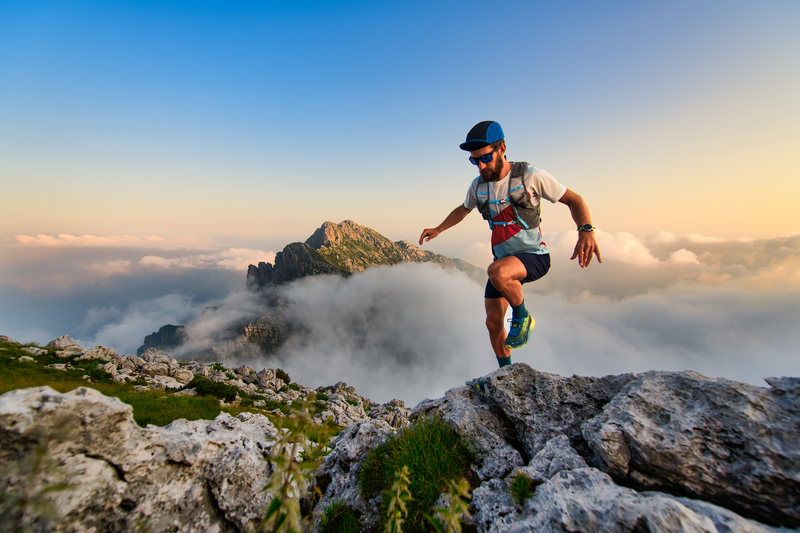
94% of researchers rate our articles as excellent or good
Learn more about the work of our research integrity team to safeguard the quality of each article we publish.
Find out more
ORIGINAL RESEARCH article
Front. Aging Neurosci. , 04 April 2022
Sec. Neurocognitive Aging and Behavior
Volume 14 - 2022 | https://doi.org/10.3389/fnagi.2022.796110
This article is part of the Research Topic Non-pharmacological Interventions in Mild Cognitive Impairment View all 9 articles
Background: Adaptive computerized working memory (WM) training has shown favorable effects on cerebral cortical thickness as compared to non-adaptive training in healthy individuals. However, knowledge of WM training-related morphological changes in mild cognitive impairment (MCI) is limited.
Objective: The primary objective of this double-blind randomized study was to investigate differences in longitudinal cortical thickness trajectories after adaptive and non-adaptive WM training in patients with MCI. We also investigated the genotype effects on cortical thickness trajectories after WM training combining these two training groups using longitudinal structural magnetic resonance imaging (MRI) analysis in Freesurfer.
Method: Magnetic resonance imaging acquisition at 1.5 T were performed at baseline, and after four- and 16-weeks post training. A total of 81 individuals with MCI accepted invitations to undergo 25 training sessions over 5 weeks. Longitudinal Linear Mixed effect models investigated the effect of adaptive vs. non-adaptive WM training. The LME model was fitted for each location (vertex). On all statistical analyzes, a threshold was applied to yield an expected false discovery rate (FDR) of 5%. A secondary LME model investigated the effects of LMX1A and APOE-ε4 on cortical thickness trajectories after WM training.
Results: A total of 62 participants/patients completed the 25 training sessions. Structural MRI showed no group difference between the two training regimes in our MCI patients, contrary to previous reports in cognitively healthy adults. No significant structural cortical changes were found after training, regardless of training type, across all participants. However, LMX1A-AA carriers displayed increased cortical thickness trajectories or lack of decrease in two regions post-training compared to those with LMX1A-GG/GA. No training or training type effects were found in relation to the APOE-ε4 gene variants.
Conclusion: The MCI patients in our study, did not have improved cortical thickness after WM training with either adaptive or non-adaptive training. These results were derived from a heterogeneous population of MCI participants. The lack of changes in the cortical thickness trajectory after WM training may also suggest the lack of atrophy during this follow-up period. Our promising results of increased cortical thickness trajectory, suggesting greater neuroplasticity, in those with LMX1A-AA genotype need to be validated in future trials.
Mild Cognitive Impairment (MCI) describes individuals with reduced cognitive function with age not severe enough to meet the criteria of dementia, but more pronounced than the normal age-related cognitive decline reported in healthy controls (Petersen et al., 1997; Langa and Levine, 2014). Individuals with MCI have a 10-fold higher risk of developing dementia compared to healthy adults at the same age (Petersen et al., 1999; Petersen, 2009). Worldwide, the cost of dementia was estimated to be more than 1 trillion USD in 2020 (Prince et al., 2015). Any treatment that can prevent or delay the conversion from MCI to dementia would thus impact the global economy significantly.
Currently, there is no treatment available for MCI, but studies suggest that cognitive training based on the principles of neuroplasticity may aid in delaying the decline in cognitively unimpaired older adults (Smith et al., 2009; Zelinski et al., 2011; Butler et al., 2018). Repeated cognitive stimulation is thought to improve myelination and increase synaptic density in the brain, thereby improving function (Maas and Angulo, 2021). Adaptive computerized cognitive training, with dynamically increased workloads, was found to be effective in improving untrained cognitive functions utilizing the same neural connections as trained tasks (Klingberg et al., 2002). Effects of training may persist for up to 10 years post intervention (Rebok et al., 2014). Studies focusing on the effect of cognitive training in MCI patients have demonstrated improvement of cognitive functions and transfer effects to non-trained domains (Belleville et al., 2006; Talassi et al., 2007; Flak et al., 2019). However, these few studies showed mixed results, possibly due to the heterogeneous phenotypes within the MCI population with various underlying brain pathologies or co-morbid conditions. In addition, the lack of standardized training methods and outcome measures in the field of cognitive training further complicates comparison across studies.
A pair of recent meta-analytic reviews of efficacy of cognitive intervention in individuals with MCI indicates significant overall effects for intervention content, with memory focused interventions appearing to be more effective than multidomain approaches (Sherman et al., 2017; Zhang et al., 2019a). Since reduced working memory (WM) is prevalent in MCI patients and occurs both in the amnestic (memory impaired) and non-amnestic (memory intact) MCI subtypes (Salthouse and Meinz, 1995), focused WM training may be particularly effective. WM is considered to be a core cognitive function and refers to the temporary maintenance of information that is no longer present in the environment for use in ongoing cognition (Nee and D’Esposito, 2018). WM capacity is dependent on a widespread brain network that includes, but not limited to, the supramarginal gyrus, the dorsolateral prefrontal cortex, and medial prefrontal and lateral parietal cortex and the insular bilaterally (Tomasi et al., 2005, 2007; Rottschy et al., 2012), as shown on functional magnetic resonance imaging (MRI) studies.
Furthermore, structural MRI provides a non-invasive in vivo approach to visualize the brain changes associated with neuroplasticity beyond theories and models. In healthy middle aged and older adults, Engvig et al. (2010) found increased insular thickness after cognitive training. In addition, increased thickness in the right caudal middle frontal cortex and increased volume of the right pallidum were found in healthy adults only after adaptive computerized WM training, but not after non-adaptive training (Metzler-Baddeley et al., 2016). In contrast, Takeuchi et al. (2011) found decreased thickness both in the frontoparietal region and left temporal superior gyrus after an adaptive multiplication task in a group of students, while Lawlor-Savage et al. (2019) reported no quantitative change after a WM task (N-back) training. Only a few morphometry studies of cognitive training were performed in patients with brain pathologies; no changes after training were found in stroke patients (Nyberg et al., 2018), and a meta review from 2018 found small but supporting evidence of structural neuro plasticity in brain-injured patients after training (Caeyenberghs et al., 2018).
Genetic factors may also impact the effect of cognitive training. Specifically, since dopaminergic function plays an important role in WM and other executive functions (Goldman-Rakic, 1996; Salami et al., 2019), polymorphism of the Lim homeobox transcription factor-alpha (LMX1A) gene, which is involved in the maintenance of dopaminergic neurons, was evaluated in relation to WM training (Bellander et al., 2011). Dopaminergic synapses are critical in plasticity (Soderqvist et al., 2012), and reduction in dopaminergic transporters or receptors were related to the effects of aging and cognitive deficits (Chang et al., 2008; Li et al., 2010). Chang et al. (2017) found that functional gain after WM training was greater in patients with HIV-associated neurocognitive disorders who had the LMX1A-AA genotype compared to those with the LMX1A-GG/GA genotypes. Further, Hernes et al. (2021) found that patients with non-amnestic MCI had greater training gains than a group with amnestic MCI, especially in those with the LMX1A-AA genotype.
Another gene that may impact the effects of cognitive training is apolipoprotein epsilon 4 (APOEε4) since having this allele is a potent risk factor for late onset Alzheimer’s disease (Huang and Mucke, 2012). Reduced synaptic plasticity in older adults with APOEε4 carriers (Belleville et al., 2011) may theoretically be related to reduced effect of cognitive training. However, Hernes et al. (2021) found improved WM training gains in MCI patients with the APOEε4 allele, since this allele may demonstrate an antagonistic pleiotropy effect benefiting younger and middle age individuals (Tuminello and Han, 2011; Chang et al., 2016). Similarly, compared to individuals without the APOEε4 allele, the ε4 carriers showed greater compensation, both in magnitude and extent in neuronal activation in the inferior frontal gyrus in the prefrontal cortex during a WM task (Scheller et al., 2017). Nevertheless, APOEε4-carriers with the amnestic type of MCI may not benefit from this allele; in the study by Hernes et al. (2021) amongst the APOEε4-carriers, the amnestic MCI patients showed significant decline in executive function at 16 weeks after the WM training while the non-amnestic MCI patients showed significant improvements. How the APOEε4 allele might impact brain morphometry after WM training is unknown and was explored in the current study.
In this prospective randomized controlled multicenter trial, we investigated the effects of adaptive and non-adaptive WM training on gray matter morphology in individuals with MCI. Based on prior reports, we hypothesized that cortical thickness would increase in regions associated with WM, in particular the prefrontal cortices and the precuneus, after WM training. Furthermore, we explored possible training effects in cortical thickness associated with allelic variations in APOEε4 and LMX1A genotypes through secondary analyzes.
The Norwegian Regional Committee for medical and health research ethics, South-Eastern Health region (no: 2013/410) and the Department of Research at each collaborating hospital approved the study registered in ClinicalTrials.gov (NCT01991405).
This is a multicenter randomized controlled double-blind trial (Flak et al., 2014). Individuals with MCI were recruited from the memory clinics at four hospitals in the South-Eastern Health Region of Norway (Sørlandet Hospital Arendal, Telemark Hospital, Oslo University Hospital, and Diakonhjemmet Hospital). The study period was from August 2013 to December 2016.
A total of 461 individuals were diagnosed at the four centers during the study period; 85 of these individuals consented to participate in the current study. The participants were assessed with neuropsychological tests, questionnaires regarding their risk factors, and MRI of the brain as specified by the Norwegian national guidelines established by the Norwegian register of persons assessed for cognitive symptoms (NorCog). Diagnosis of MCI was made in accordance with the Petersen/Winblad criteria for MCI (Petersen, 2004; Winblad et al., 2004). The Socioeconomic status (SES) was assessed with Hollingshead’s index of education and occupational position, scaled from 1 (low) to 5 (high) (Hollingshead and Redlich, 2007).
The participants underwent neuropsychological assessment and brain MRI as previously described (Flak et al., 2014, 2019). Exclusion criteria included head trauma with a history of post-traumatic brain injury with loss of consciousness, photosensitive epilepsy or any contraindication for MRI (e.g., ferromagnetic metallic implants or severe claustrophobia), use of acetylcholinesterase inhibitors or other antidementia drugs. From the 85 participants enrolled initially, one declined due to MRI contraindications, and two were not willing to travel for the MRI examinations. Eighty-two participants were then randomized for the study; from these participants, 64 completed the training, and 62 had MRI scans from at least two timepoints and were included in the current analysis. Fifty-eight of the 62 participants consented to donate saliva for genetic analysis, but 4 of these participants dropped out of the study and four samples yielded inconclusive results, see Figure 1.
The participants were randomized to either adaptive or non-adaptive cognitive training in accordance with the framework proposed by Simon et al. (2016). The cognitive training was performed at home on the participant’s own computer using the Cogmed WM training program (Klingberg et al., 2005; Klingberg, 2010; Flak et al., 2019). The Cogmed training program consists of several “games” challenging various types of WM. The adaptive training version increases the difficult as the user becomes more skilled, whereas the non-adaptive remains fixed at a low level. The trainers were Cogmed certified, followed the recommended coaching procedures, and monitored the individual participant’s progress continuously via reports from the Cogmed software. During training, all participants received phone calls, at least once a week, to follow up on their progress and to motivate them; the calls were made by one of the researchers who followed the participants’ training through an online secured site. Both intervention groups followed the standard protocol (30–40 min of training per day, 5 days per week for 5 weeks). We considered the training completed if the participants finished 20 or more of 25 training sessions (Flak et al., 2019). The different tasks in the program are described in detail in the Supplementary Material.
Magnetic resonance imaging’s were obtained before the initiation of training at baseline (timepoint 1 = tp1), time from cessation of training to second and third image acquisition were kept at similar time intervals; four ± 0.19 weeks after training (timepoint 2 = tp2), and 16 ± 0.72 weeks after training cessation (timepoint 3 = tp3). The total period of follow-up from baseline were 22 ± 1.24 weeks across all participants. Images were acquired on two Siemens 1.5 T Aera scanners (one located in Sorlandet Hospital, Arendal and one at Oslo University Hospital – Rikshospitalet). The MRI setup was identical at both scanners, with the same versions of 20 channel head and neck coils and software (VE11). The MRI sequences included two three-dimensional magnetization-prepared rapid gradient-echo (3D MP-RAGE) scans (sagittal, echo time 3.47 ms, repetition time 2,400 ms, TI 1,000 ms, flip angle 8 degrees, 1.2 mm resolution covering the whole brain). Two test persons were scanned on both machines to assess systematic errors on brain volumes. The participants were scanned on the same scanner at baseline and at each follow-up timepoint. All images were inspected at the MRI console for motion artifacts or other artifacts before the patients were discharged from the MRI facility. Scans with visible motion artifacts were repeated within the same MRI session (Reuter et al., 2015). However, one scan at timepoint 2 and 2 scans at timepoint 3 were discarded due to excessive head motion.
Images were converted from DCM image format to Nifti format by the DCM2NIX software.1 The FreeSurfer V.6.02 longitudinal processing pathway was used on a small HT condor3 computer cluster consisting of three AMD Ryzen© 1800x/1700x CPU equipped workstations, and one AMD Threadripper© 1950x CPU equipped workstation all with ECC memory correction. Cortical reconstruction and segmentation were conducted within the FreeSurfer software suite as described in previous publications (Segonne et al., 2004; Reuter et al., 2010; Reuter and Fischl, 2011).
The longitudinal pathway utilized all the available images for each patient. Twelve participants only had baseline images (tp1) and images 4 weeks after cessation of training (tp2), and 51 participants had MRI images from all three timepoints.
We inspected each scan for skull stripping failure as well as other known errors. The resulting longitudinal cortical thickness parameter was chosen, as it was considered the most reliable for being a surrogate biomarker (Winkler et al., 2018) for a potential WM training effect. Surface maps were resampled to the fsaverage sample provided with Freesurfer, and smoothed with the standard 2D Gaussian smoothing kernel at a value of 30 mm (fwhm) supplied with Freesurfer, as part of the postprocessing procedure, before statistical analysis. Furthermore, the rationale behind the smoothing was to minimize false-positive while still retaining maximum statistical power and counteract some of the anatomical differences between the subjects that the registration process didn’t adapt perfectly and decrease inter-subject variability (Lerch and Evans, 2005; Stelzer et al., 2014; Zeighami and Evans, 2021).
Saliva for genotyping was harvested in Oragene Self collection Kit (DAN Genoteck, Inc., Ottawa, ON, Canada) from the participants at study enrolment. Genomic DNA was analyzed with Restriction Fragment Length Polymorphism (RFLP-PCR) for genotype analyzes of APOEε (rs429358 and rs7412) and LMX1A (rs4657412), as reported previously (Hernes et al., 2021).
The sample size was calculated on the primary outcome: any group difference in the cortical thickness trajectories. Cohen’s effect size was used to calculate the number of patients for inclusion. To obtain a strong medium effect with Cohen’s effect size of 0.6, approximately 45 patients in either group were needed.
Longitudinal cortical data were analyzed in MATLAB (Mathworks, version 2016a) using a spatiotemporal linear mixed effects model (LME) module supplied with the Freesurfer software. The LME model was fitted for each location (vertex) of the cortical surface (Bernal-Rusiel et al., 2013). To adjust for multiple comparisons, the two p-maps from left and right hemispheres were combined to give equal threshold for both hemispheres. On all statistical analyzes, a threshold was applied to yield an expected false discovery rate (FDR) of 5% (Benjamini et al., 2006) across both hemispheres, to correct for multiple comparisons and prevent false positive results.
First, in order to investigate whether the two groups had similar brain morphometry trajectories over time, a LME model was fitted with cortical thickness as the dependent variable and time (months since first scan), sex, training type (adaptive or non-adaptive), age (at baseline), scanner site (1 at Arendal and 2 at Oslo), and interaction (time × training type) as independent variables. We also used intecept as random factor in all our LME models (Supplementary Table 1).
Cohen’s D for effect size maps were created using a GLM model fitted for each location (vertex), creating maps of the training types’ effect size in a MATLAB model for each timepoint, with the same variables as with the LME model without interaction and random factor.
Secondary analyzes of genotype effects on cortical thickness were conducted on the 50 participants with valid genotype data. Since the two training types showed no group differences on cortical thickness trajectories, the analysis was performed by combining both training types into one group, as we had done in previous analyzes (Hernes et al., 2021). To investigate a possible influence of the LMX1A genotype, an LME model was fitted with cortical thickness as dependent variable and time, gender, training type, age, study site, LMX1A (AA vs. GG/GA variant), and interaction (time × LMX1A genotype) as independent variables, and intercept as random factor. Since only two participants had the GG alleles in the LMX1A group, they were combined with the AG alleles group.
To investigate a possible influence of the APOEε gene variants on cortical thickness trajectories, a LME model was fitted with cortical thickness as a dependent variable and time, sex, training type, age, scanner site, presence of APOEε4 allele (ε2/ε2, ε2/ε3, or ε3/ε3 versus ε2/ε4, ε3/ε4 or ε4/ε4), and interaction (time × APOEε gene variants) as independent variables (Chang et al., 2017; Hernes et al., 2021).
No significant baseline group differences between the two training groups were found for age, sex, socioeconomic status, years of education and full-scale IQ (Table 1). By chance, more of the participants with the LMX1A-AA genotype were enrolled in the adaptive training group than the non-adaptive training group.
Table 1. Clinical characteristics for the adaptive and non-adaptive training groups including genome.
On a linear mixed effects model (Supplementary Table 1), no significant differences were found between the adaptive and non-adaptive training groups in the longitudinal cortical thickness trajectories below the established threshold for FDR correction of 0.000084. The averaged cortical thickness maps for each training group, together with mean cortical thickness difference maps are shown in Figure 2.
Figure 2. Top panels: Average cortical thickness maps of adaptive and non-adaptive training group, with mean differences from all three timepoints. Timepoint 1: Baseline, timepoint 2: 4 weeks after training cessation. Timepoint 3: 16 weeks after training cessation. Bottom panel: Cortical thickness analysis of training effect independent of training type (time, uncorrected), show no significant region after FDR correction (significance threshold of log10-p: 2.76 = 0.0017). Resampled surface maps, were smoothed with kernel factor of 30 mm (fwhm).
Furthermore, no significant group differences were found when assessing the main effect of time without the interaction term when using a groupwise comparison. Finally, no significant cortical thickness change was found over time when combining the two training type groups. Uncorrected effect sizes expressing longitudinal thickness change between different timepoints in both groups separately are visualized in together with uncorrected cortical trajectory maps in Figure 3.
Figure 3. Top panels: Effect maps (Cohen’s D) illustrating training type group differences at each timepoint for the left and right hemispheres. Bottom row: Cortical thickness trajectory maps showing the interaction effect (Time × Training type, uncorrected). These interaction effects were no longer significant after FDR correction. Resampled surface maps, were smoothed with kernel factor of 30 mm (fwhm).
In the LME model with the Time*LMX1A interaction, significant differences between the AA and GG/GA carriers were found after FDR correction (corrected threshold 0.00043), which are visualized in Figure 4. Significant clusters of increased cortical thickness trajectory were found in the right superior frontal gyrus, in the AA carriers compared to the GG/GA carriers. In the left hemisphere, the AA carriers showed no different trajectories compared to GG/GA carriers. The mean cortical thickness at each timepoints, and the p-values for the interaction effects for the significant clusters per region, and the size of the significant clusters in each region are included in Table 2. No other brain regions showed significant interaction effects, see Figure 4.
Figure 4. Regions with significant Time x LMX1A (AA vs. GA and GG) interaction on cortical thickness after FDR correction of the P-value, minimal significant FDR corrected P threshold is 10(– 2.58) = 0.00043. Resampled surface maps, were smoothed with kernel factor of 30 mm (fwhm). Brain regions showing significant group differences (after FDR correction) in the right hemisphere include the superior frontal region, and paracentral region. In the left hemisphere, there was no significant group difference in any region.
Table 2. Cortical thickness (Mean(±SE)) of regions of interest that show training LMX1A effects, with cluster size of significant vertices.
The LME with Time*APOEε4 interaction did not show significant differences in cortical thickness trajectories between the APOEε4 carriers and non-carriers after FDR correction (corrected threshold 0.000126).
This study has the following major findings. Contrary to our hypothesis, cortical thickness did not show significant increase 6 months after WM training in our MCI participants. In addition, the cortical thickness changes were not different between the adaptive and non-adaptive computerized WM training groups. However, a subgroup of MCI participants, those with the LMX1A-AA genotype, showed significant increase or lack of decrease of cortical thickness in the right frontal superior region and right paracentral region across WM training groups compared to the GG group. No significant difference in the cortical thickness trajectories after WM training was found between carriers of APOEε4 and non-carriers.
Few studies of patients with MCI investigated quantitative changes in brain morphology after cognitive training (Belleville and Bherer, 2012). A recent study by Zhang et al. (2019b) reported a significant correlation between gray matter volume trajectory of the right angular gyrus and the immediate recall component of Hopkins Verbal Learning Test-Revised (HVLT-R) after a multidomain training program in individuals with amnestic MCI. The study is limited by a small sample (n = 12) and a non-RCT design. Two studies that evaluated cognitively healthy adults also reported improved cortical thickness after adaptive WM training, but not in those that had non-adaptive training (Metzler-Baddeley et al., 2016; Wu et al., 2021). In contrast, the present study of participants with MCI did not find significant differences in cortical thickness changes between these two types of training. This lack of training type difference on the cortical thickness might have resulted from similar low difficulty levels between the adaptive and non-adaptive training in these MCI patients, that both groups reached a ceiling with regard to the training effect. Currently optimal training time for MCI patients is unknown. Edmonds et al. (2020) reported a 0.05 mm annual atrophy rate in the temporal lobes in individuals with MCI. In our study, no change in the cortical thickness trajectories were observed, which might suggest a lack of decline in cortical thickness after working memory training (WMT). This needs to be explored in further studies.
The lack of group difference on cortical thickness after the two training types may also be due to the study participants’ older age and the heterogeneous etiologies for their MCI (Flak et al., 2019). Therefore, they had limited compensatory processes caused by underlying brain pathologies that might have diminished the training effects on cortical thickness. We speculate that the accumulated degenerative processes in the brains of older MCI individuals might have induced different and less optimal neuroplasticity mechanisms than those present in younger “healthier brains,” leading to the lack of cortical thickness changes after WM training. Some support for this explanation is found in previous reports on task-activated fMRI with greater activity in compensatory brain regions in older adults (Belleville and Bherer, 2012), suggesting a redistribution of the training effects to more widespread brain regions as compared to a more localized effect in younger individuals (Hampstead et al., 2012; Simon et al., 2020).
We further explored the possible genotypic contributions on cortical trajectory changes after WM training since two genotype variants were shown to influence the WM training effects on cognitive outcomes (Hernes et al., 2021). The LMX1A gene is involved in the maintenance of dopaminergic neurons, and dopamine is essential for WM function (Puig et al., 2014). Regardless of the WM training type, MCI participants with the LMX1A-AA genotype showed significantly increased cortical thickness trajectories after WM training in brain regions associated with WM function. Only one previous longitudinal study has evaluated the effects of WM training on the brain in individuals with the LMX1A-AA genotype; Chang et al. (2017) reported decreased BOLD activation on a 2-back fMRI task in those with the AA genotype, suggesting improved neural efficiency, but no change or increased activation in those with GG/GA genotype in the middle frontal gyrus at 1-month after the same WM training. A significant increase in cortical thickness trajectories was observed in the right superior frontal region and continuing over into the paracentral region medially in MCI participants with the LMX1A-AA genotype, but not in participants with the GG/GA genotypes. The right superior frontal region is associated with WM functions. Nissim et al. (2016), and a meta review concluded that the superior frontal region was especially sensitive to spatial content (Nee et al., 2012), and right paracentral gyrus was recruited during WM tasks to compensate for having a poor night’s sleep by recruiting the necessary resources to complete the task in a recent neural network study (Lauharatanahirun et al., 2020). Suggesting that it can be involved as a secondary center in WM. Our reported selective increase in cortical thickness trajectory after WM training only in those with the LMX1A-AA genotype together with previously published results of increased WM function tests after WM training from the Memory Aid study, suggest that carriers of individuals with LMX1A-AA in particular might benefit more from WM training (Hernes et al., 2021) than GG/GA carriers. However, future studies that evaluate this genotypic variant on brain morphometry with a larger sample size are needed.
There were no differences in cortical thickness trajectories among our participant with or without the APOE-ε4 allele after WM training. In our previous reports from the same population, APOEε4 carriers improved in some cognitive tests after WM training as compared to non-carriers (Hernes et al., 2021); therefore, we had expected differential trajectories in cortical thickness changes after WM training. Nevertheless, this negative result should be interpreted with caution due to the relatively small sample size, and further studies with larger sample sizes are needed. To our knowledge, this is the first study that has evaluated the impact of genotypes on brain morphometry after WM training.
This study has several strengths. This is a longitudinal follow-up study in a cohort of participants with MCI, which allowed intra-subject assessment of the possible structural brain changes after WM training. Each participant was scanned on the same MRI machines for the baseline and follow-up scans using the identical imaging protocol, thereby minimizing possible variabilities from image contrast, signal-to-noise ratio, contrast-to-noise ratio, intensity non-uniformity or geometric distortion (Lee et al., 2019). Despite these strengths, a limitation to this study is the relatively small sample size for the subgroups, both for comparing the morphological changes between the adaptive and non-adaptive training group, and the comparisons between the morphometric trajectories of the participants with different genotypes. Therefore, these findings should be viewed as preliminary. Furthermore, the manual skull removal that was required for some of the MRI scans might have introduced subjectivity in the early steps of the image processing. Lastly, we also did not evaluate a cognitively healthy control group to determine whether the training effects on cortical thickness might be different between participants with MCI and cognitively healthy individuals. Furthermore the study didn’t include a passive MCI control group, this is in accordance with the framework proposed by Simons et al. (2016) to ensure high-quality computer-based WM training studies.
The current results from WM training in a heterogeneous population of MCI participants identified the need for further research, especially with respect to genotypic variations in brain neuroplasticity. Promising results of greater neuroplasticity in LMX1A-AA carriers should be further investigated in future trials since these individuals may benefit the most from WM training.
The datasets presented in this article are not readily available because the Norwegian Regional Committee for medical and health research ethics limit data sharing, and de-identified data can only be shared after an application process. The study protocol is publicly available. The statistical analysis plan is available upon request by members of the academic community for the next five years. The generated datasets are available by request to the corresponding author, though no imagesets can be shared, the completed freesurfer data can be requested. Requests to access the datasets should be directed to HH, aGFha29uLnJhbXNsYW5kLmhvbEBvdXMtaGYubm8=.
The studies involving human participants were reviewed and approved by the Norwegian Regional Committee for medical and health research ethics, South-Eastern region (2013/410) approved the study (clinicaltrials.gov NCT01991405). The patients/participants provided their written informed consent to participate in this study.
SH, MF, GL, JS, and LC conceptualized and designed the study. MF, GL, AE, B-OM, and HH collected the data. HH, MF, SH, and LC analyzed the data. SH, HH, KB, LR, and LC interpreted the data. HH, SH, KB, AE, LR, and LC drafted the manuscript. All authors have critically revised the article and approved the final version of manuscript to be published.
This study was funded by research grants from the South Eastern Norway Regional Health Authority (Grant Nos: 2015046 and 2013059), and Sørlandet Hospital HF. Chang’s effort was supported by the University of Maryland, School of Medicine, and the John A. Burns School of Medicine at the University of Hawai‘i at Manoa.
The authors declare that the research was conducted in the absence of any commercial or financial relationships that could be construed as a potential conflict of interest.
All claims expressed in this article are solely those of the authors and do not necessarily represent those of their affiliated organizations, or those of the publisher, the editors and the reviewers. Any product that may be evaluated in this article, or claim that may be made by its manufacturer, is not guaranteed or endorsed by the publisher.
We thank radiographer Marianne Rognli, Sørlandet Sykehus – Arendal, and senior radiographer Anders Tomtestad, Oslo University Hospital – Rikshospitalet, for their help in managing the participants during scans. We thank the study participants for their interest and cooperation in the project, and acknowledge the Norwegian Registry for persons being evaluated for cognitive symptoms in specialized care (NorCog) for patient data. We also thank Sørlandet Hospital, Telemark hospital, University of Maryland, School of Medicine and Oslo University Hospital - Rikshospitalet, Norway for their assistance in this project.
The Supplementary Material for this article can be found online at: https://www.frontiersin.org/articles/10.3389/fnagi.2022.796110/full#supplementary-material
Bellander, M., Brehmer, Y., Westerberg, H., Karlsson, S., Furth, D., Bergman, O., et al. (2011). Preliminary evidence that allelic variation in the LMX1A gene influences training-related working memory improvement. Neuropsychologia 49, 1938–1942. doi: 10.1016/j.neuropsychologia.2011.03.021
Belleville, S., and Bherer, L. (2012). Biomarkers of cognitive training effects in aging. Curr. Transl. Geriatr. Exp. Gerontol. Rep. 1, 104–110. doi: 10.1007/s13670-012-0014-5
Belleville, S., Clement, F., Mellah, S., Gilbert, B., Fontaine, F., and Gauthier, S. (2011). Training-related brain plasticity in subjects at risk of developing Alzheimer’s disease. Brain 134, 1623–1634. doi: 10.1093/brain/awr037
Belleville, S., Gilbert, B., Fontaine, F., Gagnon, L., Menard, E., and Gauthier, S. (2006). Improvement of episodic memory in persons with mild cognitive impairment and healthy older adults: evidence from a cognitive intervention program. Dement. Geriatr. Cogn. Disord. 22, 486–499. doi: 10.1159/000096316
Benjamini, Y., Krieger, A. M., and Yekutieli, D. (2006). Adaptive linear step-up procedures that control the false discovery rate. Biometrika 93, 491–507. doi: 10.1093/biomet/93.3.491
Bernal-Rusiel, J. L., Reuter, M., Greve, D. N., Fischl, B., Sabuncu, M. R., Alzheimer’s Disease Neuroimaging Initiative (2013). Spatiotemporal linear mixed effects modeling for the mass-univariate analysis of longitudinal neuroimage data. Neuroimage 81, 358–370. doi: 10.1016/j.neuroimage.2013.05.049
Butler, M., McCreedy, E., Nelson, V. A., Desai, P., Ratner, E., Fink, H. A., et al. (2018). Does cognitive training prevent cognitive decline: a systematic review. Ann. Intern. Med. 168, 63–68. doi: 10.7326/M17-1531
Caeyenberghs, K., Clemente, A., Imms, P., Egan, G., Hocking, D. R., Leemans, A., et al. (2018). Evidence for training-dependent structural neuroplasticity in brain-injured patients: a critical review. Neurorehabil. Neural Repair 32, 99–114. doi: 10.1177/1545968317753076
Chang, L., Douet, V., Bloss, C., Lee, K., Pritchett, A., Jernigan, T. L., et al. (2016). Gray matter maturation and cognition in children with different APOE epsilon genotypes. Neurology 87, 585–594. doi: 10.1212/WNL.0000000000002939
Chang, L., Lohaugen, G. C., Andres, T., Jiang, C. S., Douet, V., Tanizaki, N., et al. (2017). Adaptive working memory training improved brain function in human immunodeficiency virus-seropositive patients. Ann.Neurol. 81, 17–34. doi: 10.1002/ana.24805
Chang, L., Wang, G. J., Volkow, N. D., Ernst, T., Telang, F., Logan, J., et al. (2008). Decreased brain dopamine transporters are related to cognitive deficits in HIV patients with or without cocaine abuse. Neuroimage 42, 869–878. doi: 10.1016/j.neuroimage.2008.05.011
Edmonds, E. C., Weigand, A. J., Hatton, S. N., Marshall, A. J., Thomas, K. R., Ayala, D. A., et al. (2020). Patterns of longitudinal cortical atrophy over 3 years in empirically derived MCI subtypes. Neurology 94, e2532–e2544. doi: 10.1212/WNL.0000000000009462
Engvig, A., Fjell, A. M., Westlye, L. T., Moberget, T., Sundseth, O., Larsen, V. A., et al. (2010). Effects of memory training on cortical thickness in the elderly. Neuroimage 52, 1667–1676. doi: 10.1016/j.neuroimage.2010.05.041
Flak, M. M., Hernes, S. S., Chang, L., Ernst, T., Douet, V., Skranes, J., et al. (2014). The memory aid study: protocol for a randomized controlled clinical trial evaluating the effect of computer-based working memory training in elderly patients with mild cognitive impairment (MCI). Trials 15:156.
Flak, M. M., Hol, H. R., Hernes, S. S., Chang, L., Engvig, A., Bjuland, K. J., et al. (2019). Adaptive computerized working memory training in patients with mild cognitive impairment. A randomized double-blind active controlled trial. Front. Psychol. 10:807. doi: 10.3389/fpsyg.2019.00807
Goldman-Rakic, P. S. (1996). Regional and cellular fractionation of working memory. Proc. Natl. Acad. Sci. U.S.A. 93, 13473–13480. doi: 10.1073/pnas.93.24.13473
Hampstead, B. M., Stringer, A. Y., Stilla, R. F., Giddens, M., and Sathian, K. (2012). Mnemonic strategy training partially restores hippocampal activity in patients with mild cognitive impairment. Hippocampus 22, 1652–1658. doi: 10.1002/hipo.22006
Hernes, S. S., Flak, M. M., Lohaugen, G. C. C., Skranes, J., Hol, H. R., Madsen, B. O., et al. (2021). Working memory training in amnestic and Non-amnestic patients with mild cognitive impairment: preliminary findings from genotype variants on training effects. Front. Aging Neurosci. 13:624253. doi: 10.3389/fnagi.2021.624253
Hollingshead, A. B., and Redlich, F. C. (2007). Social class and mental illness: a community study. 1958. Am. J. Public Health 97, 1756–1757. doi: 10.2105/ajph.97.10.1756
Huang, Y., and Mucke, L. (2012). Alzheimer mechanisms and therapeutic strategies. Cell 148, 1204–1222. doi: 10.1016/j.cell.2012.02.040
Klingberg, T. (2010). Training and plasticity of working memory. Trends Cogn. Sci. 14, 317–324. doi: 10.1016/j.tics.2010.05.002
Klingberg, T., Fernell, E., Olesen, P. J., Johnson, M., Gustafsson, P., Dahlstrom, K., et al. (2005). Computerized training of working memory in children with ADHD–a randomized, controlled trial. J. Am. Acad. Child Adolesc. Psychiatry 44, 177–186.
Klingberg, T., Forssberg, H., and Westerberg, H. (2002). Training of working memory in children with ADHD. J. Clin. Exp. Neuropsychol. 24, 781–791.
Langa, K. M., and Levine, D. A. (2014). The diagnosis and management of mild cognitive impairment: a clinical review. JAMA 312, 2551–2561. doi: 10.1001/jama.2014.13806
Lauharatanahirun, N., Bansal, K., Thurman, S. M., Vettel, J. M., Giesbrecht, B., Grafton, S. T., et al. (2020). Flexibility of brain regions during working memory curtails cognitive consequences to lack of sleep. arXiv Neurons Cogn. [Preprint]. arXiv:2009.07233,
Lawlor-Savage, L., Clark, C. M., and Goghari, V. M. (2019). No evidence that working memory training alters gray matter structure: a MRI surface -based analysis. Behav. Brain Res. 360, 323–340. doi: 10.1016/j.bbr.2018.12.008
Lee, H., Nakamura, K., Narayanan, S., Brown, R. A., Arnold, D. L., and Alzheimer’s Disease Neuroimaging Initiative (2019). Estimating and accounting for the effect of MRI scanner changes on longitudinal whole-brain volume change measurements. Neuroimage 184, 555–565. doi: 10.1016/j.neuroimage.2018.09.062
Lerch, J. P., and Evans, A. C. (2005). Cortical thickness analysis examined through power analysis and a population simulation. Neuroimage 24, 163–173. doi: 10.1016/j.neuroimage.2004.07.045
Li, S. C., Lindenberger, U., and Backman, L. (2010). Dopaminergic modulation of cognition across the life span. Neurosci. Biobehav. Rev. 34, 625–630. doi: 10.1016/j.neubiorev.2010.02.003
Maas, D. A., and Angulo, M. C. (2021). Can enhancing neuronal activity improve myelin repair in multiple sclerosis? Front. Cell Neurosci. 15:645240. doi: 10.3389/fncel.2021.645240
Metzler-Baddeley, C., Caeyenberghs, K., Foley, S., and Jones, D. K. (2016). Task complexity and location specific changes of cortical thickness in executive and salience networks after working memory training. Neuroimage 130, 48–62.
Nee, D. E., and D’Esposito, M. (2018). The representational basis of working memory. Curr. Top. Behav. Neurosci. 37, 213–230. doi: 10.1007/7854_2016_456
Nee, D. E., Brown, J. W., Askren, M. K., Berman, M. G., Demiralp, E., Krawitz, A., et al. (2012). A meta-analysis of executive components of working memory. Cereb. Cortex 23, 264–282. doi: 10.1093/cercor/bhs007
Nissim, N. R., O’Shea, A. M., Bryant, V., Porges, E. C., Cohen, R., and Woods, A. J. (2016). Frontal structural neural correlates of working memory performance in older adults. Front. Aging Neurosci. 8:328. doi: 10.3389/fnagi.2016.00328
Nyberg, C. K., Nordvik, J. E., Becker, F., Rohani, D. A., Sederevicius, D., Fjell, A. M., et al. (2018). A longitudinal study of computerized cognitive training in stroke patients - effects on cognitive function and white matter. Top. Stroke Rehabil. 25, 241–247. doi: 10.1080/10749357.2018.1443570
Petersen, R. C. (2004). Mild cognitive impairment as a diagnostic entity. J. Intern. Med. 256, 183–194.
Petersen, R. C. (2009). Early diagnosis of Alzheimer’s disease: is MCI too late? Curr. Alzheimer Res. 6, 324–330. doi: 10.2174/156720509788929237
Petersen, R. C., Smith, G. E., Waring, S. C., Ivnik, R. J., Kokmen, E., and Tangelos, E. G. (1997). Aging, memory, and mild cognitive impairment. Int. Psychogeriatr. 9(Suppl. 1), 65–69. doi: 10.1017/s1041610297004717
Petersen, R. C., Smith, G. E., Waring, S. C., Ivnik, R. J., Tangalos, E. G., and Kokmen, E. (1999). Mild cognitive impairment: clinical characterization and outcome. Arch. Neurol. 56, 303–308. doi: 10.1001/archneur.56.3.303
Prince, M. J., Wu, F., Guo, Y., Gutierrez Robledo, L. M., O’Donnell, M., Sullivan, R., et al. (2015). The burden of disease in older people and implications for health policy and practice. Lancet 385, 549–562. doi: 10.1016/S0140-6736(14)61347-7
Puig, M. V., Rose, J., Schmidt, R., and Freund, N. (2014). Dopamine modulation of learning and memory in the prefrontal cortex: insights from studies in primates, rodents, and birds. Front. Neural Circuits 8:93. doi: 10.3389/fncir.2014.00093
Rebok, G. W., Ball, K., Guey, L. T., Jones, R. N., Kim, H. Y., King, J. W., et al. (2014). Ten-year effects of the advanced cognitive training for independent and vital elderly cognitive training trial on cognition and everyday functioning in older adults. J. Am. Geriatr. Soc. 62, 16–24. doi: 10.1111/jgs.12607
Reuter, M., and Fischl, B. (2011). Avoiding asymmetry-induced bias in longitudinal image processing. Neuroimage 57, 19–21. doi: 10.1016/j.neuroimage.2011.02.076
Reuter, M., Rosas, H. D., and Fischl, B. (2010). Highly accurate inverse consistent registration: a robust approach. Neuroimage 53, 1181–1196. doi: 10.1016/j.neuroimage.2010.07.020
Reuter, M., Tisdall, M. D., Qureshi, A., Buckner, R. L., van der Kouwe, A. J. W., and Fischl, B. (2015). Head motion during MRI acquisition reduces gray matter volume and thickness estimates. Neuroimage 107, 107–115. doi: 10.1016/j.neuroimage.2014.12.006
Rottschy, C., Langner, R., Dogan, I., Reetz, K., Laird, A. R., Schulz, J. B., et al. (2012). Modelling neural correlates of working memory: a coordinate-based meta-analysis. Neuroimage 60, 830–846. doi: 10.1016/j.neuroimage.2011.11.050
Salami, A., Garrett, D. D., Wahlin, A., Rieckmann, A., Papenberg, G., Karalija, N., et al. (2019). Dopamine D2/3 binding potential modulates neural signatures of working memory in a load-dependent fashion. J. Neurosci. 39, 537–547. doi: 10.1523/JNEUROSCI.1493-18.2018
Salthouse, T. A., and Meinz, E. J. (1995). Aging, inhibition, working memory, and speed. J. Gerontol. B Psychol. Sci. Soc. Sci. 50, 297–306.
Scheller, E., Peter, J., Schumacher, L. V., Lahr, J., Mader, I., Kaller, C. P., et al. (2017). APOE moderates compensatory recruitment of neuronal resources during working memory processing in healthy older adults. Neurobiol. Aging 56, 127–137. doi: 10.1016/j.neurobiolaging.2017.04.015
Segonne, F., Dale, A. M., Busa, E., Glessner, M., Salat, D., Hahn, H. K., et al. (2004). A hybrid approach to the skull stripping problem in MRI. Neuroimage 22, 1060–1075. doi: 10.1016/j.neuroimage.2004.03.032
Sherman, D. S., Mauser, J., Nuno, M., and Sherzai, D. (2017). The efficacy of cognitive intervention in mild cognitive impairment (MCI): a meta-analysis of outcomes on neuropsychological measures. Neuropsychol. Rev. 27, 440–484. doi: 10.1007/s11065-017-9363-3
Simon, S. S., Hampstead, B. M., Nucci, M. P., Duran, F. L. S., Fonseca, L. M., Martin, M. D. G. M., et al. (2020). Training gains and transfer effects after mnemonic strategy training in mild cognitive impairment: a fMRI study. Int. J. Psychophysiol. 154, 15–26. doi: 10.1016/j.ijpsycho.2019.03.014
Simon, S. S., Tusch, E. S., Holcomb, P. J., and Daffner, K. R. (2016). Increasing working memory load reduces processing of cross-modal task-irrelevant stimuli even after controlling for task difficulty and executive capacity. Front. Hum. Neurosci. 10:380. doi: 10.3389/fnhum.2016.00380
Simons, D. J., Boot, W. R., Charness, N., Gathercole, S. E., Chabris, C. F., Hambrick, D. Z., et al. (2016). Do “Brain-Training” programs work? Psychol. Sci. Public Interest 17, 103–186. doi: 10.1177/1529100616661983
Smith, G. E., Housen, P., Yaffe, K., Ruff, R., Kennison, R. F., Mahncke, H. W., et al. (2009). A cognitive training program based on principles of brain plasticity: results from the improvement in memory with plasticity-based adaptive cognitive training (IMPACT) study. J. Am. Geriatr. Soc. 57, 594–603. doi: 10.1111/j.1532-5415.2008.02167.x
Soderqvist, S., Bergman Nutley, S., Peyrard-Janvid, M., Matsson, H., Humphreys, K., Kere, J., et al. (2012). Dopamine, working memory, and training induced plasticity: implications for developmental research. Dev. Psychol. 48, 836–843. doi: 10.1037/a0026179
Stelzer, J., Lohmann, G., Mueller, K., Buschmann, T., and Turner, R. (2014). Deficient approaches to human neuroimaging. Front Hum Neurosci 8:462. doi: 10.3389/fnhum.2014.00462
Takeuchi, H., Taki, Y., Hashizume, H., Sassa, Y., Nagase, T., Nouchi, R., et al. (2011). Effects of training of processing speed on neural systems. J. Neurosci. 31, 12139–12148. doi: 10.1523/jneurosci.2948-11.2011
Talassi, E., Guerreschi, M., Feriani, M., Fedi, V., Bianchetti, A., and Trabucchi, M. (2007). Effectiveness of a cognitive rehabilitation program in mild dementia (MD) and mild cognitive impairment (MCI): a case control study. Arch. Gerontol. Geriatr. 44(Suppl. 1), 391–399. doi: 10.1016/j.archger.2007.01.055
Tomasi, D., Caparelli, E. C., Chang, L., and Ernst, T. (2005). fMRI-acoustic noise alters brain activation during working memory tasks. Neuroimage 27, 377–386. doi: 10.1016/j.neuroimage.2005.04.010
Tomasi, D., Chang, L., Caparelli, E. C., and Ernst, T. (2007). Different activation patterns for working memory load and visual attention load. Brain Res. 1132, 158–165. doi: 10.1016/j.brainres.2006.11.030
Tuminello, E. R., and Han, S. D. (2011). The apolipoprotein e antagonistic pleiotropy hypothesis: review and recommendations. Int. J. Alzheimers Dis. 2011:726197. doi: 10.4061/2011/726197
Winblad, B., Palmer, K., Kivipelto, M., Jelic, V., Fratiglioni, L., Wahlund, L. O., et al. (2004). Mild cognitive impairment–beyond controversies, towards a consensus: report of the International Working Group on Mild Cognitive Impairment. J. Intern. Med. 256, 240–246. doi: 10.1111/j.1365-2796.2004.01380.x
Winkler, A. M., Greve, D. N., Bjuland, K. J., Nichols, T. E., Sabuncu, M. R., Haberg, A. K., et al. (2018). Joint analysis of cortical area and thickness as a replacement for the analysis of the volume of the cerebral cortex. Cereb. Cortex 28, 738–749. doi: 10.1093/cercor/bhx308
Wu, Q., Ripp, I., Emch, M., and Koch, K. (2021). Cortical and subcortical responsiveness to intensive adaptive working memory training: an MRI surface-based analysis. Hum. Brain Mapp. 42, 2907–2920. doi: 10.1002/hbm.25412
Zeighami, Y., and Evans, A. C. (2021). Association vs. prediction: the impact of cortical surface smoothing and parcellation on brain age. Front. Big Data 4:637724. doi: 10.3389/fdata.2021.637724
Zelinski, E. M., Spina, L. M., Yaffe, K., Ruff, R., Kennison, R. F., Mahncke, H. W., et al. (2011). Improvement in memory with plasticity-based adaptive cognitive training: results of the 3-month follow-up. J. Am. Geriatr. Soc. 59, 258–265. doi: 10.1111/j.1532-5415.2010.03277.x
Zhang, H., Huntley, J., Bhome, R., Holmes, B., Cahill, J., Gould, R. L., et al. (2019a). Effect of computerised cognitive training on cognitive outcomes in mild cognitive impairment: a systematic review and meta-analysis. BMJ Open 9:e027062. doi: 10.1136/bmjopen-2018-027062
Keywords: cortical thickness, MCI, APOE genotype, LMX1A, working memory training
Citation: Hol HR, Flak MM, Chang L, Løhaugen GCC, Bjuland KJ, Rimol LM, Engvig A, Skranes J, Ernst T, Madsen B-O and Hernes SS (2022) Cortical Thickness Changes After Computerized Working Memory Training in Patients With Mild Cognitive Impairment. Front. Aging Neurosci. 14:796110. doi: 10.3389/fnagi.2022.796110
Received: 15 October 2021; Accepted: 21 February 2022;
Published: 04 April 2022.
Edited by:
Dennis Qing Wang, Southern Medical University, ChinaReviewed by:
Hao Shu, Southeast University, ChinaCopyright © 2022 Hol, Flak, Chang, Løhaugen, Bjuland, Rimol, Engvig, Skranes, Ernst, Madsen and Hernes. This is an open-access article distributed under the terms of the Creative Commons Attribution License (CC BY). The use, distribution or reproduction in other forums is permitted, provided the original author(s) and the copyright owner(s) are credited and that the original publication in this journal is cited, in accordance with accepted academic practice. No use, distribution or reproduction is permitted which does not comply with these terms.
*Correspondence: Haakon R. Hol, aGFha29uLnJhbXNsYW5kLmhvbEBvdXMtaGYubm8=
Disclaimer: All claims expressed in this article are solely those of the authors and do not necessarily represent those of their affiliated organizations, or those of the publisher, the editors and the reviewers. Any product that may be evaluated in this article or claim that may be made by its manufacturer is not guaranteed or endorsed by the publisher.
Research integrity at Frontiers
Learn more about the work of our research integrity team to safeguard the quality of each article we publish.