing identifies 2 novel presenilin 1 mutations (p.L166V and p.S230R) in British early-onset Alzheimer’s disease. Neurobiol. Aging 35, 2422.e13–2422.e16. doi: 10.1016/j.neurobiolaging.2014.04.026
Schauwecker, P. E. (2011). The relevance of individual genetic background and its role in animal models of epilepsy. Epilepsy Res. 97, 1–11. doi: 10.1016/j.eplepsyres.2011.09.005
Schneider, F., Baldauf, K., Wetzel, W., and Reymann, K. G. (2014). Behavioral and EEG changes in male 5xFAD mice. Physiol. Behav. 135, 25–33. doi: 10.1016/j.physbeh.2014.05.041
Seita, Y., Morimura, T., Watanabe, N., Iwatani, C., Tsuchiya, H., Nakamura, S., et al. (2020). Generation of transgenic cynomolgus monkeys overexpressing the gene for Amyloid-β precursor protein. J. Alzheimers Dis. 75, 45–60. doi: 10.3233/JAD-191081
Selkoe, D. J., and Hardy, J. (2016). The amyloid hypothesis of Alzheimer’s disease at 25 years. EMBO Mol. Med. 8, 595–608. doi: 10.15252/emmm.201606210
Serneels, L., Van Biervliet, J., Craessaerts, K., Dejaegere, T., Horré, K., Van Houtvin, T., et al. (2009). γ-Secretase heterogeneity in the Aph1 subunit: relevance for Alzheimer’s disease. Science 324, 639–642. doi: 10.1126/science.1171176
Severino, M., Sivasaravanaparan, M., Olesen, L. Ø, von Linstow, C. U., Metaxas, A., Bouzinova, E. V., et al. (2018). Established amyloid-β pathology is unaffected by chronic treatment with the selective serotonin reuptake inhibitor paroxetine. Alzheimers Dement. N. Y. 4, 215–223. doi: 10.1016/j.trci.2018.04.005
Sevigny, J., Chiao, P., Bussière, T., Weinreb, P. H., Williams, L., Maier, M., et al. (2016). The antibody aducanumab reduces Aβ plaques in Alzheimer’s disease. Nature 537, 50–56. doi: 10.1038/nature19323
Sierksma, A. S. R., de Nijs, L., Hoogland, G., Vanmierlo, T., van Leeuwen, F. W., Rutten, B. P. F., et al. (2016). Fluoxetine treatment induces seizure behavior and premature death in APPswe/PS1dE9 Mice. J. Alzheimers Dis. 51, 677–682. doi: 10.3233/JAD-151066
Silverman, J. L., Nithianantharajah, J., Der-Avakian, A., Young, J. W., and Sukoff Rizzo, S. J. (2020). Lost in translation: at the crossroads of face validity and translational utility of behavioral assays in animal models for the development of therapeutics. Neurosci. Biobehav. Rev. 116, 452–453. doi: 10.1016/j.neubiorev.2020.07.008
Siwek, M. E., Müller, R., Henseler, C., Trog, A., Lundt, A., Wormuth, C., et al. (2015). Altered theta oscillations and aberrant cortical excitatory activity in the 5XFAD model of Alzheimer’s disease. Neural Plast. 2015:781731. doi: 10.1155/2015/781731
Smailovic, U., Koenig, T., Kåreholt, I., Andersson, T., Kramberger, M. G., Winblad, B., et al. (2018). Quantitative EEG power and synchronization correlate with Alzheimer’s disease CSF biomarkers. Neurobiol. Aging 63, 88–95. doi: 10.1016/j.neurobiolaging.2017.11.005
Sperling, R. A., Dickerson, B. C., Pihlajamaki, M., Vannini, P., LaViolette, P. S., Vitolo, O. V., et al. (2010). Functional alterations in memory networks in early Alzheimer’s disease. Neuromol. Med. 12, 27–43. doi: 10.1007/s12017-009-8109-7
Stoiljkovic, M., Kelley, C., Hajós, G. P., Nagy, D., Koenig, G., Leventhal, L., et al. (2016). Hippocampal network dynamics in response to α7 nACh receptors activation in amyloid-β overproducing transgenic mice. Neurobiol. Aging 45, 161–168. doi: 10.1016/j.neurobiolaging.2016.05.021
Stoiljkovic, M., Kelley, C., Horvath, T. L., and Hajós, M. (2018). Neurophysiological signals as predictive translational biomarkers for Alzheimer’s disease treatment: effects of donepezil on neuronal network oscillations in TgF344-AD rats. Alzheimers Res. Ther. 10:105. doi: 10.1186/s13195-018-0433-4
Stoiljkovic, M., Kelley, C., Stutz, B., Horvath, T. L., and Hajós, M. (2019). Altered Cortical and hippocampal excitability in TgF344-AD rats modeling Alzheimer’s disease pathology. Cereb. Cortex N. Y. 1991, 2716–2727. doi: 10.1093/cercor/bhy140
Sukoff Rizzo, S. J., McTighe, S., and McKinzie, D. L. (2020). Genetic background and sex: impact on generalizability of research findings in pharmacology studies. Handb. Exp. Pharmacol. 257, 147–162. doi: 10.1007/164_2019_282
Tescarollo, F. C., Rombo, D. M., DeLiberto, L. K., Fedele, D. E., Alharfoush, E., Tomé, ÂR., et al. (2020). Role of adenosine in epilepsy and seizures. J. Caffeine Adenosine Res. 10, 45–60. doi: 10.1089/caff.2019.0022
Tort, A. B. L., Komorowski, R. W., Manns, J. R., Kopell, N. J., and Eichenbaum, H. (2009). Theta-gamma coupling increases during the learning of item-context associations. Proc. Natl. Acad. Sci. U.S.A. 106, 20942–20947. doi: 10.1073/pnas.0911331106
Uhlmann, R. E., Rother, C., Rasmussen, J., Schelle, J., Bergmann, C., Ullrich Gavilanes, E. M., et al. (2020). Acute targeting of pre-amyloid seeds in transgenic mice reduces Alzheimer-like pathology later in life. Nat. Neurosci. 23, 1580–1588. doi: 10.1038/s41593-020-00737-w
Ulrich, J. D., Ulland, T. K., Colonna, M., and Holtzman, D. M. (2017). Elucidating the role of TREM2 in Alzheimer’s disease. Neuron 94, 237–248. doi: 10.1016/j.neuron.2017.02.042
Uslaner, J. M., Kuduk, S. D., Wittmann, M., Lange, H. S., Fox, S. V., Min, C., et al. (2018). Preclinical to human translational pharmacology of the novel M1 positive allosteric modulator MK-7622. J. Pharmacol. Exp. Ther. 365, 556–566. doi: 10.1124/jpet.117.245894
Van Essen, D. C., Donahue, C. J., Coalson, T. S., Kennedy, H., Hayashi, T., and Glasser, M. F. (2019). Cerebral cortical folding, parcellation, and connectivity in humans, nonhuman primates, and mice. Proc. Natl. Acad. Sci. U.S.A. 116, 26173–26180. doi: 10.1073/pnas.1902299116
Vitek, M. P., Araujo, J. A., Fossel, M., Greenberg, B. D., Howell, G. R., Rizzo, S. J. S., et al. (2021). Translational animal models for Alzheimer’s disease: an Alzheimer’s association business consortium think tank. Alzheimers Dement. 6:e12114. doi: 10.1002/trc2.12114
Vogel, V., Sanchez, C., and Jennum, P. (2002). EEG measurements by means of radiotelemetry after intracerebroventricular (ICV) cannulation in rodents. J. Neurosci. Methods 118, 89–96. doi: 10.1016/S0165-0270(02)00148-6
Vöglein, J., Ricard, I., Noachtar, S., Kukull, W. A., Dieterich, M., Levin, J., et al. (2020). Seizures in Alzheimer’s disease are highly recurrent and associated with a poor disease course. J. Neurol. 267, 2941. doi: 10.1007/s00415-020-09937-7
Vossel, K. A., Beagle, A. J., Rabinovici, G. D., Shu, H., Lee, S. E., Naasan, G., et al. (2013). Seizures and epileptiform activity in the early stages of Alzheimer disease. JAMA Neurol. 70, 1158–1166. doi: 10.1001/jamaneurol.2013.136
Vossel, K. A., Ranasinghe, K. G., Beagle, A. J., Mizuiri, D., Honma, S. M., Dowling, A. F., et al. (2016). Incidence and impact of subclinical epileptiform activity in Alzheimer’s disease. Ann. Neurol. 80, 858–870. doi: 10.1002/ana.24794
Vossel, K. A., Tartaglia, M. C., Nygaard, H. B., Zeman, A. Z., and Miller, B. L. (2017). Epileptic activity in Alzheimer’s disease: causes and clinical relevance. Lancet Neurol. 16, 311–322. doi: 10.1016/S1474-4422(17)30044-3
Vyver, M. V., Bundel, D. D., Nagels, G., Engelborghs, S., and Smolders, I. (2020). 3xTg Alzheimer’s disease mice are more susceptible to induced seizures. Alzheimers Dement. 16:e044096. doi: 10.1002/alz.044096
Watanabe, H., and Shen, J. (2017). Dominant negative mechanism of Presenilin-1 mutations in FAD. Proc. Natl. Acad. Sci. U.S.A. 114, 12635–12637. doi: 10.1073/pnas.1717180114
What Happens to the Brain in Alzheimer’s Disease (2020). What Happens to the Brain in Alzheimer’s Disease?. Available online at: http://www.nia.nih.gov/health/what-happens-brain-alzheimers-disease (accessed November 16, 2020)
WHO (2020). The Top 10 Causes of Death. Available online at: https://www.who.int/news-room/fact-sheets/detail/the-top-10-causes-of-death (accessed November 16, 2020)
World Alzheimer Report (2015). The Global Impact of Dementia: An Analysis of Prevalence, Incidence, Cost and Trends. London: Alzheimer’s Disease International, 87.
Wright, A. L., Zinn, R., Hohensinn, B., Konen, L. M., Beynon, S. B., Tan, R. P., et al. (2013). Neuroinflammation and neuronal loss precede Aβ plaque deposition in the hAPP-J20 mouse model of Alzheimer’s disease. PLoS One 8:e59586. doi: 10.1371/journal.pone.0059586
Xiao, N.-A., Zhang, J., Zhou, M., Wei, Z., Wu, X.-L., Dai, X.-M., et al. (2015). Reduction of glucose metabolism in Olfactory bulb is an earlier Alzheimer’s disease-related biomarker in 5XFAD mice. Chin. Med. J. (Engl.) 128, 2220–2227. doi: 10.4103/0366-6999.162507
Yan, X.-X., Cai, Y., Shelton, J., Deng, S.-H., Luo, X.-G., Oddo, S., et al. (2012). Chronic temporal lobe epilepsy is associated with enhanced Alzheimer-like neuropathology in 3×Tg-AD mice. PLoS One 7:e48782. doi: 10.1371/journal.pone.0048782
Yang, M., Miao, J., Rizak, J., Zhai, R., Wang, Z., Huma, T., et al. (2014). Alzheimer’s Disease and methanol toxicity (Part 2): lessons from four rhesus macaques (Macaca mulatta) chronically fed methanol. J. Alzheimers Dis. 41, 1131–1147. doi: 10.3233/JAD-131532
Yang, P., and Sun, F. (2021). Aducanumab: the first targeted Alzheimer’s therapy. Drug Discov. Ther. 15, 166–168. doi: 10.5582/ddt.2021.01061
You, J. C., Muralidharan, K., Park, J. W., Petrof, I., Pyfer, M. S., Corbett, B. F., et al. (2017). Epigenetic suppression of hippocampal calbindin-D28k by ΔFosB drives seizure-related cognitive deficits. Nat. Med. 23, 1377–1383. doi: 10.1038/nm.4413
Yu, H., Sternad, D., Corcos, D. M., and Vaillancourt, D. E. (2007). Role of hyperactive cerebellum and motor cortex in Parkinson’s disease. Neuroimage 35, 222–233. doi: 10.1016/j.neuroimage.2006.11.047
Zhu, X., Li, X., Zhu, M., Xu, K., Yang, L., Han, B., et al. (2018). Metalloprotease Adam10 suppresses epilepsy through repression of hippocampal neuroinflammation. J. Neuroinflammation 15:221. doi: 10.1186/s12974-018-1260-z
Zhu, X.-C., Tan, L., Wang, H.-F., Jiang, T., Cao, L., Wang, C., et al. (2015). Rate of early onset Alzheimer’s disease: a systematic review and meta-analysis. Ann. Transl. Med. 3:38. doi: 10.3978/j.issn.2305-5839.2015.01.19
Zipser, C. M., Premoli, I., Belardinelli, P., Castellanos, N., Rivolta, D., Heidegger, T., et al. (2018). Cortical excitability and interhemispheric connectivity in early relapsing–remitting multiple sclerosis studied with TMS-EEG. Front. Neurosci. 12:393. doi: 10.3389/fnins.2018.00393
Keywords: EEG, Alzheimer’s disease, animal models, mice, non-human primate, biomarkers
Citation: Cope ZA, Murai T and Sukoff Rizzo SJ (2022) Emerging Electroencephalographic Biomarkers to Improve Preclinical to Clinical Translation in Alzheimer’s Disease. Front. Aging Neurosci. 14:805063. doi: 10.3389/fnagi.2022.805063
Received: 29 October 2021; Accepted: 26 January 2022;
Published: 17 February 2022.
Edited by:
Boon-Seng Wong, Singapore Institute of Technology, SingaporeReviewed by:
Romain Goutagny, UMR 7364 Laboratoire de Neurosciences Cognitives et Adaptatives (LNCA), FranceKarim Fifel, University of Tsukuba, Japan
Ruofan Wang, Tianjin University of Technology and Education, China
Copyright © 2022 Cope, Murai and Sukoff Rizzo. This is an open-access article distributed under the terms of the Creative Commons Attribution License (CC BY). The use, distribution or reproduction in other forums is permitted, provided the original author(s) and the copyright owner(s) are credited and that the original publication in this journal is cited, in accordance with accepted academic practice. No use, distribution or reproduction is permitted which does not comply with these terms.
*Correspondence: Stacey J. Sukoff Rizzo, cml6em9zQHBpdHQuZWR1