- 1Barrow Neuroimaging Innovation Center, Barrow Neurological Institute, Phoenix, AZ, United States
- 2Department of Computer Science, University of Verona, Verona, Italy
- 3Department of Neuroscience, Rehabilitation, Ophthalmology, Genetics, Maternal and Child Health (DINOGMI), University of Genoa, Genoa, Italy
- 4Muhammad Ali Parkinson Center, Barrow Neurological Institute, Phoenix, AZ, United States
White matter integrity and structural connectivity may be altered in mild cognitive impairment (MCI), and these changes may closely reflect decline in specific cognitive domains. Multi-shell diffusion data in healthy control (HC, n = 31) and mild cognitive impairment (MCI, n = 19) cohorts were downloaded from the ADNI3 database. The data were analyzed using an advanced approach to assess both white matter microstructural integrity and structural connectivity. Compared with HC, lower intracellular compartment (IC) and higher isotropic (ISO) values were found in MCI. Additionally, significant correlations were found between IC and Montreal Cognitive Assessment (MoCA) scores in the MCI cohort. Network analysis detected structural connectivity differences between the two groups, with lower connectivity in MCI. Additionally, significant differences between HC and MCI were observed for global network efficiency. Our results demonstrate the potential of advanced diffusion MRI biomarkers for understanding brain changes in MCI.
Introduction
Mild cognitive impairment (MCI) is defined by a mild but objective decline in cognitive function beyond that associated with normal aging; while patients with MCI do not yet meet the criteria for dementia, MCI is thought to represent a prodromal phase of Alzheimer’s disease (AD) (Metzler-Baddeley et al., 2012). More specifically, patients with MCI convert to AD at a rate of about 10–15% per year (Bruscoli and Lovestone, 2004), and thus diagnostic biomarkers for MCI and prognostic biomarkers for conversion along the spectrum of AD are critical for clinical care, disease management, and early intervention. While amyloid and tau pathology increase over time decades before cognitive changes are clinically observed, previous studies have suggested that incipient cognitive changes could be associated with white matter (WM) microstructural changes in cortical and subcortical regions (Zhuang et al., 2013; Luo et al., 2019).
Microscopic WM changes with aging and AD can be probed using advanced diffusion magnetic resonance imaging (dMRI) methods Bergamino et al., 2020, 2021b), revealing the underlying microstructural integrity and anatomical connectivity of the brain (Shim et al., 2017; Mayo et al., 2018). More specifically, microstructural integrity can be assessed by fitting a relevant model to obtain voxel-wise measures related to the local diffusion of water around axons, while tractography can yield biomarkers related to the large-scale structural connectivity of the brain. The most common model for dMRI is diffusion tensor imaging (DTI), where the resulting DTI parameters have previously demonstrated disease-related effects in WM in MCI and AD (Nir et al., 2013). However, the limitations of DTI are increasingly recognized, and more advanced acquisition and analysis models have been developed. For instance, standard DTI-derived metrics [e.g., fractional anisotropy (FA)] are influenced by contributions of different brain tissue compartments, including cerebral spinal fluid (CSF) and extracellular free water (Pierpaoli et al., 1996); we recently showed that these partial volume effects reduce the accuracy of DTI metrics in aging and AD populations (Bergamino et al., 2021b). Additionally, the standard single-tensor DTI model is based on a Gaussian diffusion assumption, which has only a single directional maximum; as a result, DTI cannot resolve multiple fiber orientations within a voxel (Tuch et al., 2002). This has led to the development of more complex, higher order models (Zhan et al., 2015) to resolve multiple intravoxel fiber orientations. One such model, the ball & stick model (Behrens et al., 2003), simultaneously accounts for extracellular free water diffusion (ball component) and models multiple fiber orientations (stick component). A previous study using this model, combined with a probabilistic tractography method, showed superior performance in differentiating between normal controls and MCI (Zhan et al., 2015).
Tractography is an advanced technique that enables reconstruction of major fiber tract pathways in the brain by mapping the trajectory of voxel-wise fiber orientations. Structural connectivity, which generates streamlines as a proxy for WM fiber bundles, can be subsequently inferred from tractography to reveal the anatomical organization of structural brain networks (Yeh et al., 2021). In the context of AD, previous studies have demonstrated that both structural and functional brain networks may be disrupted (Brier et al., 2014; Peraza et al., 2019). One challenge with tractography and structural connectivity is that these methods generate a significant number of false-positive connections between brain regions (Maier-Hein et al., 2017). In order to robustly decrease the number of false positives, Daducci et al. developed the Convex Optimization Modeling for Microstructure Informed Tractography (COMMIT) (Daducci et al., 2015) framework and later the COMMIT2 (Schiavi et al., 2020a) framework, which both aim to re-establish the link between tractography and tissue microstructure. The COMMIT framework effectively combines tractography with microstructural features of the tissue to enhance the robustness of connectivity estimates; however, this method is not effective for reducing false positives. To reduce the incidence of false positives, COMMIT2 aims to recover the connectome that best explains the diffusion-weighted signal while simultaneously minimizing the number of bundles. Through these two frameworks, the dMRI signal is modeled as a linear combination of local models associated with streamlines, where the weights are identified by solving a convex optimization problem. As demonstrated in Schiavi et al. (2020b), this filtering method may drastically improve the accuracy of resulting structural connectomes. To our knowledge, this method has not been assessed for differentiating between healthy aging and MCI.
In this study, we analyzed multi-shell dMRI data from the ADNI3 database1 using a novel, expanded approach to assess both white matter (WM) integrity and structural connectivity between healthy controls (HC) and a cohort with MCI. The COMMIT2 framework with ball & sticks forward model was used to remove false positive brain connections by assigning to each streamline its contribution to the diffusion MRI signal. Additionally, the isotropic (ISO) and restricted intra-cellular (IC) signal fractions maps obtained from the COMMIT2 global fitting were also used to perform voxel-wise analyses. The correlation between IC and ISO signal fractions maps and cognitive assessment scores was assessed. Lastly, we analyzed global and local network measures obtained from the connectomes using the brain connectivity toolbox (BCT). The overall purpose of this study was to assess WM microstructural differences between HC and MCI cohorts using this advanced analysis framework based on COMMIT2.
Materials and Methods
Subjects
Thirty-one HC [age mean ± standard deviation (S.D.) = 70 ± 6 years; 20 females] and 19 MCI (71 ± 9 years; 8 females) were included in this study. Subjects completed the Mini-Mental State Exam (MMSE) (Folstein et al., 1975) and the Montreal Cognitive Assessment (MoCA) (Nasreddine et al., 2005). The complete subject characteristics and cognitive scores are shown in Table 1. All data were downloaded from ADNI3, and only subjects with multi-shell DTI available were included in this study.
Data Availability
Data used in the preparation of this article were obtained from the Alzheimer’s Disease Neuroimaging Initiative (ADNI) database (adni.loni.usc.edu). The ADNI was launched in 2003 as a public-private partnership, led by Principal Investigator Michael W. Weiner, MD. The primary goal of ADNI has been to test whether serial magnetic resonance imaging (MRI), positron emission tomography (PET), other biological markers, and clinical and neuropsychological assessment can be combined to measure the progression of mild cognitive impairment (MCI) and early Alzheimer’s disease (AD). For up-to-date information, see www.adni-info.org.
Image Acquisition
Axial diffusion MRI data were acquired at 3T using a multi-shell DTI acquisition (SIEMENS Magnetom Prisma Fit Scanner) with the following parameters: 114 diffusion-encoding directions [b values: 500 (6 directions), 1,000 (48 directions), and 2,000 (60 directions) s/mm2; TR/TE: 3,400/71 ms; flip-angle = 90°; matrix: 256 × 256; voxel size 2.0 × 2.0 mm; slice thickness: 2.0 mm; number of averages = 1] and 13 non-diffusion-weighted images (B0 images). Accelerated sagittal T1-weighted (T1-w) anatomical images were acquired using a 3D magnetization-prepared rapid acquisition gradient echo (MP-RAGE) sequence with the following acquisition parameters: repetition time / echo time (TR/TE), 2,300/2.95 ms; acquisition matrix, 208 × 208; voxel size, 1.0 × 1.0 mm; slice thickness, 1.0 mm; flip angle = 9°.
T1-Weighted Image Processing
All MPRAGE were converted to NIFTI format using dcm2niix. The MPRAGE images were used for segmentation by FreeSurfer2, yielding the Desikan-Killiany parcellation atlas (Desikan et al., 2006) for each subject (file: aparc+aseg.mgz). Brain extraction on the MPRAGE images was performed by ROBEX3 (Iglesias et al., 2011). Using 5ttgen [MRtrix34, (Tournier et al., 2019)] and the MPRAGE images, we generated the five-tissue-type (5TT) segmented tissue image suitable for use in the anatomically constrained tractography (ACT).
Diffusion Magnetic Resonance Imaging Processing
Similar to the MPRAGE, all dMRI were converted to NIFTI format using dcm2niix and were preprocessed using Mrtrix3, FSL5 (Jenkinson et al., 2012), and the Advanced Normalization Tool (ANTs)6. dMRI pre-processing included: (1) denoising by dwidenoise (MRtrix3), (2) alignment, distortion, and eddy-currents corrections by eddy (FSL), and (3) bias field correction (ANTs). The eddy QC tools were used to evaluate the quality of the dMRI dataset. Slices with signal loss caused by subject movement coinciding with the diffusion encoding were detected and replaced by predictions made by a Gaussian process. Brain extraction on the B0 images was performed by dwi2mask (MRtrix3). Using dwi2response with msmt_5tt algorithm (MRtrix3), we estimated the response function(s), and the dwi2fod with msmt_csd algorithm (MRtrix3) was used to estimate fiber orientation distributions. For coregistration between MPRAGE-based 5TT images and dMRI space, maps of FA were created by a weighted least square fitting procedure (dtifit; FSL), and the MPRAGE images were coregistered to FA using a rigid+affine algorithm through ANTs.
Intra-Cellular and Isotropic Maps and Connectomes?
The COMMIT2 algorithm (with the following parameters: parallel diffusivity = 1.7 10–3 mm2/s and isotropic diffusivities = 1.7 10–3 mm2/s and 3.0.10–3 mm2/s) was used to create signal fractions maps of the ISO and IC compartments through the ball & stick model (Behrens et al., 2003; Panagiotaki et al., 2012). This model relates the local fiber structure to the diffusion signal by assuming different components within each voxel. The IC component can be viewed as a set of sticks where the water is restricted (purely anisotropic Gaussian motion), while the extra-cellular components (ISO) are modeled as purely (Gaussian) isotropic diffusion.
Tractography was performed [five million streamlines and the following parameters: min. length 4 mm, max. length = 200 mm, unidirectional tracking, maximum angle in degrees between successive steps = 45 (default) with backtrack option] through the probabilistic iFOD2 algorithm (Tournier et al., 2010) and the WM/gray-matter border as seed locations. The connectome was generated from the Desikan-Killiany atlas and was subsequently filtered by removing “non-connecting” streamlines. The COMMIT2 algorithm was then used to remove false positive brain connections.
All brain extracted B0 images were used to create a group-wise template using ANTs. In order to run voxel-based analysis, the IC and ISO signal fractions maps were non-linearly coregistered to the group-wise template using the coregistration matrices created by ANTs. Therefore, this template was used as the “standard” space for all analyses. All maps, in template space, were smoothed using FSL with an isotropic Gaussian kernel (sigma, 3 mm).
Network Measures
Network measures (binarized for global efficiency and for mean strength) were computed for each subject using the Brain Connectivity Toolbox (BCT) (Rubinov and Sporns, 2010) in MATLAB (MathWorks, Natick, MA, United States). For global networks, modularity (which is a statistic that quantifies the degree to which the network may be subdivided into clearly delineated groups) and the global efficiency (which corresponds to the average inverse of the shortest path length in the network) were analyzed. For local networks, clustering coefficient (which is the degree to which nodes tend to cluster together) and mean strength (where each nodal strength corresponds to the sum of the weights of links connected to it) were evaluated.
Statistical Analysis
Age, MMSE, and MoCA scores are presented as mean and S.D. for each group. Differences in age were evaluated by the Student’s t-test, while differences in sex were evaluated by the χ2 test. Differences in cognitive test scores were assessed using the Mann-Whitney U test. Table 1 shows the patient information and statistical analysis.
The analysis of covariance model (ANCOVA), with age and gender as covariates, was used to evaluate differences between MCI and HC for the IC and ISO signal fractions maps at the voxel-based level using randomise (FSL). Structural connectivity was analyzed by connectomestats (Mrtrix3), which runs the group-wise statistics at the edge level by using non-parametric permutation testing. The network-based statistic (NBS) (Zalesky et al., 2010) algorithm was performed with three different threshold values for t: tthr = 2.5, tthr = 3.0, and tthr = 3.5. For both analyses, significance was determined using a non-parametric test with 5,000 permutations; the family-wise error (FWE) rate was controlled at the 0.05 level for multiple comparisons.
Using an in-house R script7, ANCOVA was performed for the Hedges’ g effect size analysis (for both IC and ISO maps and structural connectivity), which in this study was set at g > 0.50 for medium effect and g > 0.82 for large effect (α= 0.05; power = 80%; two groups, n1 = 19 and n2 = 31).
Voxel-based correlations between cognitive assessment scores and IC and ISO signal fractions maps in the MCI cohort were assessed through an in-house MATLAB script with Spearman’s correlations at p < 0.05 significance (FDR-corrected). Differences between network measures were assessed using the two-sample Student’s t-test.
White matter clusters (shown in MNI-152 1-mm space) were labeled using the Montreal Neurological Institute and Hospital (MNI) structural (Hua et al., 2008) and the Harvard-Oxford sub-cortical structural atlases (Desikan et al., 2006).
Results
Demographic Results
The MMSE score was available for all participants, while the MoCA score was available for 28 (of 31) HC and 15 (of 19) MCI subjects. The two groups did not differ significantly in age (t-test: t = −0.894, p = 0.376) or sex (χ2 = 2.401, p = 0.121) but did differ in MMSE and MoCA (Mann-Whitney U test: Z = 2.108, p = 0.035; Z = 2.080, p = 0.038, respectively). Demographic results are shown in Table 1.
Voxel-Based Analysis of Intra-Cellular and Isotropic Maps
Figure 1, panels (A,B), show the voxel-based analysis for the IC and ISO metrics between HC and MCI groups by using an FWE < 0.05 (top) and an effect-size g > 0.50 (medium effect) with border clusters at g > 0.82 (large effect) (bottom). Compared with HC, lower IC values were found in MCI in much of the WM, while higher ISO values were found in MCI principally in the uncinated fasciculus (UF), corpus callosum (CC), retrolenticular part of internal capsule, corona radiata (CR), sagittal stratum (SS), and the external capsule (EC). It is important to note that the results were confirmed by both FWE < 0.05 and large effect-size. The corresponding significant cluster locations, for both analyses, are reported as percent volume, t, and g (for large effect) values in Table 2.
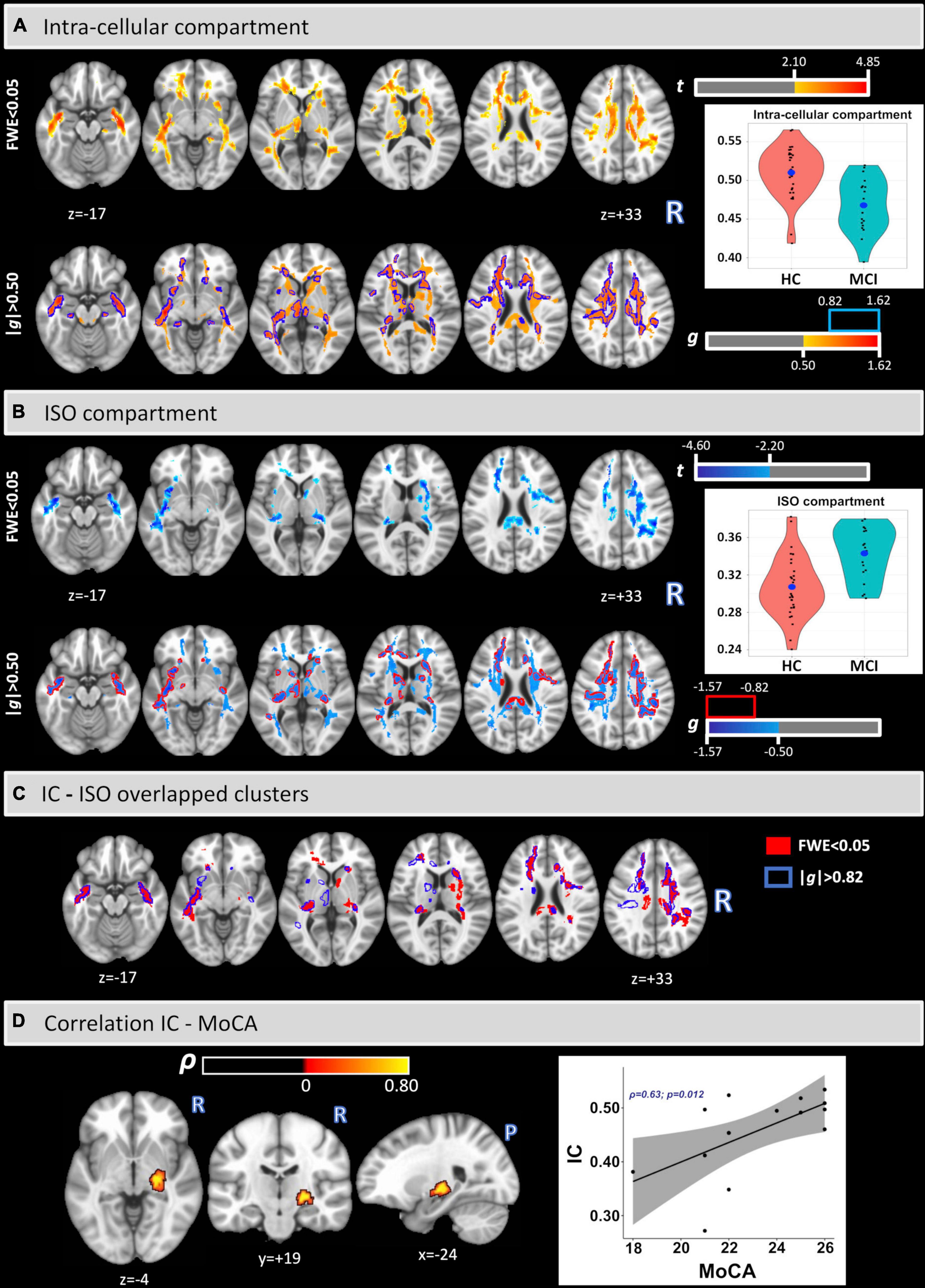
Figure 1. Voxel-based analysis of IC and ISO signal fraction maps (A,B). Lower IC and higher ISO values were found in MCI in several white matter regions and tracts. The clusters and the coordinates are in MNI152 space. Overlapped clusters by FWE < 0.05 and | g| > 0.82 (C). Significant voxel-wise Spearman’s correlations were found between IC and MoCA scores in the MCI cohort (D).
Figure 1 panel (C) shows the overlapped clusters between IC and ISO as calculated with an FWE < 0.05 (clusters in red) and an effect-size g > 0.82 (borders in blue). Additionally, Table 3 provides the relative volumes, t, and g values for all overlapped clusters.
Figure 1 panel (D) shows the voxel-based Spearman’s correlation between IC and MoCA. A significant cluster was found covering part of the right cortical spinal tract (CST) (% volume = 1.5%; ρ = 0.74), right inferior longitudinal fasciculus (ILF) (0.13%; ρ = 0.74), right cerebral peduncle (CP) (3.90%; ρ = 0.73), right posterior limb of the internal capsule (3.62%; ρ = 0.74), and the right retrolenticular part of the internal capsule (3.32%; ρ = 0.73). No significant correlations were found between IC and MMSE or for the ISO metric.
Structural Connectivity
Figure 2 shows the differences in structural connectivity (using the NBS algorithm) at FWE < 0.05 between HC and MCI cohorts by three different t-value thresholds: tthr = 2.5, tthr = 3.0, and tthr = 3.5. Additionally, the structural connectivity differences for large effect size are also shown. Compared with the HC group, lower connectivity in the MCI group was observed across all three thresholds. With increasing tthr, fewer connectivity differences were observed, with left hemispheric differences persisting at higher tthr. At the highest threshold tested (tthr = 3.5), lower structural connectivity was found in MCI between the nodes L.POP (ctx-lh-parsopercularis) and L.ITG (ctx-lh-inferiortemporal) (t = 3.883, g = 1.114) and between the nodes L.IN (ctx-lh-insula) L.LOG (ctx-lh-lateraloccipital) (t = 3.997, g = 1.146). These results are also confirmed by the effect-size analysis, where these two edges had highest values of g. Although FWE analysis did not find higher connectivity in MCI, higher connectivity was observed from effect-size analysis in MCI between nodes R.TH (Right-Thalamus) and R.LOFG (ctx-rh-lateralorbitofrontal) (t = −3.033, g = −0.925) and between nodes L.LOFG (ctx-lh-lateralorbitofrontal) and L.FP (ctx-lh-frontalpole) (t = −3.209, g = −0.960). Table 4 shows the complete connectometry results.
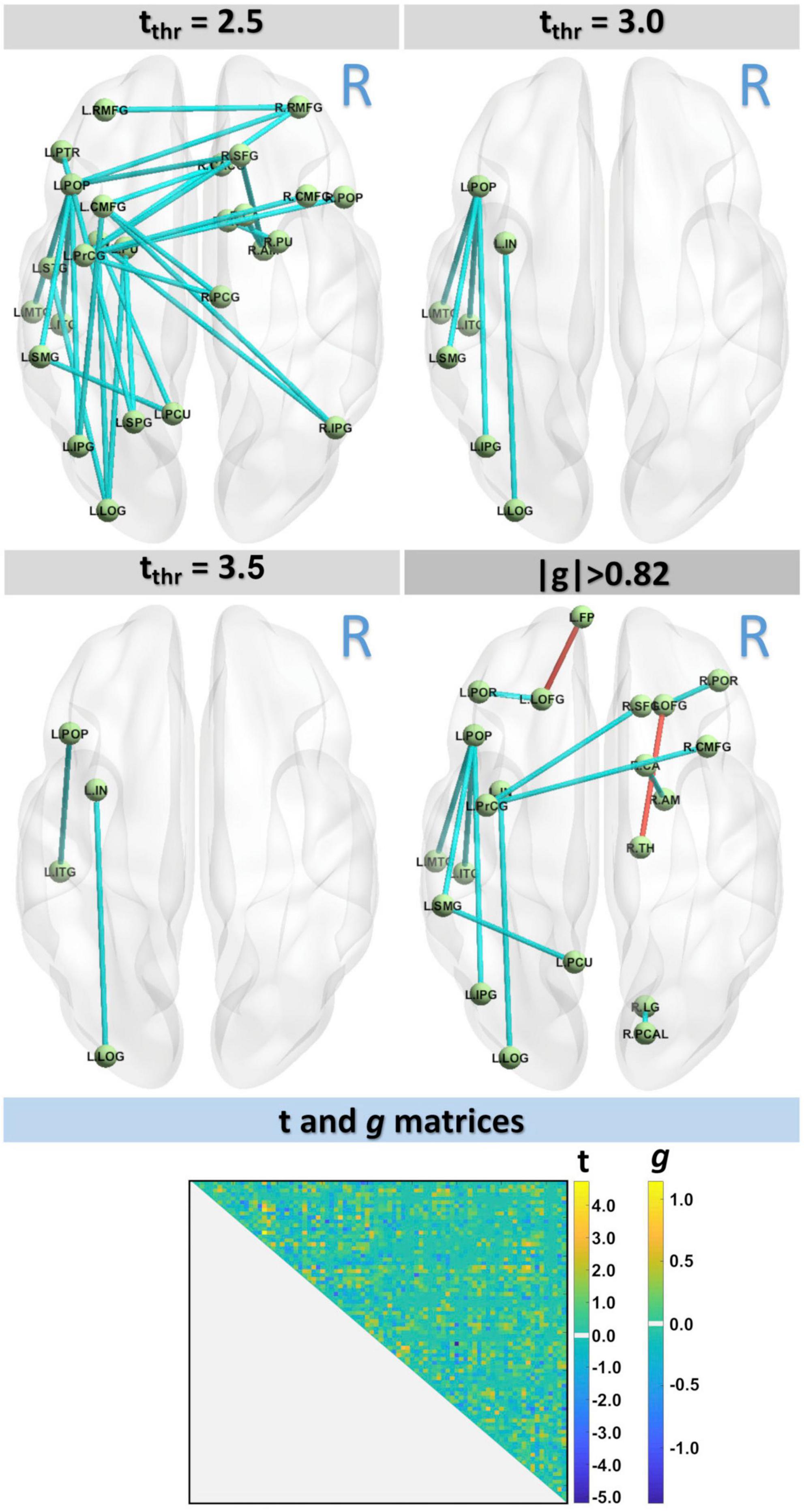
Figure 2. Connectivity analysis with three t-values thresholds, tthr = 2.5, tthr = 3.0, and tthr = 3.5, showed lower connectivity in MCI group. Results were also confirmed by the effect-size analysis.
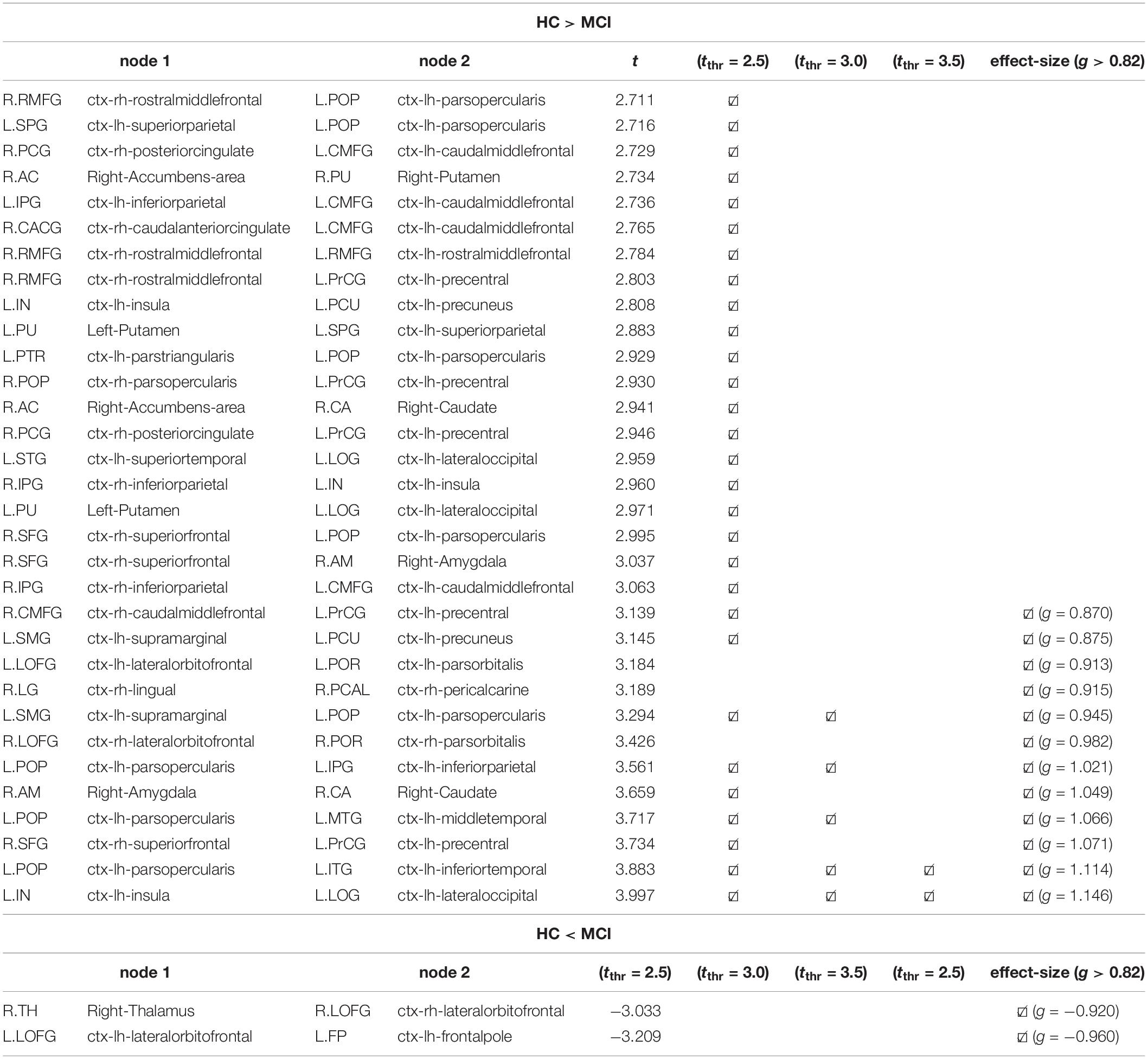
Table 4. Connectivity results with NBS (Figure 2) by different t thresholds: tthr = 2.5, tthr = 3.0, tthr = 3.5, and by the effect-size g > 0.82.
Network Measures
Figure 3 shows the differences in the network measures between the two groups. Significant differences were found in the global efficiency (t = −2.045; p(uncorrected) = 0.047). No significant differences were found in modularity, clustering coefficient, or mean strength (p > 0.05).
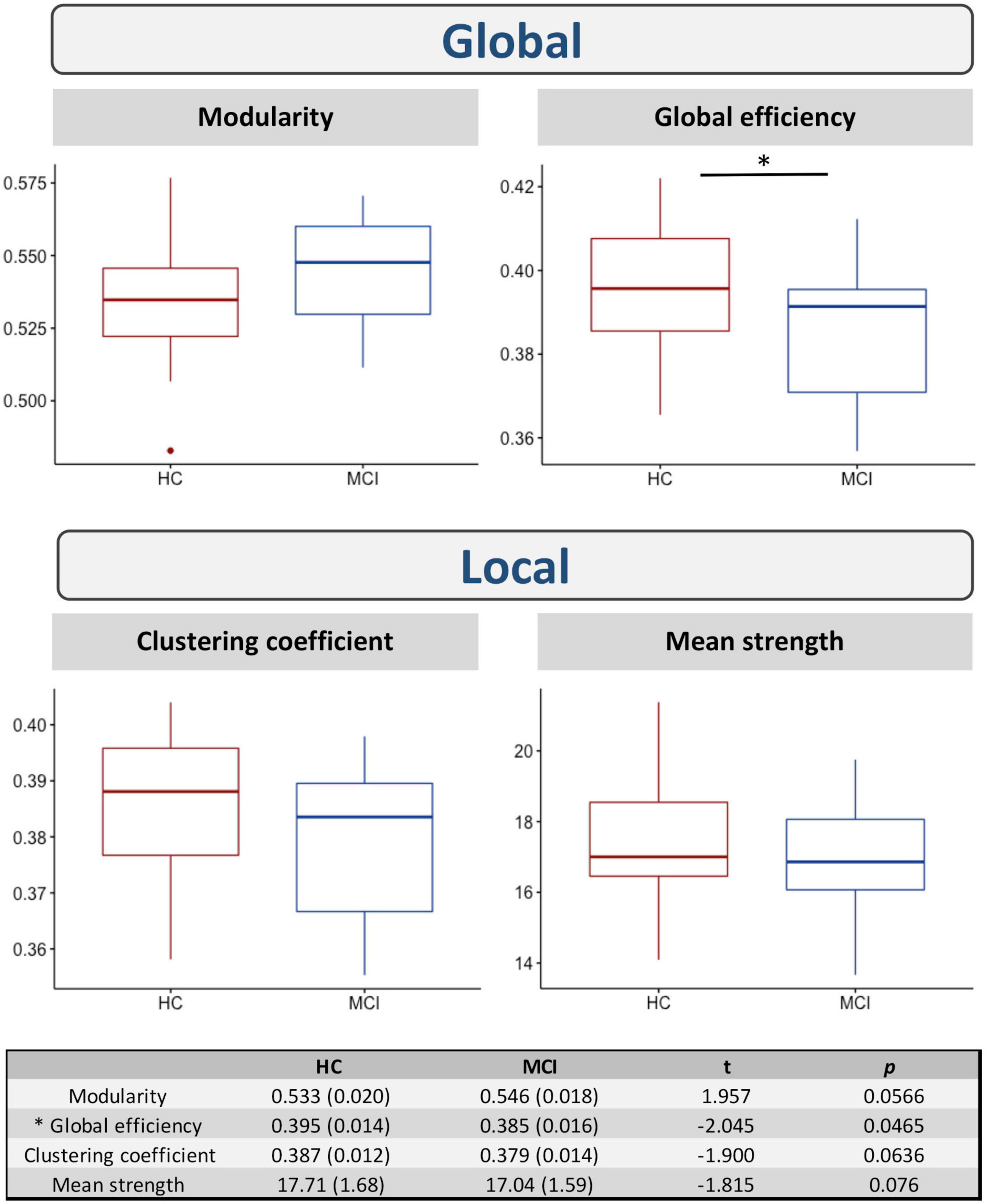
Figure 3. Differences in network measures between HC and MCI. * indicates significant differences between the two groups at p(uncorrected) < 0.05.
Discussion
In this study, the ball & stick dMRI model was used to assess differences in the restricted and isotropic compartments between HC and MCI. In regions consistent with AD, the restricted compartment associated with diffusion along WM tracts was reduced in MCI, while the isotropic component was increased. The former was also found to significantly correlate with cognitive test scores, while no correlations were observed with the latter compartment. Using probabilistic tractography with COMMIT2 filtering, lower structural connectivity was also observed in the MCI cohort, supporting the concept of AD-associated disconnection. Additional evidence of connectivity changes was quantified using network-based measures, which showed global efficiency changes in MCI. Overall, these findings are consistent with WM microstructural changes in MCI.
The neuropathological hallmarks of Alzheimer’s disease are the progressive accumulation of beta-amyloid plaques and intraneuronal tau tangles, which generally follow a well-characterized spatiotemporal pattern. These changes are thought to occur as many as decades before cognitive changes and are eventually followed by neurodegeneration. However, it is increasingly recognized that neurodegenerative patterns are highly heterogenous across AD sub-groups (Dong et al., 2017; Ekman et al., 2018). Unfortunately, identification of gross tissue atrophy patterns is unlikely to modify current therapeutic targets, as early identification and thus treatment is likely a key factor in prevention. On the other hand, the neurodegenerative pathways underlying tissue atrophy are likely associated with even earlier microscopic tissue changes that are invisible to standard MRI methods but may be probed by microstructural biomarkers with dMRI. These changes in WM microstructure can include partial loss of axons, myelin, and oligodendrocytes (Sjöbeck et al., 2005).
MRI-based dMRI measures may be sensitive to early changes associated with MCI. Standard DTI-derived measures consistently show alterations associated in MCI relative to healthy controls, including decreased FA, as well as increased axial, radial, and mean diffusivities [as reviewed in Nir et al. (2013)]. WM regions implicated in DTI studies broadly include the temporal lobe and CC. Unfortunately, standard DTI metrics are known to be affected by sub-voxel neurodegeneration, which may reduce their sensitivity and accuracy to detect changes in MCI and AD (Bergamino et al., 2021b). Another drawback to standard DTI is an inability to resolve crossing fiber tracts, which reduces the specificity of DTI metrics (such as FA, AxD, RD, and MD) in regions with multiple fiber tracts. To overcome these limitations, many advanced dMRI analysis frameworks have been developed (Afzali et al., 2021). Free-water (FW-) DTI (Pasternak et al., 2009) includes an isotropic motion term in the model and may improve DTI accuracy in aging populations (Bergamino et al., 2021b). Other advanced microstructural models include the ball-&-sticks model (used herein) and neurite orientation dispersion and density imaging (NODDI) (Zhang et al., 2012). In the context of MCI, NODDI-derived metrics may improve diagnostic performance over standard DTI metrics (Fu et al., 2020). Moving beyond voxel-based methods, WM fiber orientation distributions (FODs) can be generated from constrained spherical deconvolution methods, which then enables quantification of fiber-specific metrics and improved specificity in regions with crossing fiber tracts. Reduced fiber density has been observed in both MCI (Mito et al., 2018) and AD (Luo et al., 2021) using fixel-based analysis. The COMMIT2 framework augments probabilistic FOD-based tractography using anatomically and microstructure-informed filtering; this robust analysis framework has been demonstrated to dramatically improve the specificity of the estimated brain networks without affecting their sensitivity (Schiavi et al., 2020b). In this study, the COMMIT2 framework was applied to multi-shell dMRI data from ADNI3, yielding both voxel-wise estimates of IC and ISO and the filtered tractogram.
Biophysically, the IC component represents the fraction of dMRI signal associated with WM axons. In this study, we found reduced IC in MCI relative to HC in several WM locations, such as the retrolenticular part of the internal capsule [FWE: cluster covered 43% (right) and 47% (left); effect-size: 48% (left)], UF (FWE: 28%; effect-size: 13%), ATR (FWE: 18%; effect-size: 10%), CC (FWE: 18%; effect-size: 7%), IFOF (FWE: 15%; effect-size: 7%), forceps minor (FWE: 12.5%; effect-size: 4%), inferior and superior longitudinal fasciculus (FWE: 15% and 7%, respectively; effect-size: 6 and 4.5%, respectively), and anterior/superior/posterior corona radiata (FWE: 29%; effect-size: 22%). Notably, several of these are long-range WM pathways responsible for connecting the frontal lobe with the occipital, parietal, and temporal lobes (such as the longitudinal fasciculus and IFOF), as well as ascending and descending projection fibers (such as the corona radiata and cortical spinal tract). Several of these tracts have been implicated in cognitive impairment (Dou et al., 2020) and conversion to AD (Fu et al., 2014; Dou et al., 2020). The CC is the largest WM tract in the human brain with more than 300 million fibers interconnecting the two cerebral hemispheres. Altered WM microstructure has been observed in the genu of the CC in several studies using dMRI (Lao et al., 2017; Raghavan et al., 2020), while both cross-sectional and longitudinal studies have reported atrophy of the CC in MCI and AD (Di Paola et al., 2010; Bachman et al., 2014). Relatedly, the forceps minor connects the lateral and medial frontal cortex from the genu of the CC and has been implicated in worsening cognitive decline (Luo et al., 2019). We also found a significant cluster of correlations between IC and MoCA scores that covered the right part of the CST, ILF, CP, and the retrolenticular part and posterior limb of the internal capsule. Thus, our results similarly suggest that changes in these WM regions may be biomarkers of cognitive decline in MCI. The ISO signal fraction reflects the portion of the dMRI signal explained by tissue with isotropic diffusion, and increased ISO may be indicative of sub-voxel neurodegeneration. Consistent with this interpretation, higher ISO was found in MCI in the UF (FWE: 12.5%, effect-size: 10%), CC (FWE: 7%, effect-size: 4%), retrolenticular part and right anterior limb of the internal capsule (FWE: 33 and 18%, effect-size = 39 and 10%, respectively), and the anterior/superior/posterior corona radiata (FWE: 20%, effect-size: 13%). Similar increases in the isotropic WM diffusion have been widely documented in MCI and AD using a complementary FW-DTI approach (Dumont et al., 2019; Bergamino et al., 2021b), where the FW index was significantly elevated inside the corpus callosum and fornix, indicative of AD-associated neurodegeneration. In this study, we did not find any significant correlations between the ISO metric and cognitive scores, while IC and cognitive score correlations were observed only in the right hemisphere. In contrast, group differences were essential bilateral, with no obvious trends toward laterality.
In this study, we found differences in connectivity across various thresholds and at a large effect-size. Notably, at the lowest threshold tested, connectivity changes were observed bilaterally and interhemispherically; with higher thresholds, connectivity differences persisted only in the left hemisphere. Asymmetric connectivity changes have been previously reported in MCI and AD (Yang et al., 2017), where left hemispheric changes were dominant. At a moderate threshold, lower connectivity in MCI than HC was observed between the L.POP and the L.MTG, L.ITG, L.SMG, and L.IPG nodes, as well as between the L.IN node and L.LOG node. These connectivity differences were also associated with large effect size. The nodes L.POP and L.ITG are connected by the left SLF, which connects the occipital, parietal, and temporal lobes with the frontal cortex. WM changes in the SLF have been previously reported in AD relative to healthy controls using standard DTI metrics (Meng et al., 2012). Significant structural connectivity differences in MCI and AD have also recently been associated with the SLF (Yang et al., 2021), which is a key component of the frontoparietal network. This is further consistent with studies showing decreased functional connectivity in the frontoparietal network, concomitant with altered connectivity in the default mode network, in both AD (Zhao et al., 2019) and older adults with subtle cognitive impairment (Zanchi et al., 2017). It is important to note that the SLF plays an important role in language (Madhavan et al., 2014), attention (Mesulam, 1981), and memory (Zheng et al., 2021). While no connectivity increases (for MCI) were observed across t-value thresholds, effect-size analysis identified two pairs of nodes with higher connectivity in MCI (L.LOFG—L.FP and R.LOFG—R.TH). Increased connectivity has been reported previously in MCI and AD cohorts (Molinuevo et al., 2014); these unexpected findings have been attributed to methodological inaccuracies or a compensatory mechanism that manifests as remodeled neural networks. As these increases in connectivity were only present in the effect-size analysis, these findings should be considered in larger studies in the future.
In addition to structural connectivity changes, we also observed differences in network measures, notably in global efficiency, using a graph theory approach to derive properties of the global brain “connectome” (Rubinov and Sporns, 2010). Global efficiency is inversely related to the path length between nodes and is typically interpreted as a measure of the system capacity for parallel transfer and integrated processing of information (Bullmore and Sporns, 2012). Decreases in global efficiency and mean clustering coefficient have previously been observed in MCI (Reijmer et al., 2013; Berlot et al., 2016); consistent with that study, we found lower global efficiency and clustering coefficient, though the latter was not significant. Global network connectivity changes have been implicated in reduced cognitive control in MCI (Berlot et al., 2016), though specific cognitive domains such as episodic memory may be less sensitive to these global network changes (and more sensitive to local connections). These findings suggest that both global and local network changes play a role in the onset of MCI, although the mechanisms underlying these changes may differ in both their pathophysiological basis and associated symptoms (Reijmer et al., 2013; Berlot et al., 2016).
There are several limitations to this study. One limitation is the low number of the subjects in the MCI group (n = 19). The study population was selected from ADNI3 participants that were scanned on Siemens scanners with multi-shell dMRI and 114 diffusion-encoding directions. Other ADNI protocols involve different scanner manufacturers (GE) or fewer directions and shells (54 and 30 directions only b = 1,000 s/mm2). Unfortunately, one drawback of multicenter studies is potential bias resulting from hardware and software differences between MRI scanners and acquisition parameters. We previously showed that these variances across scanners may reduce the reliability of the MR measures or even conceal the significance of the effect of interest (Bergamino et al., 2021a). For this reason, a single acquisition was selected for this study, though future studies should confirm these findings in a larger cohort. Relatedly, we provide results related to both statistical significance and effect size for each comparison; effect size reflects the magnitude of differences found, whereas statistical significance examines whether the findings are likely to be due to chance. Another limitation relates to the inherent challenges associated with accurately modeling a biophysical system. In this study, IC and ISO parameters were obtained from the ball-&-sticks model, and a relatively new framework (COMMIT2) was used to assess structural connectivity. As the aim of this study was to assess changes in these metrics in MCI, no comparisons were performed with other advanced modeling methods. While each method has unique advantages and limitations, future work should compare various methods in the context of neurodegeneration, though validation will ultimately be critical for definitive interpretation of these findings.
In conclusion, this study shows significant differences in WM microstructural integrity between MCI and HC cohorts using three complementary methods: comparison of voxel-wise IC and ISO metrics, structural connectivity, and graph theory global metrics. At the local level, decreases in the intracellular compartment in MCI were observed across many white matter tracts, suggesting reduced white matter integrity, while increases in the isotropic compartment can be attributed to neurodegeneration and were also observed in MCI. At the global level, decreases in structural connectivity were observed in MCI in regions consistent with frontoparietal network dysfunction. Network-based metrics showed decreased global efficiency in MCI, demonstrating that changes occur over both local and global scales in MCI and may play a contributory role in cognitive impairment.
Data Availability Statement
Publicly available datasets were analyzed in this study. This data can be found here: http://adni.loni.usc.edu/.
Ethics Statement
The studies involving human participants were reviewed and approved by the Albany Medical Center Committee on Research Involving Human Subjects Institutional Review Board, Boston University Medical Campus and Boston Medical Center Institutional Review Board, Butler Hospital Institutional Review Board, Cleveland Clinic Institutional Review Board, Columbia University Medical Center Institutional Review Board, Duke University Health System Institutional Review Board, Emory Institutional Review Board, Georgetown University Institutional Review Board, Health Sciences Institutional Review Board, Houston Methodist Institutional Review Board, Howard University Office of Regulatory Research Compliance, Icahn School of Medicine at Mount Sinai Program for the Protection of Human Subjects, Indiana University Institutional Review Board, Institutional Review Board of Baylor College of Medicine, Jewish General Hospital Research Ethics Board, Johns Hopkins Medicine Institutional Review Board, Lifespan – Rhode Island Hospital Institutional Review Board, Mayo Clinic Institutional Review Board, Mount Sinai Medical Center Institutional Review Board, Nathan Kline Institute for Psychiatric Research & Rockland Psychiatric Center Institutional Review Board, New York University Langone Medical Center School of Medicine Institutional Review Board, Northwestern University Institutional Review Board, Oregon Health and Science University Institutional Review Board, Partners Human Research Committee Research Ethics, Board Sunnybrook Health Sciences Centre, Roper St. Francis Healthcare Institutional Review Board, Rush University Medical Center Institutional Review Board, St. Joseph’s Phoenix Institutional Review Board, Stanford Institutional Review Board, The Ohio State University Institutional Review Board, University Hospitals Cleveland Medical Center Institutional Review Board, University of Alabama Office of the IRB, University of British Columbia Research Ethics Board, University of California Davis Institutional Review Board Administration, University of California Los Angeles Office of the Human Research Protection Program, University of California San Diego Human Research Protections Program, University of California San Francisco Human Research Protection Program, University of Iowa Institutional Review Board, University of Kansas Medical Center Human Subjects Committee, University of Kentucky Medical Institutional Review Board, University of Michigan Medical School Institutional Review Board, University of Pennsylvania Institutional Review Board, University of Pittsburgh Institutional Review Board, University of Rochester Research Subjects Review Board, University of South Florida Institutional Review Board, University of Southern, California Institutional Review Board, UT Southwestern Institution Review Board, VA Long Beach Healthcare System Institutional Review Board, Vanderbilt University Medical Center Institutional Review Board, Wake Forest School of Medicine Institutional Review Board, Washington University School of Medicine Institutional Review Board, Western Institutional Review Board, Western University Health Sciences Research Ethics Board, and Yale University Institutional Review Board. The patients/participants provided their written informed consent to participate in this study.
Author Contributions
MB downloaded the data for this study and takes responsibility for the integrity of the data and the accuracy of the data analysis, performed the statistical analysis, and wrote the manuscript. SS and AD developed the COMMIT2 algorithm and drafted the manuscript. RRW drafted the manuscript. AMS drafted the manuscript and supervised the whole study. All authors critically reviewed the article and approved the final manuscript.
Funding
This work was supported by the Arizona Alzheimer’s Consortium and the Barrow Neurological Foundation. Data collection and sharing for this project was funded by the Alzheimer’s Disease Neuroimaging Initiative (ADNI) (National Institutes of Health Grant U01 AG024904) and DOD ADNI (Department of Defense award number W81XWH-12-2-0012). ADNI is funded by the National Institute on Aging, the National Institute of Biomedical Imaging and Bioengineering, and through generous contributions from the following: AbbVie, Alzheimer’s Association; Alzheimer’s Drug Discovery Foundation; Araclon Biotech; BioClinica, Inc.; Biogen; Bristol-Myers Squibb Company; CereSpir, Inc.; Cogstate; Eisai Inc.; Elan Pharmaceuticals, Inc.; Eli Lilly and Company; EuroImmun; F. Hoffmann-La Roche Ltd and its affiliated company Genentech, Inc.; Fujirebio; GE Healthcare; IXICO Ltd.; Janssen Alzheimer Immunotherapy Research & Development, LLC.; Johnson & Johnson Pharmaceutical Research & Development LLC.; Lumosity; Lundbeck; Merck & Co., Inc.; Meso Scale Diagnostics, LLC.; NeuroRx Research; Neurotrack Technologies; Novartis Pharmaceuticals Corporation; Pfizer Inc.; Piramal Imaging; Servier; Takeda Pharmaceutical Company; and Transition Therapeutics. The funders were not involved in the present study design, collection, analysis, interpretation of data, the writing of this article, or the decision to submit it for publication. The Canadian Institutes of Health Research is providing funds to support ADNI clinical sites in Canada. Private sector contributions are facilitated by the Foundation for the National Institutes of Health (www.fnih.org). The grantee organization is the Northern California Institute for Research and Education, and the study is coordinated by the Alzheimer’s Therapeutic Research Institute at the University of Southern California. ADNI data are disseminated by the Laboratory for Neuro Imaging at the University of Southern California.
Conflict of Interest
The authors declare that the research was conducted in the absence of any commercial or financial relationships that could be construed as a potential conflict of interest.
Publisher’s Note
All claims expressed in this article are solely those of the authors and do not necessarily represent those of their affiliated organizations, or those of the publisher, the editors and the reviewers. Any product that may be evaluated in this article, or claim that may be made by its manufacturer, is not guaranteed or endorsed by the publisher.
Acknowledgments
The authors would like to thank ADNI (http://adni.loni.usc.edu/) for providing the data used in this study. Alzheimer’s Disease Neuroimaging Initiative (ADNI) database (adni.loni.usc.edu). As such, the investigators within the ADNI contributed to the design and implementation of ADNI and/or provided data but did not participate in analysis or writing of this report. A complete listing of ADNI investigators can be found at: http://adni.loni.usc.edu/wp-content/uploads/how_to_apply/ADNI_Acknowledgement_List.pdf.
Footnotes
- ^ http://adni.loni.usc.edu/
- ^ https://surfer.nmr.mgh.harvard.edu/
- ^ https://www.nitrc.org/projects/robex
- ^ https://www.mrtrix.org/
- ^ https://fsl.fmrib.ox.ac.uk/
- ^ http://stnava.github.io/ANTs/
- ^ http://www.R-project.org/
References
Afzali, M., Pieciak, T., Newman, S., Garyfallidis, E., Özarslan, E., Cheng, H., et al. (2021). The sensitivity of diffusion MRI to microstructural properties and experimental factors. J. Neurosci. Methods 347:108951. doi: 10.1016/j.jneumeth.2020.108951
Bachman, A. H., Lee, S. H., Sidtis, J. J., and Ardekani, B. A. (2014). Corpus callosum shape and size changes in early Alzheimer’s disease: a longitudinal MRI study using the OASIS brain database. J. Alzheimer’s Dis. 39, 71–78. doi: 10.3233/JAD-131526
Behrens, T. E., Woolrich, M. W., Jenkinson, M., Johansen-Berg, H., Nunes, R. G., Clare, S., et al. (2003). Characterization and propagation of uncertainty in diffusion-weighted MR imaging. Magn. Reson. Med. 50, 1077–1088. doi: 10.1002/mrm.10609
Bergamino, M., Walsh, R. R., and Stokes, A. M. (2021b). Free-water diffusion tensor imaging improves the accuracy and sensitivity of white matter analysis in Alzheimer’s disease. Sci. Rep. 11:6990. doi: 10.1038/s41598-021-86505-7
Bergamino, M., Keeling, E. G., Walsh, R. R., and Stokes, A. M. (2021a). Systematic assessment of the impact of DTI methodology on fractional anisotropy measures in Alzheimer’s disease. Tomography 7, 20–38. doi: 10.3390/tomography7010003
Bergamino, M., Nespodzany, A., Baxter, L. C., Burke, A., Caselli, R. J., Sabbagh, M. N., et al. (2020). Preliminary assessment of intravoxel incoherent motion diffusion-weighted MRI (IVIM-DWI) metrics in Alzheimer’s disease. J. Magn. Reson. Imaging 52, 1811–1826.
Berlot, R., Metzler-Baddeley, C., Ikram, M. A., Jones, D. K., and O’sullivan, M. J. (2016). Global efficiency of structural networks mediates cognitive control in mild cognitive impairment. Front. Aging Neurosci. 8:292. doi: 10.3389/fnagi.2016.00292
Brier, M. R., Thomas, J. B., and Ances, B. M. (2014). Network dysfunction in Alzheimer’s disease: refining the disconnection hypothesis. Brain Connect 4, 299–311. doi: 10.1089/brain.2014.0236
Bruscoli, M., and Lovestone, S. (2004). Is MCI really just early dementia? A systematic review of conversion studies. Int. Psychogeriatr 16, 129–140. doi: 10.1017/s1041610204000092
Bullmore, E., and Sporns, O. (2012). The economy of brain network organization. Nat. Rev. Neurosci. 13, 336–349. doi: 10.1038/nrn3214
Daducci, A., Dal Palù, A., Lemkaddem, A., and Thiran, J. P. (2015). COMMIT: convex optimization modeling for microstructure informed tractography. IEEE Trans. Med. Imaging 34, 246–257. doi: 10.1109/tmi.2014.2352414
Desikan, R. S., Ségonne, F., Fischl, B., Quinn, B. T., Dickerson, B. C., Blacker, D., et al. (2006). An automated labeling system for subdividing the human cerebral cortex on MRI scans into gyral based regions of interest. Neuroimage 31, 968–980. doi: 10.1016/j.neuroimage.2006.01.021
Di Paola, M., Luders, E., Di Iulio, F., Cherubini, A., Passafiume, D., Thompson, P. M., et al. (2010). Callosal atrophy in mild cognitive impairment and Alzheimer’s disease: different effects in different stages. Neuroimage 49, 141–149. doi: 10.1016/j.neuroimage.2009.07.050
Dong, A., Toledo, J. B., Honnorat, N., Doshi, J., Varol, E., Sotiras, A., et al. (2017). Heterogeneity of neuroanatomical patterns in prodromal Alzheimer’s disease: links to cognition, progression and biomarkers. Brain 140, 735–747. doi: 10.1093/brain/aww319
Dou, X., Yao, H., Feng, F., Wang, P., Zhou, B., Jin, D., et al. (2020). Characterizing white matter connectivity in Alzheimer’s disease and mild cognitive impairment: an automated fiber quantification analysis with two independent datasets. Cortex 129, 390–405. doi: 10.1016/j.cortex.2020.03.032
Dumont, M., Roy, M., Jodoin, P. M., Morency, F. C., Houde, J. C., Xie, Z., et al. (2019). Free water in white matter differentiates MCI and AD from control subjects. Front. Aging Neurosci. 11:270. doi: 10.3389/fnagi.2019.00270
Ekman, U., Ferreira, D., and Westman, E. (2018). The A/T/N biomarker scheme and patterns of brain atrophy assessed in mild cognitive impairment. Sci. Rep. 8:8431. doi: 10.1038/s41598-018-26151-8
Folstein, M. F., Folstein, S. E., and Mchugh, P. R. (1975). Mini-mental state”. a practical method for grading the cognitive state of patients for the clinician. J. Psychiatr. Res. 12, 189–198.
Fu, J. L., Liu, Y., Li, Y. M., Chang, C., and Li, W. B. (2014). Use of diffusion tensor imaging for evaluating changes in the microstructural integrity of white matter over 3 years in patients with amnesic-type mild cognitive impairment converting to Alzheimer’s disease. J. Neuroimaging 24, 343–348. doi: 10.1111/jon.12061
Fu, X., Shrestha, S., Sun, M., Wu, Q., Luo, Y., Zhang, X., et al. (2020). Microstructural white matter alterations in mild cognitive impairment and Alzheimer’s disease : study based on neurite orientation dispersion and density imaging (NODDI). Clin. Neuroradiol. 30, 569–579. doi: 10.1007/s00062-019-00805-0
Hua, K., Zhang, J., Wakana, S., Jiang, H., Li, X., Reich, D. S., et al. (2008). Tract probability maps in stereotaxic spaces: analyses of white matter anatomy and tract-specific quantification. Neuroimage 39, 336–347. doi: 10.1016/j.neuroimage.2007.07.053
Iglesias, J. E., Liu, C. Y., Thompson, P. M., and Tu, Z. (2011). Robust brain extraction across datasets and comparison with publicly available methods. IEEE Trans. Med. Imaging 30, 1617–1634. doi: 10.1109/TMI.2011.2138152
Jenkinson, M., Beckmann, C. F., Behrens, T. E., Woolrich, M. W., and Smith, S. M. (2012). FSL. Neuroimage 62, 782–790.
Lao, Y., Nguyen, B., Tsao, S., Gajawelli, N., Law, M., Chui, H., et al. (2017). A T1 and DTI fused 3D corpus callosum analysis in MCI subjects with high and low cardiovascular risk profile. Neuroimage Clin. 14, 298–307. doi: 10.1016/j.nicl.2016.12.027
Luo, C., Li, M., Qin, R., Chen, H., Yang, D., Huang, L., et al. (2019). White matter microstructural damage as an early sign of subjective cognitive decline. Front. Aging Neurosci. 11:378. doi: 10.3389/fnagi.2019.00378
Luo, X., Wang, S., Jiaerken, Y., Li, K., Zeng, Q., Zhang, R., et al. (2021). Distinct fiber-specific white matter reductions pattern in early- and late-onset Alzheimer’s disease. Aging (Albany NY) 13, 12410–12430. doi: 10.18632/aging.202702
Madhavan, K. M., Mcqueeny, T., Howe, S. R., Shear, P., and Szaflarski, J. (2014). Superior longitudinal fasciculus and language functioning in healthy aging. Brain Res. 1562, 11–22. doi: 10.1016/j.brainres.2014.03.012
Maier-Hein, K. H., Neher, P. F., Houde, J. C., Côté, M. A., Garyfallidis, E., Zhong, J., et al. (2017). The challenge of mapping the human connectome based on diffusion tractography. Nat. Commun. 8:1349.
Mayo, C. D., Garcia-Barrera, M. A., Mazerolle, E. L., Ritchie, L. J., Fisk, J. D., and Gawryluk, J. R. (2018). Relationship between DTI metrics and cognitive function in Alzheimer’s disease. Front. Aging Neurosci. 10:436.
Meng, J. Z., Guo, L. W., Cheng, H., Chen, Y. J., Fang, L., Qi, M., et al. (2012). Correlation between cognitive function and the association fibers in patients with Alzheimer’s disease using diffusion tensor imaging. J. Clin. Neurosci. 19, 1659–1663. doi: 10.1016/j.jocn.2011.12.031
Mesulam, M. M. (1981). A cortical network for directed attention and unilateral neglect. Ann. Neurol. 10, 309–325. doi: 10.1002/ana.410100402
Metzler-Baddeley, C., Jones, D. K., Steventon, J., Westacott, L., Aggleton, J. P., and O’sullivan, M. J. (2012). Cingulum microstructure predicts cognitive control in older age and mild cognitive impairment. J. Neurosci. 32, 17612–17619. doi: 10.1523/JNEUROSCI.3299-12.2012
Mito, R., Raffelt, D., Dhollander, T., Vaughan, D. N., Tournier, J. D., Salvado, O., et al. (2018). Fibre-specific white matter reductions in Alzheimer’s disease and mild cognitive impairment. Brain 141, 888–902. doi: 10.1093/brain/awx355
Molinuevo, J. L., Ripolles, P., Simó, M., Lladó, A., Olives, J., Balasa, M., et al. (2014). White matter changes in preclinical Alzheimer’s disease: a magnetic resonance imaging-diffusion tensor imaging study on cognitively normal older people with positive amyloid β protein 42 levels. Neurobiol. Aging 35, 2671–2680. doi: 10.1016/j.neurobiolaging.2014.05.027
Nasreddine, Z. S., Phillips, N. A., Bédirian, V., Charbonneau, S., Whitehead, V., Collin, I., et al. (2005). The montreal cognitive assessment, MoCA: a brief screening tool for mild cognitive impairment. J Am Geriatr Soc 53, 695–699. doi: 10.1111/j.1532-5415.2005.53221.x
Nir, T. M., Jahanshad, N., Villalon-Reina, J. E., Toga, A. W., Jack, C. R., Weiner, M. W., et al. (2013). Effectiveness of regional DTI measures in distinguishing Alzheimer’s disease, MCI, and normal aging. Neuroimage Clin. 3, 180–195. doi: 10.1016/j.nicl.2013.07.006
Panagiotaki, E., Schneider, T., Siow, B., Hall, M. G., Lythgoe, M. F., and Alexander, D. C. (2012). Compartment models of the diffusion MR signal in brain white matter: a taxonomy and comparison. Neuroimage 59, 2241–2254. doi: 10.1016/j.neuroimage.2011.09.081
Pasternak, O., Sochen, N., Gur, Y., Intrator, N., and Assaf, Y. (2009). Free water elimination and mapping from diffusion MRI. Magn. Reson. Med. 62, 717–730.
Peraza, L. R., Díaz-Parra, A., Kennion, O., Moratal, D., Taylor, J. P., Kaiser, M., et al. (2019). Structural connectivity centrality changes mark the path toward Alzheimer’s disease. Alzheimers Dement (Amst) 11, 98–107. doi: 10.1016/j.dadm.2018.12.004
Pierpaoli, C., Jezzard, P., Basser, P. J., Barnett, A., and Di Chiro, G. (1996). Diffusion tensor MR imaging of the human brain. Radiology 201, 637–648.
Raghavan, S., Przybelski, S. A., Reid, R. I., Graff-Radford, J., Lesnick, T. G., Zuk, S. M., et al. (2020). Reduced fractional anisotropy of the genu of the corpus callosum as a cerebrovascular disease marker and predictor of longitudinal cognition in MCI. Neurobiol. Aging 96, 176–183. doi: 10.1016/j.neurobiolaging.2020.09.005
Reijmer, Y. D., Leemans, A., Caeyenberghs, K., Heringa, S. M., Koek, H. L., and Biessels, G. J. (2013). Disruption of cerebral networks and cognitive impairment in alzheimer disease. Neurology 80, 1370–1377. doi: 10.1212/WNL.0b013e31828c2ee5
Rubinov, M., and Sporns, O. (2010). Complex network measures of brain connectivity: uses and interpretations. Neuroimage 52, 1059–1069. doi: 10.1016/j.neuroimage.2009.10.003
Schiavi, S., Ocampo-Pineda, M., Barakovic, M., Petit, L., Descoteaux, M., Thiran, J. P., et al. (2020a). A new method for accurate in vivo mapping of human brain connections using microstructural and anatomical information. Sci. Adv. 6:eaba8245. doi: 10.1126/sciadv.aba8245
Schiavi, S., Petracca, M., Battocchio, M., El Mendili, M. M., Paduri, S., Fleysher, L., et al. (2020b). Sensory-motor network topology in multiple sclerosis: structural connectivity analysis accounting for intrinsic density discrepancy. Hum. Brain Mapp. 41, 2951–2963. doi: 10.1002/hbm.24989
Shim, G., Choi, K. Y., Kim, D., Suh, S. I., Lee, S., Jeong, H. G., et al. (2017). Predicting neurocognitive function with hippocampal volumes and DTI metrics in patients with Alzheimer’s dementia and mild cognitive impairment. Brain Behav. 7:e00766. doi: 10.1002/brb3.766
Sjöbeck, M., Haglund, M., and Englund, E. (2005). Decreasing myelin density reflected increasing white matter pathology in Alzheimer’s disease–a neuropathological study. Int .J. Geriatr. Psychiatry 20, 919–926. doi: 10.1002/gps.1384
Tournier, J. D., Calamante, F., and Connelly, A. (2010). “Improved probabilistic streamlines tractography by 2nd order integration over fibre orientation distributions,” in Proceedings of the International Society for Magnetic Resonance in Medicine, 1670.
Tournier, J. D., Smith, R., Raffelt, D., Tabbara, R., Dhollander, T., Pietsch, M., et al. (2019). MRtrix3: a fast, flexible and open software framework for medical image processing and visualisation. Neuroimage 202:116137. doi: 10.1016/j.neuroimage.2019.116137
Tuch, D. S., Reese, T. G., Wiegell, M. R., Makris, N., Belliveau, J. W., and Wedeen, V. J. (2002). High angular resolution diffusion imaging reveals intravoxel white matter fiber heterogeneity. Magn. Reson. Med. 48, 577–582. doi: 10.1002/mrm.10268
Yang, C., Zhong, S., Zhou, X., Wei, L., Wang, L., and Nie, S. (2017). The abnormality of topological asymmetry between hemispheric brain white matter networks in Alzheimer’s disease and mild cognitive impairment. Front. Aging Neurosci. 9:261. doi: 10.3389/fnagi.2017.00261
Yang, F. P. G., Bal, S. S., Lee, J. F., and Chen, C. C. (2021). White matter differences in networks in elders with mild cognitive impairment and Alzheimer’s disease. Brain Connect 11, 180–188.
Yeh, C. H., Jones, D. K., Liang, X., Descoteaux, M., and Connelly, A. (2021). Mapping structural connectivity using diffusion MRI: challenges and opportunities. J. Magn. Reson. Imaging 53, 1666–1682. doi: 10.1002/jmri.27188
Zalesky, A., Fornito, A., and Bullmore, E. T. (2010). Network-based statistic: identifying differences in brain networks. Neuroimage 53, 1197–1207. doi: 10.1016/j.neuroimage.2010.06.041
Zanchi, D., Montandon, M. L., Sinanaj, I., Rodriguez, C., Depoorter, A., Herrmann, F. R., et al. (2017). Decreased fronto-parietal and increased default mode network activation is associated with subtle cognitive deficits in elderly controls. Neurosignals 25, 127–138. doi: 10.1159/000486152
Zhan, L., Zhou, J., Wang, Y., Jin, Y., Jahanshad, N., Prasad, G., et al. (2015). Comparison of nine tractography algorithms for detecting abnormal structural brain networks in Alzheimer’s disease. Front. Aging Neurosci. 7:48. doi: 10.3389/fnagi.2015.00048
Zhang, H., Schneider, T., Wheeler-Kingshott, C. A., and Alexander, D. C. (2012). NODDI: practical in vivo neurite orientation dispersion and density imaging of the human brain. Neuroimage 61, 1000–1016.
Zhao, Q., Sang, X., Metmer, H., Swati, Z., and Lu, J. (2019). Functional segregation of executive control network and frontoparietal network in Alzheimer’s disease. Cortex 120, 36–48. doi: 10.1016/j.cortex.2019.04.026
Zheng, Y., Wang, D., Ye, Q., Zou, F., Li, Y., and Kwok, S. C. (2021). Diffusion property and functional connectivity of superior longitudinal fasciculus underpin human metacognition. Neuropsychologia 156:107847. doi: 10.1016/j.neuropsychologia.2021.107847
Keywords: Alzheimer’s disease, mild cognitive impairment, diffusion tensor imaging (DTI), tractography, structural connectivity, white matter integrity
Citation: Bergamino M, Schiavi S, Daducci A, Walsh RR and Stokes AM (2022) Analysis of Brain Structural Connectivity Networks and White Matter Integrity in Patients With Mild Cognitive Impairment. Front. Aging Neurosci. 14:793991. doi: 10.3389/fnagi.2022.793991
Received: 12 October 2021; Accepted: 06 January 2022;
Published: 31 January 2022.
Edited by:
Annalena Venneri, Brunel University London, United KingdomReviewed by:
Weihao Zheng, Lanzhou University, ChinaFederico Ramírez Toraño, Complutense University of Madrid, Spain
Copyright © 2022 Bergamino, Schiavi, Daducci, Walsh and Stokes. This is an open-access article distributed under the terms of the Creative Commons Attribution License (CC BY). The use, distribution or reproduction in other forums is permitted, provided the original author(s) and the copyright owner(s) are credited and that the original publication in this journal is cited, in accordance with accepted academic practice. No use, distribution or reproduction is permitted which does not comply with these terms.
*Correspondence: Ashley M. Stokes, YXNobGV5LnN0b2tlc0BiYXJyb3duZXVyby5vcmc=