- 1Graduate Institute of Clinical Medical Science, China Medical University, Taichung, Taiwan
- 2Division of Parkinson's Disease and Movement Disorders, Department of Neurology, China Medical University Hospital, Taichung, Taiwan
- 3Neuroscience Laboratory, Department of Neurology, China Medical University Hospital, Taichung, Taiwan
- 4School of Medicine, College of Medicine, China Medical University, Taichung, Taiwan
- 5Graduate Institute of Biomedical Sciences, China Medical University, Taichung, Taiwan
- 6Department of Medical Imaging, China Medical University Hospital, Taichung, Taiwan
- 7Neuroscience and Brain Disease Center, College of Medicine, China Medical University, Taichung, Taiwan
- 8Department of Medical Research, China Medical University Hospital, Taichung, Taiwan
- 9Department of Neurology, China Medical University Hsinchu Hospital, Hsinchu, Taiwan
- 10Department of Health and Nutrition Biotechnology, Asia University, Taichung, Taiwan
- 11Department of Physical Therapy, China Medical University, Taichung, Taiwan
- 12Institute of Education, National Yang Ming Chiao Tung University, Hsinchu, Taiwan
- 13Institute for Neural Computation, University of California, San Diego, La Jolla, CA, United States
Background: Freezing of gait (FOG) in Parkinson's disease (PD) is a devastating clinical phenomenon that has a detrimental impact on patients. It tends to be triggered more often during turning (complex) than during forwarding straight (simple) walking. The neural mechanism underlying this phenomenon remains unclear and requires further elucidation.
Objective: To investigate the differences in cerebral functional magnetic resonance imaging responses between PD patients with and without FOG during explicitly video-guided motor imagery (MI) of various complex (normal, freezing) and simple (normal, freezing) walking conditions.
Methods: We recruited 34 PD patients, namely, 20 with FOG and 14 without FOG, and 15 normal controls. Participants underwent video-guided MI of turning and straight walking, with and without freezing, while their brain blood oxygen level-dependent (BOLD) activities were measured. Gait analysis was performed.
Results: While comparing FOG turning with FOG straight walking, freezers showed higher activation of the superior occipital gyrus, left precentral gyrus, and right postcentral gyrus compared with non-freezers. Normal controls also manifest similar findings compared with non-freezers, except no difference was noted in occipital gyrus activity between the two groups. Freezers also displayed a higher effect size in the locomotor regions than non-freezers during imagery of normal turning.
Conclusions: Our findings suggest that freezers require a higher drive of cortical and locomotion regions to overcome the overinhibition of the pathways in freezers than in non-freezers. Compared with simple walking, increased dorsal visual pathway and deep locomotion region activities might play pivotal roles in tackling FOG in freezers during complex walking.
Introduction
Freezing of gait (FOG) is manifested with “brief, episodic absence of, or marked reduction of forward progression of the feet despite the intention to walk” (Nutt et al., 2011). It has been identified to be a major cause of falls in patients with Parkinson's disease (PD) (Nonnekes et al., 2015). Gait can be either simple (i.e., forward straight walking) or complex (i.e., turning), and FOG tended to be elicited more during complex walking situations (Rahman et al., 2008a; Spildooren et al., 2010; Shine et al., 2012; Smulders et al., 2016; Zhang et al., 2016). During turning, the left and right legs step asymmetrically, and the center of mass temporarily shifts laterally, causing body instability (Bengevoord et al., 2016). Therefore, turning, which requires more complex neural control than straight walking, is often more likely to cause freezing in PD patients with FOG (PDFOG). From the pharmacological perspective, the fact that dopaminergic treatment might benefit straight walking more than turning in PD patients (Smulders et al., 2016) suggests different pathophysiologies underlying the two ambulatory situations. Although different gait kinematics in PDFOG during turning have been postulated (Bertoli et al., 2019; Mitchell et al., 2019), the neurophysiological evidence at the cerebral level for FOG in these various scenarios remains pending and requires further investigation.
The current findings of the higher neural level (cerebellum, basal ganglia, locomotion regions, and cortex) activities of FOG mainly arise from the resting-state functional magnetic resonance imaging (fMRI) studies (Nutt, 2013; Gilat et al., 2015; Wang et al., 2016; Bharti et al., 2019; Potvin-Desrochers et al., 2019). However, FOG is a dynamic disorder that tended to be developed during motion. In this regard, it is difficult to reflect the neural activities by resting fMRI. To overcome such obstacle, the motor imagery (MI) method would be suitable for investigating the conditions relevant to FOG. MI has been defined as the conscious mental simulation of actions involving our brain's motor representations in a way that is similar to when we perform actual movements (Jeannerod and Decety, 1995). It can usually be achieved by purely imagining the motion or by a combo of “explicit guidance plus imagery” (Szameitat et al., 2007; La Fougere et al., 2010; Hetu et al., 2013; Duann and Chiou, 2016). MI activates the brain's mirror neurons and motor-related brain areas, including the frontal–parietal network, subcortical, cerebellar, and primary motor cortex similar to motor execution (Szameitat et al., 2007; La Fougere et al., 2010; Hetu et al., 2013; Duann and Chiou, 2016). By MI and the region of interest (ROI) in fMRI, Snijders et al. revealed the elevated activation in the mesencephalic locomotion region (MLR) in PDFOG, compared with PD patients without FOG (PDNOFOG) in simple gait condition (Snijders et al., 2011). With similar techniques, Peterson et al. (2014) found that neural activities in the supplementary motor area (SMA) and striatum were increased in PDNOFOG during MI of simple walking (Peterson et al., 2014). The aforementioned illustrated the feasibility of adopting fMRI and MI for the investigation of FOG in PD. These studies are intriguing, but two issues remain to be elucidated. First, no normal controls were used for comparison with the patients, and it remains uncertain whether the results were disease-specific. Second, the MI paradigms adopted only pure imagery with no explicit guidance. As a result, it was difficult to verify how the participants could be imagining the gait conditions with and without freezing during the experimental process.
In this current study, we adopted a novel fMRI design of MI to study FOG using the first-person perspective video clips (Iseki et al., 2008; Wang et al., 2009) of multiple gait conditions, including forward straight walking and 360° turning, with and without FOG. We aimed to investigate (A) the cerebral blood oxygen level-dependent (BOLD) responses to different gait conditions among PDFOG, PDNOFOG, and normal controls, and (B) the patterns of cerebral BOLD activation between simple (forward straight) and complex (turning) walking. We combined the activation analysis and ROI analyses of the three locomotion regions [MLR, cerebellar locomotion region (CLR), and subthalamic locomotion region (SLR)] to analyze the fMRI data to gain sensitivity and preserve the statistical power of data analysis.
Materials and Methods
Participants
Thirty-seven PD patients aged between 50 and 80 years were enrolled in this study (58.8% male; mean age 67.6 ± 7.1 years). All participants fulfilled the UK Brain Bank Criteria for idiopathic PD (Gibb et al., 1990). Among the participants, 22 PD patients who obtained 1 point from Part I of the New FOG Questionnaire (nFOG-Q) (Nieuwboer et al., 2009) and experienced at least one FOG episode during gait assessment (see clinical and gait assessment) were labeled PDFOG, whereas the other 15 PD patients who had never experienced a FOG attack were grouped as PDNOFOG. Fifteen age- and education-level-matched normal controls were also recruited (33.3% male; mean age 63.4 ± 7.0 years; see Table 1). To confirm the MI ability during fMRI of each participant, the Vividness of Motor Imagery Questionnaire (VMIQ) (Isaac et al., 1986) was administered before recruitment. According to the previous report (Snijders et al., 2011), poor MI ability was defined as VMIQ scores >200, and no participants were excluded from this study due to VMIQ scores >200. Each PD patient was then investigated during the “off” state by withholding his/her medications overnight for over 12 h. Informed consent was obtained before the investigation. The study was approved by the Local Ethics Committee of the hospital in accordance with the Declaration of Helsinki (CMUH106-REC2-171) and was registered on ClinicalTrials.gov, with the identifier number NCT03127475.
Clinical and Gait Assessment
The clinical assessments used are as follows: the Unified Parkinson's Disease Rating Scale (UPDRS) (Gibb and Lees, 1988), the UPDRS motor subsection (UPDRS-III), Hoehn–Yahr (H&Y) staging, levodopa equivalent daily dose (LEDD) (Tomlinson et al., 2010), Parkinson's Disease Questionnaire (PDQ-39) (Rahman et al., 2008b), nFOG-Q (Nieuwboer et al., 2009), and the Mini-Mental State Examination (MMSE) (Folstein et al., 1975).
For the gait assessment, the participants were instructed to walk back and forth on a 5-m walkway for five laps (50 m in total) in the gait analysis laboratory equipped with a Zeno 16 × 2 feet pressure-sensitive carpet. Concomitant video clips were also recorded throughout the entire gait assessment. Freezing episodes were identified by two independent, experienced neurologists from the video clips. The gait parameters were then analyzed by Protokinetics Movement Analysis System 5.08 (Peekskill, NY, United States). These parameters included velocity (cm/s), cadence (steps/min), stride length (cm) (Chaudhuri et al., 2007), stride width (cm) (Chaudhuri et al., 2007), gait cycle (Cugusi et al., 2017), stance time (% of gait cycle), swing time (% of gait cycle), single support time (% of gait cycle), and total double support time (% of gait cycle). We also analyzed the correlation between the nFOG-Q score of PDFOG and gait parameters using Spearman's rank-order correlation coefficient test. The threshold for statistical significance was set at bivariate p < 0.05.
Functional Magnetic Resonance Imaging Experiment
Paradigm of MI
For the MI fMRI experiment, two fMRI sessions were conducted with a blocked experimental design. Each session comprised six pseudorandomized blocks of video clips of different gait conditions, including three types of walking (straight walking, 360° clockwise turning, and 360° counter-clockwise turning) with and without FOG (Figure 1). As a result, each participant watched the video clips of six gait stimulus conditions (normal straight walking, normal clockwise turning, normal counter-clockwise turning, FOG straight walking, FOG clockwise turning, and FOG counter-clockwise turning). The video clips recorded the same actor, viewing from the top and watching his ambulating feet in a first-person perspective, walking in six conditions. The velocities of normal straight walking and normal turning were 100 cm/s and 25°/s, respectively. The FOG condition was a video clip starting with normal walking or turning for 4 s, followed by FOG walking or turning (70% freezing in each FOG condition; Figure 1B). Each gait stimulus video clip lasted for 14–16 s. In between the consecutive gait blocks, a 5-s “standing” video clip was used as a baseline condition for further contrasting different gait conditions. The total duration of each functional session was 425 s, with counterbalanced order for the six gait conditions across participants.
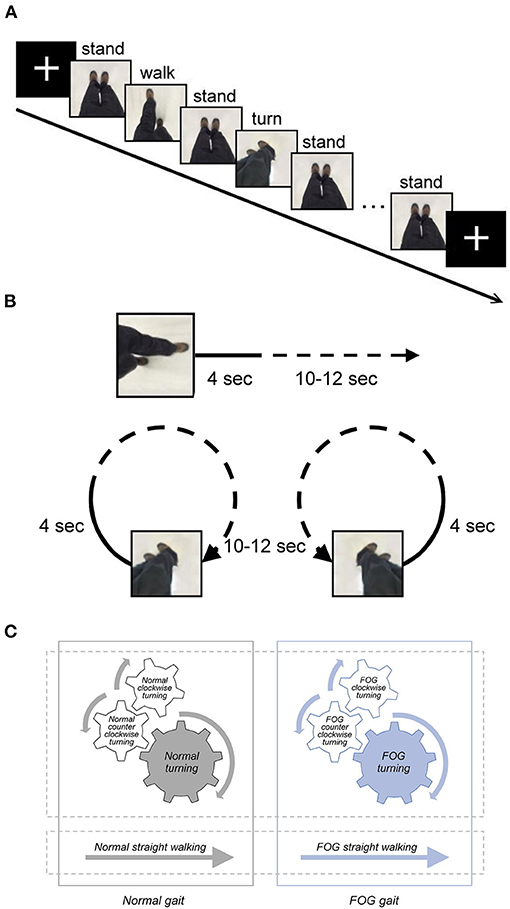
Figure 1. (A) Graphical representation of the fMRI paradigm, including snapshots from the gait video clips to represent the stimulus. The duration of the stimuli, including normal straight walking, FOG straight walking (not shown in this figure), normal clockwise turning (not shown), normal counter-clockwise turning, FOG clockwise turning (not shown), and FOG counter-clockwise turning (not shown), varied between 14 and 16 s. The duration of the fixed cross and standing stimuli was 5 s. (B) Graphical representation of the video of FOG condition. The video clip started with normal walking or turning for 4 sec, followed by 10–12 s of FOG walking or turning (70 % freezing in each FOG condition). (C) Diagram illustrating the content relationship among various gait conditions in the study. Normal clockwise turning combined with normal counter-clockwise turning together as normal turning; FOG clockwise turning combined with FOG counter-clockwise turning as FOG turning. All FOG-related conditions, including FOG straight walking and FOG turning, were jointly referred to as the FOG gait condition, and all the normal conditions were jointly referred to as normal gait.
Image Acquisition
The fMRI/MRI data were collected using a 3.0 T MR system (Signa HDxt, GE, Milwaukee, WI, United States). The video clips of different gait conditions were projected on the screen installed at the rear side of the MR scanner. Each participant watched the video through the reflective mirror mounted on the head coil and mentally imagined themselves performing the gait action currently played during the functional experiment. T2*-weighted echo planar functional images were acquired in an interleaved slice order. Other imaging parameters included were as follows: TR = 2 s, TE = 35 ms, flip angle = 90°, 32 axial slices covering the whole brain, field of view = 224 × 224 mm2, matrix = 64 × 64, and slice thickness = 4 mm with 0.4 mm inter-slice gap. This resulted in an effective image resolution of 3.5 × 3.5 × 4.4 mm in voxel size. High-resolution 3D T1-weighted anatomical images (voxel size 1 × 1 × 1 mm) were obtained using a three-dimensional inversion recovery prepped spoiled gradient recalled echo pulse sequence for co-registering the functional data to the individual structure and finally the standard brain template.
Data Analysis of fMRI
Pre-processing and activation analysis of the fMRI data were performed using Statistical Parametric Mapping 8 (SPM8) software (the Wellcome Trust Centre for Neuroimaging, London, United Kingdom) running under MATLAB R2014b (MathWorks, Inc., Boston, MA United States). The pre-processing steps included slice timing correction, realignment, co-registration, spatial normalization, and spatial smoothing. Among these pre-processing steps, Montreal Neurological Institute coordinate space was used in the spatial normalization, and a Gaussian filter with an 8-mm full-width at half-maximum was used in the spatial smoothing (Evans et al., 1991, http://www.bic.mni.mcgill.ca).
General Linear Model
In this study, we used general linear model (GLM) (Friston et al., 1994), which includes linear multiple regression, to find the voxels with their BOLD time courses highly in line with the task-related reference function, with other nuisance factor, such as the six degree-of-freedom motion parameters, etc., controlled in the GLM. This is so-called the activation analysis at the single-subject level for the active cerebral regions under the contrast of different gait conditions. Then, directional t-test (also called two-sample t-test) for comparing each set of two groups (PDFOG vs. controls, PDNOFOG vs. controls, and PDFOG vs. PDNOFOG) were performed during group analysis. This statistical comparison procedure also highly complied with what had been reported in the literature (Poline and Brett, 2012). The GLM contains forms of experimental layout and ensuing statistical analysis including linear regression and ANOVA. This approach connects the general linear model and the theory of Gaussian fields to give a simple and complete framework for the analysis of imaging data. In this study, GLMs (Friston et al., 1994, 1995) were estimated with a high-pass filter of 128 s.
Statistical Analysis
Activation Analysis at the Single-Subject Level
The pre-processed fMRI time-series data were analyzed on a subject-by-subject basis using a blocked design approach in the context of the GLM to find the cerebral regions responding to the contrasts of different gait conditions. The contrasts of normal straight walking > standing (baseline), FOG straight walking > standing, normal clockwise turning > standing, normal counter-clockwise turning > standing, FOG clockwise turning > standing, and FOG counter-clockwise turning > standing were used in the statistical analysis. Directional T-contrasts for comparison of each group were estimated. The threshold value for statistical significance was set to p < 0.05 after multiple-comparison correction by false discovery rate (FDR) at the voxel level (Genovese et al., 2002).
To ensure that the participants were fully engaged in the MI task, we have carefully reviewed the activation of the GLM analysis at the single-subject level. Further, those participants who showed no activation in the motion-sensitive visual areas (e.g., the MT/V5 areas, Figure 2A) (Kaas et al., 2010; Kolster et al., 2010), but pronounced default mode network activation (Figure 2B), indicating that they might not actively watch the video clips in the scanner, were further excluded from the latter group analysis. Such a criterion excluded two PDFOG patients and one PDNOFOG patient from further analysis.
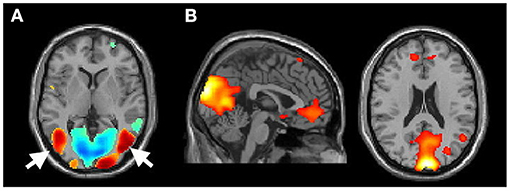
Figure 2. (A) Activation of the MT/V5 area (arrow) of participant 11 (P11) while performing the motor imagery task during fMRI and (B) activation of the default mode (from the excluded P3).
Group Analysis
Given that the activation analysis at the single-subject level did not differ between clockwise and counter-clockwise turning, we opted to combine the conditions of clockwise and counter-clockwise turning to form the conditions of normal turning and FOG turning for the group analysis to increase the data trial number and improve the statistical power. Further, all FOG-related conditions, such as the FOG straight walking and FOG turning, were combined into the FOG gait condition, and all normal conditions were also combined as normal gait (Figure 1C).
For each participant, six contrasts (normal straight walking, FOG straight walking, normal turning, FOG turning, normal gait, and FOG gait) were conducted. Furthermore, to examine the different cerebral activation patterns between simple gait and complex gait, additional two contrasts, namely, normal turning > normal straight walking and FOG turning > FOG straight walking, were also conducted. Directional T-contrasts for comparing each set of two groups (PDFOG vs. controls, PDNOFOG vs. controls, and PDFOG vs. PDNOFOG) were performed. Given that the H&Y staging was higher in PDFOG than in PDNOFOG, the resulting contrasts from the two PD groups (PDFOG vs. PDNOFOG) were further statistically compared against the H&Y staging as a covariate during group analysis to minimize the interference of the fMRI result by PD severity. The threshold value for statistical significance was set to p < 0.05 after correction by FDR at the voxel level. Furthermore, as the differences between each set of two groups when compared contrasts with normal turning > normal straight walking and FOG turning > FOG straight walking were too small when statistical significance was set to p < 0.05 after multiple-comparison correction by FDR at the voxel level, a statistically significant threshold of p < 0.001 (without multiple-comparison correction) was used when comparing these two contrasts.
Analysis of ROI
In addition to the activation analysis mentioned above, we have further compared the BOLD activation of the three locomotion regions, namely, the MLR, CLR, and SLR, to investigate the role of these three areas in PDFOG, PDNOFOG, and normal controls in different gait conditions. Due to their small sizes, these three brain areas failed to show a significant activation in the regular activation analysis. As a result, we delineated these three brain areas based on the coordinates as reported in the previous literature, that is (x = ±7, y = −52, z = −16), for the CLR; (x = ±4, y = −30, z = −16), for the MLR; and (x = ±11, y = −14, z = −3) for the SLR, bilaterally (Snijders et al., 2011; Fling et al., 2014). A 4-mm radius for the MLR and an 8-mm radius for the CLR and the SLR surrounding the designated coordinates were used to extract the effect sizes (beta values) of the three ROIs using the MarsBaR toolbox in SPM8.
The mean effect sizes (beta values) were then extracted and averaged from the designated ROIs and then imported into SPSS v.19 (SPSS, Inc., Chicago, IL, United States) for the group-level analysis. One-way ANOVA was performed to determine whether each of the ROIs was significantly associated with the contrast of normal straight walking, FOG straight walking, normal turning, FOG turning, normal gait, and FOG gait. Furthermore, the effect size from each ROI of the PDFOG was compared against the nFOG-Q score, whereas the effect size from each ROI of the PD population (PDFOG and PDNOFOG, respectively), was compared against the H&Y staging, PD disease duration, and gait parameters from the gait assessment using bivariate Spearman's rank-order correlations. The threshold for statistical significance was set at p < 0.05.
Results
Results of Clinical and Gait Assessments
Table 1 shows the demographic characteristics of the participants. There were no significant differences in age, gender, number of years of education, VMIQ, and MMSE among PDFOG, PDNOFOG, and normal controls. There were also no significant differences for UPDRS, UPDRS-III, LEDD, and disease duration between PDFOG and PDNOFOG. Moreover, no significant differences were noted in the motor symptom lateralization in PDFOG (seven left-sided and seven right-sided predominating) and PDNOFOG (13 left-sided and seven right-sided predominating, with no significant difference in the summation of UPDRS motor score on each side; left-sided, 6.9 ± 4.8; right-sided, 6.3 ± 4.6; p = 0.991). However, PDFOG showed more severe H&Y staging and PDQ-39 than PDNOFOG.
Significant differences were observed in the velocity, stride length, stance time, swing time, single support time, and total double support time among PDFOG, PDNOFOG, and normal controls (Table 1). PDFOG had the slowest gait, smallest stride length, increased stance time, decreased swing time, least single support time, and increased total double support time. Furthermore, the nFOG-Q score in PDFOG was significantly positively correlated with stance time (ρ = 0.492, p = 0.032) and total double support time (ρ = 0.506, p = 0.023), whereas it was significantly negatively correlated with velocity (ρ = −0.505, p = 0.023), swing time (ρ = −0.492, p = 0.032), and single support time (ρ = −0.45, p = 0.047). No significant correlations were found between the nFOG-Q score and cadence (ρ = −0.358, p = 0.121), stride length (ρ = −0.374, p = 0.105), stride width (ρ = 0.069, p = 0.774), and gait cycle (ρ = 0.345, p = 0.136).
Imaging Results
Activation Analysis
Different Patterns of Cerebral Responses of Normal Gait and FOG Gait Conditions Among PDFOG, PDNOFOG, and Normal Controls
Normal Gait. The results comparing the BOLD responses of normal gait among PDFOG, PDNOFOG, and normal controls have revealed significantly higher activation in the bilateral SMA, right superior temporal, and right medial superior frontal gyrus in normal controls than PDNOFOG (Table 2 and Figure 3A). Moreover, a significant activation was observed in the right SMA in normal controls compared with PDNOFOG during the normal straight walking (Table 2). No significant difference was detected in the brain activations associated with normal turning among the three groups.
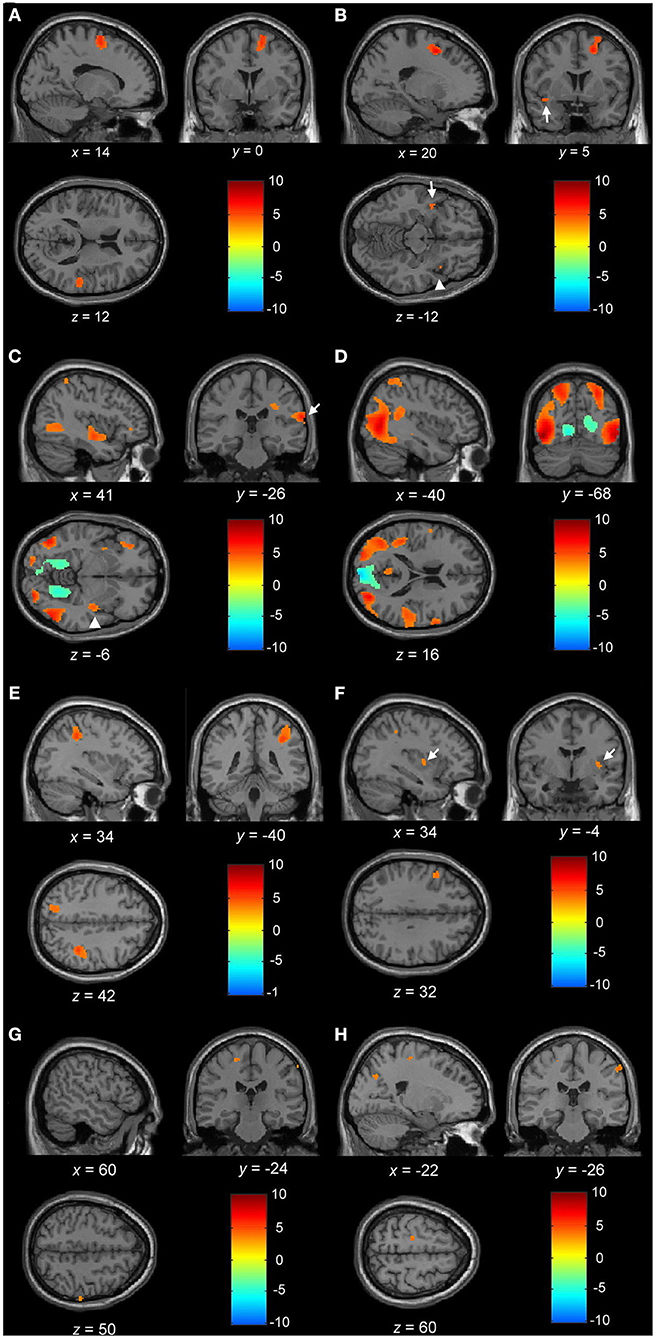
Figure 3. (A) Blood oxygen level-dependent (BOLD) activation during motor imagery (MI) of normal gait with the contrast of normal controls to PDNOFOG. Increased BOLD in the right SMA, right superior temporal gyrus, left SMA (not shown), and right medial superior frontal gyrus (not shown). (B) BOLD activation during MI of FOG gait with the contrast of PDFOG to PDNOFOG. Increased BOLD in the right superior and middle frontal gyrus, right insula (arrowhead), left superior temporal cortex (arrow), and left superior frontal gyrus (not shown). (C) BOLD activation during MI of FOG straight walking in PDFOG. Increased BOLD in the right insula (arrowhead), right postcentral gyrus (arrow), right middle temporal, left middle occipital, and right inferior occipital lobule. (D) BOLD activation during MI of FOG turning in PDFOG. Increased BOLD in the right precentral gyrus, right middle temporal, left superior temporal, bilateral superior parietal, left middle and inferior occipital, and right superior occipital lobule. (E) BOLD activation during MI of FOG turning > FOG straight walking in PDFOG. Increased BOLD in the right superior and inferior parietal, left superior parietal lobule, and right middle occipital lobule (not shown). (F) BOLD activation during MI of normal turning > normal straight walking in PDFOG > controls. Increased BOLD in the left inferior frontal, right putamen (arrow), and right inferior parietal lobule. (G) BOLD activation during MI of FOG turning > FOG straight walking in control > PDNOFOG. Increased BOLD in the left precentral and right postcentral gyrus. (H) BOLD activation during MI of FOG turning > FOG straight walking in PDFOG > PDNOFOG. Increased BOLD in the left precentral, right postcentral gyrus, and left superior occipital lobule. (A–E) Statistical maps were created and corrected for the false discovery rate (FDR) at p < 0.05 at the voxel level. (F–H) Statistical maps were created at p < 0.001 (without multiple-comparison correction).
FOG Gait. During the FOG gait, a significant activation occurred in the bilateral superior frontal, right middle frontal, right insula, and left superior temporal gyrus of PDFOG compared with PDNOFOG (Table 2 and Figure 3B). No significant difference was found in the brain activation associated with FOG turning and FOG straight walking among the three groups.
By only considering the PDFOG patient data, the increased cerebral activation of the right insula, right postcentral gyrus, right middle temporal, left middle occipital, and right inferior occipital lobule was detected in FOG straight walking (Table 2 and Figure 3C). The increased cerebral activation of the right precentral gyrus, right middle temporal, left superior temporal, bilateral superior parietal, left middle and inferior occipital, and right superior occipital was detected in FOG turning (Table 2 and Figure 3D). By subtracting FOG straight walking cerebral activation from that of FOG turning, the right superior and inferior parietal, left superior parietal lobule, and right middle occipital activation were retained (Table 2 and Figure 3E).
Analysis of ROI of the Locomotion Regions
Different Patterns of Locomotion Region Responses Between Normal Gait and FOG Gait Conditions Among PDFOG, PDNOFOG, and Normal Controls
Increased Beta Value of Locomotion Regions During Normal Turning but Not FOG turning in PDFOG. Analysis of ROI of the CLR activity against different gait conditions among PDFOG, PDNOFOG, and normal controls revealed a significantly higher beta value of CLR in PDFOG than in controls and PDNOFOG during the normal turning conditions (p = 0.003; PDFOG vs. controls, p = 0.007; PDFOG vs. with PDNOFOG, p = 0.015; all FDR-corrected, Figure 4A). No significant difference was noted during FOG turning (p = 0.394).
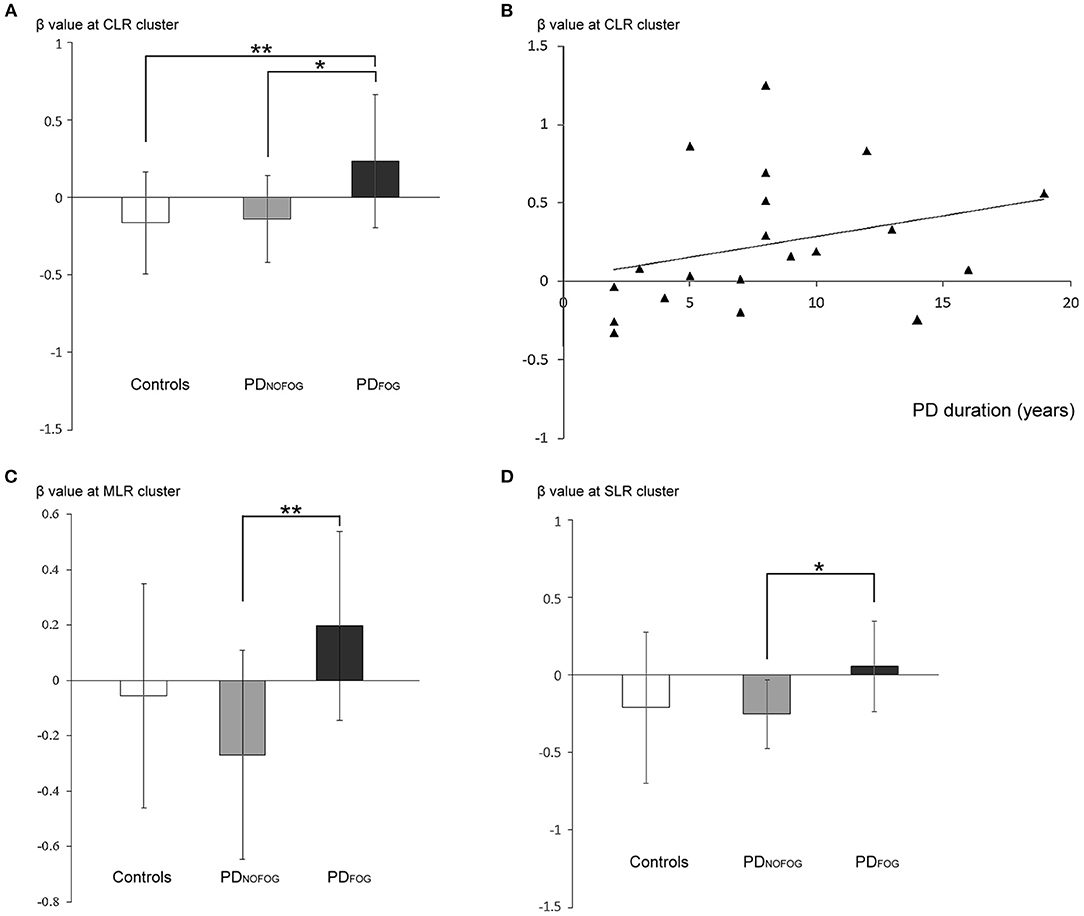
Figure 4. (A) β-weights of the contrast between normal turning and standing (mean ± standard deviation) from the cerebellar locomotor region (CLR) clusters in normal controls, PDNOFOG, and PDFOG. (B) Scatterplots of β-weights at the CLR cluster (y-axis) against PD duration (x-axis; Spearman's correlation ρ = 0.455, R2 = 0.088, p = 0.044) during normal turning in PDFOG. (C) β-weights of the contrast between normal turning and standing (mean ± standard deviation) from the mesencephalic locomotor region (MLR) clusters in normal controls, PDNOFOG, and PDFOG. (D) β-weights of the contrast between normal turning and standing (mean ± standard deviation) from the subthalamic locomotor region (SLR) clusters in normal controls, PDNOFOG, and PDFOG. *p < 0.05, **p < 0.01.
The ROI analysis of the MLR activity against different gait conditions among PDFOG, PDNOFOG, and normal controls revealed a significantly higher beta value of MLR in PDFOG compared with PDNOFOG during the normal turning conditions (p = 0.003, FDR-corrected, Figure 4C). No significant difference was detected during FOG turning (p = 0.14).
The ROI analysis of the SLR activity against different gait conditions among PDFOG, PDNOFOG, and normal controls revealed a significantly higher beta value of SLR in PDFOG than in PDNOFOG during the normal turning conditions (p = 0.023, FDR-corrected, Figure 4D). No significant difference was detected during FOG turning (p = 0.084).
Complex (Turning) vs. Simple Gait (Forward Straight Walking) in Normal Gait and FOG Gait Among PDFOG, PDNOFOG, and Normal Controls
Results of the Activation Analysis
When comparing complex to simple gait via conducting contrast of normal turning > normal straight walking and FOG turning > FOG straight walking among PDFOG, PDNOFOG, and normal controls, no significant difference was noted between each group using the statistical significance threshold of p < 0.05 (FDR-corrected). Therefore, we lowered the statistical significance to p < 0.001 (without multiple-comparison correction) and showed the subtle differences among the three groups. While comparing normal turning > normal straight walking among groups, PDFOG showed an increased activation of the left inferior frontal, right putamen, and right inferior parietal lobule when compared with controls (Table 2 and Figure 3F).
Furthermore, while comparing FOG turning > FOG straight walking among the three groups, controls showed an increased activation of left precentral and right postcentral gyrus compared with PDNOFOG (Table 2 and Figure 3G). In addition, PDFOG showed an increased activation of the left superior occipital gyrus, left precentral gyrus, and right postcentral gyrus when compared with PDNOFOG (Table 2 and Figure 3H).
Results of ROI
No significant difference in the beta values from ROI analysis of the MLR, CLR, or SLR activity of normal turning > normal straight walking and FOG turning > FOG straight walking was observed within each individual group (normal turning > normal straight walking: CLR, p = 0.07; MLR, p = 0.16; SLR, p = 0.92. FOG turning > FOG straight walking: CLR, p = 0.26; MLR, p = 0.11; SLR, p = 0.74).
Relationships Between the Beta Values of Locomotion Regions and Disease Severity
The beta values of CLR activity during normal turning in PDFOG were significantly positively correlated with PD disease duration (Figure 4B; p = 0.044). Moreover, no correlation was found between the beta values of the CLR activity and disease severity (UPDRS, p = 0.705; UPDRS-III, p = 0.84; H&Y, p = 0.647; and nFOG-Q, p = 0.845). Furthermore, no correlation was found between the beta values of the MLR or SLR activity and disease severity, and no significant correlation was detected between the beta values of the CLR, MLR, or SLR and gait parameters either.
Discussion
This study used MI of different gait conditions to investigate neural substrates of FOG. The “first-person-perspective” video, including normal/FOG straight walking and normal/FOG turning, was used to explicitly guide the MI. In addition, we have also measured the basic gait performance and clinical features of all subjects. We found that during imagery of normal gait, the PDNOFOG had a significantly reduced BOLD response in the bilateral SMA, right superior temporal, and right medial superior frontal gyrus relative to the controls. During MI of FOG gait, the greater BOLD response was found in the bilateral frontal lobe, left superior temporal lobe, and right insula of the PDFOG than in those of the PDNOFOG. Furthermore, PDFOG manifested a higher beta value in the CLR than in controls and PDNOFOG and a higher beta value in the MLR and SLR than in PDNOFOG during imagery of normal turning. The cerebral activity during complex gait was more activated than simple gait in PDFOG.
Less Gait-Related Cerebral Activation in PDNOFOG Than in PDFOG and Normal Controls
Overt cerebral deactivation during the MI of different gait conditions was observed in PDNOFOG. In particular, the activity of bilateral SMA and right frontal and right superior temporal lobules in PDNOFOG decreased compared with normal controls during normal gait MI. These findings are consistent with the results of an action observation fMRI study of normal walking in PD (Bommarito et al., 2020). From the physiological point of view, the SMA and premotor cortex have a tight connection to the spinal cord and brainstem reticular formation, including the pedunculopontine nucleus (PPN) area and MLR (Aravamuthan et al., 2009). Thus, the SMA has been identified to be crucial for gait initiation and postural control (Jacobs et al., 2009). Moreover, changes in body schema information originating from the temporoparietal cortex to the frontal cortex might promote motor programming in the SMA and premotor cortex to improve postural control and anticipatory postural adjustment during bipedal locomotion (Takakusaki, 2013). Furthermore, the basal ganglia control the locomotion and posture through the GABAergic pathway from the substantia nigra reticulata that blocks the PPN-induced muscle atonia and the MLR-induced locomotion (Takakusaki et al., 2003). However, the overinhibition from the basal ganglia in PD patients might cause them to experience gait disturbances, accounting for the perturbation of SMA, and frontal and temporal activities in PDNOFOG (Jenkins et al., 1992).
Different Freezing-Related Cerebral Activities Between PDFOG and PDNOFOG
During MI of the FOG gait, a significant activation occurred in the bilateral superior frontal, right middle frontal, right insula, and left superior temporal gyrus of PDFOG compared with PDNOFOG (Table 2 and Figure 3B). The increased activity in the frontal lobe and insula was in line with the results of the study carried out by Shine et al. (2013) and might be associated with the increased demand for cognitive gait control in PDFOG to overcome freezing while autonomic gait control was being blocked. Frontal executive dysfunction has been associated with freezing in PDFOG because FOG is more likely to occur during dual tasking (Spildooren et al., 2010) and PDFOG patients show a poor performance in set-shifting tasks (Rahman et al., 2008a; Naismith et al., 2010; Mckay et al., 2018). A functional near-infrared spectroscopy (fNIRS)-based study confirmed the increased activation of the frontal lobe before and during anticipated turns in PDFOG (Maidan et al., 2015). This result is similar to the increased freezing-related frontal activity observed in PDFOG. Furthermore, during the freezing attack in PDFOG, the increased information with body schema from the temporoparietal cortex to the frontal cortex might help PDFOG compensate and maintain anticipatory postural adjustment and locomotion (Takakusaki et al., 2003).
Motor Imagery of Normal Turning but Not FOG Turning Distinguishes PDFOG From PDNOFOG and Controls
Our ROI analysis revealed a significantly higher beta value in the CLR in PDFOG compared with controls and PDNOFOG during MI of normal turning. However, the difference between PDFOG with controls and PDNOFOG was quenched when the patients performed FOG turning MI. It is currently uncertain if the increased normal turning-related CLR is a compensatory adaptation for overcoming FOG or a pathological maladaptation leading to FOG. Since the phenomenon was not observed when doing FOG turning MI, a compensatory adaptation seems likely. A recent study illustrated that the CLR activities increased after receiving a course of “adapted resistance training with instability” in PDFOG patients (Vieira-Yano et al., 2021). The increased CLR activities of fMRI were associated with the dual-task cost on stride length improvement of freezers (Vieira-Yano et al., 2021). The notion strengthened the viewpoint that an increased CLR activity is a compensatory adaptation rather than a pathological maladaptation. We also noticed that increased normal turning-related CLR activity in PDFOG was significantly positively correlated with PD disease duration. The phenomenon implies that along with the progression of PD, stronger CLR compensation might be required to catch up the normality of walking. There was no significant correlation between CLR activity and disease severity in PDFOG. This might be due to the parameters (UPDRS, UPDRS-III, H&Y, and nFOG-Q) we used for disease severity assessment could not vividly reflect the degree of dynamic gait disorders in PDFOG. The cerebellum was anatomically and functionally connected with brainstem structures involved in gait and balance control (Youn et al., 2015). Several recent studies have provided evidence on the increased cerebellar functional connectivity with the posterior cortical areas in PDFOG (Bharti et al., 2019; Jung et al., 2020). These findings further support our notion that CLR might play a compensatory role in PDFOG.
Furthermore, a similar phenomenon was also encountered in the MLR and the SLR, with higher beta value in the MLR and SLR found in PDFOG than in PDNOFOG during MI of normal turning. It is likely that PDFOG patients may require a high impulse drive of locomotion regions to maintain their non-freezing ambulation (Gratsch et al., 2019). la Fougère et al. reported that the CLR, MLR, and SLR activity increases during MI of planning and modulation of locomotion (La Fougere et al., 2010), which could partially explain the increased burden of the locomotion regions to modify the complex gait during normal turning imagery in PDFOG. Increased functional connectivity between SMA with MLR and CLR in PDFOG compared with PDNOFOG was also reported (Fling et al., 2014). However, the increased activation in the CLR, MLR, and SLR in PDFOG might not sustain adequately to stop the ongoing freezing all the time, and FOG might breach the compensation intermittently throughout the course of walking (Gratsch et al., 2019). Furthermore, Mori et al. demonstrated that stimulation of the CLR can independently induce locomotion in decerebrate cats (Mori et al., 1999; Rahimpour et al., 2021). However, although stimulation of the SLR or MLR also could evoke locomotion in decerebrate cats, the coordination of the limbs was greatly disrupted and extensor rigidity was elicited (Orlovskii, 1970; Rahimpour et al., 2021). These findings suggest that the CLR may play a pivotal role in coordinating SLR and MLR for generating and monitoring locomotion (Rahimpour et al., 2021). The current findings of no significant difference between the PDFOG and normal subjects in MLR and SLR activities as what we saw in CLR also strengthen the notion.
Unique Complex (Turning) vs. Simple (Straight Walking) Walking Patterns of PDFOG
Turning requires more complex neural control than straight walking. While comparing normal turning to normal straight walking among groups, PDFOG showed an increased activation of the left inferior frontal lobe, right putamen, and right inferior parietal lobule compared to controls. The parietal and frontal regions were responsible for attention shifts and generating a high-level perception of motion (Culham et al., 1998), whereas the putamen regulates movement preparation and execution. As a result, the increased putamen activity together with the frontal and parietal lobule in PDFOG during normal turning in this study might indicate the requirement of attentive motion control in PDFOG during complex gait. This might also explain why dual tasks easily trigger FOG attacks (Spildooren et al., 2010).
While comparing FOG turning to FOG straight walking condition, we found increased bilateral parietal and right occipital cerebral activities in PDFOG. In addition, an increased activation of the superior occipital lobule, together with the precentral and postcentral gyrus, was detected when PDFOG was compared to PDNOFOG. These findings are in line with those observed by Piramide et al. (2020), suggesting that the dorsal visual pathway of the parieto-occipital networks may enhance the spatiotemporal demands during locomotion. As a result, an increased dorsal visual pathway activation might also play a compensatory role in overcoming the fronto-striatal failure in PD during complex walking (Piramide et al., 2020). This condition might also explain why visual cue or action observation training could attenuate freezing attacks (Agosta et al., 2017). On the other hand, no significant differences were found in Peterson's study between imagined forward straight walking and turning in PDFOG and PDNOFOG (Peterson et al., 2014). The lack of significant difference might be attributed to the differences in the MI tasks used in their study, or the brain activation associated with their MI tasks was too subtle to produce detectable activity changes. In the current study, we incorporated the videos of different gait conditions to explicitly guide MI performance of participants, and it consistently elicited different brain responses to different gait conditions. This may be one of the pivotal reasons that cause the discrepancy between Peterson's study and the current study.
Correlation Between Gait Parameters and FOG
In this study, PDFOG had the slowest gait, smallest stride length, increased stance time, decreased swing time, least single support time, and increased total double support time. These results are in line with those of recently conducted studies (Vervoort et al., 2016; Mitchell et al., 2019). However, no significant correlation between gait parameters and brain activation was detected in this study. This suggested that FOG was a complex phenomenon involving multiple brain areas or brain networks. It could not simply be explained by a single gait parameter or the activity in one single brain region. A recent study illustrated that gait variability of stride length and walking velocity positively correlated with precuneus neural activity in PDFOG (Bommarito et al., 2020). The finding charged a new possibility for exploiting the relationship among the sophisticated FOG brain activities and gait parameters in the future.
Conclusions
In conclusion, the central neural function of PDFOG differs from that of PDNOFOG. The alternation of the central neural function in PDFOG can be triggered by the MI of various walking conditions. Complex gait requires more complex neural control than simple gait in both normal and FOG conditions, and the escalation of the locomotion region activities was required for compensating the insufficient function of fronto-striatal circuitry during the MI of complex gait in PDFOG than in other participant groups.
Data Availability Statement
The raw data supporting the conclusions of this article will be made available by the authors, without undue reservation.
Ethics Statement
The studies involving human participants were reviewed and approved by China Medical University and Hospital Research Ethics Committee. The patients/participants provided their written informed consent to participate in this study.
Author Contributions
B-LL, C-MC, H-CH, J-CC, M-KL, J-RD, and C-HT were responsible for the conception and design of the study. B-LL, C-MC, H-CH, C-IL, H-CL, and G-JW contributed to the collection and analysis of data. H-CH, M-KL, J-RD, and C-HT were responsible for the drafting of the manuscript. All authors critically revised the draft and approved the final version.
Funding
This study was supported in part by grants from the Ministry of Science and Technology (MOST 105-2314-B-039-004-MY2, MOST 106-2410-H-008-054-, MOST 107-2314-B-039-017-MY3, MOST 107-2221-E-008-072-MY2, MOST 105-2410-H-039-003-, and MOST 110-2314-B-039-039-) and the China Medical University Hospital (Taiwan) (DMR-105-055, DMR-110-218, and DMR-109-069).
Conflict of Interest
The authors declare that the research was conducted in the absence of any commercial or financial relationships that could be construed as a potential conflict of interest.
Publisher's Note
All claims expressed in this article are solely those of the authors and do not necessarily represent those of their affiliated organizations, or those of the publisher, the editors and the reviewers. Any product that may be evaluated in this article, or claim that may be made by its manufacturer, is not guaranteed or endorsed by the publisher.
References
Agosta, F., Gatti, R., Sarasso, E., Volonte, M. A., Canu, E., Meani, A., et al. (2017). Brain plasticity in Parkinson's disease with freezing of gait induced by action observation training. J. Neurol. 264, 88–101. doi: 10.1007/s00415-016-8309-7
Aravamuthan, B. R., Mcnab, J. A., Miller, K. L., Rushworth, M., Jenkinson, N., Stein, J. F., et al. (2009). Cortical and subcortical connections within the pedunculopontine nucleus of the primate Macaca mulatta determined using probabilistic diffusion tractography. J. Clin. Neurosci. 16, 413–420. doi: 10.1016/j.jocn.2008.03.018
Bengevoord, A., Vervoort, G., Spildooren, J., Heremans, E., Vandenberghe, W., Bloem, B. R., et al. (2016). Center of mass trajectories during turning in patients with Parkinson's disease with and without freezing of gait. Gait Posture 43, 54–59. doi: 10.1016/j.gaitpost.2015.10.021
Bertoli, M., Croce, U. D., Cereatti, A., and Mancini, M. (2019). Objective measures to investigate turning impairments and freezing of gait in people with Parkinson's disease. Gait Posture 74, 187–193. doi: 10.1016/j.gaitpost.2019.09.001
Bharti, K., Suppa, A., Pietracupa, S., Upadhyay, N., Gianni, C., Leodori, G., et al. (2019). Abnormal cerebellar connectivity patterns in patients with Parkinson's disease and freezing of gait. Cerebellum 18, 298–308. doi: 10.1007/s12311-018-0988-4
Bommarito, G., Putzolu, M., Avanzino, L., Cosentino, C., Botta, A., Marchese, R., et al. (2020). Functional correlates of action observation of gait in patients with Parkinson's disease. Neural Plast. 2020:8869201. doi: 10.1155/2020/8869201
Chaudhuri, K. R., Martinez-Martin, P., Brown, R. G., Sethi, K., Stocchi, F., Odin, P., et al. (2007). The metric properties of a novel non-motor symptoms scale for Parkinson's disease: Results from an international pilot study. Mov. Disord. 22, 1901–1911. doi: 10.1002/mds.21596
Cugusi, L., Manca, A., Dragone, D., Deriu, F., Solla, P., Secci, C., et al. (2017). Nordic walking for the management of people with Parkinson disease: a systematic review. PMR 9, 1157–1166. doi: 10.1016/j.pmrj.2017.06.021
Culham, J. C., Brandt, S. A., Cavanagh, P., Kanwisher, N. G., Dale, A. M., and Tootell, R. B. (1998). Cortical fMRI activation produced by attentive tracking of moving targets. J. Neurophysiol. 80, 2657–2670. doi: 10.1152/jn.1998.80.5.2657
Duann, J. R., and Chiou, J. C. (2016). A comparison of independent event-related desynchronization responses in motor-related brain areas to movement execution, movement imagery, and movement observation. PLoS ONE 11:e0162546. doi: 10.1371/journal.pone.0162546
Evans, A. C., Marrett, S., Torrescorzo, J., Ku, S., and Collins, L. (1991). MRI-PET correlation in three dimensions using a volume-of-interest (VOI) atlas. J. Cereb. Blood Flow Metab. 11, A69–78. doi: 10.1038/jcbfm.1991.40
Fling, B. W., Cohen, R. G., Mancini, M., Carpenter, S. D., Fair, D. A., Nutt, J. G., et al. (2014). Functional reorganization of the locomotor network in Parkinson patients with freezing of gait. PLoS ONE 9:e100291. doi: 10.1371/journal.pone.0100291
Folstein, M. F., Folstein, S. E., and Mchugh, P. R. (1975). “Mini-mental state”. A practical method for grading the cognitive state of patients for the clinician. J. Psychiatr. Res. 12, 189–198. doi: 10.1016/0022-3956(75)90026-6
Friston, K. J., Frith, C. D., Frackowiak, R. S., and Turner, R. (1995). Characterizing dynamic brain responses with fMRI: a multivariate approach. Neuroimage 2, 166-172.
Friston, K. J., Holmes, A. P., Worsley, K. J., Poline, J.-P., Frith, C. D., and Frackowiak, R. S. J. (1994). Statistical parametric maps in functional imaging: A general linear approach. Hum. Brain Mapp. 2, 189–210. doi: 10.1002/hbm.460020402
Genovese, C. R., Lazar, N. A., and Nichols, T. (2002). Thresholding of statistical maps in functional neuroimaging using the false discovery rate. Neuroimage 15, 870–878. doi: 10.1006/nimg.2001.1037
Gibb, W. R., Fearnley, J. M., and Lees, A. J. (1990). The anatomy and pigmentation of the human substantia nigra in relation to selective neuronal vulnerability. Adv. Neurol. 53, 31–34.
Gibb, W. R., and Lees, A. J. (1988). The relevance of the Lewy body to the pathogenesis of idiopathic Parkinson's disease. J. Neurol. Neurosurg. Psychiatry 51, 745–752. doi: 10.1136/jnnp.51.6.745
Gilat, M., Shine, J. M., Walton, C. C., O'callaghan, C., Hall, J. M., and Lewis, S. J. G. (2015). Brain activation underlying turning in Parkinson's disease patients with and without freezing of gait: a virtual reality fMRI study. NPJ Parkinsons Dis 1:15020. doi: 10.1038/npjparkd.2015.20
Gratsch, S., Auclair, F., Demers, O., Auguste, E., Hanna, A., Buschges, A., et al. (2019). A brainstem neural substrate for stopping locomotion. J. Neurosci. 39, 1044–1057. doi: 10.1523/JNEUROSCI.1992-18.2018
Hetu, S., Gregoire, M., Saimpont, A., Coll, M. P., Eugene, F., Michon, P. E., et al. (2013). The neural network of motor imagery: an ALE meta-analysis. Neurosci. Biobehav. Rev. 37, 930–949. doi: 10.1016/j.neubiorev.2013.03.017
Isaac, A. R., Marks, D. F., and Russell, D. G. (1986). An instrument for assessing imagery of movement. The vividness of movement imagery questionnaire. J Mental Imagery 10, 23–30.
Iseki, K., Hanakawa, T., Shinozaki, J., Nankaku, M., and Fukuyama, H. (2008). Neural mechanisms involved in mental imagery and observation of gait. Neuroimage 41, 1021–1031. doi: 10.1016/j.neuroimage.2008.03.010
Jacobs, J. V., Lou, J. S., Kraakevik, J. A., and Horak, F. B. (2009). The supplementary motor area contributes to the timing of the anticipatory postural adjustment during step initiation in participants with and without Parkinson's disease. Neuroscience 164, 877–885. doi: 10.1016/j.neuroscience.2009.08.002
Jeannerod, M., and Decety, J. (1995). Mental motor imagery: a window into the representational stages of action. Curr. Opin. Neurobiol. 5, 727–732. doi: 10.1016/0959-4388(95)80099-9
Jenkins, I. H., Fernandez, W., Playford, E. D., Lees, A. J., Frackowiak, R. S., Passingham, R. E., et al. (1992). Impaired activation of the supplementary motor area in Parkinson's disease is reversed when akinesia is treated with apomorphine. Ann. Neurol. 32, 749–757. doi: 10.1002/ana.410320608
Jung, J. H., Kim, B. H., Chung, S. J., Yoo, H. S., Lee, Y. H., Baik, K., et al. (2020). Motor cerebellar connectivity and future development of freezing of gait in de novo parkinson's disease. Mov. Disord. 35, 2240–2249. doi: 10.1002/mds.28243
Kaas, A., Weigelt, S., Roebroeck, A., Kohler, A., and Muckli, L. (2010). Imagery of a moving object: the role of occipital cortex and human MT/V5+. Neuroimage 49, 794–804. doi: 10.1016/j.neuroimage.2009.07.055
Kolster, H., Peeters, R., and Orban, G. A. (2010). The retinotopic organization of the human middle temporal area MT/V5 and its cortical neighbors. J. Neurosci. 30, 9801–9820. doi: 10.1523/JNEUROSCI.2069-10.2010
La Fougere, C., Zwergal, A., Rominger, A., Forster, S., Fesl, G., Dieterich, M., et al. (2010). Real versus imagined locomotion: a [18F]-FDG PET-fMRI comparison. Neuroimage 50, 1589–1598. doi: 10.1016/j.neuroimage.2009.12.060
Maidan, I., Bernad-Elazari, H., Gazit, E., Giladi, N., Hausdorff, J. M., and Mirelman, A. (2015). Changes in oxygenated hemoglobin link freezing of gait to frontal activation in patients with Parkinson disease: an fNIRS study of transient motor-cognitive failures. J. Neurol. 262, 899–908. doi: 10.1007/s00415-015-7650-6
Mckay, J. L., Lang, K. C., Ting, L. H., and Hackney, M. E. (2018). Impaired set shifting is associated with previous falls in individuals with and without Parkinson's disease. Gait Posture 62, 220–226. doi: 10.1016/j.gaitpost.2018.02.027
Mitchell, T., Conradsson, D., and Paquette, C. (2019). Gait and trunk kinematics during prolonged turning in Parkinson's disease with freezing of gait. Parkinsonism Relat. Disord. 64, 188–193. doi: 10.1016/j.parkreldis.2019.04.011
Mori, S., Matsui, T., Kuze, B., Asanome, M., Nakajima, K., and Matsuyama, K. (1999). Stimulation of a restricted region in the midline cerebellar white matter evokes coordinated quadrupedal locomotion in the decerebrate cat. J. Neurophysiol. 82, 290–300. doi: 10.1152/jn.1999.82.1.290
Naismith, S. L., Shine, J. M., and Lewis, S. J. (2010). The specific contributions of set-shifting to freezing of gait in Parkinson's disease. Mov. Disord. 25, 1000–1004. doi: 10.1002/mds.23005
Nieuwboer, A., Rochester, L., Herman, T., Vandenberghe, W., Emil, G. E., Thomaes, T., et al. (2009). Reliability of the new freezing of gait questionnaire: agreement between patients with Parkinson's disease and their carers. Gait Posture 30, 459–463. doi: 10.1016/j.gaitpost.2009.07.108
Nonnekes, J., Snijders, A. H., Nutt, J. G., Deuschl, G., Giladi, N., and Bloem, B. R. (2015). Freezing of gait: a practical approach to management. Lancet Neurol. 14, 768–778. doi: 10.1016/S1474-4422(15)00041-1
Nutt, J. G. (2013). Higher-level gait disorders: an open frontier. Mov. Disord. 28, 1560–1565. doi: 10.1002/Mds.25673
Nutt, J. G., Bloem, B. R., Giladi, N., Hallett, M., Horak, F. B., and Nieuwboer, A. (2011). Freezing of gait: moving forward on a mysterious clinical phenomenon. Lancet Neurol. 10, 734–744. doi: 10.1016/S1474-4422(11)70143-0
Orlovskii, G. N. (1970). Relations between reticulo-spinal neurons and locomotor regions of the brain stem. Biofizika 15, 171–178.
Peterson, D. S., Pickett, K. A., Duncan, R., Perlmutter, J., and Earhart, G. M. (2014). Gait-related brain activity in people with Parkinson disease with freezing of gait. PLoS ONE 9:e90634. doi: 10.1371/journal.pone.0090634
Piramide, N., Agosta, F., Sarasso, E., Canu, E., Volonte, M. A., and Filippi, M. (2020). Brain activity during lower limb movements in Parkinson's disease patients with and without freezing of gait. J. Neurol. 267, 1116–1126. doi: 10.1007/s00415-019-09687-1
Poline, J. B., and Brett, M. (2012). The general linear model and fMRI: does love last forever? Neuroimage 62, 871–880. doi: 10.1016/j.neuroimage.2012.01.133
Potvin-Desrochers, A., Mitchell, T., Gisiger, T., and Paquette, C. (2019). Changes in resting-state functional connectivity related to freezing of gait in Parkinson's disease. Neuroscience 418, 311–317. doi: 10.1016/j.neuroscience.2019.08.042
Rahimpour, S., Gaztanaga, W., Yadav, A. P., Chang, S. J., Krucoff, M. O., Cajigas, I., et al. (2021). Freezing of gait in Parkinson's disease: invasive and noninvasive neuromodulation. Neuromodulation 24, 829–842. doi: 10.1111/ner.13347
Rahman, S., Griffin, H. J., Quinn, N. P., and Jahanshahi, M. (2008a). The factors that induce or overcome freezing of gait in Parkinson's disease. Behav. Neurol. 19, 127–136. doi: 10.1155/2008/456298
Rahman, S., Griffin, H. J., Quinn, N. P., and Jahanshahi, M. (2008b). Quality of life in Parkinson's disease: the relative importance of the symptoms. Mov. Disord. 23, 1428–1434. doi: 10.1002/mds.21667
Shine, J. M., Matar, E., Ward, P. B., Frank, M. J., Moustafa, A. A., Pearson, M., et al. (2013). Freezing of gait in Parkinson's disease is associated with functional decoupling between the cognitive control network and the basal ganglia. Brain 136, 3671–3681. doi: 10.1093/brain/awt272
Shine, J. M., Moore, S. T., Bolitho, S. J., Morris, T. R., Dilda, V., Naismith, S. L., et al. (2012). Assessing the utility of freezing of gait questionnaires in Parkinson's disease. Parkinsonism Relat. Disord. 18, 25–29. doi: 10.1016/j.parkreldis.2011.08.002
Smulders, K., Dale, M. L., Carlson-Kuhta, P., Nutt, J. G., and Horak, F. B. (2016). Pharmacological treatment in Parkinson's disease: effects on gait. Parkinsonism Relat. Disord. 31, 3–13. doi: 10.1016/j.parkreldis.2016.07.006
Snijders, A. H., Leunissen, I., Bakker, M., Overeem, S., Helmich, R. C., Bloem, B. R., et al. (2011). Gait-related cerebral alterations in patients with Parkinson's disease with freezing of gait. Brain 134, 59–72. doi: 10.1093/brain/awq324
Spildooren, J., Vercruysse, S., Desloovere, K., Vandenberghe, W., Kerckhofs, E., and Nieuwboer, A. (2010). Freezing of gait in Parkinson's disease: the impact of dual-tasking and turning. Mov. Disord. 25, 2563–2570. doi: 10.1002/mds.23327
Szameitat, A. J., Shen, S., and Sterr, A. (2007). Motor imagery of complex everyday movements. An fMRI study. Neuroimage 34, 702–713. doi: 10.1016/j.neuroimage.2006.09.033
Takakusaki, K. (2013). Neurophysiology of gait: from the spinal cord to the frontal lobe. Mov. Disord. 28, 1483–1491. doi: 10.1002/Mds.25669
Takakusaki, K., Habaguchi, T., Ohtinata-Sugimoto, J., Saitoh, K., and Sakamoto, T. (2003). Basal ganglia efferents to the brainstem centers controlling postural muscle tone and locomotion: a new concept for understanding motor disorders in basal ganglia dysfunction. Neuroscience 119, 293–308. doi: 10.1016/s0306-4522(03)00095-2
Tomlinson, C. L., Stowe, R., Patel, S., Rick, C., Gray, R., and Clarke, C. E. (2010). Systematic review of levodopa dose equivalency reporting in Parkinson's disease. Mov. Disord. 25, 2649–2653. doi: 10.1002/mds.23429
Vervoort, G., Heremans, E., Bengevoord, A., Strouwen, C., Nackaerts, E., Vandenberghe, W., et al. (2016). Dual-task-related neural connectivity changes in patients with Parkinson' disease. Neuroscience 317, 36–46. doi: 10.1016/j.neuroscience.2015.12.056
Vieira-Yano, B., Martini, D. N., Horak, F. B., De Lima-Pardini, A., Almeida, F., Santana, V. P., et al. (2021). The adapted resistance training with instability randomized controlled trial for gait automaticity. Mov. Disord. 36, 152–163. doi: 10.1002/mds.28298
Wang, J., Wai, Y., Weng, Y., Ng, K., Huang, Y. Z., Ying, L., et al. (2009). Functional MRI in the assessment of cortical activation during gait-related imaginary tasks. J. Neural. Transm. 116, 1087–1092. doi: 10.1007/s00702-009-0269-y
Wang, M., Jiang, S., Yuan, Y., Zhang, L., Ding, J., Wang, J., et al. (2016). Alterations of functional and structural connectivity of freezing of gait in Parkinson's disease. J. Neurol. 263, 1583–1592. doi: 10.1007/s00415-016-8174-4
Youn, J., Lee, J. M., Kwon, H., Kim, J. S., Son, T. O., and Cho, J. W. (2015). Alterations of mean diffusivity of pedunculopontine nucleus pathway in Parkinson's disease patients with freezing of gait. Parkinsonism Relat. Disord. 21, 12–17. doi: 10.1016/j.parkreldis.2014.10.003
Keywords: Parkinson's disease, freezing of gait, motor imagery, functional magnetic resonance imaging, gait disorders
Citation: Huang H-C, Chen C-M, Lu M-K, Liu B-L, Li C-I, Chen J-C, Wang G-J, Lin H-C, Duann J-R and Tsai C-H (2021) Gait-Related Brain Activation During Motor Imagery of Complex and Simple Ambulation in Parkinson's Disease With Freezing of Gait. Front. Aging Neurosci. 13:731332. doi: 10.3389/fnagi.2021.731332
Received: 26 June 2021; Accepted: 23 August 2021;
Published: 22 September 2021.
Edited by:
Allison B. Reiss, New York University, United StatesReviewed by:
Tiffany Morris, Bard Early College, United StatesVinita Ganesh Chittoor, Stanford University, United States
Copyright © 2021 Huang, Chen, Lu, Liu, Li, Chen, Wang, Lin, Duann and Tsai. This is an open-access article distributed under the terms of the Creative Commons Attribution License (CC BY). The use, distribution or reproduction in other forums is permitted, provided the original author(s) and the copyright owner(s) are credited and that the original publication in this journal is cited, in accordance with accepted academic practice. No use, distribution or reproduction is permitted which does not comply with these terms.
*Correspondence: Chon-Haw Tsai, d2luZHltb3ZlbWVudCYjeDAwMDQwO2dtYWlsLmNvbQ==; Jeng-Ren Duann, amVuZ3JlbjAwJiN4MDAwNDA7Z21haWwuY29t
†These authors have contributed equally to this work and share first authorship