- 1Stomatological Hospital, Southern Medical University, Guangzhou, China
- 2Laboratory of Molecular Cell Biology, Beijing Tibetan Hospital, China Tibetology Research Center, Beijing, China
- 3Department of Experimental and Clinical Medicine, University of Florence, Florence, Italy
- 4Faculty of Physics, University of Münster, Münster, Germany
- 5Department of Cranio Maxillofacial Surgery, University Clinic Leipzig, Leipzig, Germany
- 6Department of Cariology, Endodontology and Periodontology, University of Leipzig, Leipzig, Germany
Objective: To identify the genetic linkage mechanisms underlying Parkinson’s disease (PD) and periodontitis, and explore the role of immunology in the crosstalk between both these diseases.
Methods: The gene expression omnibus (GEO) datasets associated with whole blood tissue of PD patients and gingival tissue of periodontitis patients were obtained. Then, differential expression analysis was performed to identify the differentially expressed genes (DEGs) deregulated in both diseases, which were defined as crosstalk genes. Inflammatory response-related genes (IRRGs) were downloaded from the MSigDB database and used for dividing case samples of both diseases into different clusters using k-means cluster analysis. Feature selection was performed using the LASSO model. Thus, the hub crosstalk genes were identified. Next, the crosstalk IRRGs were selected and Pearson correlation coefficient analysis was applied to investigate the correlation between hub crosstalk genes and hub IRRGs. Additionally, immune infiltration analysis was performed to examine the enrichment of immune cells in both diseases. The correlation between hub crosstalk genes and highly enriched immune cells was also investigated.
Results: Overall, 37 crosstalk genes were found to be overlapping between the PD-associated DEGs and periodontitis-associated DEGs. Using clustering analysis, the most optimal clustering effects were obtained for periodontitis and PD when k = 2 and k = 3, respectively. Using the LASSO feature selection, five hub crosstalk genes, namely, FMNL1, MANSC1, PLAUR, RNASE6, and TCIRG1, were identified. In periodontitis, MANSC1 was negatively correlated and the other four hub crosstalk genes (FMNL1, PLAUR, RNASE6, and TCIRG1) were positively correlated with five hub IRRGs, namely, AQP9, C5AR1, CD14, CSF3R, and PLAUR. In PD, all five hub crosstalk genes were positively correlated with all five hub IRRGs. Additionally, RNASE6 was highly correlated with myeloid-derived suppressor cells (MDSCs) in periodontitis, and MANSC1 was highly correlated with plasmacytoid dendritic cells in PD.
Conclusion: Five genes (i.e., FMNL1, MANSC1, PLAUR, RNASE6, and TCIRG1) were identified as crosstalk biomarkers linking PD and periodontitis. The significant correlation between these crosstalk genes and immune cells strongly suggests the involvement of immunology in linking both diseases.
Introduction
Parkinson’s disease (PD) is a chronic, debilitating, neurodegenerative disorder with both motor and nonmotor symptoms that gradually worsen over time (Blaszczyk, 2016). Systemic inflammatory response plays a critical role in the progression of PD by activating microglial cells and initiating the cascade of neurodegeneration (Kaur et al., 2016). Periodontitis is a highly prevalent, multifactorial, chronic inflammatory disease of the periodontium, which causes destruction of the supportive tissues of the teeth and, eventually, tooth loss (Kaur et al., 2018). Several previous epidemiological studies have indicated a possible link between periodontal disease and PD. Some studies have reported that PD patients have a higher prevalence and risk of developing periodontal disease compared to a person without PD (Schwarz et al., 2006; Hanaoka and Kashihara, 2009). The cognitive dysfunction of PD patients prevented them from effectively performing routine daily oral hygiene activities (Al-Omari et al., 2014). Additionally, one of the side effects of multiple medications was altered salivary flow rates, thereby affecting self-cleaning oral mechanisms (Auffret et al., 2021). In other studies, periodontitis was found to be associated with an increased risk of developing PD (Chen et al., 2017). The ulcerations in the periodontal pocket lining provided easy access for periodontal pathogens (e.g., Aggregatibacter actinomycetemcomitans (Aa), Porphyromonas gingivalis (Pg), Tannerella forsythia (Tf), Treponema denticola (Td), and Fusobacterium nucleatum (Fn)) and inflammatory mediators (e.g., pro-inflammatory cytokines) to infiltrate the systemic peripheral blood circulation (Scannapieco, 2004). Lipopolysaccharide (LPS), an endotoxin and component of the Gram-negative bacterial cell wall, caused the breakdown of the blood brain barrier (BBB) and activated the microglial cells, which led to the necrosis and apoptosis of dopaminergic neurons in the substantia nigra (SN) of the midbrain (Bohatschek et al., 2001; Olsen et al., 2020).
Apart from inflammatory response, systemic immune activation may be another link connecting periodontitis and PD. It is suggested that periodontitis triggers the systemic immune response, following which the activated immune cells from the peripheral blood overcome the BBB and promote central nervous system (CNS) inflammation in PD patients (Zhang et al., 2021). Further, certain genetic susceptibility factors have been demonstrated to increase the risk of both periodontitis and PD (Tettamanti et al., 2017; Karimi-Moghadam et al., 2018). A previous study conducted by João Botelho and colleagues analyzed the Genome-Wide Association Studies (GWAS) data and identified the protein variants strongly associated with PD and periodontitis onset (Botelho et al., 2020). However, it may be worthy to note that a potential limitation of this research is that its study design was not from an inflammatory or immunological perspective. Thus, to fill this gap, our current study aimed to explore the missing genetic links between PD and periodontitis, particularly focusing on inflammatory response-associated genes and immune cells.
Many previous studies used computational biology approaches to explore the shared genetic linkages between two pathogenesis-related diseases (Li et al., 2018; Chen et al., 2021, 2022; He et al., 2021; Alves et al., 2022; Pan et al., 2022; Yan et al., 2022; Liu et al., 2022a,b). In order to investigate the shared genetic links between PD and periodontitis, integrated bioinformatics analyses were also utilized. First, differentially expressed genes (DEGs) deregulated in both diseases were obtained, and crosstalk genes were identified. The hub crosstalk genes were selected by performing k-means cluster analysis based on inflammatory response-related genes (IRRGs). Then, the correlation between hub crosstalk genes and IRRGs as well as the correlation between hub crosstalk genes and immune cells were investigated.
Materials and methods
Study design of the current research
Figure 1 used a schematic diagram to show the study flowchart of the current research. Firstly, differential expression analysis was performed to identify the DEGs belonging to PD and periodontitis respectively, and thus crosstalk genes were identified. Secondly, IRRGs were obtained and used for performing the K-means cluster analysis, and then differentially expressed (DE)- cluster crosstalk genes were identified. Thirdly, hub crosstalk genes were identified by building the Lasso logistic regression model. Lastly, hub crosstalk genes as the investigation focus were researched from many aspects, for example, correlation with IRRGs; expression patterns of hub crosstalk genes in clusters of both diseases; correlation with immune cells; related PPI network; related pathway network.
Datasets
The datasets related to periodontitis and PD were, respectively, downloaded from the gene expression omnibus (GEO)1 database (Edgar et al., 2002). The inclusion criteria for the periodontitis-related GEO datasets are as below: (1) The study design should be set as: established periodontitis samples as the experimental group and healthy gingival samples as the control group. (2) Periodontitis was defined in accordance with the case definition presented in the 2017 World Workshop: ① interdental CAL detectable at ≥2 nonadjacent teeth or ② buccal or oral with CAL ≥3 mm with pocketing >3 mm detectable at ≥2 teeth (Tonetti et al., 2018). (3) The sample size of the disease samples should be more than 20. The inclusion criteria for the PD-related GEO datasets are as below: (1) The study design should aim to compare the transcriptomic profiling of the blood samples (e.g., whole blood, peripheral blood mononuclear cells) between PD patients and healthy control subjects. (2) The sample size of the disease samples should be more than 20. Following such inclusion criteria, the GSE16134 dataset (Papapanou et al., 2009; Kebschull et al., 2014) was selected to be a periodontitis-related GEO dataset, and three datasets [GSE6613 (Scherzer et al., 2007, 2008), GSE49126 (Mutez et al., 2014), and GSE99039 (Shamir et al., 2017)] were selected to be PD-related datasets. Table 1 shows detailed information about these four included datasets.
By downloading the human gene set “HALLMARK_INFLAMMATORY_RESPONSE”2 from the MSigDB database3 (Lopez et al., 2018), 200 IRRGs were obtained. In addition, the gene signatures of 28 tumor-infiltrating lymphocytes were downloaded from the TISIDB database4 (Zhang et al., 2013).
Data preprocessing
The Probe ID in the expression matrix was converted to the gene symbol based on the respective platform information for the downloaded data. If one Probe ID corresponds to multiple gene symbols, the gene symbols were de-duplicated based on the average of the sample expression values. When the number of samples with a gene of 0 in the expression matrix exceeds half of the total number of samples, the gene was removed from the expression matrix. In addition, Log2 was calculated for the dataset with large sample expression values, in order to achieve data standardization.
For PD, three datasets related to PD (i.e., GSE6613, GSE49126, GSE99039) were combined based on a common Gene Symbol. These three datasets were designed under different experimental platforms: GSE6613—GPL96 [HG-U133A] Affymetrix Human Genome U133A Array; GSE49126—GPL4133 Agilent-014850 Whole Human Genome Microarray 4x44K G4112F (Feature Number version); GSE99039—GPL570 [HG-U133_Plus_2] Affymetrix Human Genome U133 Plus 2.0 Array. After merging, the ComBat funtion in the “sva” package (version 4.1.3) (Leek et al., 2019) was used to eliminate the batch effect of the merged data. The PCA analysis was carried out, respectively, on the combined expression matrix and the expression matrix after ComBat correction, in order to examine the effects after ComBat correction.
Differential expression analysis
The “limma” package (version 3.15) (Ritchie et al., 2015) was used to analyze the differential expression between Case-sample and Normal-sample and identify the DEGs. DEGs were identified according to different thresholds of P.adjust and log2FC (log2 fold change) for periodontitis dataset and PD dataset, respectively. The P.adjust<0.05 and | log2FC | > 0.5 were selected as the threshold for selecting DEGs in periodontitis dataset. The P.adjust<0.05 and | log2FC | > 0.15 was selected as the threshold when selecting DEGs in PD datasets.
Cross-talk gene between periodontitis disease and PD
To explore potential relationships between Periodontitis disease and PD, DEGs deregulated in periodontitis and DEGs deregulated in PD were obtained respectively, and their intersection genes were labelled as cross-talk genes. These intersection genes could be considered as the bridge genes connecting the relationship between periodontitis and PD. The “pheatmap” package (version 1.0.12) (Kolde and Kolde, 2018) was used to analyze the expression level of these cross-talk genes. The “ClusterProfiler” package (version 3.15) (Yu et al., 2012; Wu et al., 2021) in R was utilized to analyze the cross-talk gene for Gene Ontology (GO) term-biological processes (BPs) and KEGG pathways to observe the biological functions affected by these cross-talk genes.
Consensus cluster plus analysis of periodontitis and Parkinson’s based on the IRRG dataset
The differentially expressed IRRGs were obtained from periodontitis and PD, respectively. Then the expression values of the differentially expressed IRRGs in case samples were obtained for periodontitis and PD, respectively. The Consensus Cluster Plus algorithm (Wilkerson and Hayes, 2010) was utilized to carry out k-means cluster analysis based on the expression matrix of case samples.
Before using the Consensus Cluster Plus algorithm, it is important to determine the number of clusters. To determine the optimal number of samples, the interval silhouette width and elbow method were used to analyze the IRRGs expression matrix of periodontitis and PD, respectively. The fviz_nbclust method of “factoextra” package (version 1.0.7) (Kassambara and Mundt, 2017) was used for the analysis. The average silhouette width automatically calculated the number of the optimum clusters. The elbow method was used to observe the slope change of the vertical within sum of square (WSS). When the WSS decreases very slowly, increasing the number of clusters can no longer increase the clustering effect, and the existence of this “elbow point” is the optimal number of clusters. The optimal number of clusters was determined by considering the results of the two methods together. The “ConsensusClusterPlus” algorithm was used, and optimum cluster value was defined as the value of the parameter maxK. The case samples in periodontitis and PD were divided into different clusters based on IRRGs. Afterward, the expression levels of IRRG in different clusters were analyzed.
Identification of hub cross-talk genes based on cluster
The expression values of cross-talk gene in the case sample of the two diseases were obtained, and then “limma” package (version 3.15) (Ritchie et al., 2015) was used to analyze the cross-talk gene differentially expressed in different clusters according to the respective cluster categories. The cross-talk genes of p.adjust <0.01 were labeled as DE-cluster cross-talk genes. The least absolute shrinkage and selection operator (LASSO) Logistic Regression approach was used to further filter the DE-cluster cross-talk gene.
The expression values of the DE-cluster cross-talk gene in the respective disease’s case samples were obtained, and then LASSO model was used for feature screening according to the respective cluster. According to the lambda.min value in the analysis results, the feature genes of both diseases were obtained. Finally, the intersection of the feature genes of the two diseases were obtained, and these intersection genes were regarded as the final hub cross-talk genes. These hub cross-talk genes were not only differentially expressed in periodontitis and PD, but also had a potential relationship with IRRG.
The correlation between hub cross-talk genes and IRRGs
To observe the relationship between hub cross-talk genes and IRRGs, the overlapping between IRRGs and cross-talk genes were obtained and considered as the IRR cross-talk genes. The expression values of hub cross-talk genes and IRR cross-talk genes in the case sample of two diseases were obtained. The Pearson correlation coefficient analysis was used to analyze the relationship among hub cross-talk genes, as well as the correlation between hub cross-talk genes and hub IRRGs.
Hub cross-talk gene expression analysis
The expression matrix of hub cross-talk genes in periodontitis and PD were obtained, and the Wilcoxon test was performed to examine the significance of hub cross-talk genes. Meanwhile, the expression matrix of the hub cross-talk genes under different clusters were obtained. Periodontitis data set has two clusters, and Wilcoxon test was used to examine the significance of hub cross-talk genes. PD-related data set had three clusters, and Kruskal test was used to examine the significance of hub cross-talk genes. ROC analysis was carried out to investigate the predictive effects of hub cross-talk genes, based on the expression matrices of these genes in case-samples and control-samples.
Immune infiltrates
After downloading the immune cell-related data from TISDB database, we performed ssGSEA quantitative analysis was performed to calculate the abundance of immune cells in both diseases. The “GSVA” package (version 3.15) (Hänzelmann et al., 2013) was used in this analysis. The enriched and abundant immune cells were obtained through hierarchical clustering. The correlation between the abundant immune cells and other immune cells was analyzed by using Pearson correlation coefficient analysis. The Wilcoxon test was performed to analyze the differences of the abundance levels of these immune cells between case samples and control samples.
Correlation between immune cells and hub cross-talk genes
The fractions of abundant immune cells in the case samples and the expression of hub cross-talk gene in the case samples were obtained. The correlation between immune cells and the hub cross-talk genes were analyzed by using the Pearson correlation coefficient analysis.
Hub cross-talk gene PPI network and pathway network
The protein–protein interaction (PPI) relationship pair between the Hub cross-talk gene and other genes were extracted from the human protein reference database (HPRD)5 (Peri et al., 2004) and the biological general repository for interaction datasets (BIOGRID)6 (Oughtred et al., 2021). These interaction pairs were regarded as the hub cross-talk genes-target interaction pairs. The relevant genes under all pathways were procured from the Kyoto Encyclopedia of Genes and Genomes (KEGG) database7 (Kanehisa and Goto, 2000). The hub cross-talk gene-pathway relationship pair was firstly obtained. Afterward, the pathway-target relationship pair was obtained according to the pathways in the hub cross-talk gene-pathway interaction pairs. Therefore the hub cross-talk gene-pathway-target interaction pairs were identified, based on which the complex network of Hub cross-talk gene-Target and Hub cross-talk gene-Pathway-Target were constructed by using Cytoscape software (version 3.8) (Shannon et al., 2003).
Results
Data preprocessing
The three datasets related to PD (i.e., GSE6613, GSE49126, GSE99039) were merged, and then the PCA analysis was performed (Figure 2A). Figure 2A shows that the 3 datasets differed significantly. Figure 2B shows that the batch effect generated by merging datasets was significantly eliminated. By comparing Figures 2A,B, it was found that the differences between the three datasets were significantly reduced.
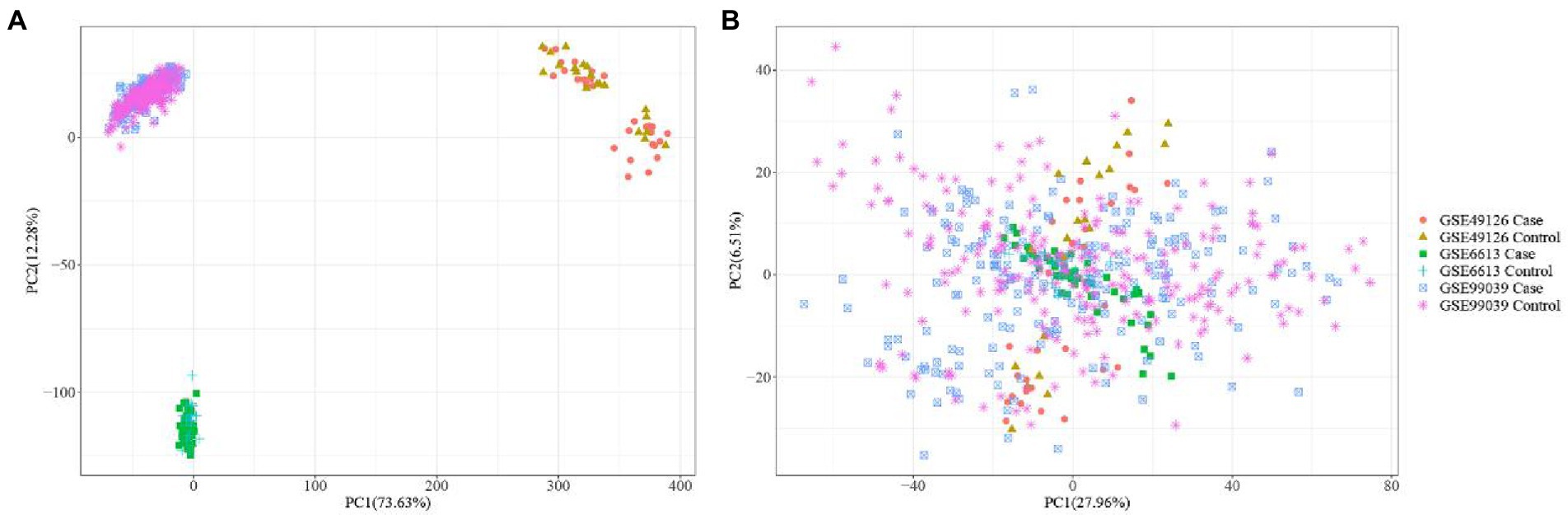
Figure 2. PCA analysis plots before (A) and after batch correction (B) of Parkinson’s disease related dataset.
Differentially expressed genes
Differential expression analysis was conducted for the periodontitis and PD-related datasets. For periodontitis-related dataset (GSE16134), the genes of P.adjust < 0.05 and |log2FC| > 0.5 were selected as DEGs, where log2FC >0.5 was the upregulated DEGs and log2FC < −0.5 was the downregulated DEGs. For PD-related datasets (GSE6613, GSE49126, GSE99039), the genes of P.adjust < 0.05 and | log2FC| > 0.15 were selected as DEGs, where log2FC > 0.15 were upregulated DEGs and log2FC < −0.15 were down-regulated DEGs. Table 2 shows the number of upregulated and downregulated DEGs deregulated in periodontitis and PD.
A volcano map was utilized to show the distribution of DEGs in Periodontitis- (Figure 3A) and PD-related data (Figure 3B). The top5 up-regulated and down-regulated DEGs with the most significant P.adjust values were labeled.
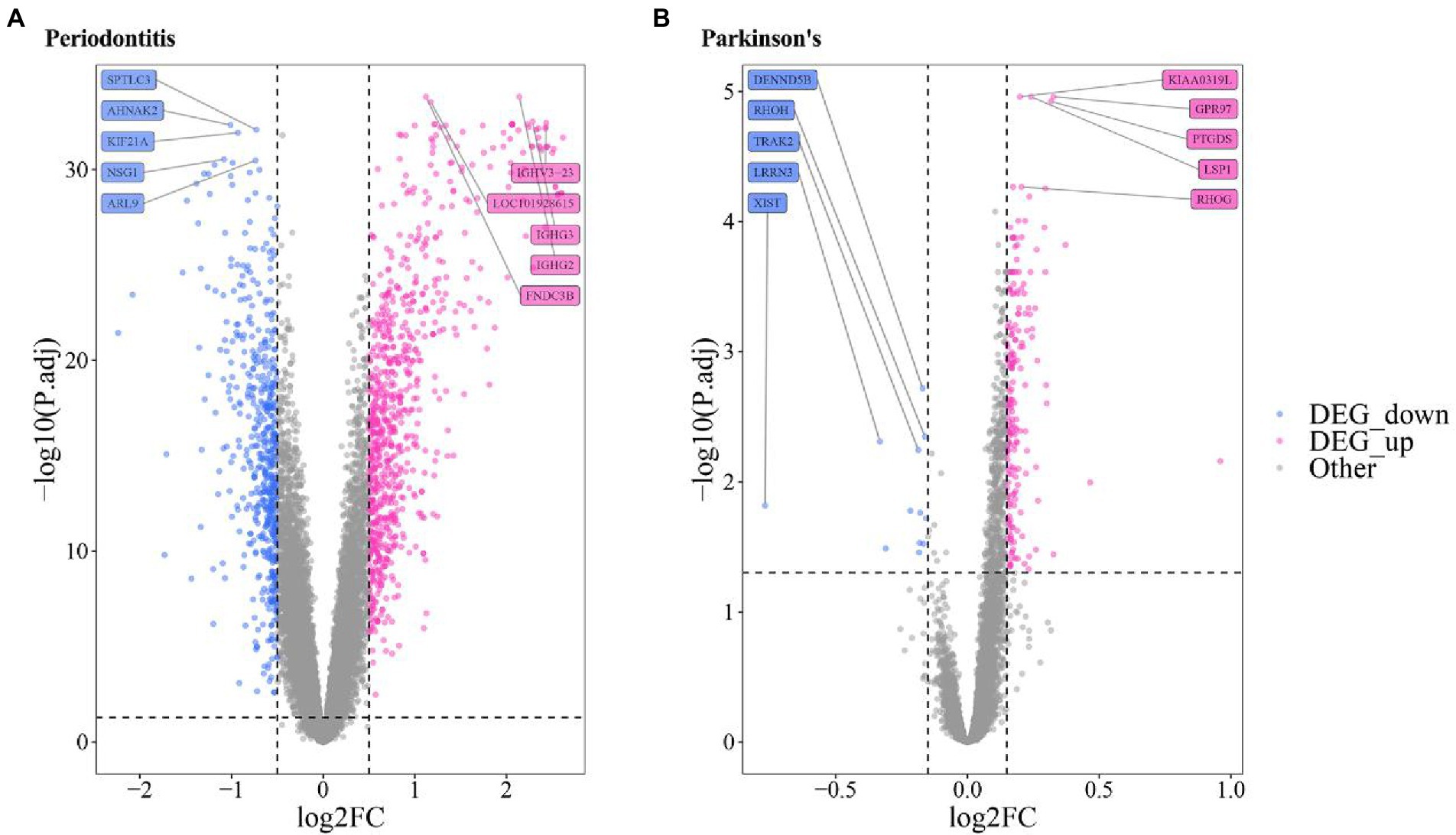
Figure 3. Volcanic map of the differentially expressed gene of (A) Periodontitis disease (A) and (B) Parkinson’s disease.
Cross-talk gene
The common DEGs of Periodontitis disease and PD were extracted, and thus 37 Cross-talk genes were obtained (Figure 4A). Then the expression values of 37 cross-talk genes in periodontitis and PD were extracted, base on which heatmaps were plotted for periodontitis (Figure 4B) and PD (Figure 4C).
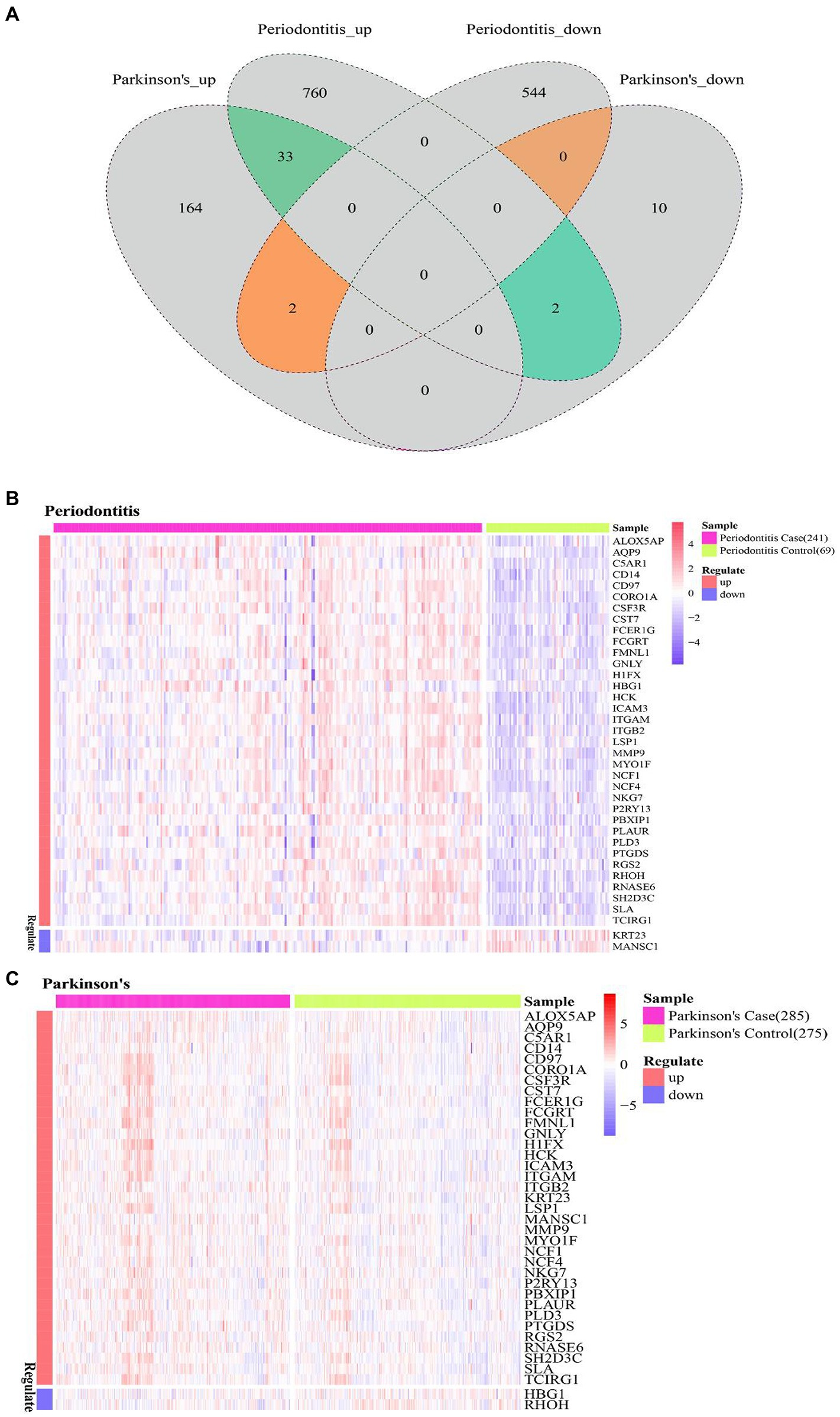
Figure 4. Cross-talk gene expression level. (A) Venn diagram of periodontitis disease and Parkinson’s disease differentially expressed genes; (B,C) cross-talk gene at the level of expression of Periodontitis disease and Parkinson’s disease.
The “ClusterProfiler” package in R was used to identify the BPs and KEGG pathways enriched by 37 cross-talk genes. The p < 0.05 was established as statistical significance and the top 20 terms were visualized (Figures 5A,B; Tables 3, 4).
The cross-talk genes were mainly involved in several BPs, for example, cellular defense response, leukocyte degranulation, neutrophil migration, and neutrophil activation involved in immune response (Figure 5A; Table 3). In addition, cross-talk genes regulate several KEGG pathways, for instance, phagotsome, leukocyte transendothelial migration, neutrophil extracellular trap formation, staphylococcus aureus infection, cell adhesion molecules, and Fc epsilon RI Signaling pathways (Figure 5B; Table 4).
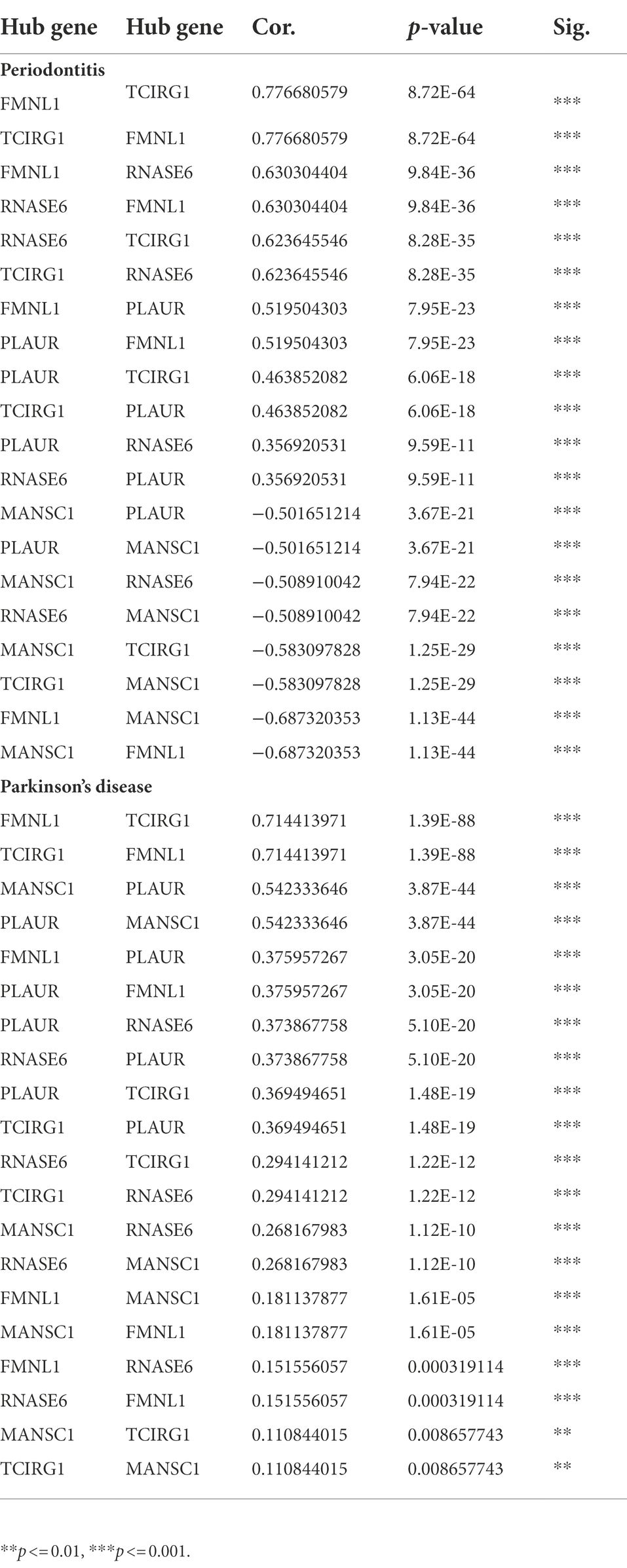
Table 5. The correlation among hub crosstalk genes in periodontitis and Parkinson’s disease, respectively.
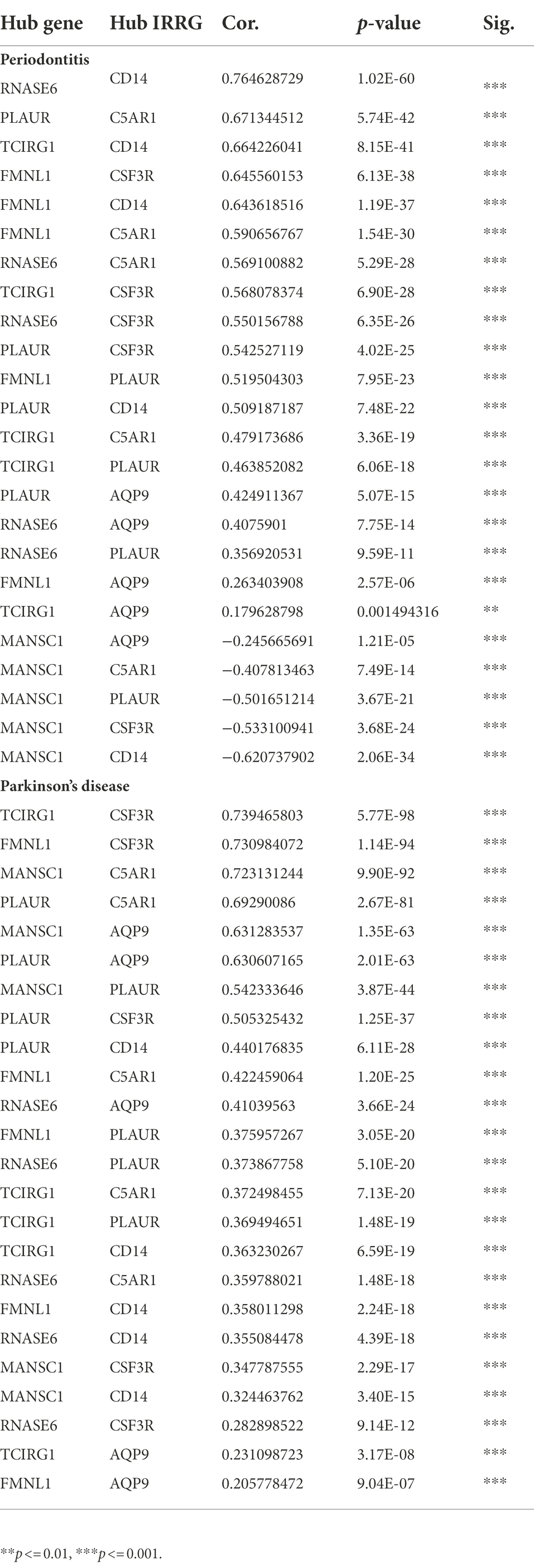
Table 6. The correlation between hub crosstalk genes and hub IRRGs in periodontitis and Parkinson’s disease.
Consensus cluster plus analysis results
The differentially expressed IRRGs in periodontitis and PD were extracted; thereby 47 periodontitis-related IRRGs and 9 PD-related IRRGs were identified. The expression values of periodontitis-related IRRGs and PD-related IRRGs were obtained, respectively. Afterward, the IRRG-related expression profiles were analyzed by using the average silhouette width and elbow method (Figures 6A–D).
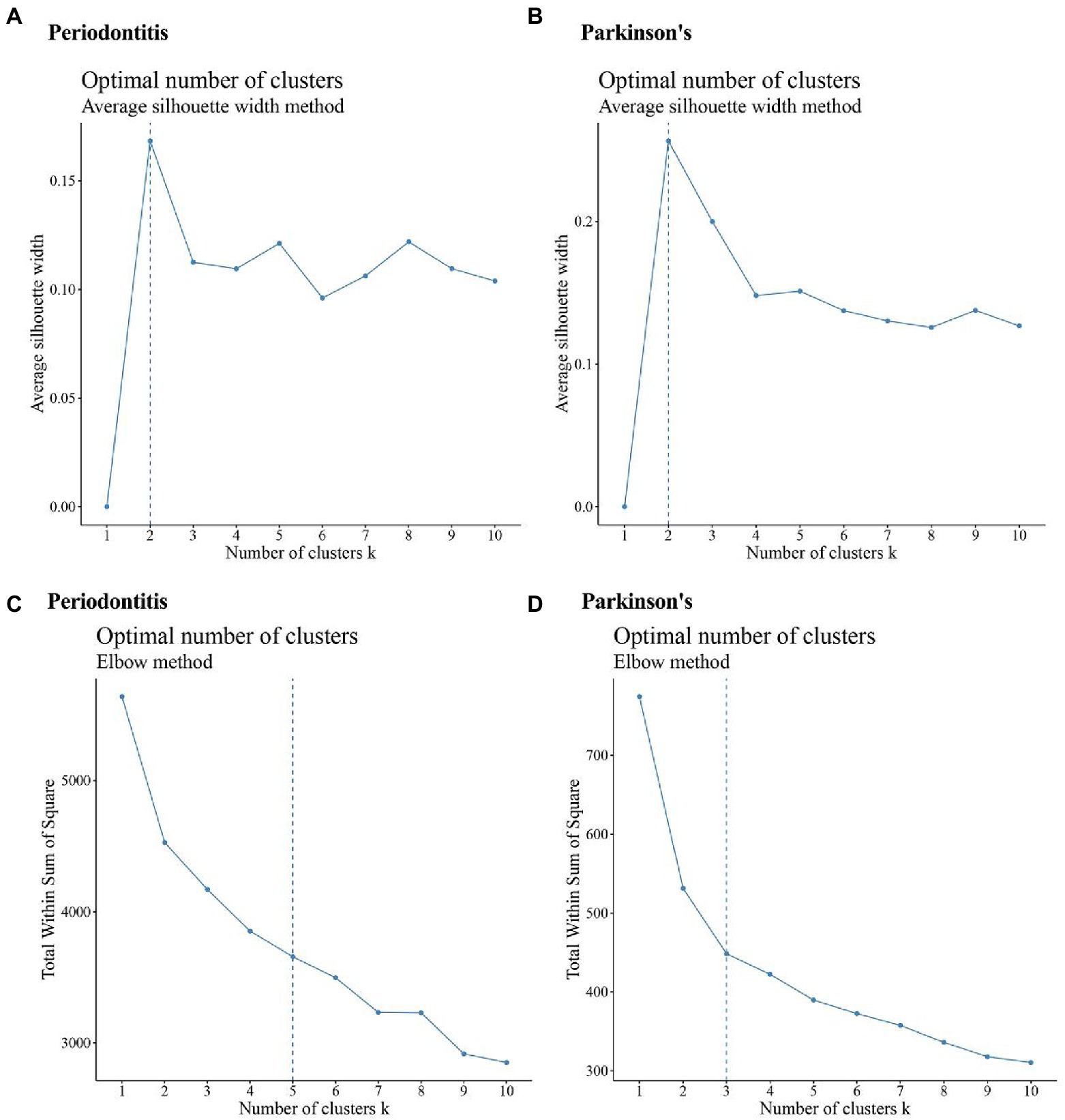
Figure 6. Results of a cluster analysis of periodontitis and Parkinson’s disease. (A,B) Results of a silhouette width analysis of the IRRGs associated with periodontitis and the average of IRRGs associated with Parkinson’s disease; (C,D) Results of an elbow method analysis of Periodontitis disease-related IRRGs and Parkinson’s disease-related IRRGs.
The clusters’ numbers of Periodontitis disease were determined as 2 (Figure 6A) and 5 (Figure 6C) respectively. Based on this, the maxK parameter was set as 5 when periodontitis dataset was calculated by using “Consensus Cluster Plus” function. The clusters’ numbers of PD are 2 (Figure 6B) and 3 (Figure 6D). Based on this, the maxK parameter was set as 3 when PD-related data set was calculated by using “Consensus Cluster Plus” function.
By using “Consensus Cluster Plus” function, two diseases’ clustering results were obtained (Figures 7A–D). Figures 7A,C show that the periodontitis-related data achieved the best clustering effects when k = 2. Figures 7B,D show that the PD-related data achieved the best clustering effects when k = 3. Figures 7E,F shows the sample correlation results when k = 2 being selected for periodontitis data (Figure 7E) and when k = 3 being selected for PD data (Figure 7F).
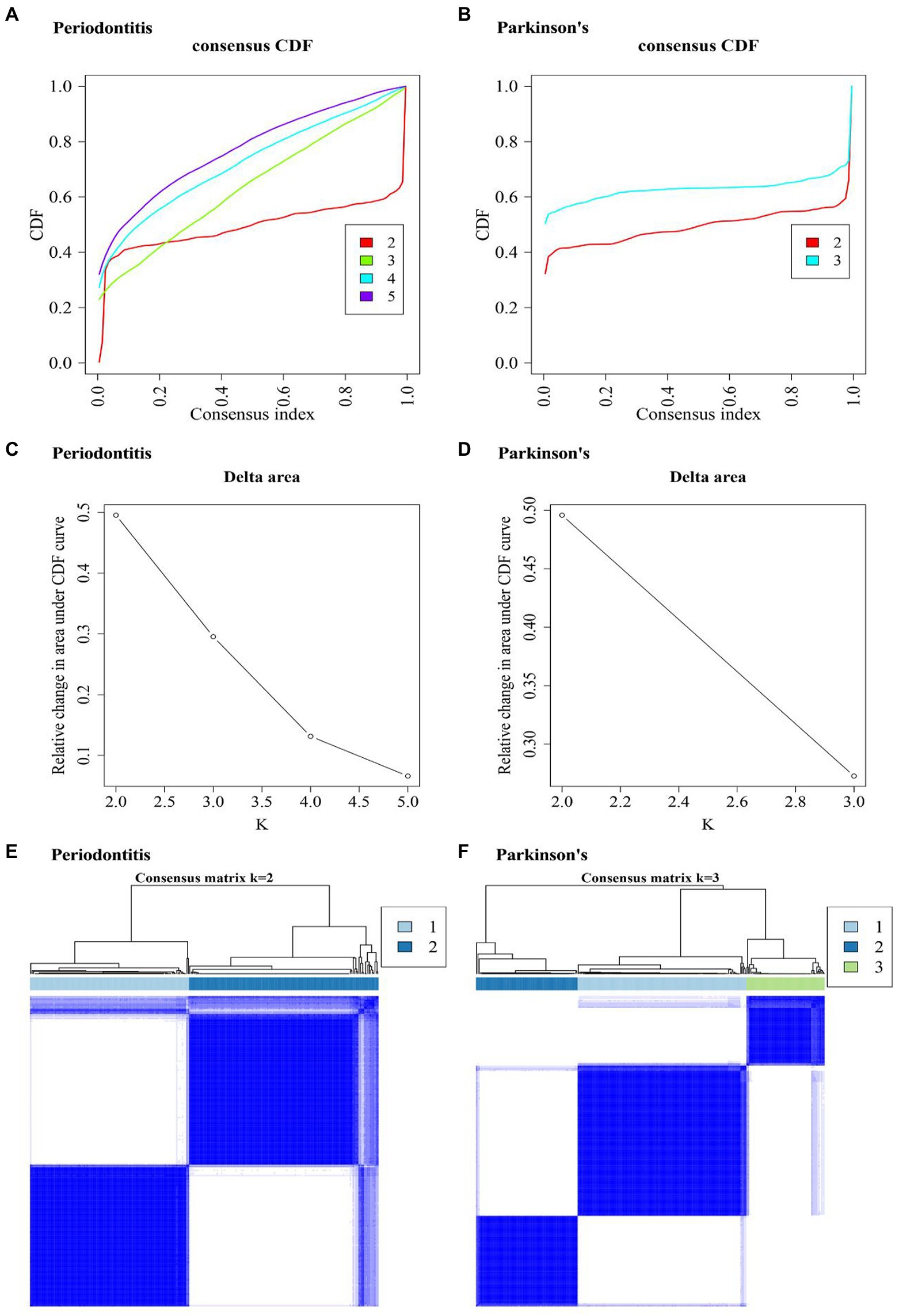
Figure 7. Consensus Cluster Plus analysis results. (A,B) Consistent cumulative distribution function (CDF) plot of periodontitis and Parkinson’s disease. This plot shows the cumulative distribution function of the score when k takes different values, which is used to determine when k is taken, CDF reaches an approximate maximum, and the cluster analysis results are the most reliable. That is, consider the k-value of the CDF descent slope is small. (C,D) The k and k-1 relative changes in area under the CDF curve in periodontitis and Parkinson’s disease. The total area under the CDF curve at k = 2 (the area below the line in (A,B) rather than the relative change in area). To select the final k-value, we should consider that the descending slope of the midline of (A,B) is as small as possible, and also consider that the relative change in the area under k and k-1 in (C,D) compared to the CDF curve is as small as possible. (E,F) Consistent cluster diagram of periodontitis and Parkinson’s disease.
Hub cross-talk genes screening
A multi-group differential expression analysis of cross-talk genes were carried out based on the clusters of two diseases, and genes with P.adjust < 0.01 were selected to be the DE-cluster cross-talk genes. The DE-cluster cross-talk genes for both diseases were overlapped and thereby 26 common DE-cluster cross-talk genes were obtained (Figure 8A). Based on the clusters of both diseases, LASSO regression analysis was used to further screen the DE-cluster cross-talk genes (Figures 8B–E). After further screening for DE-cluster cross-talk genes common to both diseases, 5 Hub cross-talk genes (i.e., FMNL1, MANSC1, PLAUR, RNASE6, TCIRG1) were obtained (Figure 8F).
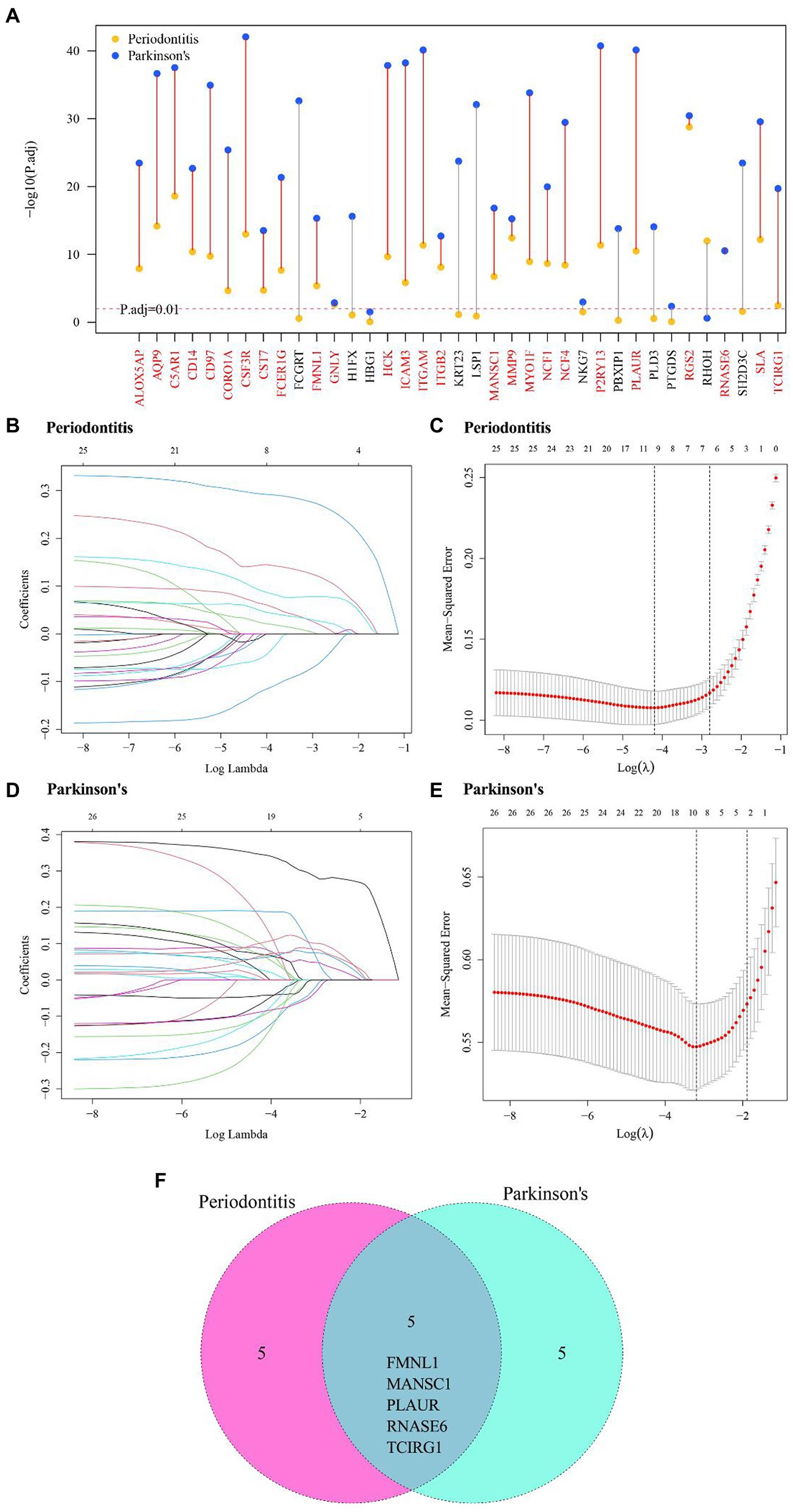
Figure 8. Hub cross-talk gene screening. (A) DE-cluster cross-talk gene of periodontitis and Parkinson’s disease’s. (B–D) Results of LASSO analysis for periodontitis and Parkinson’s disease. Each line in the graph represents a gene, and a larger value of the log lambda as the gene tends to 0 indicates that the gene is more critical. (C–E) cross-validation results of Periodontitis disease and Parkinson’s disease model. (F) Periodontitis disease and Parkinson’s disease’s hub cross-talk genes.
Correlation between hub cross-talk genes and hub IRRGs
The inflammatory response-related cross-talk genes were obtained and 5 genes (i.e., AQP9, C5AR1, CD14, CSF3R, PLAUR) were labeled as hub IRRGs. The expression values of 5 hub IRRGs and 5 hub cross-talk genes in the case sample of periodontitis and PD were obtained. Afterward, the relationship among the hub cross-talk genes, as well as the relationship between the hub cross-talk genes and the hub IRRGs were analyzed by using the Pearson correlation coefficient analysis (Figures 9A,B; Tables 5–6). MANSC1 was significantly negatively correlated with 4 hub cross-talk genes in periodontitis, and MANSC1 was significantly negatively correlated with 5 hub IRRGs. There was a significant positive correlation among the remaining 4 hub cross-talk genes, and the remaining 4 Hub cross-talk genes were also significantly positively correlated with 5 Hub IRRGs (Figure 9A). Five hub cross-talk genes were significantly positively correlated with five hub IRRGs in PD. Among these correlation, the correlation between CD14 and five hub cross-talk genes showed comparatively higher correlation (Figure 9B).
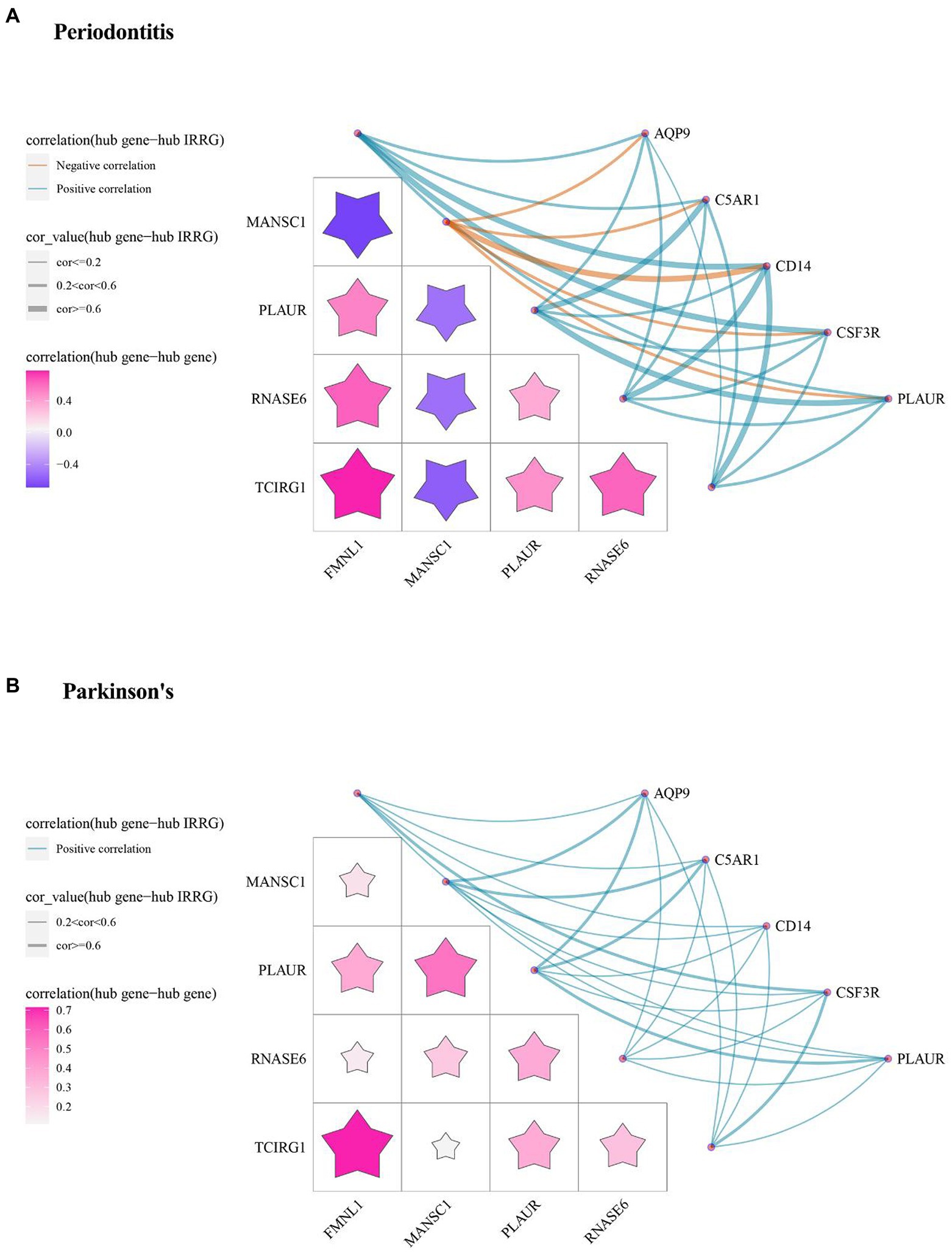
Figure 9. The relationships among Hub cross-talk genes and Hub IRRG in (A) Periodontitis disease and (B) Parkinson’s disease. The matrix graph in the lower left part of the figure shows the correlation coefficient between the hub cross-talk gene and the hub cross-talk gene, and the upper right part of the network diagram shows the correlation coefficient between the hub cross-talk gene and the hub IRRG.
Hub cross-talk genes’ expression level analysis
The expression values of five hub cross-talk genes in periodontitis and PD were obtained. The Wilcoxon test results showed that five hub cross-talk genes had significant differences between disease samples and normal samples (Figures 10A,B). Afterward, the expression matrix of the five hub cross-talk genes in different clusters were obtained. The Wilcoxon test and Kruskal test results showed that the five hub cross-talk genes had significant differences between different cluster samples (Figures 10C,D). Furthermore, ROC analysis results showed that the AUC values of all five hub cross-talk gene in periodontitis were greater than 70% (Figure 10E). The AUC values of the five hub cross-talk gene in PD were greater than 58%. The AUC values of FMNL1 and PLAUR in PD were comparatively higher compared to the remaining three hub cross-talk genes (Figure 10F).
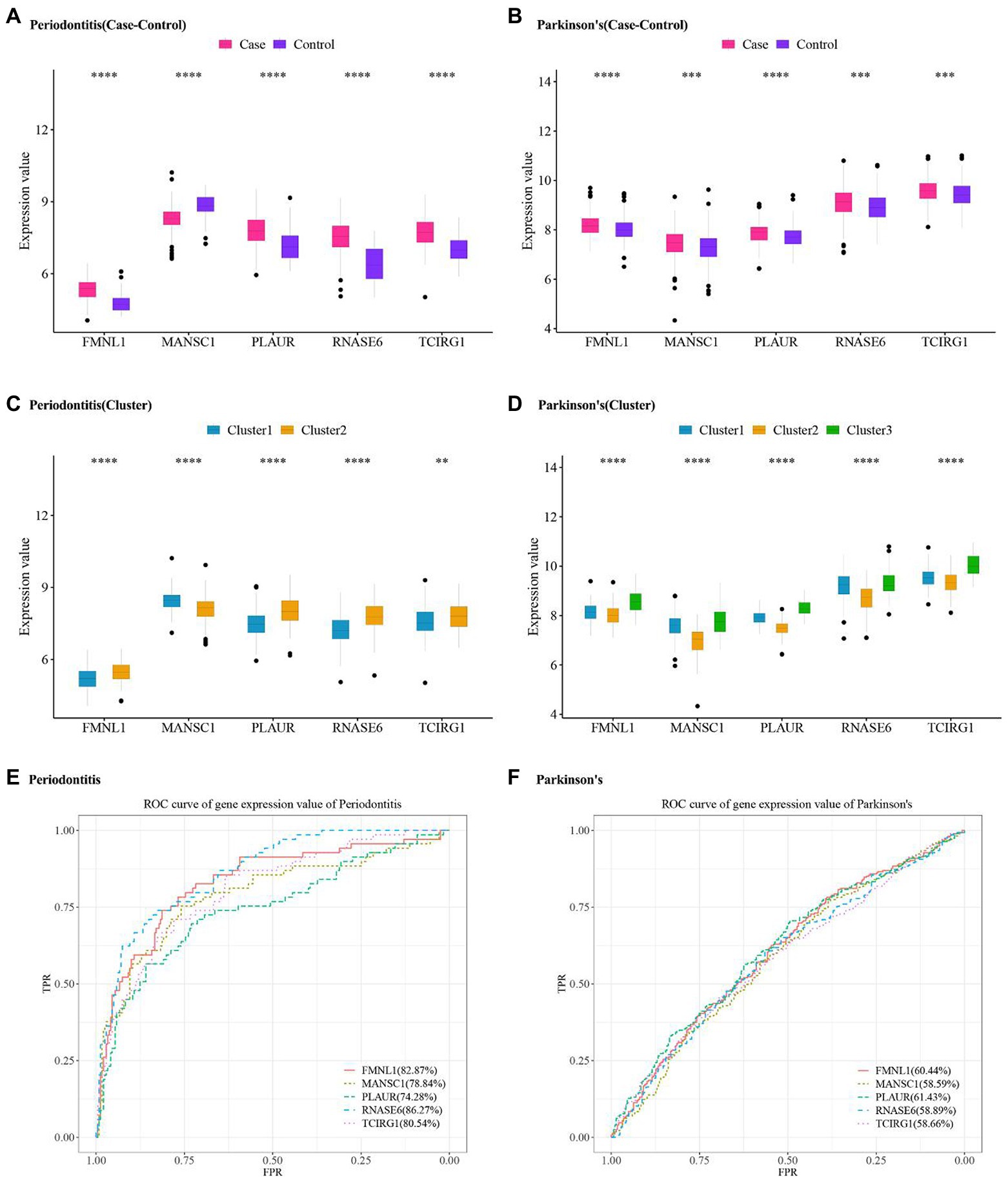
Figure 10. Hub cross-talk gene expression level and ROC analysis in periodontitis and Parkinson’s disease. (A,B) Hub cross-talk gene expression levels and differences between disease samples and normal samples in periodontitis and Parkinson’s disease, respectively. (C,D) Hub cross-talk gene expresses levels and differences in different clusters of periodontitis and Parkinson’s disease. The smaller the test result, the more “*” on the plot, and the correspondence between the p value and the “*” sign is ns: p > 0.05, *: p ≤ 0.05, **: p ≤ 0.01, ***: p ≤ 0.001, ****: p ≤ 0.0001. (E,F) ROC analysis results regarding the diagnostic accuracy of hub cross-talk genes in periodontitis and Parkinson’s disease.
Immune infiltration analysis of periodontitis and PD
The immune cell-related dataset were obtained from TISDB database, which included 782 genes and 28 types of immune cells. The immune infiltration quantification analysis was performed by using the ssGSEA algorithm, and the enrichment fractions of immune cells in periodontitis and PD were obtained. The “pheamap” package of R was used to demonstrate immune-infiltrating cell scores for case samples in periodontitis and PD. The results showed that central memory CD4 T cell, MDSC, Effector memory CD8 T cell, plasmacytoid dendritic cell, activated CD8 T cell, activated dendritic cell and monocyte were highly expressed in periodontitis and PD (Figures 11A,B).
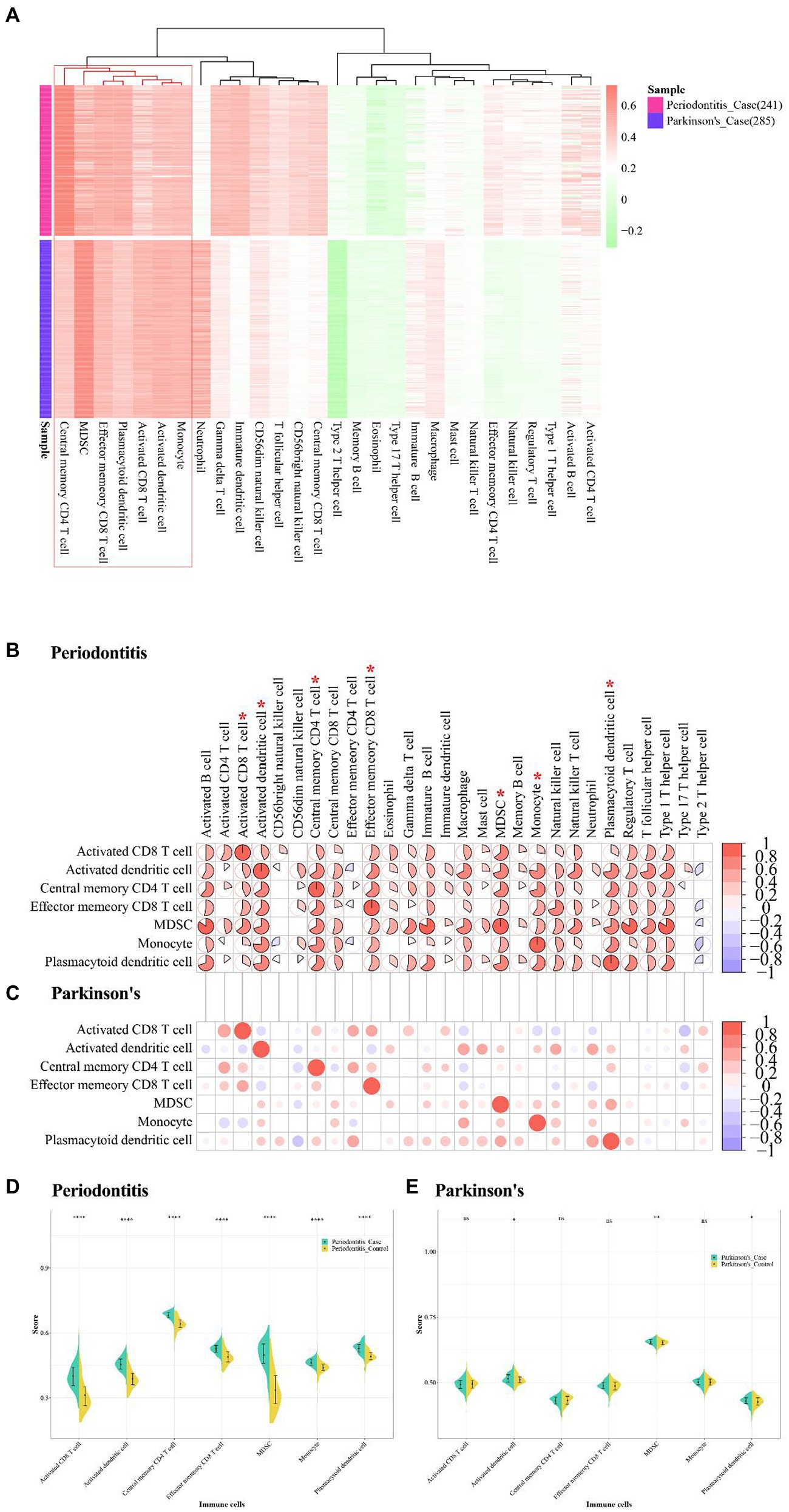
Figure 11. Immune infiltrative analysis of Periodontitis disease and Parkinson’s disease. (A) The abundance fraction of immune cells in disease samples of Periodontitis disease and Parkinson’s disease. (B,C) Correlation of higher abundance immune cells and other immune cells in Periodontitis disease and Parkinson’s disease. (D,E) Immune cell enrichment fractions in different sample types of Periodontitis and Parkinson’s disease.
The correlation between these high-abundance immune cells and other immune cells were analyzed by using the Pearson correlation coefficient, and the immune cells with value of p <0.05 were visualized (Table 7). The results showed that MDSC was highly positively related with active B cell, type 1 T helper cell, regulatory T cell, and immature B cell in periodontitis (cor > 0.8; Figure 11C). Activated CD4 T cell and Activated CD8 T cell were positively correlated in PD (cor = 0.6; Figure 11D). The active CD4 T cell was positively correlated with activated CD8 T cell in periodontitis.
The violin plots were used to show the fractional distribution of high-abundance immune cells in periodontitis and PD, and the Wilcoxon test was applied to observe the significance of samples. The results showed that the high abundance of immune cells varied greatly between case samples and normal samples in periodontitis (Figure 11D). The high-abundance immune cells differed less between case samples and normal samples in PD (Figure 11E). The effector memory CD8 T cell was highly enriched in both periodontitis and PD.
Relationship between high-abundance immune cells and hub cross-talk genes
The case sample scores for 7 high-abundance immune cells and 5 hub cross-talk genes were obtained. The correlation analysis was carried out to invesitgate the correlation between immune cells and hub cross-talk genes. The relationship pairs with value of p < 0.05 was defined to be significant. The absolute value of correlation coefficient was calculated and the results of the top12 were visualized. The results shwoed that RNASE6 gene was highly correlated with MDSC in periodontitis (Figure 12). MANSC1 was highly correlated with plasmacytoid dendritic cell in PD (Figure 13; Table 8).
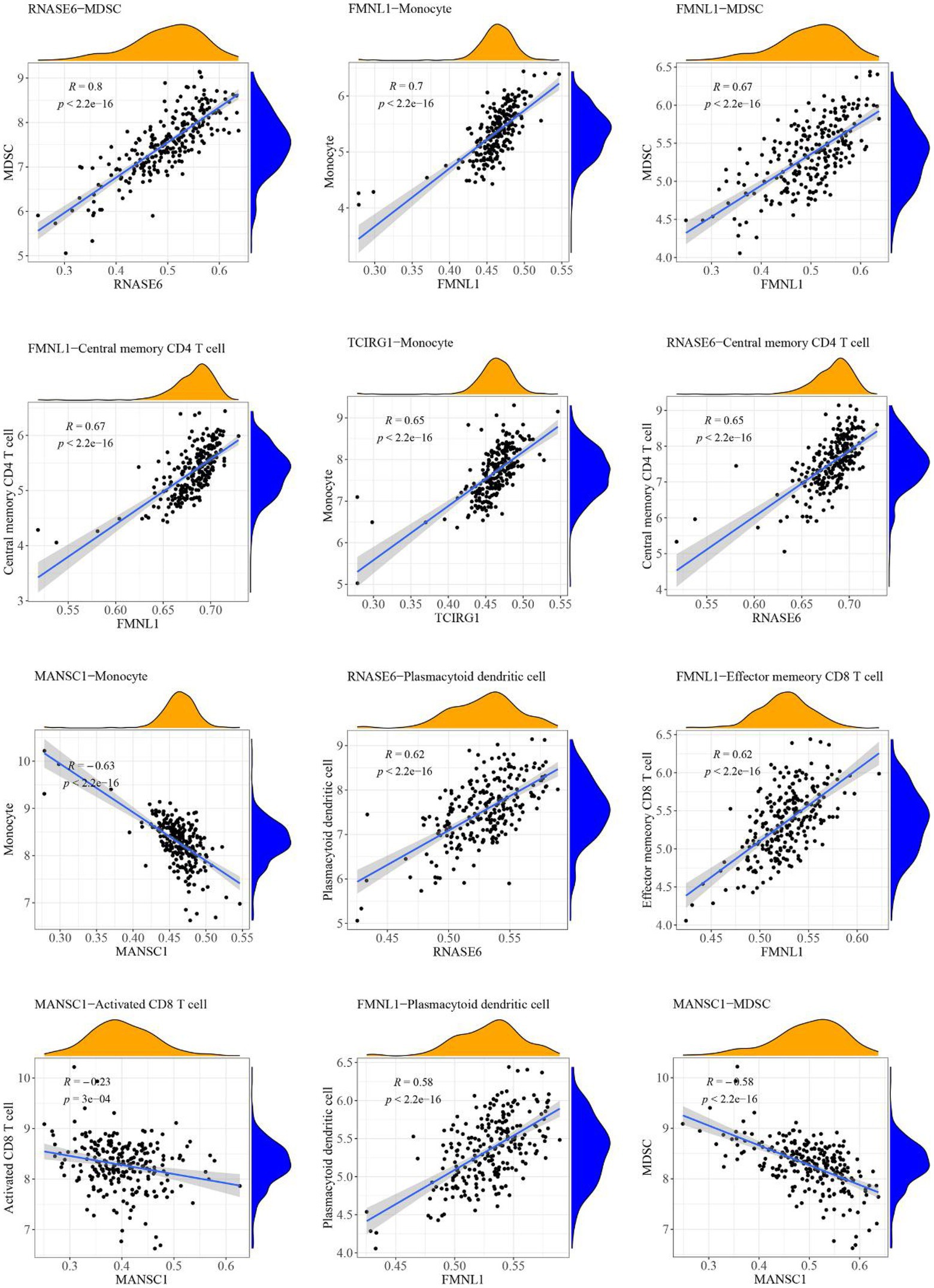
Figure 12. Relationship between high-abundance immune cells and hub cross-talk genes in periodontitis.
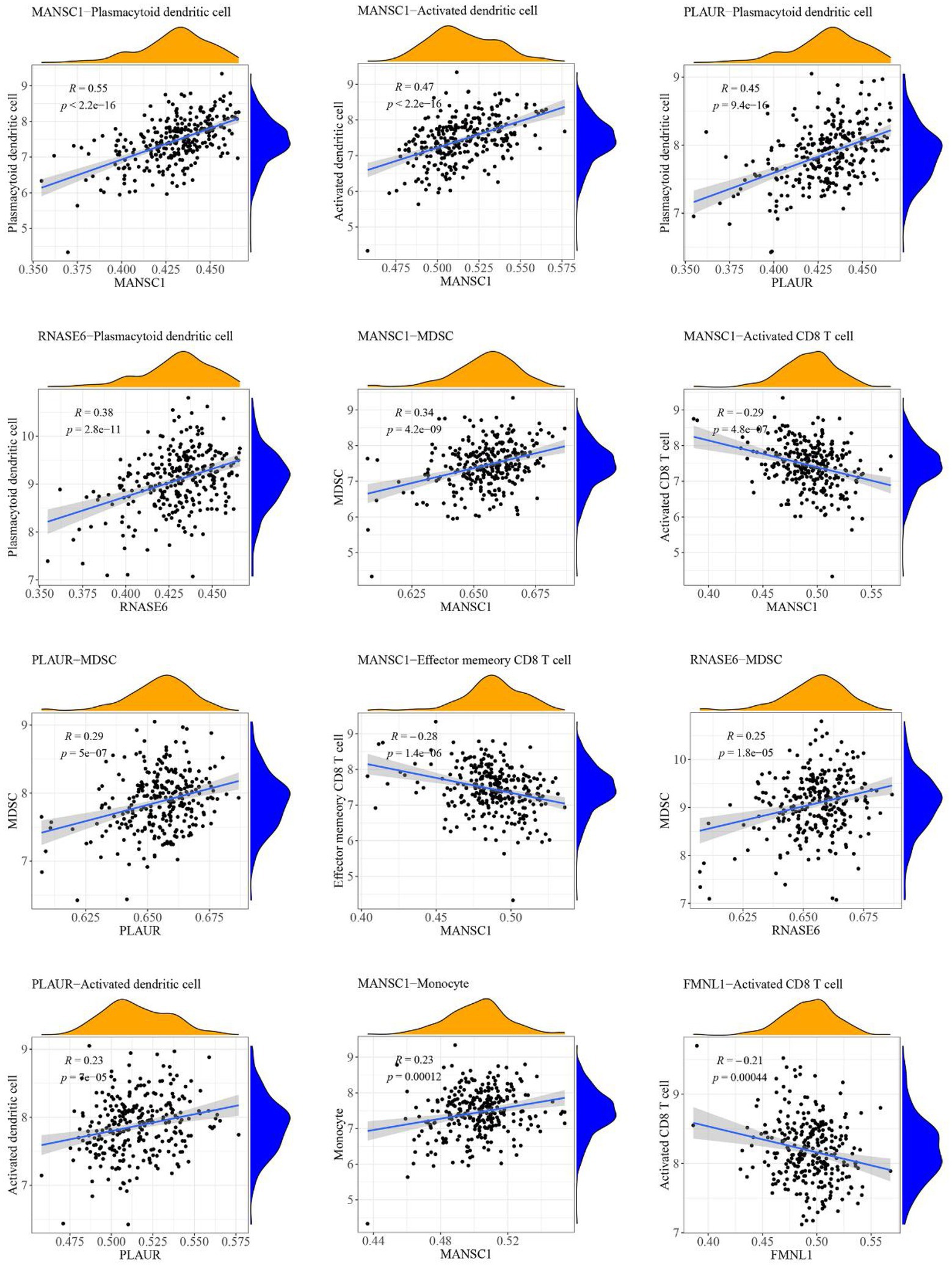
Figure 13. Relationship between high-abundance immune cells and hub cross-talk gene in Parkinson’s disease.
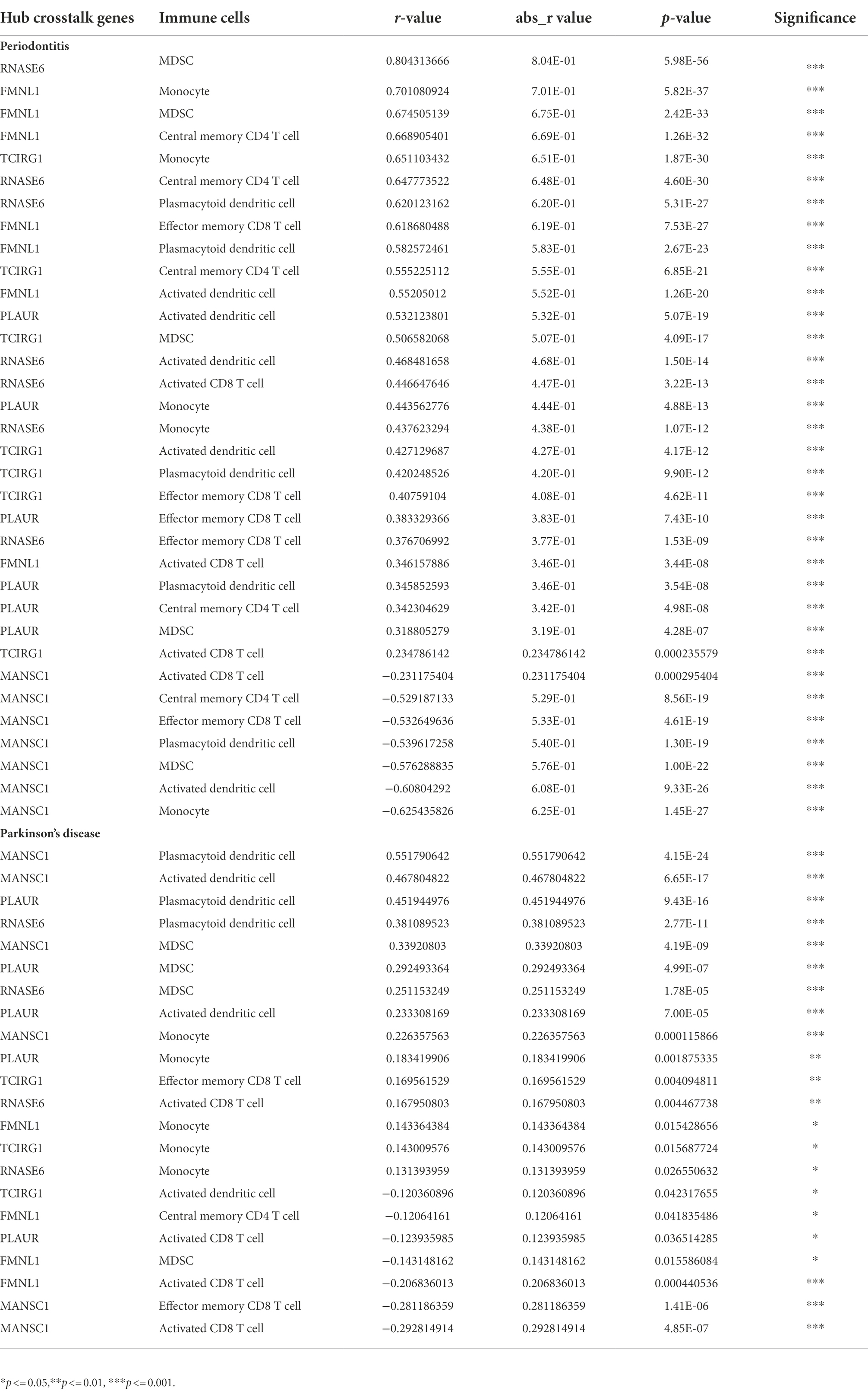
Table 8. The correlation between hub crosstalk genes and immune cells in periodontitis and Parkinson’s disease, respectively.
Hub cross-talk gene PPI-pathway network
The 192 hub cross-talk gene-target interaction pairs were obtained from the HPRD and BIOGRID database. Based on the KEGG database, 105 hub cross-talk gene-pathway-target interaction pairs were obtained. The Cytoscape software was used to integrate the hub cross-talk gene-target pairs with the hub cross-talk gene-pathway-target interaction pairs; thereby a hub cross-talk gene related complex network was constructed (Figure 14). Figure 14 shows that PLAUR interacted with other genes and regulated the composite and coagulation cascades pathway. TCIRG1 interacted with other hub cross-talk genes and was involved in regulating oxidative phosphorylation pathway and phagosome pathway.
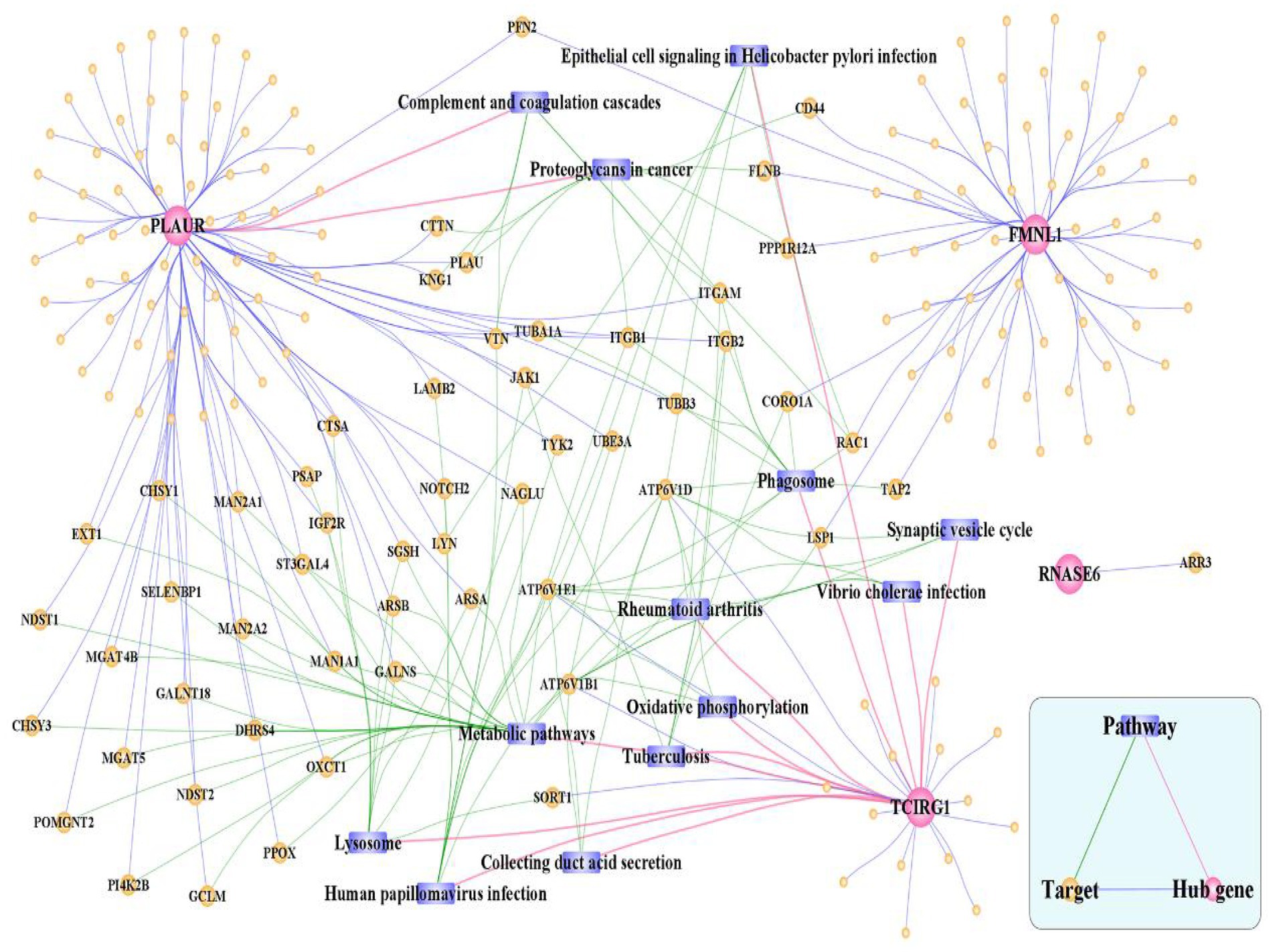
Figure 14. Hub cross-talk gene related complex network. The network consists of 210 nodes and 303 edges.
Discussion
The main findings of the current study identified five hub crosstalk genes (i.e., FMNL1, MANSC1, PLAUR, RNASE6, and TCIRG1) to be the linking mechanisms between periodontitis and PD. In addition, this study also identified several signaling pathways enriched by the crosstalk genes, for example, complement and coagulation cascades, neutrophil extracellular trap formation, transendothelial leukocyte migration, and phagosome formation. In this section, previous literature were reviewed to explain the linking role of these genetic factors in the pathogenesis of both diseases.
The formin FRL1 (FMNL1) resides in the actin-rich cores of primary macrophage podosomes. During adhesion and migration of macrophages, FMNL1 is responsible for modifiying actin at the podosome in macrophages (Mersich et al., 2010). By stabilizing podosome lifespans without interacting with fast-growing actin termini, FMNL1 promotes migration and recruitment activity of macrophages (Miller et al., 2017). Additionally, FMNL1 is upregulated in the course of monocyte differentiation to macrophages (Mersich et al., 2010), which might be used for explaining the significant correlation between FMNL1 and monocytes in periodontitis (Table 8: r = 0.701080924; p = 5.82E-37) and PD (Table 8: r = 0.143364384; p = 0.015428656). The potential role of FMNL1 in enhancing macrophage activity might be involved in the mechanism of periodontitis inducing PD progression. In periodontitis, overgrowth of Gram-negative bacteria and LPS access to the blood circulation may enhance the activation of macrophages in peripheral blood (Pussinen et al., 2004). One of the hallmarks of PD is the impairment of BBB integrity and function (Al-Bachari et al., 2020), due to which immune cells, including macrophage clusters, can infiltrate from the peripheral blood into the CNS (Su and Zhou, 2021). The periodontal pathogen, Escherichia coli (E. coli), can also penetrate the impaired BBB of a PD patient. In a study, the LPS extracted from the cell wall of E. coli was found to induce rapid and intense activation of microglia and macrophages (Blaylock, 2017). The macrophages stimulated by LPS caused robust neurotoxicity and immunoexcitotoxicity, which may play a central role in PD-associated neurodegeneration progression (Blaylock, 2017). Based on these studies, it may be suggested that the crosstalk gene FMNL1 is a common link between periodontitis and PD via macrophage activation. However, there is no published research investigating the deregulation and function of FMNL1 in linking periodontitis and PD to date.
The Plasminogen Activator Urokinase (PLAU) gene encodes the receptor for Urokinase-type Plasminogen Activator (uPA). uPA has a role in localizing and promoting plasmin formation and was found to be implicated in the pathological processes associated with cell-surface plasminogen activation and localized degradation of the extracellular matrix. In periodontitis, uPA converts plasminogen to plasmin, leading to the uPA proteolytic cascade activated by P. gingivalis and inducing tissue destruction, particularly, alveolar bone loss (Fleetwood et al., 2015). Additionally, uPA was found to be a marker of macrophage activation based on its role in regulating macrophage motility and macrophage-mediated matrix degradation in periodontitis (Fleetwood et al., 2014). In PD, uPA was found to act as an activator protease in the process of plasminogen activation and upregulate the expression of the serine protease inhibitor (serpin) plasminogen activator inhibitor-1 (PAI-1), leading to neuroinflammation (Reuland and Church, 2020). Further, an increased expression of Urokinase Plasminogen-activator Receptor (uPAR) was observed in activated microglia in the brain of a patient with another neurodegenerative disease (Alzheimer’s disease), and uPAR expression was found to be mediated by oxidative stress-related mechanisms (Walker et al., 2002).
TCIRG1 (T Cell Immune Regulator 1, ATPase H+ Transporting V0) encodes the a3 isoform of V-ATPase a subunit, which is essential for osteoclastic bone resorption. The promoter activity of serial-deletion fragments of the TCIRG1 gene promoter was observed to be enhanced throughout the osteoclastic differentiation process of osteoclast RAW264.7 cells, and such alteration was found to be induced by the receptor activator of nuclear factor kB ligand (RANKL) (Beranger et al., 2007). TCIRG1 expression was found to induce the bone resorption activity of osteoclasts in periodontal disease (Heo et al., 2022). TCIRG1 was highly expressed in myeloid cells and involved in myeloid cell activation and microglial neuroinflammation (Funatsu et al., 2022). The mRNA expression of TCIRG1 gene was recently examined in human alloactivated T lymphocytes, which indicates that TCIRG1 was essential in T cell activation (Heinemann et al., 1999). Our present study found that the TCIRG1 expression level was positively correlated with effector memory cluster of differentiation (CD)8+ T cells (r = 0.41; p = 4.62E-11) in periodontitis. Similarly, a positive and significant correlation was found between the TCIRG1 expression level and effector memory CD8+ T cells (r = 0.17; p = 0.004) in PD. In periodontitis, the CD8+ T cells with an effector memory phenotype were shown to release anti-inflammatory cytokines (interleukin [IL]-10 and transforming growth factor [TGF]-β) and suppress bone-destructive cytokines (interferon [IFN]-γ and IL-17), and thus play a protective role for the alveolar bone (Cardoso and Arosa, 2017). In PD, the cytotoxic attack of a robust CD8+ T cell infiltration might initiate and propagate neuronal death and synucleinopathy by secreting cytolytic enzymes (granzymes A, B, and K) and/or pro-inflammatory cytokines (IFN-γ) (Galiano-Landeira et al., 2020). These studies validated the possibility of our findings that the hub crosstalk gene TCIRG1 links periodontitis and PD via regulating effector memory CD8+ T cells.
The complement and coagulation cascade pathway was found to be enriched by the crosstalk genes linking periodontitis and PD. The complement system plays a vital role in immune surveillance, homeostasis, and bridges the innate and adaptive immune systems (Ricklin et al., 2010). A study showed that suppressing component 3 (C3) in the complement cascade directly inhibits periodontal inflammation and indirectly counteracts dysbiosis, thus showing promising clinical potential for treating periodontitis (Hajishengallis et al., 2019). The complement C3-positive astrocytes were increased in the ventral midbrain of the intrastriatal α-synuclein preformed fibril (PFF)-injected mice, and C3 secreted from astrocytes could induce the degeneration of dopaminergic neurons, suggesting the potential involvement of complement and coagulation cascades in dopaminergic neurodegeneration in PD (Ma et al., 2021). Considering the involvement of complement system activation in both periodontitis and PD, this pathway might link both diseases by means of neuroimmune interaction. The complement system activation triggered by periodontitis may regulate the migration and invasion function of the peripheral immune cells (Merle et al., 2015), which may penetrate the damaged BBB and induce neuroinflammation and neurodegeneration in PD patients (Chao et al., 2014). Apart from the complement and coagulation cascade pathway, oxidative phosphorylation was identified to be an important pathogenic pathway contributing the linkages between both diseases. It is through oxygen oxidative phosphorylation that oxygen inhaled by the body is used to produce energy (Cole, 2016). Mutations in mitochondrial DNA result in reduced efficiency of oxidative phosphorylation and ATP production, overproduction of ROS, and a significant reduction in mitochondrial membrane potential (MMP) levels in pathophysiological conditions (Xu et al., 2021). The downregulation of PD-related gene-complex I was found to be involved in oxidative phosphorylation by leading to reduced ATP formation in neurons and further inducing neuronal apoptosis (Ali and Dholaniya, 2022). In addition, P. gingivalis infection was found to promote mitochondrial fragmentation and dysfunction, increase the levels of mitochondrial reactive oxygen species (mtROS), and upregulate the phosphorylation of Drp1 gene (Xu et al., 2021).
The current study found that several immune cells (e.g., central memory CD4 T cells, effector memory CD8 T cells, activated CD8 T cells, myeloid-derived suppressor cells (MDSCs), plasmacytoid dendritic cells, activated dendritic cells, and Monocytes) were highly expressed in periodontitis disease and PD. Among these immune cells, MDSCs and dendritic cells obtained our particular interest. It is believed that MDSCs have the most potential for restoring homeostasis after inflammation, as well as being able to suppress adaptive immunity by suppressing T cell response (Kauppinen et al., 2020). The MDSCs was detected to be significantly increased in peripheral blood of patients with PD compared with healthy control individuals, which led to the increased production of immunosuppression-related genes [arginase 1 (ARG1), interleukin-10 (IL-10), and cyclooxygenase 2 (COX-2)] (Yang et al., 2018). MDSCs were also found to be expanded, activated, and recruited as the result of the inflammatory response induced by P. gingivalis infection in periodontitis (Valero-Monroy et al., 2016; Su et al., 2017). As professional antigen-presenting cells, dendritic cells link innate and adaptive immunity and are vital to the induction of protective immune responses against pathogens (Liu et al., 2021). Peripheral blood dendritic cells in chronic periodontitis was found to carry P. gingivalis. Such microbial carriage state not only enhanced the differentiation of monocytes into immature myeloid dendritic cells, but also promoted the production of matrix metalloproteinase-9 and upregulated C1q, heat shock protein 60, heat shock protein 70, CCR2, and CXCL16 (Carrion et al., 2012). The recruitment of activated subsets of dendritic cells in the brain was found to increase the production of pro-inflammatory cytokines (e.g., TNFα, IL1β, and IL6) and aggregate alpha synuclein (Agg α-syn) fuels neuroinflammation in PD (Magnusen et al., 2018).
It is noteworthy to highlight the limitation of the current study. The genetic factors identified in the current study are obtained solely using computational prediction based on the periodontitis-and PD-associated datasets; however, these factors were not validated by performing related experiments. The representative periodontal pathogen P. gingivalis or its derived LPS could be used for infecting the microglia and its activated immune and inflammation-related signaling pathways could be examined. The transcriptomic alterations of microglial cells under such stimulation can be examined by performing next generation sequencing approach. The animal model with PD could be established to identify the influence of P. gingivalis or its derived LPS on the immune cells in the brain. Another limitation is regarding the GEO datasets analyzed in the current research. Although four datasets were included and analyzed in the current research, the sample size included in each dataset restricted the prediction accuracy of the results. In addition, there is no public dataset integrating both diseases and investigating the alteration in mRNA expression of the peripheral blood tissue in PD with/without periodontitis. Nevertheless, this study has potential for clinical application by suggesting putative genetic mechanisms underlying the increased risk of periodontitis in PD progression. The five hub crosstalk genes discussed in this study hold promise to be developed as a chair-side kit for predicting the risk of PD in elderly periodontitis patients. Further investigation is needed to validate the prediction accuracy of these genetic findings.
Conclusion
Five genes (i.e., FMNL1, MANSC1, PLAUR, RNASE6, and TCIRG1) were identified as crosstalk biomarkers linking PD and periodontitis. The significant correlation between these crosstalk genes and immune cells strongly suggests the involvement of immunology in linking both diseases.
Data availability statement
The original contributions presented in the study are included in the article/Supplementary material, further inquiries can be directed to the corresponding author.
Author contributions
SHu and SL: conceptualization, funding acquisition, methodology, formal analysis, and writing—original draft. WN, XH, XL, YD, DF, AO, BL, VS, HL, SG, RZ, and DZ: methodology, formal analysis, review, and editing. GS, SHu, and SHuang: project administration and supervision. All authors contributed to the article and approved the submitted version.
Funding
We appreciate the funding by the Science Research Cultivation Program of Stomatological Hospital, Southern Medical University (Grant No.: PY2022001 for supporting the postdoc research of Dr. Shaonan Hu (Email: c2hhb25hbl9odUBzbXUuZWR1LmNu) and Grant No.: PY2020004 for supporting the postdoc research of Dr. Simin Li (Email: c2ltaW4ubGkuZGVudGlzdEAgZ21haWwuY29t)).
Conflict of interest
The authors declare that the research was conducted in the absence of any commercial or financial relationships that could be construed as a potential conflict of interest.
Publisher’s note
All claims expressed in this article are solely those of the authors and do not necessarily represent those of their affiliated organizations, or those of the publisher, the editors and the reviewers. Any product that may be evaluated in this article, or claim that may be made by its manufacturer, is not guaranteed or endorsed by the publisher.
Supplementary material
The Supplementary material for this article can be found online at: https://www.frontiersin.org/articles/10.3389/fnagi.2022.1032401/full#supplementary-material
Footnotes
1. ^http://www.ncbi.nlm.nih.gov/
2. ^https://www.gsea-msigdb.org/gsea/msigdb/human/geneset/HALLMARK_INFLAMMATORY_RESPONSE.html
3. ^https://www.gsea-msigdb.org/gsea/msigdb/
References
Al-Bachari, S., Naish, J. H., Parker, G. J. M., Emsley, H. C. A., and Parkes, L. M. (2020). Blood–brain barrier leakage is increased in Parkinson’s disease. Front. Physiol. 11:593026. doi: 10.3389/fphys.2020.593026
Ali, M. Z., and Dholaniya, P. S. (2022). Oxidative phosphorylation mediated pathogenesis of Parkinson's disease and its implication via Akt signaling. Neurochem. Int. 157:105344. doi: 10.1016/j.neuint.2022.105344
Al-Omari, F. A., Al Moaleem, A. M., Al-Qahtani, S. S., Al Garni, A. S., Sadatullah, S., Luqman, M., et al. (2014). Oral rehabilitation of Parkinson’s disease patient: a review and case report. Case Rep. Dent. 2014, 1–4. doi: 10.1155/2014/432475
Alves, S. R., Silva, C., Rosa, I. M., Henriques, A. G., and Silva, O. A. B. (2022). A bioinformatics approach toward unravelling the synaptic molecular crosstalk between Alzheimer’s disease and diabetes. J. Alzheimers Dis. 86, 1917–1933. doi: 10.3233/JAD-215059
Auffret, M., Meuric, V., Boyer, E., Bonnaure-Mallet, M., and Vérin, M. (2021). Oral health disorders in Parkinson’s disease: more than meets the eye. J. Parkinsons Dis. 11, 1507–1535. doi: 10.3233/JPD-212605
Beranger, G. E., Momier, D., Guigonis, J. M., Samson, M., Carle, G. F., and Scimeca, J. C. (2007). Differential binding of poly (ADP-ribose) polymerase-1 and JunD/Fra2 accounts for RANKL-induced Tcirg1 gene expression during osteoclastogenesis. J. Bone Miner. Res. 22, 975–983. doi: 10.1359/jbmr.070406
Blaszczyk, J. W. (2016). Parkinson's disease and Neurodegeneration: GABA-collapse hypothesis. Front. Neurosci. 10:269. doi: 10.3389/fnins.2016.00269
Blaylock, R. L. (2017). Parkinson's disease: microglial/macrophage-induced immunoexcitotoxicity as a central mechanism of neurodegeneration. Surg. Neurol. Int. 8:65. doi: 10.4103/sni.sni_441_16
Bohatschek, M., Werner, A., and Raivich, G. (2001). Systemic LPS injection leads to granulocyte influx into normal and injured brain: effects of ICAM-1 deficiency. Exp. Neurol. 172, 137–152. doi: 10.1006/exnr.2001.7764
Botelho, J., Mascarenhas, P., Mendes, J. J., and Machado, V. (2020). Network protein interaction in Parkinson's disease and periodontitis interplay: a preliminary Bioinformatic analysis. Genes (Basel) 11:1385. doi: 10.3390/genes11111385
Cardoso, E. M., and Arosa, F. A. (2017). CD8+ T cells in chronic periodontitis: roles and rules. Front. Immunol. 8:145. doi: 10.3389/fimmu.2017.00145
Carrion, J., Scisci, E., Miles, B., Sabino, G. J., Zeituni, A. E., Gu, Y., et al. (2012). Microbial carriage state of peripheral blood dendritic cells (DCs) in chronic periodontitis influences DC differentiation, atherogenic potential. J. Immunol. 189, 3178–3187. doi: 10.4049/jimmunol.1201053
Chao, Y., Wong, S. C., and Tan, E. K. (2014). Evidence of inflammatory system involvement in Parkinson’s disease. Biomed. Res. Int. 2014, 1–9. doi: 10.1155/2014/308654
Chen, H., Peng, L., Wang, Z., He, Y., Tang, S., and Zhang, X. (2022). Exploration of cross-talk and pyroptosis-related gene signatures and molecular mechanisms between periodontitis and diabetes mellitus via peripheral blood mononuclear cell microarray data analysis. Cytokine 159:156014. doi: 10.1016/j.cyto.2022.156014
Chen, C. K., Wu, Y. T., and Chang, Y. C. (2017). Periodontal inflammatory disease is associated with the risk of Parkinson's disease: a population-based retrospective matched-cohort study. PeerJ 5:e3647. doi: 10.7717/peerj.3647
Chen, Y., Yu, X., and Kong, J. (2021). Identification of neuropeptides as potential cross talks linking down syndrome and periodontitis revealed by transcriptomic analyses. Dis. Markers 2021, 1–18. doi: 10.1155/2021/7331821
Cole, L. A. (2016). “Chapter 10 - Adenosine triphosphate energetics” in Biology of Life. ed. L. A. Cole (Elsevier Academic Press), 65–77.
Edgar, R., Domrachev, M., and Lash, A. E. (2002). Gene expression omnibus: NCBI gene expression and hybridization array data repository. Nucleic Acids Res. 30, 207–210. doi: 10.1093/nar/30.1.207
Fleetwood, A. J., Achuthan, A., Schultz, H., Nansen, A., Almholt, K., Usher, P., et al. (2014). Urokinase plasminogen activator is a central regulator of macrophage three-dimensional invasion, matrix degradation, and adhesion. J. Immunol. 192, 3540–3547. doi: 10.4049/jimmunol.1302864
Fleetwood, A. J., O'Brien-Simpson, N. M., Veith, P. D., Lam, R. S., Achuthan, A., Cook, A. D., et al. (2015). Porphyromonas gingivalis-derived RgpA-Kgp complex activates the macrophage urokinase plasminogen activator system: implications for periodontitis. J. Biol. Chem. 290, 16031–16042. doi: 10.1074/jbc.M115.645572
Funatsu, J., Murakami, Y., Shimokawa,, Nakatake, S., Fujiwara, K., Okita, A., et al. (2022). Circulating inflammatory monocytes oppose microglia and contribute to cone cell death in retinitis pigmentosa. PNAS Nexus 1:pgac003. doi: 10.1093/pnasnexus/pgac003
Galiano-Landeira, J., Torra, A., Vila, M., and Bové, J. (2020). CD8 T cell nigral infiltration precedes synucleinopathy in early stages of Parkinson’s disease. Brain 143, 3717–3733. doi: 10.1093/brain/awaa269
Hajishengallis, G., Kajikawa, T., Hajishengallis, E., Maekawa, T., Reis, E. S., Mastellos, D. C., et al. (2019). Complement-dependent mechanisms and interventions in periodontal disease. Front. Immunol. 10:406. doi: 10.3389/fimmu.2019.00406
Hanaoka, A., and Kashihara, K. (2009). Increased frequencies of caries, periodontal disease and tooth loss in patients with Parkinson's disease. J. Clin. Neurosci. 16, 1279–1282. doi: 10.1016/j.jocn.2008.12.027
Hänzelmann, S., Castelo, R., and Guinney, J. (2013). GSVA: gene set variation analysis for microarray and RNA-seq data. BMC Bioinformatics 14, 1–15. doi: 10.1186/1471-2105-14-7
He, Z., Jiang, Q., Li, F., and Chen, M. (2021). Crosstalk between venous thromboembolism and periodontal diseases: a bioinformatics analysis. Dis. Markers 2021, 1–16. doi: 10.1155/2021/1776567
Heinemann, T., Bulwin, G. C., Randall, J., Schnieders, B., Sandhoff, K., Volk, H. D., et al. (1999). Genomic organization of the gene coding for TIRC7, a novel membrane protein essential for T cell activation. Genomics 57, 398–406. doi: 10.1006/geno.1999.5751
Heo, S. C., You, J. C., Jung, S., Kim, Y. N., Shin, S. H., Lee, J. Y., et al. (2022). Pim-2 regulates bone resorptive activity of osteoclasts via V-ATPase a3 isoform expression in periodontal disease. J. Cell. Physiol. 237, 3381–3393. doi: 10.1002/jcp.30814
Kanehisa, M., and Goto, S. (2000). KEGG: Kyoto encyclopedia of genes and genomes. Nucleic Acids Res. 28, 27–30. doi: 10.1093/nar/28.1.27
Karimi-Moghadam, A., Charsouei, S., Bell, B., and Jabalameli, M. R. (2018). Parkinson disease from Mendelian forms to genetic susceptibility: new molecular insights into the Neurodegeneration process. Cell. Mol. Neurobiol. 38, 1153–1178. doi: 10.1007/s10571-018-0587-4
Kassambara, A., and Mundt, F. (2017). Package ‘factoextra’. Extract and visualize the results of multivariate data analyses. 1–74. Available at: http://www.sthda.com/english/rpkgs/factoextra
Kauppinen, A., Kaarniranta, K., and Salminen, A. (2020). Potential role of myeloid-derived suppressor cells (MDSCs) in age-related macular degeneration (AMD). Front. Immunol. 11:384. doi: 10.3389/fimmu.2020.00384
Kaur, G., Grover, V., Bhaskar, N., Kaur, R. K., and Jain, A. (2018). Periodontal infectogenomics. Inflamm. Regen. 38:8. doi: 10.1186/s41232-018-0065-x
Kaur, T., Uppoor, A., and Naik, D. (2016). Parkinson’s disease and periodontitis - the missing link? A review. Gerodontology 33, 434–438. doi: 10.1111/ger.12188
Kebschull, M., Demmer, R. T., Grün, B., Guarnieri, P., Pavlidis, P., and Papapanou, P. N. (2014). Gingival tissue transcriptomes identify distinct periodontitis phenotypes. J. Dent. Res. 93, 459–468. doi: 10.1177/0022034514527288
Leek, J. T., Johnson, W. E., Parker, H. S., Fertig, E. J., Jaffe, A. E., Storey, J. D., et al. (2019). Sva: surrogate variable analysis. R Package Version 3, 882–883.
Li, S., Liu, X., Zhou, Y., Acharya, A., Savkovic, V., Xu, C., et al. (2018). Shared genetic and epigenetic mechanisms between chronic periodontitis and oral squamous cell carcinoma. Oral Oncol. 86, 216–224. doi: 10.1016/j.oraloncology.2018.09.029
Liu, S., Fu, Y., Ziebolz, D., Li, S., Schmalz, G., and Li, F. (2022a). Transcriptomic analysis reveals pathophysiological relationship between chronic obstructive pulmonary disease (COPD) and periodontitis. BMC Med. Genet. 15, 1–16. doi: 10.1186/s12920-022-01278-w
Liu, J., Zhang, D., Cao, Y., Zhang, H., Li, J., Xu, J., et al. (2022b). Screening of crosstalk and pyroptosis-related genes linking periodontitis and osteoporosis based on bioinformatics and machine learning. Front. Immunol. 13:955441. doi: 10.3389/fimmu.2022.955441
Liu, J., Zhang, X., Cheng, Y., and Cao, X. (2021). Dendritic cell migration in inflammation and immunity. Cell. Mol. Immunol. 18, 2461–2471. doi: 10.1038/s41423-021-00726-4
Lopez, R., Regier, J., Cole, M. B., Jordan, M. I., and Yosef, N. (2018). Deep generative modeling for single-cell transcriptomics. Nat. Methods 15, 1053–1058. doi: 10.1038/s41592-018-0229-2
Ma, S.-X., Seo, B. A., Kim, D., Xiong, Y., Kwon, S. H., Brahmachari, S., et al. (2021). Complement and coagulation cascades are potentially involved in dopaminergic neurodegeneration in α-synuclein-based mouse models of Parkinson’s disease. J. Proteome Res. 20, 3428–3443. doi: 10.1021/acs.jproteome.0c01002
Magnusen, D., Nyamajenjere, T., Rapien, J., Mckay, M., Magnusen, A., and Pandey, M. (2018). Dendritic cells, CD4+ T cells, and α synuclein triangle fuels neuroinflammation in Parkinson’s disease (P3. 053). Neurology 90.
Merle, N. S., Noe, R., Halbwachs-Mecarelli, L., Fremeaux-Bacchi, V., and Roumenina, L. T. (2015). Complement system part II: role in immunity. Front. Immunol. 6:257. doi: 10.3389/fimmu.2015.00257
Mersich, A. T., Miller, M. R., Chkourko, H., and Blystone, S. D. (2010). The formin FRL1 (FMNL1) is an essential component of macrophage podosomes. Cytoskeleton 67, 573–585. doi: 10.1002/cm.20468
Miller, M. R., Miller, E. W., and Blystone, S. D. (2017). Non-canonical activity of the podosomal formin FMNL1γ supports immune cell migration. J. Cell Sci. 130, 1730–1739. doi: 10.1242/jcs.195099
Mutez, E., Nkiliza, A., Belarbi, K., de Broucker, A., Vanbesien-Mailliot, C., Bleuse, S., et al. (2014). Involvement of the immune system, endocytosis and EIF2 signaling in both genetically determined and sporadic forms of Parkinson's disease. Neurobiol. Dis. 63, 165–170. doi: 10.1016/j.nbd.2013.11.007
Olsen, I., Kell, D. B., and Pretorius, E. (2020). Is Porphyromonas gingivalis involved in Parkinson’s disease? Eur. J. Clin. Microbiol. Infect. Dis. 39, 2013–2018. doi: 10.1007/s10096-020-03944-2
Oughtred, R., Rust, J., Chang, C., Breitkreutz, B. J., Stark, C., Willems, A., et al. (2021). The BioGRID database: a comprehensive biomedical resource of curated protein, genetic, and chemical interactions. Protein Sci. 30, 187–200. doi: 10.1002/pro.3978
Pan, S., Hu, B., Sun, J., Yang, Z., Yu, W., He, Z., et al. (2022). Identification of cross-talk pathways and ferroptosis-related genes in periodontitis and type 2 diabetes mellitus by bioinformatics analysis and experimental validation. Front. Immunol. 13:1015491. doi: 10.3389/fimmu.2022.1015491
Papapanou, P. N., Behle, J. H., Kebschull, M., Celenti, R., Wolf, D. L., Handfield, M., et al. (2009). Subgingival bacterial colonization profiles correlate with gingival tissue gene expression. BMC Microbiol. 9:221. doi: 10.1186/1471-2180-9-221
Peri, S., Navarro, J. D., Kristiansen, T. Z., Amanchy, R., Surendranath, V., Muthusamy, B., et al. (2004). Human protein reference database as a discovery resource for proteomics. Nucleic Acids Res. 32, 497D–4501D. doi: 10.1093/nar/gkh070
Pussinen, P. J., Vilkuna-Rautiainen, T., Alfthan, G., Palosuo, T., Jauhiainen, M., Sundvall, J., et al. (2004). Severe periodontitis enhances macrophage activation via increased serum lipopolysaccharide. Arterioscler. Thromb. Vasc. Biol. 24, 2174–2180. doi: 10.1161/01.ATV.0000145979.82184.9f
Reuland, C. J., and Church, F. C. (2020). Synergy between plasminogen activator inhibitor-1, α-synuclein, and neuroinflammation in Parkinson’s disease. Med. Hypotheses 138:109602. doi: 10.1016/j.mehy.2020.109602
Ricklin, D., Hajishengallis, G., Yang, K., and Lambris, J. D. (2010). Complement: a key system for immune surveillance and homeostasis. Nat. Immunol. 11, 785–797. doi: 10.1038/ni.1923
Ritchie, M. E., Phipson, B., Wu, D., Hu, Y., Law, C. W., Shi, W., et al. (2015). Limma powers differential expression analyses for RNA-sequencing and microarray studies. Nucleic Acids Res. 43:e47. doi: 10.1093/nar/gkv007
Scannapieco, F. A. (2004). Periodontal inflammation: from gingivitis to systemic disease? Compend. Contin. Educ. Dent. 25, 16–25.
Scherzer, C. R., Eklund, A. C., Morse, L. J., Liao, Z., Locascio, J. J., Fefer, D., et al. (2007). Molecular markers of early Parkinson's disease based on gene expression in blood. Proc. Natl. Acad. Sci. 104, 955–960. doi: 10.1073/pnas.0610204104
Scherzer, C. R., Grass, J. A., Liao, Z., Pepivani, I., Zheng, B., Eklund, A. C., et al. (2008). GATA transcription factors directly regulate the Parkinson's disease-linked gene alpha-synuclein. Proc. Natl. Acad. Sci. U. S. A. 105, 10907–10912. doi: 10.1073/pnas.0802437105
Schwarz, J., Heimhilger, E., and Storch, A. (2006). Increased periodontal pathology in Parkinson's disease. J. Neurol. 253, 608–611. doi: 10.1007/s00415-006-0068-4
Shamir, R., Klein, C., Amar, D., Vollstedt, E. J., Bonin, M., Usenovic, M., et al. (2017). Analysis of blood-based gene expression in idiopathic Parkinson disease. Neurology 89, 1676–1683. doi: 10.1212/WNL.0000000000004516
Shannon, P., Markiel, A., Ozier, O., Baliga, N. S., Wang, J. T., Ramage, D., et al. (2003). Cytoscape: a software environment for integrated models of biomolecular interaction networks. Genome Res. 13, 2498–2504. doi: 10.1101/gr.1239303
Su, L., Xu, Q., Zhang, P., Michalek, S. M., and Katz, J. (2017). Phenotype and function of myeloid-derived suppressor cells induced by Porphyromonas gingivalis infection. Infect. Immun. 85:e00213-17. doi: 10.1128/IAI.00213-17
Su, R., and Zhou, T. (2021). Alpha-synuclein induced immune cells activation and associated therapy in Parkinson’s disease. Frontiers in aging. Neuroscience 13. doi: 10.3389/fnagi.2021.769506
Tettamanti, L., Gaudio, R. M., Iapichino, A., Mucchi, D., and Tagliabue, A. (2017). Genetic susceptibility and periodontal disease: a retrospective study on a large Italian sample. Oral Implantol. (Rome) 10, 20–27. doi: 10.11138/orl/2017.10.1.020
Tonetti, M. S., Greenwell, H., and Kornman, K. S. (2018). Staging and grading of periodontitis: framework and proposal of a new classification and case definition. J. Periodontol. 89, S159–S172. doi: 10.1002/JPER.18-0006
Valero-Monroy, O., Garcia-Cervantes, G., Marquez-Corrales, L. F., Leija-Montoya, A. G., Sandoval-Basilio, J., Martinez-Coronilla, G., et al. (2016). Myeloid derived suppressor cell: a new player in periodontal disease? Med. Hypotheses 95, 35–38. doi: 10.1016/j.mehy.2016.08.010
Walker, D. G., Lue, L. F., and Beach, T. G. (2002). Increased expression of the urokinase plasminogen-activator receptor in amyloid beta peptide-treated human brain microglia and in AD brains. Brain Res. 926, 69–79. doi: 10.1016/S0006-8993(01)03298-X
Wilkerson, M. D., and Hayes, D. N. (2010). ConsensusClusterPlus: a class discovery tool with confidence assessments and item tracking. Bioinformatics 26, 1572–1573. doi: 10.1093/bioinformatics/btq170
Wu, T., Hu, E., Xu, S., Chen, M., Guo, P., Dai, Z., et al. (2021). clusterProfiler 4.0: a universal enrichment tool for interpreting omics data. The. Innovations 2:100141. doi: 10.1016/j.xinn.2021.100141
Xu, T., Dong, Q., Luo, Y., Liu, Y., Gao, L., Pan, Y., et al. (2021). Porphyromonas gingivalis infection promotes mitochondrial dysfunction through Drp1-dependent mitochondrial fission in endothelial cells. Int. J. Oral Sci. 13, 1–11. doi: 10.1038/s41368-021-00134-4
Yan, B., Ren, F., Shang, W., and Gong, X. (2022). Transcriptomic analysis reveals genetic cross-talk between periodontitis and hypothyroidism. Dis. Markers 2022, 1–12. doi: 10.1155/2022/5736394
Yang, Y., Zhang, X. L., Guan, Q. B., Guo, L., Zhang, H., Han, C. Y., et al. (2018). Detection and clinical significance of myeloid-derived suppressor cells in peripheral blood of patients with Parkinson disease. Chin. J. Pathophysiol. 12, 107–111.
Yu, G., Wang, L. G., Han, Y., and He, Q. Y. (2012). clusterProfiler: an R package for comparing biological themes among gene clusters. Omics J. Integr. Biol. 16, 284–287. doi: 10.1089/omi.2011.0118
Zhang, X., Shao, Z., Xu, S., Liu, Q., Liu, C., Luo, Y., et al. (2021). Immune profiling of Parkinson's disease revealed its association with a subset of infiltrating cells and signature genes. Front. Aging Neurosci. 13:605970. doi: 10.3389/fnagi.2021.605970
Keywords: neurodegenerative disease, Parkinson’s disease, periodontitis, crosstalk genes, bioinformatics
Citation: Hu S, Li S, Ning W, Huang X, Liu X, Deng Y, Franceschi D, Ogbuehi AC, Lethaus B, Savkovic V, Li H, Gaus S, Zimmerer R, Ziebolz D, Schmalz G and Huang S (2022) Identifying crosstalk genetic biomarkers linking a neurodegenerative disease, Parkinson’s disease, and periodontitis using integrated bioinformatics analyses. Front. Aging Neurosci. 14:1032401. doi: 10.3389/fnagi.2022.1032401
Edited by:
Jun Xu, Capital Medical University, ChinaReviewed by:
Sakhawat Ali, Beijing Institute of Technology, ChinaMuqaddas Masood, Shanghai Institutes for Biological Sciences (CAS), China
Copyright © 2022 Hu, Li, Ning, Huang, Liu, Deng, Franceschi, Ogbuehi, Lethaus, Savkovic, Li, Gaus, Zimmerer, Ziebolz, Schmalz and Huang. This is an open-access article distributed under the terms of the Creative Commons Attribution License (CC BY). The use, distribution or reproduction in other forums is permitted, provided the original author(s) and the copyright owner(s) are credited and that the original publication in this journal is cited, in accordance with accepted academic practice. No use, distribution or reproduction is permitted which does not comply with these terms.
*Correspondence: Shaonan Hu, c2hhb25hbl9odUBzbXUuZWR1LmNu; Shaohong Huang, aHNoLmNoaW5hQHRvbS5jb20=
†These authors have contributed equally to this work and share first authorship
‡Senior author