- 1Department of Stomatology, China–Japan Union Hospital of Jilin University, Changchun, China
- 2Key Laboratory of Lymphatic Surgery Jilin Province, Jilin Engineering Laboratory for Lymphatic Surgery, China-Japan Union Hospital of Jilin University, Changchun, China
- 3Oral and Maxillofacial Surgery, Changchun Stomatological Hospital, Changchun, China
- 4Department of Gynecology and Obstetrics, China-Japan Union Hospital of Jilin University, Changchun, China
- 5Department of Pathophysiology, College of Basic Medical Sciences, Jilin University, Changchun, China
- 6Scientific Research Center, China-Japan Union Hospital of Jilin University, Changchun, China
Periodontitis is a chronic inflammatory disease inextricably linked to both the innate and acquired immune systems of the body. Parkinson’s disease (PD) is a neurodegenerative disease caused by immune system dysfunction. Although recent studies suggest that a clinical relationship exists between PD and periodontitis, the pathogenesis of this relationship is unclear. Therefore, in the present study, we obtained datasets of periodontitis and PD from the Gene Expression Omnibus (GEO) database and extracted 785 differentially expressed genes (DEGs), including 15 common upregulated genes and four common downregulated genes. We performed enrichment analyses of these DEGs using Gene Ontology (GO) and Kyoto Encyclopedia of Genes and Genomes analyses. We found that the genes were mainly enriched in keratinocyte differentiation, neuronal cell bodies, and structural constituents of epidermis terms, and pathways such as immune response and synaptic pathways. In addition, we screened matching hub genes by constructing a protein–protein interaction (PPI) network map and a Molecular Complex Detection (MCODE) map using the Cytoscape software. The hub genes were then subjected to GO enrichment analysis, which revealed that the dopamine biosynthetic process, dopaminergic synapse and dopamine-binding terms, and dopaminergic synapse and serotonergic synapse pathways were primarily where they were expressed. Finally, we selected four of these genes for validation in the periodontitis and PD datasets, and we confirmed that these hub genes were highly sensitive and specific for diagnosing and monitoring PD and periodontitis. In conclusion, the above experimental results indicate that periodontitis is a high-risk factor for PD, and the association between these two conditions is mainly manifested in immune and dopamine-related pathways. Hub genes, such as the CDSN, TH, DDC, and SLC6A3 genes, may serve as potential biomarkers for diagnosing or detecting PD.
Introduction
Periodontitis is an infectious, multifactorial condition that damages supportive tissues of the teeth (Corrêa et al., 2017). As one of the most prevalent inflammatory diseases in the world, periodontitis is not only associated with the enhancement of multiple inflammatory conditions, such as cardiovascular disease and rheumatoid arthritis (Dutzan et al., 2017), but also leads to a variety of chronic diseases, such as hypertension (Czesnikiewicz-Guzik et al., 2019) and diabetes (Xiao et al., 2017). Porphyromonas gingivalis (P. gingivalis) is the main oral pathogen responsible for the onset and progression of periodontitis (Xu et al., 2016; Ye et al., 2017). Many reports have suggested that P. gingivalis infection is strongly associated with Alzheimer’s disease (Liang et al., 2020).
Neurodegenerative diseases are characterized by progressive loss of neurons and neuronal death, such as Alzheimer’s disease (AD), Parkinson’s disease (PD), and Huntington’s disease (HD) (Hashimoto et al., 2019). Currently, an increasing number of studies have been suggesting the existence of an association between neurodegenerative disease and periodontitis. For example, lipopolysaccharides (LPS) from P. gingivalis upregulate the expression of amyloid precursor protein (APP) in the brain, promote the deposition of Aβ in neurons, and promote the development and progression of AD (Zeng et al., 2021). Moreover, a recent study using bioinformatics analysis identified a series of potential key genes, along with enriched signaling cascades, serving as promising molecular markers for periodontitis and AD (Jiang et al., 2021).
Similarly, PD is the second most common neurodegenerative disorder after AD (Kern et al., 2021). Some studies have suggested that periodontitis may lead to the occurrence and development of PD. For instance, periodontitis has been associated with changes in leukocyte counts in patients with PD (Botelho et al., 2020a). Moreover, a recent study revealed that major virulence factors of P. gingivalis, such as gingipain R1 (RgpA) and lipopolysaccharide, circulate in the blood of PD patients (Olsen et al., 2020). Nonetheless, researchers constructed a network of potential protein–protein interactions (PPI) between PD and periodontitis using preliminary bioinformatics analyses, in an attempt to identify new potential targets (Botelho et al., 2020b). The potential biomarkers of periodontitis-related PD and their underlying molecular mechanisms still remain poorly understood.
Recently, high-throughput sequencing has been widely used for prognostic evaluation, molecular diagnosis, and target discovery (Field, 2021). The Gene Expression Omnibus (GEO) database has huge disease gene expression patterns and a wide range of key genes that can be utilized to explore disease initiation and progression (Patra et al., 2020). The GEO database can be also used to explore the correlation between pathogenesis and molecular mechanisms, which have important clinical significance in disease research (Alameer and Chicco, 2021). Therefore, we attempted to use the GEO expression profile to identify novel biomarkers for diagnosis and therapy.
In the present study, we obtained the expression patterns of human PD and periodontitis genes from the GEO database. Using the R package (V. 3.6.3), differentially expressed genes (DEGs) were screened and the common genes were intersected. The DEGs were then used for gene ontology (GO) enrichment and Kyoto Encyclopedia of Genes and Genomes (KEGG) pathway analyses. The Search Tool for the Retrieval of Interacting Genes (STRING) was used to identify the PPI network between periodontitis and PD. Using the Cytoscape software, highly related functional modules and candidate hub genes were screened. Finally, the diagnostic values of the hub genes were determined using different independent periodontitis and PD cohorts. Summarily, in the present study, we provide promising biomarkers that might be associated with PD, and we explore the molecular mechanisms underlying PD. Our findings provide evidence for the correlation between periodontitis and PD, and provide information that could be helpful in the diagnosis and treatment of patients with PD. The article research process is shown in Figure 1.
Materials and methods
Data abstraction
We downloaded the gene expression array data, GSE23586 and GSE7621, from the Gene Expression Omnibus (GEO).1 TheGSE23586 dataset contains data obtained from the gingival tissues from three healthy subjects and three patients affected by periodontitis. Contrarily, the GSE7621 dataset contains data obtained from nine substantia nigra tissues from postmortem brain specimens of normal patients and 16 substantia nigra tissues from postmortem brain specimens of patients with PD.
Identification of differentially expressed genes
To identify DEGs in the GSE23586 and GSE7621 datasets, the original files downloaded from the GEO database were processed and normalized using the R package (version 3.6.3; Edgar et al., 2002; Barrett et al., 2013). A|logFC| > 2 (Fold change) and p < 0.05 were considered the threshold for the DEGs of GSE23586. However, a|logFC| > 1 and p < 0.05 were considered the threshold for the DEGs of GSE7621. Genes with p < 0.05 and log FC > 1 were considered upregulated (UP), while genes with p < 0.05 and log FC < −1 were considered downregulated (DOWN). After utilizing these screening conditions, two sets of DEGs were identified, which were then put into the online Venn diagram analysis tool2 to obtain up- and downregulated intersection genes. To better visualize these DEGs, heat maps and volcano plots were made produced using the R package. These intersecting (common) genes were used in the subsequent analyses.
Functional enrichment analysis of DEGs
Gene Ontology (GO) terms (for biological processes, cellular components, and molecular functions categories), and KEGG functional enrichment were performed on the intersecting DEGs using the R package.
Protein–protein interaction network construction and module analysis
To construct a PPI association between periodontitis and PD, we used the Search Tool for the Retrieval of Interacting Genes (STRING) 11.0 (Szklarczyk et al., 2015).3 An interaction with a combined score > 0.7 was selected, and the PPI network was constructed using the Cytoscape software. Cytoscape (version 3.9.1) is an open-source bioinformatics software platform for visualizing networks of molecular interactions (Szklarczyk et al., 2019). Analysis of densely connected regions was performed using the Cytoscape plug-in molecular complex detection (MCODE), and the top 15 genes with the greatest relevance were screened. GO and KEGG analyses were performed using the R package.
Statistical analyses
As previously stated, statistical analyses of all data were performed using the R software (version 3.6.3). Statistical significance was set at p < 0.05. Using the Statistical Packages for the Social Sciences software (SPSS 22.0; SPSS, Inc., Chicago, IL, United States), we constructed receiver operating characteristic (ROC) curves and calculated the areas under the curve (AUC) of the hub gene. We compared AUC as an indicator of the models. These results demonstrated the diagnostic efficiency of these genes. Statistical significance was set at p < 0.05.
Results
DEGs identification
First, 785 DEGs were identified from healthy samples and periodontitis samples in the GSE23586 dataset, including 538 UP genes and 247 DOWN genes. Heat maps and volcano plots were used to visualize the DEGs (Figures 2A,C) using the|logFC| > 2 criterion with p < 0.05. However, 552 DEGs between normal and PD samples, including 330 UP genes and 222 DOWN genes, were from the GSE7621 dataset using|logFC| > 1 criterion with p < 0.05 (Figures 2B,D).
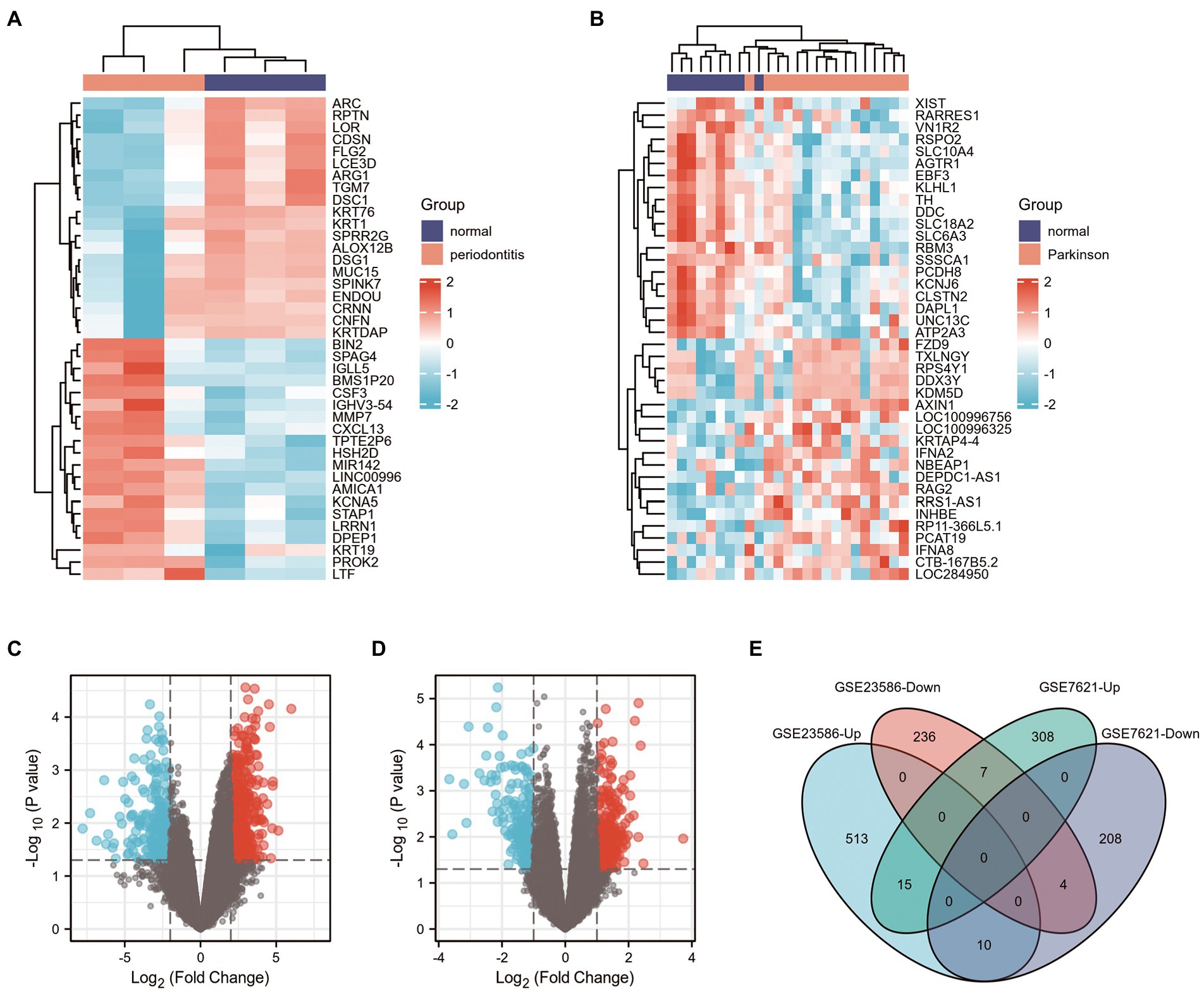
Figure 2. Screening of DEGs in GSE23586 and GSE7621 datasets. (A) Heatmap of DEGs between the normal sample and periodontitis sample in GSE23586 dataset. (B) Heatmap of DEGs between the normal sample and Parkinson’s disease sample in GSE7621 dataset. Red rectangles represent high expression, and blue rectangles represent low expression. (C) Volcano plot of DEGs between the normal sample and periodontitis sample in GSE23586 dataset. (D) Volcano plot of DEGs between the normal sample and Parkinson’s disease sample in GSE7621 dataset. Red plots represent upregulated genes, the black plots represent nonsignificant genes, and the blue plots represent downregulated genes. (E) Venn diagram of common DEGs from GSE23586 (|logFC| >2, and p < 0.05) and GSE7621 (|logFC| >1, and p < 0.05) dataset.
Venn diagrams of the DEGs in the GSE23586 and GSE7621 datasets are presented in Figure 2E. As seen, there were 15 common UP genes and four common DOWN genes. Details of these common genes are presented in Table 1.
Functional and pathway enrichment of DEGs
In the present study, 39 GO terms were identified, including 23 biological process (BP) terms, 4 cellular component (CC) terms, and 12 molecular function (MF) terms (adjusted p-value, P. adj. <0.1 & q-value <0.2). The top enriched functional category terms were are shown in Figure 3A and are presented in Table 2. The enriched GO terms included cornification, keratinocyte differentiation, skin development, and epidermis development in the BP category; cornified envelope, desmosome, neuronal cell body, and parallel fiber to Purkinje cell synapse in the CC category; and structural constituent of the epidermis, endopeptidase inhibitor activity, peptidase inhibitor activity, and endopeptidase regulator activity in the MF category.
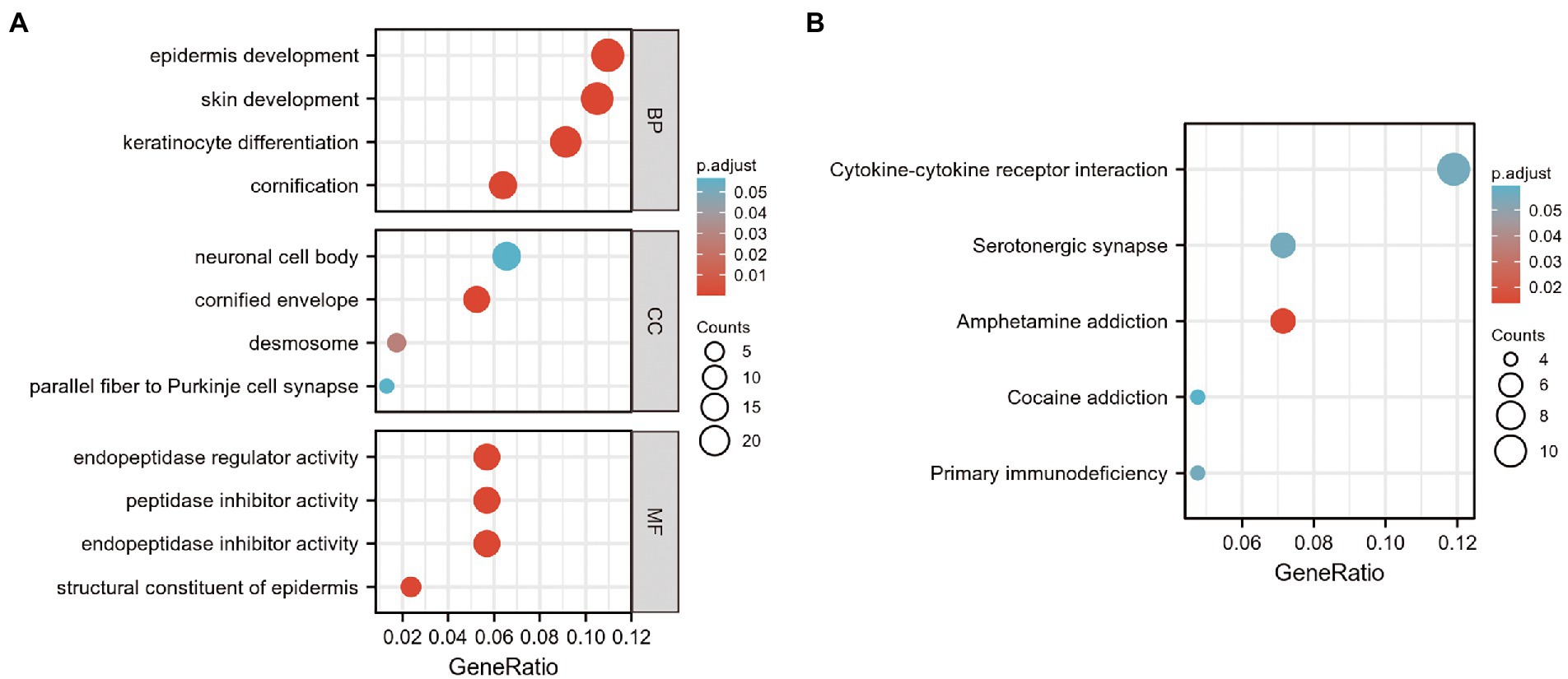
Figure 3. GO and KEGG analysis of DEGs in GSE23586 and GSE7621 datasets. (A) GO function enrichment analysis of DEGs in GSE23586 and GSE7621 dataset. GO, Gene Ontology; MF, Molecular Function; BP, Biological Process; CC, Cellular Components; DEGs, differentially expressed genes. (B) Enrichment analysis of the KEGG signaling pathway of DEGs in GSE23586 and GSE7621 dataset.
Furthermore, seven enriched KEGG terms with P. adj. < 0.1 & q-value <0.2 were identified. The most enriched functional pathway terms are shown in Figure 3B and presented in Table 3. They included amphetamine addiction, primary immuno-deficiency, cytokine-cytokine receptor interaction, serotonergic synapse, and cocaine addiction.
PPI network analysis of DEGs and hub gene selection
To investigate the association of the DEGs, PPI networks with an interaction score > 0.7 were analyzed in the STRING online database (Figure 4). We used the nodes to represent genes and the edges to represent connections between genes. Cytoscape was used to present the key PPI network modules. As shown in Figures 5A–D, four key modules were identified: late cornified envelope 2B (LCE2B), late cornified envelope 3D (LCE3D), corneodesmosin (CDSN), small proline-rich protein 2G (SPRR2G), loricrin cornified envelope precursor protein (LOR), tyrosine hydroxylase (TH), Dopa decarboxylase (DDC), solute carrier family 6 member 3 (SLC6A3), solute carrier family 18 member A2 (SLC18A2), arachidonate 12-lipoxygenase 12R type (ALOX12B), ATP-binding cassette subfamily A member 12 (ABCA12), cytochrome P450 family 4 subfamily F member 22 (CYP4F22), immunoglobulin lambda-like polypeptide 5 (IGLL5), CD19 molecule (CD19), and CD79a molecule (CD79A), which interacted with other proteins. These were the central nodes in the protein interaction network.
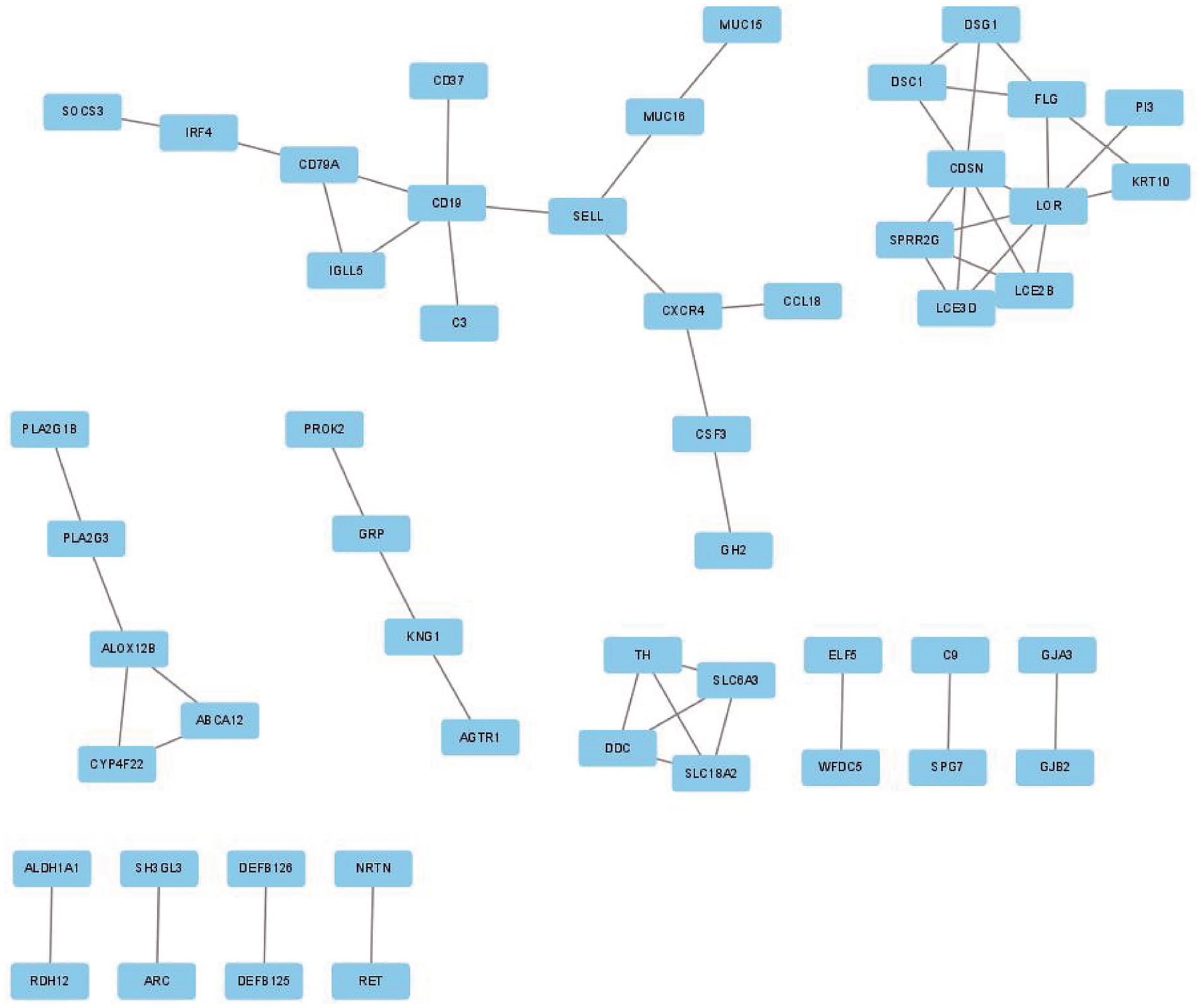
Figure 4. PPI network of DEGs in GSE23586 and GSE7621 dataset. PPI, protein–protein interaction; DEGs, differentially expressed genes.
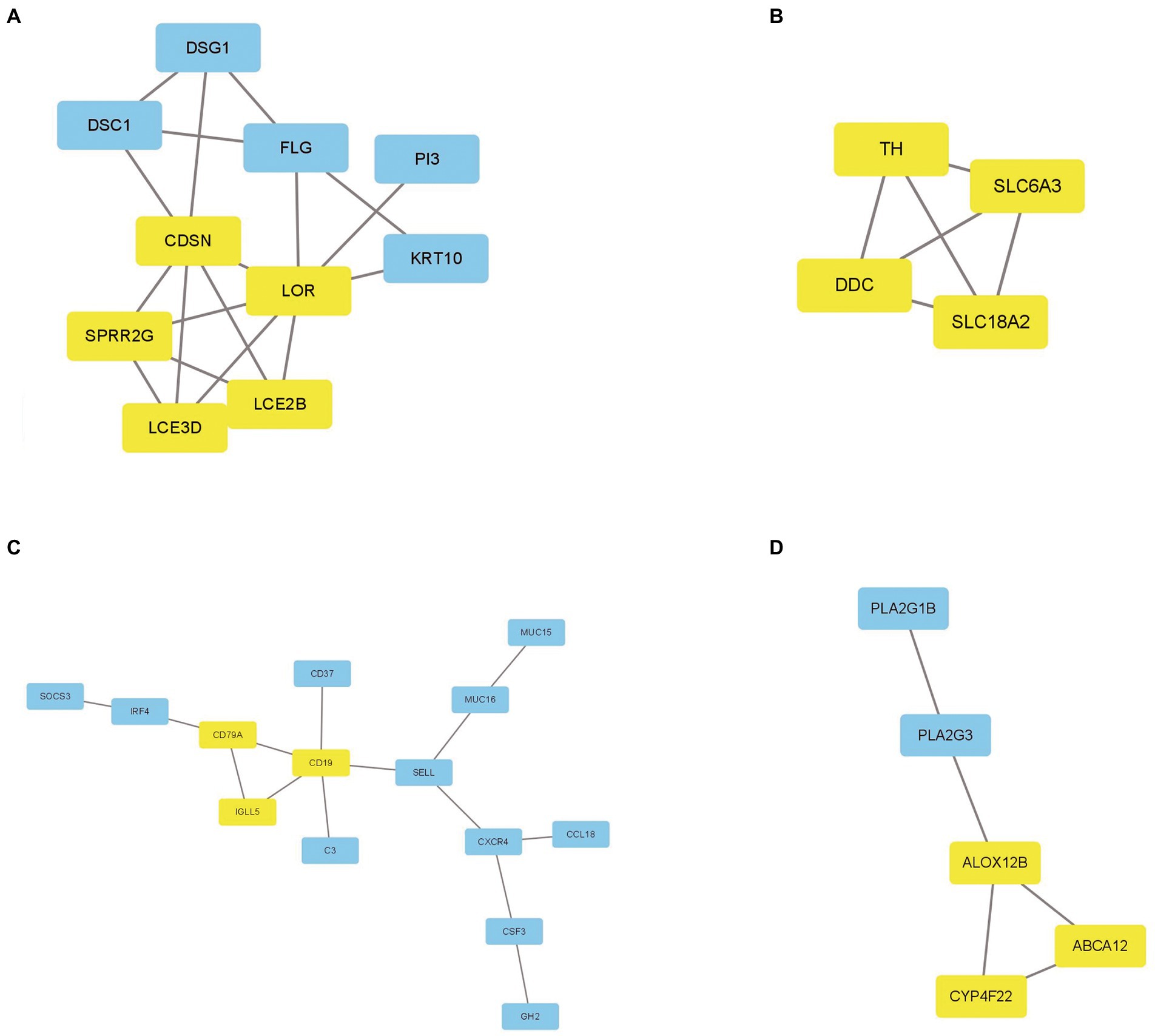
Figure 5. The key PPI network modules in GSE23586 and GSE7621 dataset. (A–D) Four key PPI network modules were performed by Cytoscape.
Functional and pathway enrichment of hub genes
The scores of these hub genes are shown in Figure 6A; Table 4. Functional enrichment analysis showed that the 15 hub genes were mainly linked to the dopamine biosynthetic process, skin development, and keratinization. There were three BP terms, as well as three CC terms (namely dopaminergic synapse, synaptic vesicle, and presynapse), and three MF terms (including sodium: chloride symporter activity, anion: sodium symporter activity, and dopamine binding; Figure 6B; Table 5).
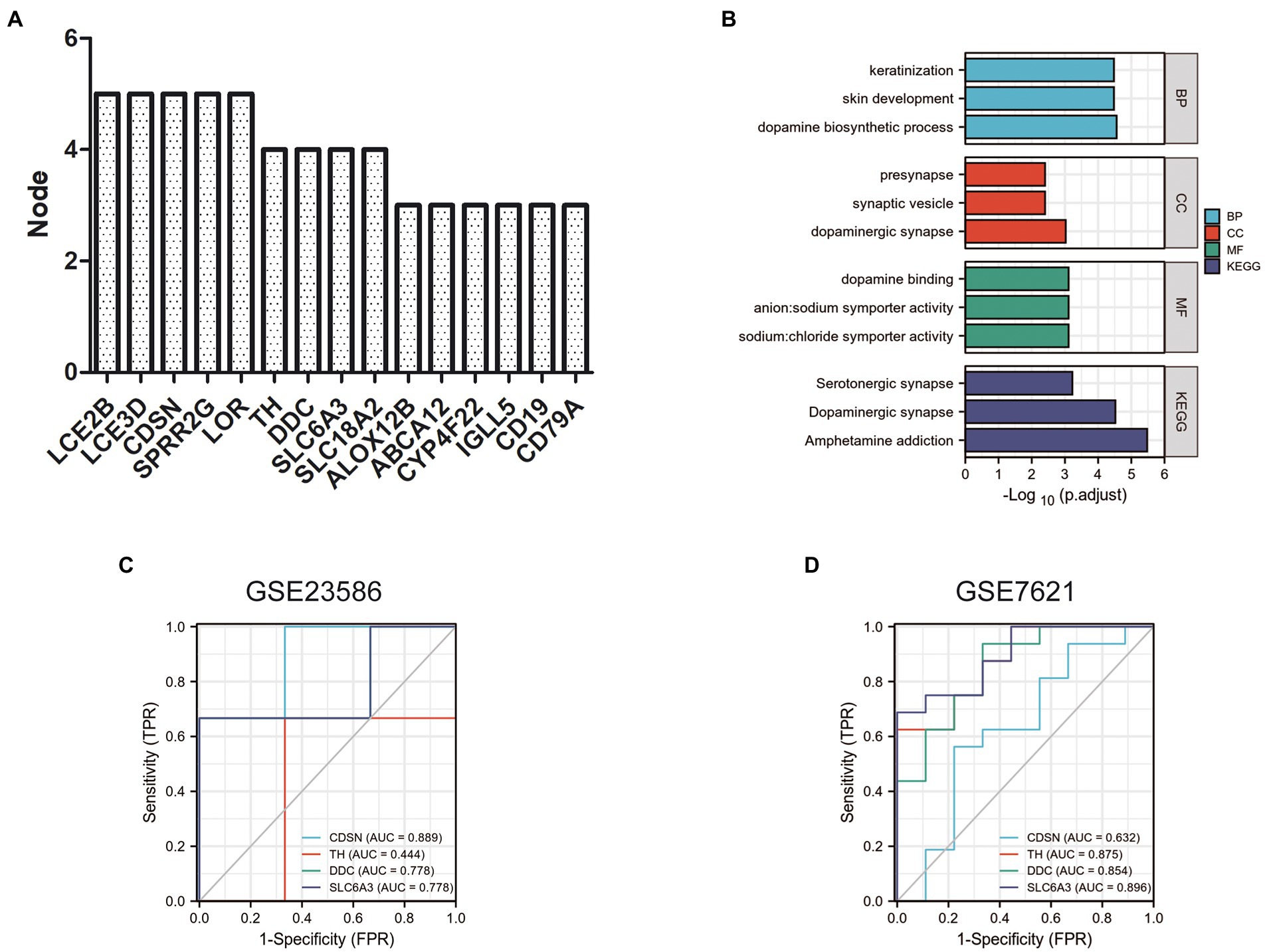
Figure 6. Functional enrichment analysis and ROC curve of hub genes. (A) Top 15 nodes of PPI networks of DEGs in GSE23586 and GSE7621 dataset. (B) GO and KEGG analysis of hub genes. Diagnostic value of 4 hub genes with ROC curves in GSE23586 dataset (C) and in GSE23586 dataset (D). AUC area under the ROC curve.
Furthermore, these 15 hub genes were significantly enriched in three KEGG signaling pathways: amphetamine addiction, dopaminergic synapses, and serotonergic synapses (Figure 6B; Table 5).
Diagnostic values of the hub genes
To verify the diagnostic value of the hub genes obtained from the above analysis, we randomly selected four hub genes for further study analyses. The ROC curves were used to calculate the corresponding area under the curve (AUC) of the hub gene expression levels in the GSE23586 and GSE23586 datasets. As shown in Figure 6C, the AUC for CDSN, TH, DDC, and SLC6A3 in periodontitis patients and normal controls were 0.889 (95% confidence interval [CI], 0.581–1.000, p < 0.05), 0.444 (95% CI, 0.000–1.000; p < 0.05), 0.778 (95% CI, 0.291–1.000; p < 0.05), and 0.778 (95% CI, 0.291–1.000; p < 0.05), respectively. In addition, the AUCs from the ROC curve of the four hub genes in the GSE7621 dataset (between PD patients and normal controls) were 0.632 (95% CI, 0.377–0.887; p < 0.05), 0.875 (95% CI, 0.737–1.000; p < 0.05), 0.854 (95% CI, 0.696–1.000; p < 0.05), and 0.896 (95% CI, 0.774–1.000; p < 0.05) (Figure 6D).
Discussion
Neurodegenerative diseases are a group of progressive diseases that affect the central nervous system (CNS); examples include AD, PD, and HD (Sengupta and Kayed, 2022). Inflammation is considered as one of the contributing factors to neurodegeneration; it plays a key role in the occurrence and progression of these injuries (Kaur et al., 2016). Periodontitis is an inflammatory disease, caused by oral biofilms in the supporting tissues of teeth, which can lead to systemic and chronic inflammatory (Alvarenga et al., 2021). It has been reported that chronic oral infections, such as periodontitis, might aggravate the neuroinflammation of HD (Coyago and Temiño, 2015). Moreover, some studies demonstrated the association between periodontitis and neuroinflammation (Sansores-España et al., 2021), cognitive decline (Wang et al., 2019). Periodontitis, which impairs the insulin signaling pathway and stimulates gliosis and neuroinflammation, is a risk factor for AD (Duan et al., 2022). Moreover, phosphoglycerol dihydroceramide (produced by the periodontal pathogen Porphyromonas gingivalis) promotes amyloidogenesis and hyperphosphorylation, and promotes the pathogenesis of AD (Yamada et al., 2020).
It is well known that the loss of dopaminergic neurons in the substantia nigra is the major cause of PD (Poewe et al., 2017). Recently, multiple lines of evidence have supported the association between PD and inflammatory processes (Joutsa et al., 2018). For example, abnormal activation of the NLRP3 inflammasome can upregulate the expression of inflammatory cytokines interleukin-1β (IL-1β) and IL-18, leading to pathological inflammation in PD (He et al., 2020). Therefore, given that periodontitis is a chronic systemic inflammation, its association with PD has attracted increasing attention from researchers. To explore causality in this bidirectional association, some researchers have used two-sample Mendelian randomization (MR) in European ancestry populations. However, the MR study found no bidirectional causal genetic predisposition between periodontitis and PD (Botelho et al., 2021). Surprisingly, some studies using population-based retrospectively matched cohorts have found that patients with periodontitis are at a higher risk of developing PD (Chen et al., 2017).
P. gingivalis, the most common bacterium responsible for chronic periodontitis, was recently found to play an important role in the pathophysiology of PD (Olsen et al., 2020). For example, oral administration of P. gingivalis not only found reduce dopaminergic neurons and increase activated microglia in the substantia nigra of mice, but it also induced an inflammatory response and promoted PD (Feng et al., 2020). In addition, there are other oral microbial infections that also play a key role in the development of PD lesions. For instance, the microbiota of patients with PD has been found to contain less Firmicutes (such as Faecalibacterium, Clostridium, and Blautia) and more Bacteroidetes (Bacteroides and Prevotella) than healthy controls (Boeri et al., 2021). The study aimed to investigate the association between neurodegenerative disorders and periodontitis.
In the present study, we obtained the common DEGs between periodontitis and PD from two GEO datasets, and performed GO and KEGG enrichment analyses. Our results suggest that the common DEGs were mainly enriched in nervous, immunity, and other related pathways, indicating that there may be a relationship between these two inflammation-related diseases. By constructing a PPI network, we identified 15 key hub genes. Through more in-depth enrichment analyses of these hub genes, our speculation was verified; that is, these genes were found to be mainly involved in PD-related pathways, such as dopamine, nervous, and immunity pathways. These results suggest that the identified hub genes may play a key role in the occurrence and progression of PD. To further verify whether these genes could serve as potential PD diagnostic or therapeutic markers, we evaluated the ROC diagnostic value of four genes (TH, CDSN, DDC, and SLC6A3), and the results showed that they have a high diagnostic value. It has been reported that most of midbrain dopamine (mDA) neurons is permanently lost by the clinical diagnosis of PD (Kishinevsky et al., 2018). Therefore, the development of predictive biomarkers is a key area of PD research. The hub genes screened in this study might become potential diagnostic or therapeutic biomarkers of PD in future.
Tyrosine hydroxylase (TH) is a key enzyme that controls the synthesis of catecholamines, including dopamine, and plays a key role in PD caused by the degeneration of the dopaminergic neurons (Nagatsu et al., 2019). It has been reported that surviving neurons in the brains of PD patients have significantly increased levels of TH phosphorylation (Shehadeh et al., 2019). Moreover, obesity-related stimuli upregulate TH levels in periodontal cells and tissues, indicating that TH may be a key pathogenic factor in obesity-induced periodontitis (Memmert et al., 2019). Corneodesmosin (CDSN) is primarily involved in the development of the nervous system and in keratinization. It has been reported that CDSN gene mutation causes scalp peeling and hypotrichosis simplex of the scalp (van der Velden et al., 2020). Dopa Decarboxylase (DDC) is a pyridoxal 5′-phosphate (PLP) enzyme involved in the biosynthesis of dopamine and serotonin, and is associated with PD (Daidone et al., 2012). In addition, SLC6A3 is a risk factor for PD (Zhai et al., 2014). The SLC6A3 genotype has a significant effect on fronto-striatal activation and performance in PD (Habak et al., 2014). These findings are consistent with the diagnostic value we assessed. Overall, TH, DDC, and SLC6A3 were the hub genes obtained in our study, indicating that our results are verifiable and credible.
In summary, using a series of bioinformatics tools for gene expression profiling, we established PPI network between PD and periodontitis, and screened key candidate genes, including CDSN, TH, DDC, and SLC6A3. Simultaneously, we enriched signaling pathways in the molecular modulation network through bioinformatics analysis. Our findings suggest a close relationship between chronic periodontitis and PD. Our results provide prospective targets for the future diagnosis and treatment of PD patients with chronic periodontitis. Furthermore, subsequent in vitro and in vivo studies should be performed to validate our findings. Our results may guide future research on the molecular mechanisms underlying the relationship between PD and periodontitis, and may provide new potential targets for diagnosis and therapy.
Data availability statement
The datasets presented in this study can be found in online repositories. The names of the repository/repositories and accession number (s) can be found in the article/Supplementary material.
Author contributions
MH and CY designed this study. XW and MH were responsible for the interpretation of the results. LY and BW processed the data processing and performed the statistical analysis. XW and NS wrote and revised the manuscript. All authors contributed to the article and approved the submitted version.
Funding
This research was supported by Department of Science and Technology of Jilin Province (grant nos. YDZJ202201ZYTS021, YDZJ202201ZYTS033, and 20200201398JC).
Conflict of interest
The authors declare that the research was conducted in the absence of any commercial or financial relationships that could be construed as a potential conflict of interest.
Publisher’s note
All claims expressed in this article are solely those of the authors and do not necessarily represent those of their affiliated organizations, or those of the publisher, the editors and the reviewers. Any product that may be evaluated in this article, or claim that may be made by its manufacturer, is not guaranteed or endorsed by the publisher.
Footnotes
References
Alameer, A. X., and Chicco, D. (2021). geoCancerPrognosticDatasetsRetriever: a bioinformatics tool to easily identify cancer prognostic datasets on gene expression omnibus (GEO). Bioinformatics. 22:btab852. doi: 10.1093/bioinformatics/btab852
Alvarenga, M. O. P., Frazão, D. R., de Matos, I. G., Bittencourt, L. O., Fagundes, N. C. F., Rösing, C. K., et al. (2021). Is there any association between neurodegenerative diseases and periodontitis? A systematic review. Front. Aging Neurosci. 13:651437. doi: 10.3389/fnagi.2021.651437
Barrett, T., Fau-Ledoux, W. S., Pierre, L. P., Fau-Evangelista, C., Fau-Kim, E. C., Irene, F., et al. (2013). NCBI GEO: archive for functional genomics data sets--update. Nucleic Acids Res. 41, D991–D995. doi: 10.1093/nar/gks1193
Boeri, L., Perottoni, S., Izzo, L., Giordano, C., and Albani, D. (2021). Microbiota-host immunity communication in neurodegenerative disorders: bioengineering challenges for in vitro modeling. Adv. Healthc. Mater. 10:e2002043. doi: 10.1002/adhm.202002043
Botelho, J., Lyra, P., Proença, L., Godinho, C., Mendes, J. J., Machado, V., et al. (2020a). Relationship between blood and standard biochemistry levels with periodontitis in Parkinson's disease patients: data from the NHANES 2011-2012. J. Pers. Med. 10:69. doi: 10.3390/jpm10030069
Botelho, J., Machado, V. X., Mendes, J. J., and Mascarenhas, P. (2021). Causal association between periodontitis and Parkinson's disease: a bidirectional Mendelian randomization study. Genes 12:772. doi: 10.3390/genes12050772
Botelho, J., Mascarenhas, P., Mendes, J. J., and Machado, V. X. (2020b). Network protein interaction in Parkinson's disease and periodontitis interplay: a preliminary Bioinformatic analysis. Genes 11:1385. doi: 10.3390/genes11111385
Chen, C. K., Wu, Y. T., and Chang, Y. C. (2017). Periodontal inflammatory disease is associated with the risk of Parkinson's disease: a population-based retrospective matched-cohort study. PeerJ 5:e3647. doi: 10.7717/peerj.3647
Corrêa, J. D., Calderaro, D. C., Ferreira, G. A., Mendonça, S. M., Fernandes, G. R., Xiao, E., et al. (2017). Subgingival microbiota dysbiosis in systemic lupus erythematosus: association with periodontal status. Microbiome 5:34. doi: 10.1186/s40168-017-0252-z
Coyago, M. L., and Temiño, V. E. (2015). Periodontitis determining the onset and progression of Huntington's disease: review of the literature. Medwave 15:e6293. doi: 10.5867/medwave.2015.09.6293
Czesnikiewicz-Guzik, M., Osmenda, G., Siedlinski, M., Nosalski, R., Pelka, P., Nowakowski, D., et al. (2019). Causal association between periodontitis and hypertension: evidence from Mendelian randomization and a randomized controlled trial of non-surgical periodontal therapy. Eur. Heart J. 40, 3459–3470. doi: 10.1093/eurheartj/ehz646
Daidone, F., Fau-Paiardini, M. R., Alessandro, P. A., Fau-Cellini, B., Fau-Macchiarulo, C. B., Antonio, M. A., et al. (2012). Identification by virtual screening and in vitro testing of human DOPA decarboxylase inhibitors. PLoS One 7:e31610. doi: 10.1371/journal.pone.0031610
Duan, L., Qian, X., Wang, Q., Huang, L., and Ge, S. (2022). Experimental periodontitis deteriorates cognitive function and impairs insulin signaling in a Streptozotocin-induced Alzheimer's disease rat model. J. Alzheimers Dis. 88, 57–74. doi: 10.3233/JAD-215720
Dutzan, N., Abusleme, L., Bridgeman, H., Greenwell-Wild, T., Zangerle-Murray, T., Fife, M. E., et al. (2017). On-going mechanical damage from mastication drives homeostatic Th17 cell responses at the Oral barrier. Immunity 46, 133–147. doi: 10.1016/j.immuni.2016.12.010
Edgar, R., Fau-Lash, D. M., Alex, E., and Lash, A. E. (2002). Gene expression omnibus: NCBI gene expression and hybridization array data repository. Nucleic Acids Res. 30, 207–210. doi: 10.1093/nar/30.1.207
Feng, Y. K., Wu, Q. L., Peng, Y. W., Liang, F. Y., You, H. J., Feng, Y. W., et al. (2020). Oral P. gingivalis impairs gut permeability and mediates immune responses associated with neurodegeneration in LRRK2 R1441G mice. J. Neuroinflammat. 17:347. doi: 10.1186/s12974-020-02027-5
Field, M. A. (2021). Detecting pathogenic variants in autoimmune diseases using high-throughput sequencing. Immunol. Cell Biol. 99, 146–156. doi: 10.1111/imcb.12372
Habak, C., Noreau, A., Nagano-Saito, A., Mejía-Constaín, B., Degroot, C., Strafella, A. P., et al. (2014). Dopamine transporter SLC6A3 genotype affects cortico-striatal activity of set-shifts in Parkinson's disease. Brain 137, 3025–3035. doi: 10.1093/brain/awu251
Hashimoto, S., Matsuba, Y., Kamano, N., Mihira, N., Sahara, N., Takano, J., et al. (2019). Tau binding protein CAPON induces tau aggregation and neurodegeneration. Nat. Commun. 10:2394. doi: 10.1038/s41467-019-10278-x
He, M., Chiang, H. H., Luo, H., Zheng, Z., Qiao, Q., Wang, L., et al. (2020). An acetylation switch of the NLRP3 inflammasome regulates aging-associated chronic inflammation and insulin resistance. Cell Metab. 31, 580–591.e5. doi: 10.1016/j.cmet.2020.01.009
Jiang, Z., Shi, Y., Zhao, W., Zhou, L., Zhang, B., Xie, Y., et al. (2021). Association between chronic periodontitis and the risk of Alzheimer's disease: combination of text mining and GEO dataset. BMC Oral. Health 21:466. doi: 10.1186/s12903-021-01827-2
Joutsa, J., Horn, A., Hsu, J., and Fox, M. D. (2018). Localizing parkinsonism based on focal brain lesions. Brain 141, 2445–2456. doi: 10.1093/brain/awy161
Kaur, T., Uppoor, A., and Naik, D. (2016). Parkinson's disease and periodontitis – the missing link? A review. Gerodontology 33, 434–438. doi: 10.1111/ger.12188
Kern, F., Krammes, L., Danz, K., Diener, C., Kehl, T., Küchler, O., et al. (2021). Validation of human microRNA target pathways enables evaluation of target prediction tools. Nucleic Acids Res. 49, 127–144. doi: 10.1093/nar/gkaa1161
Kishinevsky, S., Wang, T., Rodina, A., Chung, S. Y., Xu, C., Philip, J., et al. (2018). HSP90-incorporating chaperome networks as biosensor for disease-related pathways in patient-specific midbrain dopamine neurons. Nat. Commun. 9:4345. doi: 10.1038/s41467-018-06486-6
Liang, G., Shi, H., Qi, Y., Li, J., Jing, A., Liu, Q., et al. (2020). Specific anti-biofilm activity of carbon quantum dots by Destroying P. gingivalis biofilm related genes. Int. J. Nanomed. 15, 5473–5489. doi: 10.2147/IJN.S253416
Memmert, S., Damanaki, A., Nogueira, A. V. B., Nokhbehsaim, M., Götz, W., Cirelli, J. A., et al. (2019). Regulation of tyrosine hydroxylase in periodontal fibroblasts and tissues by obesity-associated stimuli. Cell Tissue Res. 375, 619–628. doi: 10.1007/s00441-018-2941-8
Nagatsu, T., Nakashima, A., Ichinose, H., and Kobayashi, K. (2019). Human tyrosine hydroxylase in Parkinson's disease and in related disorders. J. Neural Transm. 126, 397–409. doi: 10.1007/s00702-018-1903-3
Olsen, I., Kell, D. B., and Pretorius, E. (2020). Is Porphyromonas gingivalis involved in Parkinson's disease? Eur. J. Clin. Microbiol. Infect. Dis. 39, 2013–2018. doi: 10.1007/s10096-020-03944-2
Patra, B. G., Maroufy, V., Soltanalizadeh, B., Deng, N., Zheng, W. J., Roberts, K., et al. (2020). A content-based literature recommendation system for datasets to improve data reusability – a case study on gene expression omnibus (GEO) datasets. J. Biomed. Inform. 104:103399. doi: 10.1016/j.jbi.2020.103399
Poewe, W., Seppi, K., Tanner, C. M., Halliday, G. M., Brundin, P., Volkmann, J., et al. (2017). Parkinson disease. Nat. Rev. Dis. Primers. 3:17013. doi: 10.1038/nrdp.2017.13
Sansores-España, L., Melgar-Rodríguez, S., Olivares-Sagredo, K., Cafferata, E., Martínez-Aguilar, V., Vernal, R., et al. (2021). Oral-gut-brain Axis in experimental models of periodontitis: associating gut Dysbiosis with neurodegenerative diseases. Front. Aging 2:781582. doi: 10.3389/fragi.2021.781582
Sengupta, U., and Kayed, R. (2022). Amyloid β, tau, and α-Synuclein aggregates in the pathogenesis, prognosis, and therapeutics for neurodegenerative diseases. Prog. Neurobiol. 214:102270. doi: 10.1016/j.pneurobio.2022.102270
Shehadeh, J., Double, K. L., Murphy, K. E., Bobrovskaya, L., Reyes, S., Dunkley, P. R., et al. (2019). Expression of tyrosine hydroxylase isoforms and phosphorylation at serine 40 in the human nigrostriatal system in Parkinson's disease. Neurobiol. Dis. 130:104524. doi: 10.1016/j.nbd.2019.104524
Szklarczyk, D., Franceschini, A., Wyder, S., Forslund, K., Heller, D., Huerta-Cepas, J., et al. (2015). STRING v10: Protein-protein interaction networks, integrated over the tree of life. Nucleic Acids Res. 43, D447–D452. doi: 10.1093/nar/gku1003
Szklarczyk, D., Gable, A. L., Lyon, D., Junge, A., Wyder, S., Huerta-Cepas, J., et al. (2019). STRING v11: protein-protein association networks with increased coverage, supporting functional discovery in genome-wide experimental datasets. Nucleic Acids Res. 47, D607–D613. doi: 10.1093/nar/gky1131
van der Velden, J. J. A. J., van Geel, M., Engelhart, J. J., Jonkman, M. F., and Steijlen, P. M. (2020). Mutations in the CDSN gene cause peeling skin disease and hypotrichosis simplex of the scalp. J. Dermatol. 47, 3–7. doi: 10.1111/1346-8138.15136
Wang, R., Ho, Y. S., Leung, W. K., Goto, T., and Chang, R. C. (2019). Systemic inflammation linking chronic periodontitis to cognitive decline. Brain Behav. Immun. 81, 63–73. doi: 10.1016/j.bbi.2019.07.002
Xiao, E., Mattos, M., Vieira, G. H. A., Chen, S., Corrêa, J. D., Wu, Y., et al. (2017). Diabetes enhances IL-17 expression and alters the Oral microbiome to increase its pathogenicity. Cell Host Microbe 22, 120–128.e4. doi: 10.1016/j.chom.2017.06.014
Xu, Q., Shoji, M., Shibata, S., Naito, M., Sato, K., Elsliger, M. A., et al. (2016). A distinct type of pilus from the human microbiome. Cells 165, 690–703. doi: 10.1016/j.cell.2016.03.016
Yamada, C., Akkaoui, J., Ho, A., Duarte, C., Deth, R., Kawai, T., et al. (2020). Potential role of phosphoglycerol dihydroceramide produced by periodontal pathogen Porphyromonas gingivalis in the pathogenesis of Alzheimer's disease. Front. Immunol. 11:591571. doi: 10.3389/fimmu.2020.591571
Ye, P., Chang, J., Foo, L. F., and Yap, B. C. (2017). An early report: a modified porphyrin-linked metronidazole targeting intracellular Porphyromonas gingivalis in cultured oral epithelial cells. Int. J. Oral Sci. 9, 167–173. doi: 10.1038/ijos.2017.31
Zeng, F., Liu, Y., Huang, W., Qing, H. X., Kadowaki, T., Kashiwazaki, H., et al. (2021). Receptor for advanced glycation end products up-regulation in cerebral endothelial cells mediates cerebrovascular-related amyloid β accumulation after Porphyromonas gingivalis infection. J. Neurochem. 158, 724–736. doi: 10.1111/jnc.15096
Keywords: periodontitis, Parkinsion’s disease, protein–protein network interaction, differentially expressed genes, bioinformatics
Citation: Wang X, Shi N, Wu B, Yuan L, Chen J, Ye C and Hao M (2022) Bioinformatics analysis of gene expression profile and functional analysis in periodontitis and Parkinson’s disease. Front. Aging Neurosci. 14:1029637. doi: 10.3389/fnagi.2022.1029637
Edited by:
Michael J. Hurley, University College London, United KingdomReviewed by:
Chiara Fenoglio, University of Milan, ItalyYicheng Zhao, Changchun University of Chinese Medicine, China
Copyright © 2022 Wang, Shi, Wu, Yuan, Chen, Ye and Hao. This is an open-access article distributed under the terms of the Creative Commons Attribution License (CC BY). The use, distribution or reproduction in other forums is permitted, provided the original author(s) and the copyright owner(s) are credited and that the original publication in this journal is cited, in accordance with accepted academic practice. No use, distribution or reproduction is permitted which does not comply with these terms.
*Correspondence: Miao Hao, miaohao@jlu.edu.cn; Cong Ye, yecong0228@jlu.edu.cn