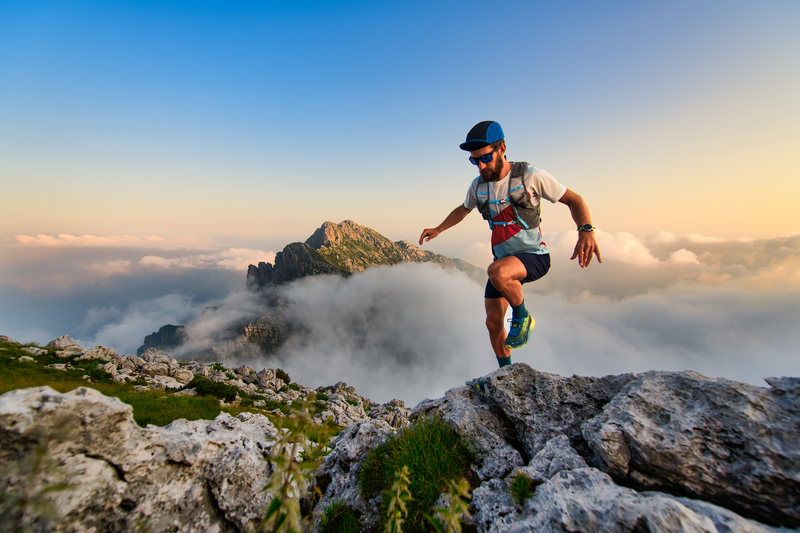
94% of researchers rate our articles as excellent or good
Learn more about the work of our research integrity team to safeguard the quality of each article we publish.
Find out more
ORIGINAL RESEARCH article
Front. Aging Neurosci. , 18 November 2021
Sec. Neurocognitive Aging and Behavior
Volume 13 - 2021 | https://doi.org/10.3389/fnagi.2021.755630
This article is part of the Research Topic Affective, Cognitive and Social Neuroscience: New Knowledge in Normal Aging, Minor and Major Neurocognitive Disorders View all 25 articles
Background: Mild cognitive impairment (MCI) is the prodromal phase of Alzheimer’s disease (AD) and has a high risk of progression to AD. Cigarette smoking is one of the important modifiable risk factors in AD progression. Cholinergic dysfunction, especially the nucleus basalis of Meynert (NBM), is the converging target connecting smoking and AD. However, how cigarette smoking affects NBM connectivity in MCI remains unclear.
Objective: This study aimed to evaluate the interaction effects of condition (non-smoking vs. smoking) and diagnosis [cognitively normal (CN) vs. MCI] based on the resting-state functional connectivity (rsFC) of the NBM.
Methods: After propensity score matching, we included 86 non-smoking CN, 44 smoking CN, 62 non-smoking MCI, and 32 smoking MCI. All subjects underwent structural and functional magnetic resonance imaging scans and neuropsychological tests. The seed-based rsFC of the NBM with the whole-brain voxel was calculated. Furthermore, the mixed effect analysis was performed to explore the interaction effects between condition and diagnosis on rsFC of the NBM.
Results: The interaction effects of condition × diagnosis on rsFC of the NBM were observed in the bilateral prefrontal cortex (PFC), bilateral supplementary motor area (SMA), and right precuneus/middle occipital gyrus (MOG). Specifically, the smoking CN showed decreased rsFC between left NBM and PFC and increased rsFC between left NBM and SMA compared with non-smoking CN and smoking MCI. The smoking MCI showed reduced rsFC between right NBM and precuneus/MOG compared with non-smoking MCI. Additionally, rsFC between the NBM and SMA showed a significant negative correlation with Wechsler Memory Scale-Logical Memory (WMS-LM) immediate recall in smoking CN (r = −0.321, p = 0.041).
Conclusion: Our findings indicate that chronic nicotine exposure through smoking may lead to functional connectivity disruption between the NBM and precuneus in MCI patients. The distinct alteration patterns on NBM connectivity in CN smokers and MCI smokers suggest that cigarette smoking has different influences on normal and impaired cognition.
Alzheimer’s disease (AD) is the most common type of dementia in the elderly people, accompanied by progressive and irreversible cognitive decline, affecting over 50 million people worldwide (Prince et al., 2015). Mild cognitive impairment (MCI) refers to the symptomatic pre-dementia phase of AD. Numerous longitudinal studies have reported that MCI is associated with a high risk of progression to AD (Larrieu et al., 2002). However, there is currently no effective treatment to reverse the course of AD. Exploring the early cerebral functional changes in patients with MCI could provide objective bases for guiding clinical intervention and treatment.
Human neuropathological studies showed that the degeneration of cholinergic systems is one of the earliest pathophysiological events in AD (Mesulam et al., 2004; Geula et al., 2008). The cholinergic system mediates a wide range of cognitive functions, including memory, learning, attention, and other high-order brain functions (Hampel et al., 2018). As the most important component of the basal forebrain cholinergic system, the nucleus basalis of Meynert (NBM) provides the primary sources of cholinergic innervation to the limbic and neocortical regions (Gratwicke et al., 2013; Liu et al., 2015). Early postmortem studies of AD patients have emphasized a profound loss of cholinergic neurons, particularly the NBM (Whitehouse et al., 1981, 1982). More importantly, cholinergic dysfunction in the NBM is highly associated with the neurofibrillary tangle, which is the hallmark of AD neuropathology (Mesulam et al., 2004; Mesulam, 2013).
Cigarette smoking is one of the important modifiable risk factors in AD progression (Durazzo et al., 2014; Kivipelto et al., 2018; Johnson et al., 2021). Heavy smoking is associated with a greater than 100% increase in the risk of AD after two decades of exposure (Rusanen et al., 2011). Besides the negative effects on the cerebrovascular burden, cigarette smoking also affects cholinergic functions by its action on nicotinic acetylcholine receptors (nAChRs). For example, chronic nicotine exposure could cause the upregulation of nAChRs or the loss of nicotine-sensitive nAChRs functional activity (Mehta et al., 2012). In addition, smoking-related oxidative stress could also facilitate the extracellular fibrillar β-amyloid (Aβ) aggregation and abnormal tau phosphorylation (Ho et al., 2012; Moreno-Gonzalez et al., 2013), which are the neuropathological hallmarks of AD. Furthermore, Aβ accumulation shows strong co-localization at nAChRs, causing the amyloid-mediated degeneration of the cholinergic system (Buckingham et al., 2009). Thus, cigarette smoking might induce cognitive decline by cholinergic systems, and the NBM could be an important target.
Structural and functional magnetic resonance imaging (MRI) could evaluate the atrophy and functional activity alterations of the NBM in vivo. In cognitively healthy individuals, past or current smoking was significantly associated with smaller NBM volume (Teipel et al., 2016). In addition, reduced volume in the NBM was also observed in MCI patients, especially the smokers, and atrophy was more extensive and included the whole basal forebrain in AD patients (Grothe et al., 2012; Kilimann et al., 2014; Brueggen et al., 2015). Numerous functional MRI studies have provided consistent evidence that functional connectivity (FC) disruption precedes structural atrophy and reflect the underlying pathophysiological alterations (Pievani et al., 2011). Based on the resting-state functional MRI (rsfMRI), the NBM showed decreased FC with the posterior cingulate cortex, precuneus, and cuneus in smokers as compared with non-smokers (Zhang et al., 2017). Few studies also found decreased FC of the NBM to insula, precuneus, and lingual gyrus in MCI patients when compared with healthy control (Li et al., 2017; Xu et al., 2021). However, how cigarette smoking affects the FC alterations of the NBM in MCI patients remains unclear.
In this study, we aimed to investigate the smoking effects on the FC alterations of the NBM in patients with MCI. Based on previous work, we hypothesized that the smoking MCI group might have more serious FC disruption of the NBM than the non-smoking MCI group, especially in regions related to nicotine addiction or memory.
The data set used in this study was obtained from the Alzheimer’s Disease Neuroimaging Initiative (ADNI) database1. The ADNI was launched in 2003 by the National Institute on Aging (NIA), the National Institute of Biomedical Imaging and Bioengineering (NIBIB), the Food and Drug Administration (FDA), private pharmaceutical companies, and non-profit organizations, as a $60 million 5-year public–private partnership. The primary goal of ADNI is to test whether serial MRI, positron emission tomography (PET), other biological markers, and clinical and neuropsychological assessment can be combined to measure the progression of MCI and early AD. Determination of sensitive and specific markers of very early AD progression is intended to aid researchers and clinicians in developing new treatments and monitor their effectiveness, as well as lessen the time and cost of clinical trials.
The Institutional Review Board approved the study of all the participating institutions. Informed written consent was obtained from all participants at each site. Based on the ADNI three databases, we identified 378 right-handed CN subjects and 182 MCI patients with complete neuropsychological evaluations, structural MRI, and rsfMRI scans. We downloaded data from the ADNI database before March 2021. The criteria for MCI in ADNI were: (1) subjective memory complaints, either self-reported, reported by a study partner, or reported by a clinician; (2) objective memory loss defined as scoring below education adjusted cutoff score on delayed recall of the Wechsler Memory Scale-Logical Memory (WMS-LM) test; (3) a global Clinical Dementia Rating (CDR) score of 0.5; (4) a Mini-Mental State Examination (MMSE) score of equal to, or higher than, 24 out of 30; (5) general cognitive and functional performance sufficiently preserved such that a diagnosis of dementia could not be made by the site physician at the time of screening; and (6) no signs of depression (Geriatric Depression Scale, GDS < 6). Criteria for CN were defined as an MMSE score equal to or higher than 24 out of 30, a CDR score of 0, having no report of any cognition complaint, and no signs of depression. Then, we classified MCI and CN subjects into non-smoking and smoking subgroups separately based on self-reported smoking history. Non-smoking subgroup included participants who reported that they never smoked cigarettes during their lifetime, and smoking subgroup included participants who reported any history of smoking (Supplementary Table 1). After screening, 49 participants (including 29 non-smoking CN, 1 smoking CN, and 19 non-smoking MCI) were excluded for excessive head motion (details later). Finally, 304 non-smoking CN, 44 smoking CN, 130 non-smoking MCI, and 33 smoking MCI subjects entered subsequent analyses.
Demographics, including age, sex, education level, hypertension, diabetes mellitus, and hypercholesterolemia, were assessed. Hypertension was defined as systolic blood pressure >140 mm Hg, diastolic blood pressure >90 mm Hg, medical history of hypertension, or treatment with antihypertensive medication. Diabetes mellitus was defined as random blood glucose ≥11 mmol/dL, medical history of diabetes mellitus, or treatment with glucose-lowering medication. Hypercholesterolemia was defined as random blood cholesterol ≥11 mmol/L, medical history of hypercholesterolemia, or treatment with lipid-lowering medication. Neuropsychological tests in different cognitive domains, such as memory (WMS-LM, immediate and delayed recall), attention [trail-making test, Part A (TMT-A)], execution [trail-making test, Part B (TMT-B)], and language [semantic verbal fluency (SVF)], were included. The demographics and neuropsychological performance of all subjects are listed in Supplementary Table 2.
All subjects were scanned using a 3.0-Tesla Philips MRI scanner. The structural images were acquired using a 3D magnetization prepared rapid gradient echo (MPRAGE) T1-weighted sequence with the following parameters: repetition time (TR) = 2,300 ms; echo time (TE) = 2.98 ms; inversion time (TI) = 900 ms; 170 sagittal slices; within plane FOV = 256 mm × 240 mm; voxel size = 1 mm × 1 mm × 1 mm; flip angle = 9°; bandwidth = 240 Hz/pix. The rsfMRI images were obtained using an echo-planar imaging sequence with the following parameters: 197 time points; TR = 3,000 ms; TE = 30 ms; slice thickness = 3.39 mm; spatial resolution = 3.39 mm × 3.39 mm × 3.39 mm; flip angle = 90°; and matrix = 64 × 64.
We preprocessed rsfMRI data using the Data Processing & Analysis for Brain Imaging (DPABI2) (Yan et al., 2016) with Statistical Parametric Mapping software (SPM123) on the MATLAB platform (MathWorks, Natick, MA, United States). The first 10 time points of rsfMRI data were discarded due to the instability of the initial MRI signal and the adaptation of subjects to the scanning noise. The remaining 187 images were corrected for timing differences between each slice and head motion (six-parameter rigid body). Subjects with more than 3.0 mm maximum displacement in any of the x, y, or z directions or 3.0° of any angular motion were discarded (including 29 non-smoking CN, 1 smoking CN, and 19 non-smoking MCI). Subsequently, rsfMRI images were spatially normalized to the standard EPI template and resampled into 3 mm × 3 mm × 3 mm. The rsfMRI images were spatially smoothed with a Gaussian kernel of 6 mm × 6 mm × 6 mm full width at half maximum. Finally, linear trends and temporally filter (0.01 Hz < f < 0.08 Hz) were performed. Nuisance covariate regression was performed to minimize physiological noise using the Friston-24 head motion parameters (6 head motion parameters, 6 head motion parameters from the previous time point, and the 12 corresponding squared items), as well as white matter (WM) signal and corticospinal fluid (CSF) signal. In addition, the framewise displacement (FD) Jenkinson value of each subject was calculated to correct for the head motion artifacts.
The T1-weighted images were preprocessed and analyzed using the Computational Anatomy Toolbox (CAT124) and SPM12. The images were bias-corrected, tissue-classified [gray matter (GM), WM, and CSF], and registered using linear (12 parameter affine) and non-linear transformations (warping) within the CAT12 default preprocessing pipeline.
The seed NBM was identified using a probabilistic anatomical map from the SPM Anatomy Toolbox, which was microscopically delineated, 3D reconstructed, and warped to the reference space of the Montreal Neurological Institute (MNI) brain from 10 postmortem human brains (Zaborszky et al., 2008). The NBM, which is the largest of the cholinergic cell clusters constituting the basal forebrain, provides the primary source of cholinergic innervation to the cortex (Gratwicke et al., 2013; Liu et al., 2015). Dynamic brain connectome (DynamicBC) analysis toolbox5 (Liao et al., 2014) was used to create individual subject seed-to-voxel connectivity maps. First, each mask was resampled to the dimension of our normalized functional image with 3 × 3 × 3 voxel size for seed-based resting-state functional connectivity (rsFC) analyses. Next, the rsFC maps were generated by calculating the Pearson’s correlation between the time course of the NBM and whole brain areas. Finally, the resulting rsFC maps were transformed to Z maps using Fisher’s Z transformation.
Propensity score matching (PSM) implanted in Statistical Product and Service Solutions (SPSS version 26) was performed to balance the differences in demographic features between non-smoking and smoking subgroups in CN and MCI and to reduce the bias due to confounding factors. A propensity score was estimated using multiple logistic regression analysis based on the following covariates: age, sex, and education level. A 1:2 matching was used to pair participants with smoking CN and smoking MCI, respectively. Significant testing and standardized difference (d) were applied to assess the balance of covariates before and after PSM. Through PSM, 86 non-smoking CN, 44 smoking CN, 62 non-smoking MCI, and 32 smoking MCI were selected from the initial population. The distribution of propensity scores was relatively balanced after matching (Supplementary Figure 1). There was no significant difference of the key covariates between the smoking and non-smoking groups in both CN and MCI, and the d values were acceptable (Supplementary Table 3), suggesting balanced demographic features in CN and MCI subgroups.
We rated the total MRI burden of small vessel disease (SVD) on an ordinal scale from 0 to 4, by counting the presence of each of the four MRI features of SVD, including white matter hyperintensity (WMH), lacunes, cerebral microbleeds (CMBs), and perivascular spaces (PVS) (Staals et al., 2014). The presence of WMH was defined as either confluent deep WMH (Fazekas score 2 or 3) or irregular periventricular WMH extending into the deep WM (Fazekas score 3) on T2 fluid-attenuated inversion recovery (FLAIR) (1 point if present). The presence of lacunes and CMBs was defined as the presence of one or more lacunes on T2 FLAIR (1 point if present) or any CMB on T2∗-weighted gradient echo images (1 point if present). The presence of PVS was counted if there were moderate to severe (grades 2–4) PVS in the basal ganglia on T1-weighted axial images (1 point if present) (Zhu et al., 2010). The detailed SVD scores are listed in Supplementary Table 4.
The statistical analyses of demographics and neuropsychological data were performed using IBM SPSS 26.0 statistical software. We performed a group-level analysis using a one-way ANOVA for continuous variables. If group-level test results were significant, post hoc pairwise comparisons were performed (Bonferroni’s multiple comparison correction in parametric tests and Dunn’s multiple comparison tests in non-parametric tests). Binary data, such as sex, hypertension, diabetes mellitus, and hypercholesterolemia, were compared between groups using a chi-square test.
The statistical analyses of the rsFC of the NBM were performed using the DPABI toolbox (Yan et al., 2016). Specifically, we performed a 2 × 2 mixed effect analysis to explore the main effects of condition (non-smoking vs. smoking) and diagnosis (CN vs. MCI), as well as the interaction effects of condition × diagnosis. Age, sex, education level, and head motion (FD value) were used as covariates. To control the effect of cortical atrophy on the functional analysis, normalized modulated (with the volumetric information encoded) GM maps were used as covariate images. The threshold was set to the voxel level at p < 0.005 and the cluster level at p < 0.05 after Gaussian random field (GRF) correction. The interaction effects between condition and diagnosis before PSM are listed in Supplementary Table 5 and Figure 2. In addition, we also evaluated the interaction effects after controlling for SVD scores (Supplementary Figure 3). To further understand how condition and diagnosis interacted on the rsFC of the NBM, we extracted the mean rsFC values from the interaction regions and further performed post hoc pairwise comparisons (p < 0.05, Bonferroni’s correction). Finally, partial correlation analysis was performed to investigate the correlation between the mean rsFC values of interaction regions and neuropsychological scores with age, sex, and education level as covariates (p < 0.05).
After PSM, a total of 86 non-smoking CN, 44 smoking CN, 62 non-smoking MCI, and 32 smoking MCI were included. The demographics and neuropsychological performance are summarized in Table 1. There were no significant differences between subgroups in age, sex, education level, hypertension, diabetes mellitus, and hypercholesterolemia (p > 0.05). The MCI subgroups (non-smoking and smoking) showed significantly poor neuropsychological performance on memory, attention, execution, and language compared with CN subgroups (p < 0.05). The smoking subgroups (CN and MCI) showed no significant differences in neuropsychological performance compared with corresponding non-smoking subgroups (p > 0.05).
The interaction effects of condition (non-smoking vs. smoking) × diagnosis (CN vs. MCI) on rsFC of the NBM after PSM are displayed in Figure 1 and Table 2. Based on the seed of left NBM, the regions with interaction effects were primarily located in the bilateral prefrontal cortex (PFC) and bilateral supplementary motor area (SMA). Based on the seed of the right NBM, the interaction region was located in the right precuneus/middle occipital gyrus (MOG) (p < 0.005). The main effects of the condition or diagnosis were not detected.
Figure 1. The interaction regions of condition × diagnosis on rsFC of the NBM. (A) Based on the seed of left NBM, the interaction regions were primarily located in bilateral PFC and SMA. (B) Based on the seed of the right NBM, the interaction region was observed in the right precuneus/MOG. NBM, nucleus basalis of Meynert; PFC, prefrontal cortex; SMA, supplementary motor area; MOG, middle occipital gyrus. The statistical threshold was set at p < 0.005 with a cluster level of p < 0.05 (two-tailed, GRF corrected).
The post hoc test revealed the group differences of rsFC values of interaction regions in four subgroups after PSM (Figure 2). Specifically, the smoking CN showed decreased rsFC between left NBM and PFC, and increased rsFC between left NBM and SMA compared with non-smoking CN and smoking MCI. The smoking MCI showed reduced rsFC between right NBM and precuneus/MOG compared with non-smoking MCI.
Figure 2. The post hoc analysis of interaction regions of rsFC of the NBM. (A) The smoking CN (sCN) showed significantly decreased rsFC of left NBM to PFC compared to non-smoking CN (nsCN), non-smoking MCI (nsMCI), and smoking MCI (sMCI). (B) The sCN showed increased rsFC of left NBM to SMA compared to nsCN and sMCI. (C) The sMCI showed decreased rsFC of right NBM to precuneus/MOG compared with nsMCI. NBM, nucleus basalis of Meynert; PFC, prefrontal cortex; SMA, supplementary motor area; MOG, middle occipital gyrus. ∗p < 0.05, ∗∗p < 0.005, ∗∗∗p < 0.001.
We studied the relationship between rsFC of the NBM and neuropsychological performance in different domains (Table 3). We only found that rsFC of the NBM to the SMA showed a significant negative correlation with memory (WMS-LM immediate recall) in the smoking CN group (r = −0.321, p = 0.041; Figure 3). After Bonferroni’s correction (p < 0.05/15) for multiple comparisons, there was no significant correlation between rsFC of the NBM and neuropsychological scores.
Figure 3. Correlation between rsFC of the NBM and neuropsychological scores. The rsFC of left NBM to SMA was negatively correlated with WMS-LM immediate recall in smoking CN (r = –0.321, p = 0.041). NBM, nucleus basalis of Meynert; SMA, supplementary motor area; WMS-LM, Wechsler Memory Scale-Logical Memory.
In this study, we mainly investigated the interaction effects between cigarette smoking and cognitive status based on the rsFC of the NBM. The interaction regions were primarily located in the bilateral PFC, bilateral SMA, and right precuneus/MOG. Furthermore, we observed different alteration patterns on rsFC of the NBM in the smoking CN group and the smoking MCI group. To the best of our knowledge, this is the first study to explore the effects of cigarette smoking on the brain function activity of MCI through the cholinergic pathway.
In the smoking CN group, decreased rsFC between the NBM and PFC was observed compared with the non-smoking CN group. The PFC underlies higher cognitive processes, such as decision-making, working memory, and attention (Passetti et al., 2002; Euston et al., 2012), modulated by nAChRs activation with cholinergic inputs (Dehaene and Changeux, 2011; Bloem et al., 2014a). As the largest cholinergic cell clusters constituting the basal forebrain cholinergic system, the NBM cholinergic neurons mainly project to the PFC (Bloem et al., 2014b; Ballinger et al., 2016). Moreover, the PFC is also an important region of the reward circuit, which plays a key role in addiction (Baker et al., 2003). Thus, our result that showed decreased rsFC between the NBM and PFC could potentially reflect the cholinergic dysfunction by cigarette smoking, which might also explain the working memory and attention decline in healthy smokers observed by previous studies (Chamberlain et al., 2012; Zhang et al., 2016). Previous findings in healthy smokers suggested that smoking was associated with reduced brain volumes (Gallinat et al., 2006; Fritz et al., 2014) or MR spectroscopy markers of neuronal viability (Durazzo et al., 2016; Faulkner et al., 2020), particularly in the PFC. In addition, rsfMRI studies (Janes et al., 2012) also demonstrated that PFC network interactions might serve as biomarkers for nicotine dependence severity and treatment efficacy. Combining our results and previous findings, we speculated that the PFC is the key brain area associated with smoking addiction and cholinergic abnormality.
We also found increased rsFC between the NBM and SMA in the smoking CN group compared with the non-smoking CN group, which is consistent with previous work (Zhang et al., 2017). Many studies have proposed that habitual mechanism plays an important role in addiction (Yalachkov et al., 2009; Jasinska et al., 2014). Individuals with greater nicotine dependence severity had increased engagement of motor preparation circuits, suggesting increased reliance on habitual behaviors (Claus et al., 2013). The SMA is the key brain area associated with automatized behavior and motor planning (Desmurget and Sirigu, 2012). Thus, we inferred that increased rsFC between NBM and SMA might suggest more automatized smoking behaviors for smokers. In previous studies on smoking addiction using rsfMRI, the SMA showed widespread brain connections with reward-related regions, such as the anterior cingulate cortex, insula, and precuneus (Ding and Lee, 2013; Shen et al., 2018; Addicott et al., 2019). Moreover, the increased connectivity of SMA with other regions such as the insula and NBM was associated with the severity of nicotine dependence (Claus et al., 2013; Zhang et al., 2017). Taken together, the SMA could be a vital functional region involved in smoking-related habitual addiction and motor planning.
Additionally, the rsFC of the NBM to SMA in smoking CN showed a negative correlation with WMS-LM immediate recall. The role of SMA in cognitive function such as memory has also been reported in previous studies (Canas et al., 2018; Marvel et al., 2019), and the long-term cigarette smoking could cause difficulty in concentration which in turn induced memory decline (Chamberlain et al., 2012; Zhang et al., 2016). Thus, we speculated that the negative correlation between rsFC of the NBM to SMA and memory could be an early compensatory mechanism to underlying attention-related memory impairment. Our result of decreased rsFC of the NBM to SMA in smoking MCI compared with smoking CN further indicated that this compensatory mechanism might be weakened as cognitive decline progressed.
In the smoking MCI group, the NBM showed decreased rsFC with precuneus/MOG compared with the non-smoking MCI group. The precuneus is an important node of the default-mode network (DMN), a system that contributes to episodic memory retrieval (Raichle et al., 2001). Patients with AD and MCI showed disrupted FC of the precuneus/DMN, which is associated with cognitive decline (Dennis and Thompson, 2014; Eyler et al., 2019). Moreover, the precuneus is also one of the earliest brain regions with Aβ deposition (Long and Holtzman, 2019), which is the hallmark of AD pathology. Longitudinal studies have emphasized that Aβ deposition in the precuneus and other cortical regions comprising the DMN in the pre-dementia stage would sequentially induce regional cortical hypometabolism, accumulation of tau pathology, and hippocampal volume loss (Gordon et al., 2016, 2018; Hanseeuw et al., 2019). However, our study lacked evidence of the correlation between rsFC values of precuneus and Aβ deposition because only a few smoking MCI patients had positive biomarker evidence (i.e., Aβ+). Further studies with Aβ+ smoking MCI patients are needed to verify the role of the precuneus in addiction and cognition. In addition, as for smoking addiction, the precuneus is also recognized as an important contributor to cue reactivity in smokers, through its role in attentional bias toward smoking cues (Engelmann et al., 2012; Courtney et al., 2014). The abnormal brain activity of the precuneus/DMN has also been observed in healthy smokers (Weiland et al., 2015; Chen and Mo, 2017; Zhang et al., 2017; Wilcox et al., 2018). It should be noted that cholinergic systems play a vital role in cognitive resilience and brain plasticity (Drever et al., 2011). Preserved cholinergic forebrain integrity could enable adaptation to the structural degeneration of MCI patients (Ray et al., 2015; Berlot et al., 2021), while smoking could be a risk factor that destroyed this balance. Therefore, we deduced that the precuneus could be an important target of cigarette smoking affecting the cognitive function of MCI through the cholinergic pathway.
Several limitations need to be addressed for this study. First, due to the limited sample size of the ADNI database, the sample sizes of the smoking CN and smoking MCI groups are relatively small compared with the non-smoking groups. In addition, the biomarkers were not included in this analysis because only a few smoking MCI patients had positive biomarker evidence (i.e., Aβ+). Future studies with a larger sample size and biomarker evidence are needed to verify our work. Second, this cross-sectional study is lacking clinical follow-up to make any possible inference between smoking and AD. Thus, longitudinal studies are needed to determine whether rsFC of the NBM alterations in smokers is related to disease progression. Third, our results of the correlation analysis did not survive after multiple comparison corrections. However, this is an explorative study, and our results could partly reflect the effects of cigarette smoking on different cognitions. Finally, smoking history in the ADNI database is defined by subjective self-report from the medical record, including former and current smokers. Many participants lack detailed records such as the number, duration, and status (former or current) of smoking. Future studies may further explore the effects of different smoking degrees or statuses on cognitive functions in MCI because these factors could affect cerebral functional activity.
Our findings indicate that chronic nicotine exposure through smoking may lead to FC disruption between the NBM and precuneus in MCI patients. Moreover, the distinct alteration patterns on NBM connectivity in CN smokers and MCI smokers suggest that cigarette smoking has different influences on normal and impaired cognition.
The data used in the preparation of this article were obtained from the Alzheimer’s Disease Neuroimaging Initiative (ADNI) database: http://adni.loni.usc.edu/.
All procedures performed in studies involving human participants were in accordance with the ethical standards of the institutional and/or national research committee and with the 1964 Helsinki declaration and its later amendments or comparable ethical standards. Written informed consent was obtained from all participants and/or authorized representatives and the study partners before any protocol-specific procedures were carried out in the ADNI study.
TQ and QZ designed the study and wrote the first draft of the manuscript. XL analyzed the MRI data and wrote the protocol. QZ and XL collected the clinical and MRI data. TX, ZS, XX, CW, KL, PH, XDL, FX, SD, and MZ assisted with the research design and interpretation of results. All authors contributed to the final manuscript and read and approved the final manuscript.
This study was funded by the National Key Research and Development Program of China (Grant No. 2016YFC1306600), Linyi Science and Technology Development Program (Grant No. 202020024), Shandong Medicine and Health Science and Technology Program (Grant No. 202009010844), Zhejiang Provincial Natural Science Foundation of China (Grant No. LY21H180003), and National Natural Science Foundation of China (Grant Nos. 81901707, 82001766, and 81901721). The data collection and sharing for this project were funded by the ADNI (National Institutes of Health Grant U01 AG024904) and DOD ADNI (Department of Defense Award No. W81XWH-12-2-0012).
The authors declare that the research was conducted in the absence of any commercial or financial relationships that could be construed as a potential conflict of interest.
All claims expressed in this article are solely those of the authors and do not necessarily represent those of their affiliated organizations, or those of the publisher, the editors and the reviewers. Any product that may be evaluated in this article, or claim that may be made by its manufacturer, is not guaranteed or endorsed by the publisher.
The Supplementary Material for this article can be found online at: https://www.frontiersin.org/articles/10.3389/fnagi.2021.755630/full#supplementary-material
Addicott, M. A., Sweitzer, M. M., and McClernon, F. J. (2019). The Effects of Nicotine and Tobacco Use on Brain Reward Function: Interaction With Nicotine Dependence Severity. Nicotine Tob. Res. 21, 764–771. doi: 10.1093/ntr/nty059
Baker, D. A., McFarland, K., Lake, R. W., Shen, H., Tang, X. C., Toda, S., et al. (2003). Neuroadaptations in cystine-glutamate exchange underlie cocaine relapse. Nat. Neurosci. 6, 743–749. doi: 10.1038/nn1069
Ballinger, E. C., Ananth, M., Talmage, D. A., and Role, L. W. (2016). Basal Forebrain Cholinergic Circuits and Signaling in Cognition and Cognitive Decline. Neuron 91, 1199–1218. doi: 10.1016/j.neuron.2016.09.006
Berlot, R., Koritnik, B., Pirtošek, Z., and Ray, N. J. (2021). Preserved cholinergic forebrain integrity reduces structural connectome vulnerability in mild cognitive impairment. J. Neurol. Sci. 425:117443. doi: 10.1016/j.jns.2021.117443
Bloem, B., Poorthuis, R. B., and Mansvelder, H. D. (2014a). Cholinergic modulation of the medial prefrontal cortex: the role of nicotinic receptors in attention and regulation of neuronal activity. Front. Neural. Circuits 8:17. doi: 10.3389/fncir.2014.00017
Bloem, B., Schoppink, L., Rotaru, D. C., Faiz, A., Hendriks, P., Mansvelder, H. D., et al. (2014b). Topographic mapping between basal forebrain cholinergic neurons and the medial prefrontal cortex in mice. J. Neurosci. 34, 16234–16246. doi: 10.1523/jneurosci.3011-14.2014
Brueggen, K., Dyrba, M., Barkhof, F., Hausner, L., Filippi, M., Nestor, P. J., et al. (2015). Basal Forebrain and Hippocampus as Predictors of Conversion to Alzheimer’s Disease in Patients with Mild Cognitive Impairment - A Multicenter DTI and Volumetry Study. J. Alzheimers Dis. 48, 197–204. doi: 10.3233/jad-150063
Buckingham, S. D., Jones, A. K., Brown, L. A., and Sattelle, D. B. (2009). Nicotinic acetylcholine receptor signalling: roles in Alzheimer’s disease and amyloid neuroprotection. Pharmacol. Rev. 61, 39–61. doi: 10.1124/pr.108.000562
Canas, A., Juncadella, M., Lau, R., Gabarros, A., and Hernandez, M. (2018). Working Memory Deficits After Lesions Involving the Supplementary Motor Area. Front. Psychol. 9:765. doi: 10.3389/fpsyg.2018.00765
Chamberlain, S. R., Odlaug, B. L., Schreiber, L. R., and Grant, J. E. (2012). Association between tobacco smoking and cognitive functioning in young adults. Am. J. Addict. 21(Suppl. 1), S14–S19. doi: 10.1111/j.1521-0391.2012.00290.x
Chen, H., and Mo, S. (2017). Regional Homogeneity Changes in Nicotine Addicts by Resting-State fMRI. PLoS One 12:e0170143. doi: 10.1371/journal.pone.0170143
Claus, E. D., Blaine, S. K., Filbey, F. M., Mayer, A. R., and Hutchison, K. E. (2013). Association between nicotine dependence severity, BOLD response to smoking cues, and functional connectivity. Neuropsychopharmacology 38, 2363–2372. doi: 10.1038/npp.2013.134
Courtney, K. E., Ghahremani, D. G., London, E. D., and Ray, L. A. (2014). The association between cue-reactivity in the precuneus and level of dependence on nicotine and alcohol. Drug Alcohol. Depend 141, 21–26. doi: 10.1016/j.drugalcdep.2014.04.026
Dehaene, S., and Changeux, J. P. (2011). Experimental and theoretical approaches to conscious processing. Neuron 70, 200–227. doi: 10.1016/j.neuron.2011.03.018
Dennis, E. L., and Thompson, P. M. (2014). Functional brain connectivity using fMRI in aging and Alzheimer’s disease. Neuropsychol. Rev. 24, 49–62. doi: 10.1007/s11065-014-9249-6
Desmurget, M., and Sirigu, A. (2012). Conscious motor intention emerges in the inferior parietal lobule. Curr. Opin. Neurobiol. 22, 1004–1011. doi: 10.1016/j.conb.2012.06.006
Ding, X., and Lee, S. W. (2013). Changes of functional and effective connectivity in smoking replenishment on deprived heavy smokers: a resting-state FMRI study. PLoS One 8:e59331. doi: 10.1371/journal.pone.0059331
Drever, B. D., Riedel, G., and Platt, B. (2011). The cholinergic system and hippocampal plasticity. Behav. Brain Res. 221, 505–514. doi: 10.1016/j.bbr.2010.11.037
Durazzo, T. C., Mattsson, N., and Weiner, M. W. (2014). Smoking and increased Alzheimer’s disease risk: a review of potential mechanisms. Alzheimers Dement 3(Suppl.), S122–S145. doi: 10.1016/j.jalz.2014.04.009
Durazzo, T. C., Meyerhoff, D. J., Mon, A., Abé, C., Gazdzinski, S., and Murray, D. E. (2016). Chronic Cigarette Smoking in Healthy Middle-Aged Individuals Is Associated With Decreased Regional Brain N-acetylaspartate and Glutamate Levels. Biol. Psychiatry 79, 481–488. doi: 10.1016/j.biopsych.2015.03.029
Engelmann, J. M., Versace, F., Robinson, J. D., Minnix, J. A., Lam, C. Y., Cui, Y., et al. (2012). Neural substrates of smoking cue reactivity: a meta-analysis of fMRI studies. Neuroimage 60, 252–262. doi: 10.1016/j.neuroimage.2011.12.024
Euston, D. R., Gruber, A. J., and McNaughton, B. L. (2012). The role of medial prefrontal cortex in memory and decision making. Neuron 76, 1057–1070. doi: 10.1016/j.neuron.2012.12.002
Eyler, L. T., Elman, J. A., Hatton, S. N., Gough, S., Mischel, A. K., Hagler, D. J., et al. (2019). Resting State Abnormalities of the Default Mode Network in Mild Cognitive Impairment: A Systematic Review and Meta-Analysis. J Alzheimers Dis 70, 107–120. doi: 10.3233/jad-180847
Faulkner, P., Lucini Paioni, S., Kozhuharova, P., Orlov, N., Lythgoe, D. J., Daniju, Y., et al. (2020). Daily and intermittent smoking are associated with low prefrontal volume and low concentrations of prefrontal glutamate, creatine, myo-inositol, and N-acetylaspartate. Addict. Biol. 2020:e12986. doi: 10.1111/adb.12986
Fritz, H. C., Wittfeld, K., Schmidt, C. O., Domin, M., Grabe, H. J., Hegenscheid, K., et al. (2014). Current smoking and reduced gray matter volume-a voxel-based morphometry study. Neuropsychopharmacology 39, 2594–2600. doi: 10.1038/npp.2014.112
Gallinat, J., Meisenzahl, E., Jacobsen, L. K., Kalus, P., Bierbrauer, J., Kienast, T., et al. (2006). Smoking and structural brain deficits: a volumetric MR investigation. Eur. J. Neurosci. 24, 1744–1750. doi: 10.1111/j.1460-9568.2006.05050.x
Geula, C., Nagykery, N., Nicholas, A., and Wu, C. K. (2008). Cholinergic neuronal and axonal abnormalities are present early in aging and in Alzheimer disease. J. Neuropathol. Exp. Neurol. 67, 309–318. doi: 10.1097/NEN.0b013e31816a1df3
Gordon, B. A., Blazey, T., Su, Y., Fagan, A. M., Holtzman, D. M., Morris, J. C., et al. (2016). Longitudinal β-Amyloid Deposition and Hippocampal Volume in Preclinical Alzheimer Disease and Suspected Non-Alzheimer Disease Pathophysiology. JAMA Neurol. 73, 1192–1200. doi: 10.1001/jamaneurol.2016.2642
Gordon, B. A., Blazey, T. M., Su, Y., Hari-Raj, A., Dincer, A., Flores, S., et al. (2018). Spatial patterns of neuroimaging biomarker change in individuals from families with autosomal dominant Alzheimer’s disease: a longitudinal study. Lancet Neurol. 17, 241–250. doi: 10.1016/s1474-4422(18)30028-0
Gratwicke, J., Kahan, J., Zrinzo, L., Hariz, M., Limousin, P., Foltynie, T., et al. (2013). The nucleus basalis of Meynert: a new target for deep brain stimulation in dementia? Neurosci. Biobehav. Rev. 37(10 Pt 2), 2676–2688. doi: 10.1016/j.neubiorev.2013.09.003
Grothe, M., Heinsen, H., and Teipel, S. J. (2012). Atrophy of the cholinergic Basal forebrain over the adult age range and in early stages of Alzheimer’s disease. Biol. Psychiatry. 71, 805–813. doi: 10.1016/j.biopsych.2011.06.019
Hampel, H., Mesulam, M. M., Cuello, A. C., Farlow, M. R., Giacobini, E., Grossberg, G. T., et al. (2018). The cholinergic system in the pathophysiology and treatment of Alzheimer’s disease. Brain 141, 1917–1933. doi: 10.1093/brain/awy132
Hanseeuw, B. J., Betensky, R. A., Jacobs, H. I. L., Schultz, A. P., Sepulcre, J., Becker, J. A., et al. (2019). Association of Amyloid and Tau With Cognition in Preclinical Alzheimer Disease: A Longitudinal Study. JAMA Neurol. 76, 915–924. doi: 10.1001/jamaneurol.2019.1424
Ho, Y. S., Yang, X., Yeung, S. C., Chiu, K., Lau, C. F., Tsang, A. W., et al. (2012). Cigarette smoking accelerated brain aging and induced pre-Alzheimer-like neuropathology in rats. PLoS One 7:e36752. doi: 10.1371/journal.pone.0036752
Janes, A. C., Nickerson, L. D., Frederick Bde, B., and Kaufman, M. J. (2012). Prefrontal and limbic resting state brain network functional connectivity differs between nicotine-dependent smokers and non-smoking controls. Drug Alcohol Depend. 125, 252–259. doi: 10.1016/j.drugalcdep.2012.02.020
Jasinska, A. J., Stein, E. A., Kaiser, J., Naumer, M. J., and Yalachkov, Y. (2014). Factors modulating neural reactivity to drug cues in addiction: a survey of human neuroimaging studies. Neurosci. Biobehav. Rev. 38, 1–16. doi: 10.1016/j.neubiorev.2013.10.013
Johnson, A. L., Nystrom, N. C., Piper, M. E., Cook, J., Norton, D. L., Zuelsdorff, M., et al. (2021). Cigarette Smoking Status, Cigarette Exposure, and Duration of Abstinence Predicting Incident Dementia and Death: A Multistate Model Approach. J. Alzheimers Dis. 80, 1013–1023. doi: 10.3233/jad-201332
Kilimann, I., Grothe, M., Heinsen, H., Alho, E. J., Grinberg, L., Amaro, E. Jr., et al. (2014). Subregional basal forebrain atrophy in Alzheimer’s disease: a multicenter study. J. Alzheimers Dis. 40, 687–700. doi: 10.3233/jad-132345
Kivipelto, M., Mangialasche, F., and Ngandu, T. (2018). Lifestyle interventions to prevent cognitive impairment, dementia and Alzheimer disease. Nat. Rev. Neurol. 14, 653–666. doi: 10.1038/s41582-018-0070-3
Larrieu, S., Letenneur, L., Orgogozo, J. M., Fabrigoule, C., Amieva, H., Le Carret, N., et al. (2002). Incidence and outcome of mild cognitive impairment in a population-based prospective cohort. Neurology 59, 1594–1599. doi: 10.1212/01.wnl.0000034176.07159.f8
Li, H., Jia, X., Qi, Z., Fan, X., Ma, T., Ni, H., et al. (2017). Altered Functional Connectivity of the Basal Nucleus of Meynert in Mild Cognitive Impairment: A Resting-State fMRI Study. Front. Aging Neurosci. 9:127. doi: 10.3389/fnagi.2017.00127
Liao, W., Wu, G. R., Xu, Q., Ji, G. J., Zhang, Z., Zang, Y. F., et al. (2014). DynamicBC: a MATLAB toolbox for dynamic brain connectome analysis. Brain Connect. 4, 780–790. doi: 10.1089/brain.2014.0253
Liu, A. K., Chang, R. C., Pearce, R. K., and Gentleman, S. M. (2015). Nucleus basalis of Meynert revisited: anatomy, history and differential involvement in Alzheimer’s and Parkinson’s disease. Acta Neuropathol. 129, 527–540. doi: 10.1007/s00401-015-1392-5
Long, J. M., and Holtzman, D. M. (2019). Alzheimer Disease: An Update on Pathobiology and Treatment Strategies. Cell 179, 312–339. doi: 10.1016/j.cell.2019.09.001
Marvel, C. L., Morgan, O. P., and Kronemer, S. I. (2019). How the motor system integrates with working memory. Neurosci. Biobehav. Rev. 102, 184–194. doi: 10.1016/j.neubiorev.2019.04.017
Mehta, M., Adem, A., Kahlon, M. S., and Sabbagh, M. N. (2012). The nicotinic acetylcholine receptor: smoking and Alzheimer’s disease revisited. Front. Biosci. 4:169–180. doi: 10.2741/367
Mesulam, M., Shaw, P., Mash, D., and Weintraub, S. (2004). Cholinergic nucleus basalis tauopathy emerges early in the aging-MCI-AD continuum. Ann. Neurol. 55, 815–828. doi: 10.1002/ana.20100
Mesulam, M. M. (2013). Cholinergic circuitry of the human nucleus basalis and its fate in Alzheimer’s disease. J. Comp. Neurol. 521, 4124–4144. doi: 10.1002/cne.23415
Moreno-Gonzalez, I., Estrada, L. D., Sanchez-Mejias, E., and Soto, C. (2013). Smoking exacerbates amyloid pathology in a mouse model of Alzheimer’s disease. Nat. Commun. 4:1495. doi: 10.1038/ncomms2494
Passetti, F., Chudasama, Y., and Robbins, T. W. (2002). The frontal cortex of the rat and visual attentional performance: dissociable functions of distinct medial prefrontal subregions. Cereb Cortex 12, 1254–1268. doi: 10.1093/cercor/12.12.1254
Pievani, M., de Haan, W., Wu, T., Seeley, W. W., and Frisoni, G. B. (2011). Functional network disruption in the degenerative dementias. Lancet Neurol. 10, 829–843. doi: 10.1016/s1474-4422(11)70158-2
Prince, M., Wimo, A., Guerchet, M., Ali, G. C., and Prina, M. (2015). World Alzheimer Report 2015. The Global Impact of Dementia. An Analysis of Prevalence, Incidence, Cost and Trends. London: Alzheimer’s Disease International.
Raichle, M. E., MacLeod, A. M., Snyder, A. Z., Powers, W. J., Gusnard, D. A., and Shulman, G. L. (2001). A default mode of brain function. Proc. Natl. Acad. Sci. U S A 98, 676–682. doi: 10.1073/pnas.98.2.676
Ray, N. J., Metzler-Baddeley, C., Khondoker, M. R., Grothe, M. J., Teipel, S., Wright, P., et al. (2015). Cholinergic basal forebrain structure influences the reconfiguration of white matter connections to support residual memory in mild cognitive impairment. J. Neurosci. 35, 739–747. doi: 10.1523/jneurosci.3617-14.2015
Rusanen, M., Kivipelto, M., Quesenberry, C. P. Jr., Zhou, J., and Whitmer, R. A. (2011). Heavy smoking in midlife and long-term risk of Alzheimer disease and vascular dementia. Arch. Intern. Med. 171, 333–339. doi: 10.1001/archinternmed.2010.393
Shen, Z., Huang, P., Wang, C., Qian, W., Yang, Y., and Zhang, M. (2018). Cerebellar Gray Matter Reductions Associate With Decreased Functional Connectivity in Nicotine-Dependent Individuals. Nicotine Tob. Res. 20, 440–447. doi: 10.1093/ntr/ntx168
Staals, J., Makin, S. D., Doubal, F. N., Dennis, M. S., and Wardlaw, J. M. (2014). Stroke subtype, vascular risk factors, and total MRI brain small-vessel disease burden. Neurology 83, 1228–1234. doi: 10.1212/wnl.0000000000000837
Teipel, S., Grothe, M. J., and Alzheimer’s Disease Neuroimaging, I. (2016). Association Between Smoking and Cholinergic Basal Forebrain Volume in Healthy Aging and Prodromal and Dementia Stages of Alzheimer’s Disease. J. Alzheimers Dis. 52, 1443–1451. doi: 10.3233/JAD-151100
Weiland, B. J., Sabbineni, A., Calhoun, V. D., Welsh, R. C., and Hutchison, K. E. (2015). Reduced executive and default network functional connectivity in cigarette smokers. Hum. Brain Mapp. 36, 872–882. doi: 10.1002/hbm.22672
Whitehouse, P. J., Price, D. L., Clark, A. W., Coyle, J. T., and DeLong, M. R. (1981). Alzheimer disease: evidence for selective loss of cholinergic neurons in the nucleus basalis. Ann. Neurol. 10, 122–126. doi: 10.1002/ana.410100203
Whitehouse, P. J., Price, D. L., Struble, R. G., Clark, A. W., Coyle, J. T., and Delon, M. R. (1982). Alzheimer’s disease and senile dementia: loss of neurons in the basal forebrain. Science 215, 1237–1239. doi: 10.1126/science.7058341
Wilcox, C. E., Claus, E. D., Calhoun, V. D., Rachakonda, S., Littlewood, R. A., Mickey, J., et al. (2018). Default mode network deactivation to smoking cue relative to food cue predicts treatment outcome in nicotine use disorder. Addict. Biol. 23, 412–424. doi: 10.1111/adb.12498
Xu, W., Rao, J., Song, Y., Chen, S., Xue, C., Hu, G., et al. (2021). Altered Functional Connectivity of the Basal Nucleus of Meynert in Subjective Cognitive Impairment, Early Mild Cognitive Impairment, and Late Mild Cognitive Impairment. Front. Aging Neurosci. 13:671351. doi: 10.3389/fnagi.2021.671351
Yalachkov, Y., Kaiser, J., and Naumer, M. J. (2009). Brain regions related to tool use and action knowledge reflect nicotine dependence. J. Neurosci. 29, 4922–4929. doi: 10.1523/jneurosci.4891-08.2009
Yan, C. G., Wang, X. D., Zuo, X. N., and Zang, Y. F. (2016). DPABI: Data Processing & Analysis for (Resting-State) Brain Imaging. Neuroinformatics 14, 339–351. doi: 10.1007/s12021-016-9299-4
Zaborszky, L., Hoemke, L., Mohlberg, H., Schleicher, A., Amunts, K., and Zilles, K. (2008). Stereotaxic probabilistic maps of the magnocellular cell groups in human basal forebrain. Neuroimage 42, 1127–1141. doi: 10.1016/j.neuroimage.2008.05.055
Zhang, S., Hu, S., Fucito, L. M., Luo, X., Mazure, C. M., Zaborszky, L., et al. (2017). Resting-State Functional Connectivity of the Basal Nucleus of Meynert in Cigarette Smokers: Dependence Level and Gender Differences. Nicotine Tob. Res. 19, 452–459. doi: 10.1093/ntr/ntw209
Zhang, X. Y., Tan, Y. L., Chen, D. C., Tan, S. P., Yang, F. D., Zunta-Soares, G. B., et al. (2016). Effects of cigarette smoking and alcohol use on neurocognition and BDNF levels in a Chinese population. Psychopharmacology 233, 435–445. doi: 10.1007/s00213-015-4124-6
Keywords: mild cognitive impairment, smoking, cholinergic dysfunction, nucleus basalis of Meynert, resting-state functional connectivity
Citation: Qiu T, Zeng Q, Luo X, Xu T, Shen Z, Xu X, Wang C, Li K, Huang P, Li X, Xie F, Dai S and Zhang M (2021) Effects of Cigarette Smoking on Resting-State Functional Connectivity of the Nucleus Basalis of Meynert in Mild Cognitive Impairment. Front. Aging Neurosci. 13:755630. doi: 10.3389/fnagi.2021.755630
Received: 09 August 2021; Accepted: 13 October 2021;
Published: 18 November 2021.
Edited by:
Sara Palermo, Carlo Besta Neurological Institute (IRCCS), ItalyReviewed by:
Dahua Yu, Inner Mongolia University of Science and Technology, ChinaCopyright © 2021 Qiu, Zeng, Luo, Xu, Shen, Xu, Wang, Li, Huang, Li, Xie, Dai and Zhang. This is an open-access article distributed under the terms of the Creative Commons Attribution License (CC BY). The use, distribution or reproduction in other forums is permitted, provided the original author(s) and the copyright owner(s) are credited and that the original publication in this journal is cited, in accordance with accepted academic practice. No use, distribution or reproduction is permitted which does not comply with these terms.
*Correspondence: Fei Xie, YTI1Njk4ODlAMTI2LmNvbQ==; Shouping Dai, ZGFpc2hvdXBpbmdAc2luYS5jbg==; Minming Zhang, emhhbmdtaW5taW5nQHpqdS5lZHUuY24=
†These authors have contributed equally to this work and share first authorship
‡These authors have contributed equally to this work and share senior authorship
$Data used in preparation of this article were obtained from the Alzheimer’s Disease Neuroimaging Initiative (ADNI) database (adni.loni.usc.edu). As such, the investigators within the ADNI contributed to the design and implementation of ADNI and/or provided data but did not participate in analysis or writing of this report. A complete listing of ADNI investigators can be found at: http://adni.loni.usc.edu/wp-content/uploads/how_to_apply/ADNI_Acknowledgement_List.pdf
Disclaimer: All claims expressed in this article are solely those of the authors and do not necessarily represent those of their affiliated organizations, or those of the publisher, the editors and the reviewers. Any product that may be evaluated in this article or claim that may be made by its manufacturer is not guaranteed or endorsed by the publisher.
Research integrity at Frontiers
Learn more about the work of our research integrity team to safeguard the quality of each article we publish.