- 1Department of Neurology, Xiangya Hospital, Central South University, Changsha, China
- 2National Clinical Research Center for Geriatric Disorders, Central South University, Changsha, China
- 3Key Laboratory of Hunan Province in Neurodegenerative Disorders, Central South University, Changsha, China
- 4Department of Geriatrics Neurology, Xiangya Hospital, Central South University, Changsha, China
- 5Department of Radiology, Xiangya Hospital, Central South University, Changsha, China
- 6Key Laboratory of Organ Injury, Aging and Regenerative Medicine of Hunan Province, Changsha, China
The genes involved in the metabolic pathways of amyloid-β (Aβ) and tau proteins significantly influence the etiology of Alzheimer’s disease (AD). Various studies have explored the associations between some of these genes and AD in the Caucasian population; however, researches regarding these associations remain limited in the Chinese population. To systematically evaluate the associations of these genes with AD, we investigated 19 genes involved in the metabolism of Aβ and tau based on previous studies selected using the PubMed database. This study included 372 patients with sporadic late-onset AD (sLOAD) and 345 cognitively healthy individuals from southern China. The results were replicated in the International Genomics of Alzheimer’s Project (IGAP). Protein–protein interactions were determined using the STRING v11 database. We found that a single-nucleotide polymorphism, rs11682128, of BIN1 conferred susceptibility to sLOAD after adjusting for age, sex, and APOE ε4 status and performing the Bonferroni correction {corrected P = 0.000153, odds ratio (OR) [95% confidence interval (CI)] = 1.403 (1.079–1.824)}, which was replicated in the IGAP. Protein–protein interactions indicated that BIN1 was correlated with MAPT. Moreover, rare variants of NEP and FERMT2 (0.0026 < corrected P < 0.05), and the Aβ degradation, tau pathology, and tau phosphatase pathways (0.01 < corrected P < 0.05), were nominally significantly associated with sLOAD. This study suggested that the genes involved in the metabolic pathways of Aβ and tau contributed to the etiology of sLOAD in the southern Han Chinese population.
Introduction
Alzheimer’s disease (AD) is the most common neurodegenerative disorder characterized by cognitive impairment and neuropsychiatric symptoms (Scheltens et al., 2016). The prevalence of AD is rising rapidly owing to the aging of the populations. It is estimated that 50 million individuals have dementia worldwide, two thirds of whom are diagnosed with AD (Saez-Atienzar and Masliah, 2020). The global incidence of patients with dementia is expected to reach 152 million by 2050 (Patterson, 2018). AD can be classified as early onset AD (EOAD) (age of onset <65 years) or late-onset AD (LOAD) (age of onset ≥65 years), depending on the age at the time of onset (Li et al., 2018). Moreover, AD can be divided into familial AD (FAD) and sporadic AD based on family history (Dorszewska et al., 2016). The term sporadic LOAD (sLOAD) is used to describe sporadic AD patients with an onset age ≥65 years (Cruchaga et al., 2018).
Amyloid precursor protein (APP), presenilin 1 (PSEN1), and presenilin 2 (PSEN2) are causative genes associated with FAD (Jiao et al., 2014). However, the etiology of sLOAD remains elusive. Multiple genetic and environmental risk factors contribute to the pathogenesis of sLOAD. To date, more than 40 risk loci for AD have been identified by genome-wide association studies (GWASs), which have implicated amyloid-β (Aβ), tau, and lipid metabolism in the development of AD (Lambert et al., 2013; Kunkle et al., 2019). Aβ and tau protein deposition are the two principal pathological hallmarks of AD. The imbalance between Aβ production and clearance leads to the aggregation of senile plaques, which result in neuronal loss in AD (Hardy and Selkoe, 2002). Meanwhile, the hyperphosphorylated tau protein can transform into neurofibrillary tangles under pathological conditions, also causing neuronal degeneration in AD. Thus, the metabolism of Aβ and tau proteins plays a critical role in the pathogenesis of AD (Kametani and Hasegawa, 2018).
Amyloid-β is generated by the proteolytic cleavage of the Aβ protein precursor by β-secretases and γ-secretases. The beta-amyloid cleaving enzyme 1 (BACE1) is a β-secretase enzyme, which initiates the cleavage of APP to form Aβ. A recent study showed that the beta-amyloid cleaving enzyme 2 (BACE2), a homolog of BACE1, can also process APP at the β-site and contribute to the pathogenesis of AD (Wang et al., 2019). The Aβ clearance rate was significantly impaired in patients with LOAD compared with cognitively healthy individuals, while no differences in the Aβ production rate were observed between the two groups (Mawuenyega et al., 2010). Thus, the clearance of Aβ is of great importance in the pathogenesis of LOAD. Aβ-degrading proteases are involved in the proteolytic degradation of Aβ, including neprilysin (NEP), endothelin-converting enzyme-1 (ECE-1), insulin-degrading enzyme (IDE), membrane metallo-endopeptidase-like 1 (MMEL1), angiotensin-converting enzyme (ACE), and matrix metalloproteinase-9 (MMP-9) (Saido and Leissring, 2012).
The equilibrium of tau phosphorylation is regulated by tau kinases and phosphatases (Oliveira et al., 2017). Tau kinases contribute to the phosphorylation of tau protein by transferring the phosphate group from high-energy donor molecules to the tau protein. Common tau kinases include glycogen synthase kinase-3 β (GSK3β), cyclin-dependent protein kinase-5 (CDK5), and mitogen-activated protein kinase 1 (MAPK1) (Martin et al., 2013). Phosphorylated tau proteins can be dephosphorylated by tau protein phosphatases. Protein phosphatase-2A (PP2A) is one of the primary tau phosphatases associated with decreased Aβ production in the human brain (Shentu et al., 2018). The overall activity of PP2A is facilitated by holoenzymes consisting of a catalytic subunit alpha (PP2CA) and a regulatory subunit B alpha (PP2R2A). Its activity is inhibited by the acidic nuclear phosphoprotein 32 family member A (ANP32A), which acts as an inhibitor. The methylation of PP2A by leucine carboxyl methyltransferase-1 (LCMT1) can increase PP2A activity (Stanevich et al., 2011); in contrast, demethylation by protein phosphatase methylesterase-1 (PPME1) reduces its activity (Liu et al., 2016). Peptidyl-prolyl cis-trans isomerase NIMA-interacting 1 (PIN1) induces conformational changes in tau protein and promotes tau dephosphorylation by PP2A (Vázquez-Higuera et al., 2011). Additionally, fermitin family member 2 (FERMT2) and bridging integrator 1 (BIN1) are involved in the modulation of tau pathology in both Drosophila and humans (Chapuis et al., 2013; Farfel et al., 2016).
The genes involved in the metabolic pathways of Aβ and tau have been reported to play a critical role in AD. Various studies have explored the association between some of these genes and AD. However, most of these studies were conducted in the Caucasian population (Clarimon et al., 2003; Helisalmi et al., 2004; Blomqvist et al., 2010), whereas data regarding the Chinese population remain limited (Zhou et al., 2018; Zhang et al., 2019b). In this study, we selected 19 genes involved in the metabolism of Aβ and tau, including Aβ degradation (ECE1, MMEL1, NEP, IDE, MMP9, and ACE), Aβ generation (BACE1 and BACE2), tau kinases (GSK3B, CDK5, and MARK1), tau pathology (FERMT2 and BIN1), and tau phosphatases (PPP2CA, PPP2R2A, ANP32A, LCMT1, PPME1, and PIN1) based on previously published studies available in the PubMed database (Figure 1). These five different metabolic pathways of genes associated with Aβ and tau proteins were screened in 372 patients with sLOAD and 345 cognitively healthy individuals. The single common variant association test and cumulative rare variant association test (gene and pathway based) were conducted for patients with sLOAD and cognitively healthy individuals [common variants: minor allele frequency (MAF) > 0.05; rare variant: MAF ≤ 0.05].
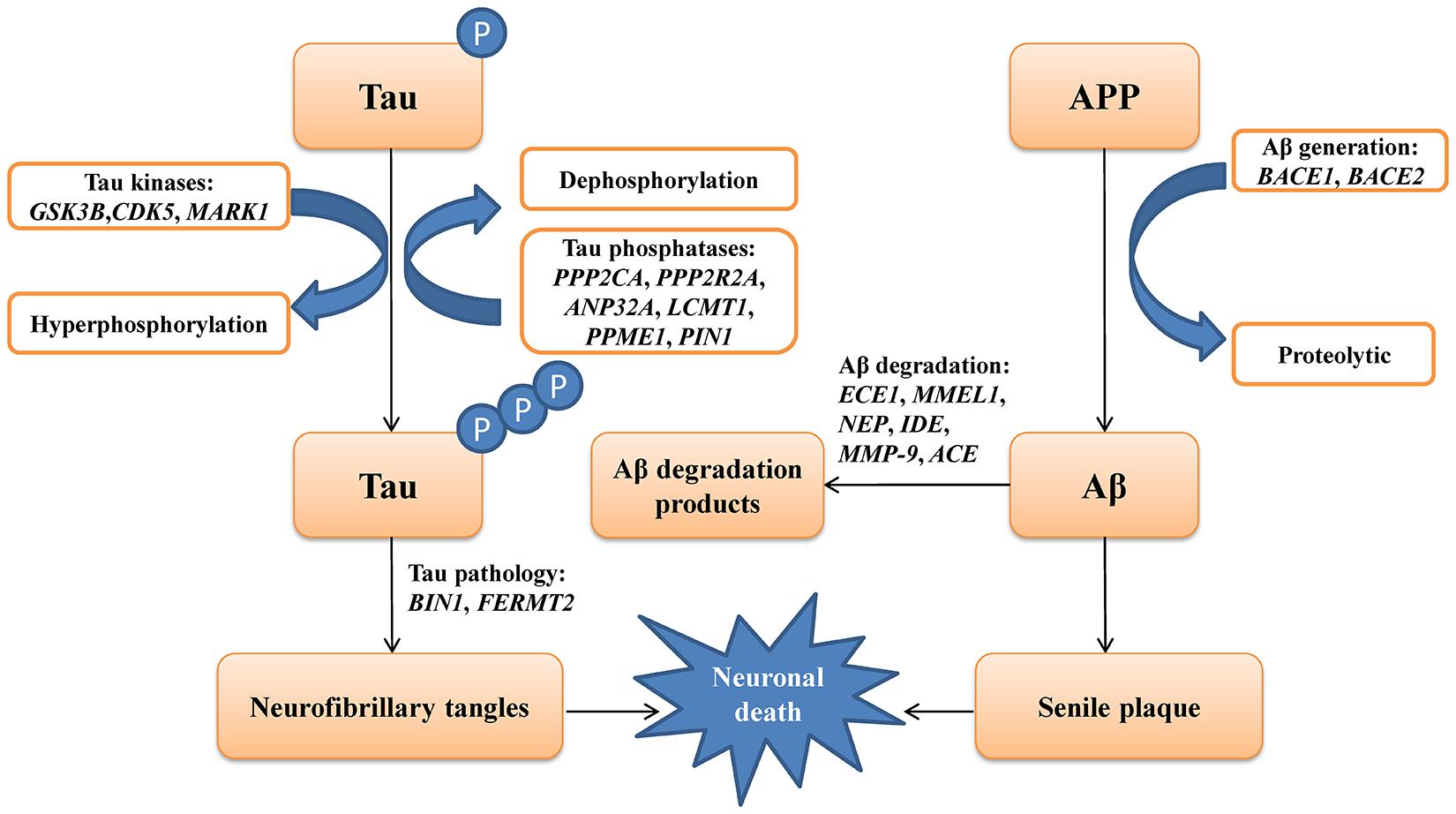
Figure 1. A schematic picture showing the genes involving the metabolic pathways of amyloid-β (Aβ) and tau protein in Alzheimer’s disease.
Materials and Methods
Participants
We recruited 372 patients with sLOAD (mean age at onset was 72.42 ± 7.82 years) from the outpatients and inpatients of the Department of Neurology, Xiangya Hospital, Central South University, and 345 cognitively healthy individuals (mean age at onset was 70.58 ± 5.34 years) from a community in Changsha. Mini-Mental State Examination (MMSE) scores were used to assess the cognitive ability of the cognitively healthy individuals. Their MMSE scores were equal or above 26 points (Table 1). The sLOAD patients met the National Institute on Aging/Alzheimer’s Association criteria for probable AD (McKhann et al., 2011). The courses of cognitive impairment for patients with sLOAD were over 6 months. The scores of the modified Hachinski ischemia scale were all less than 4. All selected participants were of southern Han Chinese descent, mainly from the provinces of Hunan, Jiangxi, Guizhou, and Guangxi provinces. Participants with causative mutations for AD (APP, PSEN1, and PSEN2) were excluded by Sanger sequencing. Additionally, we did not enroll the AD patients with secondary AD causes, such as vitamin deficiency, hypothyroidism, HIV, and syphilis infection. Patients with cerebral diseases and other neurological diseases were also excluded. This study was approved by the Ethics Committee of Xiangya Hospital of the Central South University (equivalent to an institutional review board). Written informed consent was obtained from each participant or their legal representatives.
Gene Screening and Genotyping
Genomic DNA was extracted from the peripheral blood leukocytes of each participant using the QIAGEN kit according to the manufacturer’s instructions. The quality and quantity of DNA were assessed using a fluorometer. All DNA samples were normalized to 50–100 ng/μl. We designed a targeted panel that included the following genes: Aβ degradation (ECE1, MMEL1, NEP, IDE, MMP9, and ACE) (Natunen et al., 2012; Baranello et al., 2015), Aβ generation (BACE1 and BACE2) (Wang et al., 2019), tau kinases (GSK3B, CDK5, and MARK1) (Martin et al., 2013), tau pathology (FERMT2 and BIN1) (Shulman et al., 2014; Calafate et al., 2016), and tau phosphatases (PPP2CA, PPP2R2A, ANP32A, LCMT1, PPME1, and PIN1) (Vázquez-Higuera et al., 2011). The participants’ genomic DNA was fragmented into 150–200 base-pair long fragments using the Bioruptor Pico (Belgium). Subsequently, the fragmented DNA was subjected to end-repairing, A-tailing, adaptor ligation, and an 11-cycle pre-capture polymerase chain reaction (PCR) amplification. After the PCR amplification, the DNA fragments were captured by the targeted panel, which was followed by sequencing using the Illumina NovaSeq 6000 platform. The mean sequencing depth was 641.6×, and the average sample coverage was 99.94%. The proportion of target region that covered ≥20× (%) and ≥30× (%) was 98.76% and 97.51%, respectively. The reads were mapped to the human reference genome (UCSC hg19/GRCH37) using the BWA software (version 0.7.15)1 (Li and Durbin, 2010), and duplicate sequence reads were removed by using Picard (version 2.18.7)2. Variant calling was conducted using the Genome Analysis Toolkit (GATK) (version 3.2)3 (McKenna et al., 2010). The variants were annotated using ANNOVAR4 (Wang et al., 2010) and named according to the guidelines of the Human Genome Variation Society (HGVS)5 (den Dunnen et al., 2016).
Statistical Analyses
Common Variant Association Test
Variants with MAF ≤ 0.05, genotyping rate <80%, and Hardy–Weinberg P value < 0.001 were filtered out using PLINK 1.9 (Purcell et al., 2007). For the remaining common variants, the association test was performed between sLOAD patients and cognitively healthy individuals by using PLINK 1.9. Furthermore, age, sex, and APOEε4 status (APOEε4+ and APOEε4−) were adjusted for each common variant with PLINK 1.9. Linkage disequilibrium (LD) patterns of the genes with nominally significant variants were reconstructed using Haploview version 4.2 (Barrett et al., 2005). Additionally, we performed the meta-analysis of nominally significant variants from our study and reported studies using the RevMan 5.4 software6.
Gene-Based Association Test
The optimized sequence kernel association test (SKAT-O) (Lee et al., 2012), which optimally combined the SKAT and burden tests, was installed in R using SKAT v1.0.9 to compare the aggregate burden of each gene between sLOAD patients and cognitively healthy individuals. The rare variants (MAF ≤ 0.05) within each gene were collapsed together, and their effects on sLOAD were assessed using the SKAT-O. Age, sex, and APOEε4 status were adjusted with the SKAT-O. Additionally, the “Chinese AD Exome” is the large whole-exome sequencing database of the Han Chinese population (Xu et al., 2018; Zhang et al., 2019a). The rare variants in our study were compared with those in the “Chinese AD Exome” available at www.alzdata.org (Xu et al., 2018).
Pathway-Based Association Test
We combined the rare variants in each pathway and performed the association test between sLOAD patients and cognitively healthy individuals using the SKAT-O test. SKAT-O was also used to adjust for age, sex, and APOEε4 status.
A P-value of 0.05 was defined as the threshold for nominal significance. Moreover, Bonferroni corrections were performed for the common variant association test, gene-based association test, and pathway-based association test. A cutoff P value ∗ n < 0.05 was considered as statistically significant (n is defined by the number of common variants, genes, or pathways in the tests).
Replication and Protein–Protein Interaction
The statistically significant common variants in our study were replicated in 30,344 LOAD patients and 52,427 cognitively healthy individuals of European ancestry from four consortia-conducted GWASs, which were meta-analyzed by the International Genomics of Alzheimer’s Project (IGAP). IGAP is a large two-stage study based upon GWASs on individuals of European ancestry. In stage 1, IGAP used genotyped and imputed data on 7,055,881 single-nucleotide polymorphisms (SNPs) to meta-analyze four previously published GWAS datasets consisting of 17,008 AD cases and 37,154 controls (The European Alzheimer’s disease Initiative-EADI, The Alzheimer Disease Genetics Consortium-ADGC, The Cohorts for Heart and Aging Research in Genomic Epidemiology consortium-CHARGE, The Genetic and Environmental Risk in AD consortium-GERAD). In stage 2, 11,632 SNPs were genotyped and tested for association in an independent set of 8,572 AD cases and 11,312 controls. Finally, a meta-analysis was performed combining results from stages 1 and 2 (Lambert et al., 2013). Additionally, protein–protein interactions (PPIs) were assessed using the STRING v11 database to determine whether the proteins involved in Aβ and tau metabolism were interacted with other AD-associated proteins using the STRING v11 database (Szklarczyk et al., 2019).
Results
This study included 372 sLOAD patients and 345 cognitively healthy individuals, all of whom were of southern Han Chinese ancestry (Table 1).
Common Variant Association Test
Ninety-eight variants remained after filtering out those with MAF ≤ 0.05, genotyping rate <80%, and Hardy–Weinberg P value < 0.001. We identified 13 nominally significant variants of genes involved in the metabolic pathways of tau pathology, tau phosphorylation, and Aβ degradation between sLOAD patients and cognitively healthy individuals (uncorrected P value < 0.05) (Table 2). Among the 13 nominally significant variants, six (46.2%) were UTR5 variants, four (30.8%) were UTR3 variants, two (15.4%) were intronic variants, and one (7.6%) was an exonic variant. SNP rs11682128 in BIN1 reached statistical significance between sLOAD patients and cognitively healthy individuals after adjusting for age, sex, and APOE ε4 status and after performing the Bonferroni correction [corrected P-value = 0.000153, OR (95% CI) = 1.403 (1.079–1.824)], based on a corrected P-value [P-value < 0.00051 (0.05/98)] (Table 2). None of the common variants in the metabolic pathways of Aβ degradation, Aβ generation, tau kinases, and tau phosphatase differed significantly between sLOAD patients and cognitively healthy individuals after correction. The detailed information of the 98 variants is shown in Supplementary Table 1. The LD patterns of SNPs in the BIN1 (rs11682128–rs11690153–rs11554586–rs58402148–rs4663093) and the NEP (rs1436633–rs1126662–rs701109–rs6665–rs12765) were similar between sLOAD patients and cognitively healthy individuals (Figure 2). Additionally, we performed the meta-analysis of nominally significant variants by using the data from our study and previous studies. We found that only NEP rs6665 and rs701109 were previously reported. No significant association was observed for NEP rs6665 and rs701109 in the meta-analysis of the current study and existing studies (Figure 3).
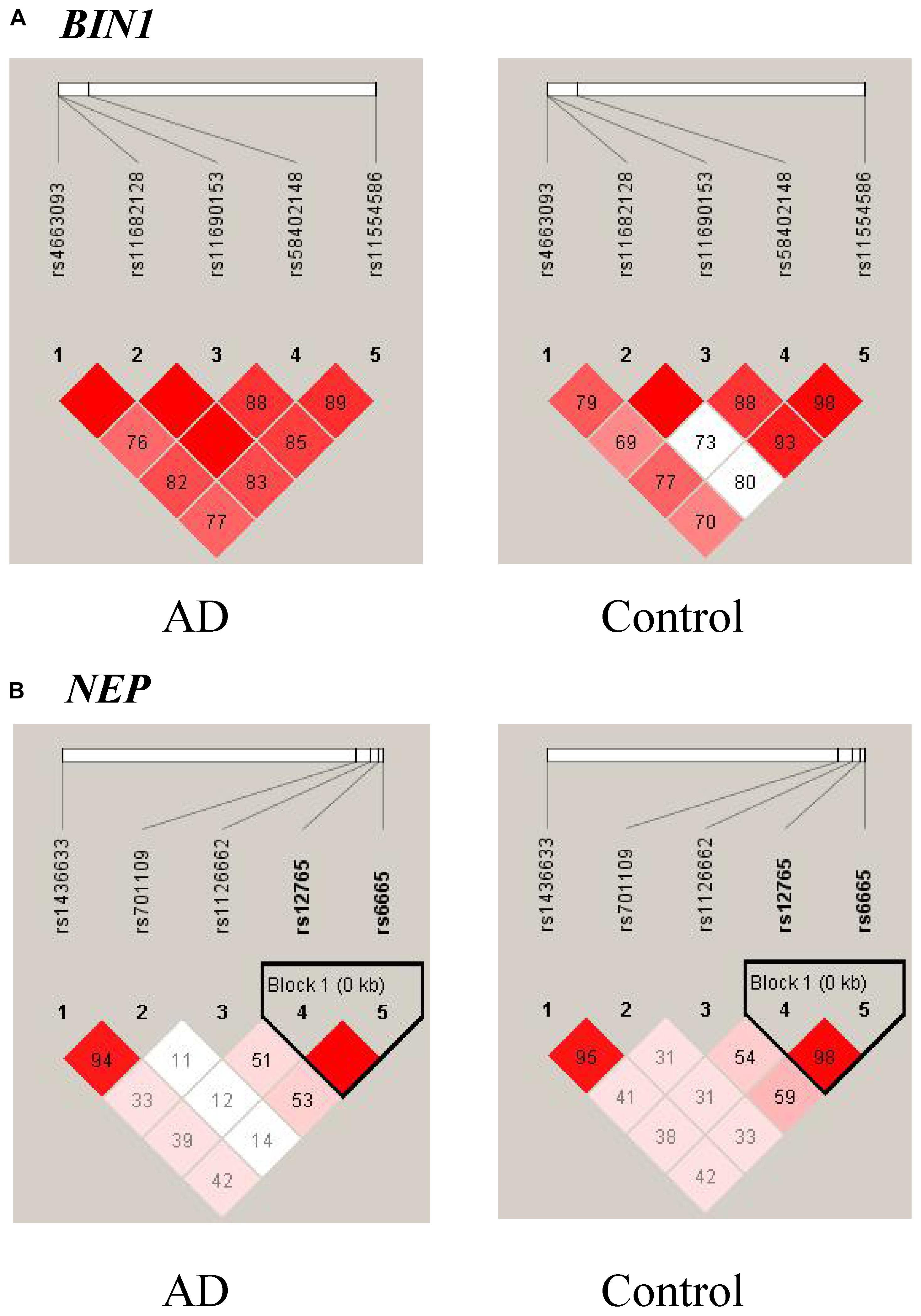
Figure 2. Linkage disequilibrium (LD) patterns of BIN1 (A) and NEP (B). The value in each square is equal to r2×100.
Gene-Based Association Test
In this study, the rare variants including coding and non-coding variants with MAF ≤ 0.05 were collapsed together, and their effects were studied between sLOAD patients and cognitively healthy individuals. After the Bonferroni correction was performed and age, sex, and APOE ε4 status were adjusted for, the rare variants of NEP and FERMT2 reached nominal significance between sLOAD patients and cognitively healthy individuals (0.0026 < corrected P-value < 0.05) (Table 3). The detailed information of rare synonymous and non-synonymous variants is shown in Supplementary Table 2. Nine rare variants existed both in our study and in the “Chinese AD Exome” with similar frequencies. The results are added in Supplementary Table 3.
Pathway-Based Association Test
In addition to the gene-based association test, we performed the pathway-based association test between sLOAD patients and cognitively healthy individuals by combining the rare variants with MAF ≤ 0.05 within each pathway using the SKAT-O test. After adjustment for age, sex, and APOEε4 status, the rare variants in the Aβ degradation, tau pathology, and tau phosphatase pathways were nominally significantly different between sLOAD patients and cognitively healthy individuals (0.01 < corrected P-value < 0.05). The rare variants in the Aβ generation and tau kinase pathways did not differ significantly between sLOAD patients and cognitively healthy individuals (corrected P-value > 0.05) (Table 4).
Replication and Protein–Protein Interaction
We replicated the BIN1 rs11682128 based on 30,344 LOAD patients and 52,427 cognitively healthy individuals from the IGAP, which showed a significant association between BIN1 rs11682128 and LOAD patients (P value: 1.451 × 10–10). PPI was performed to determine whether BIN1 was associated with proteins encoded by genes involved in the metabolic pathways of tau proteins (MAPT, GSK3B, CDK5, MARK1, FERMT2, PPP2CA, PPP2R2A, ANP32A, LCMT1, PPME1, and PIN1), which showed high confidence for the association between BIN1 and MAPT (Table 5 and Figure 4).
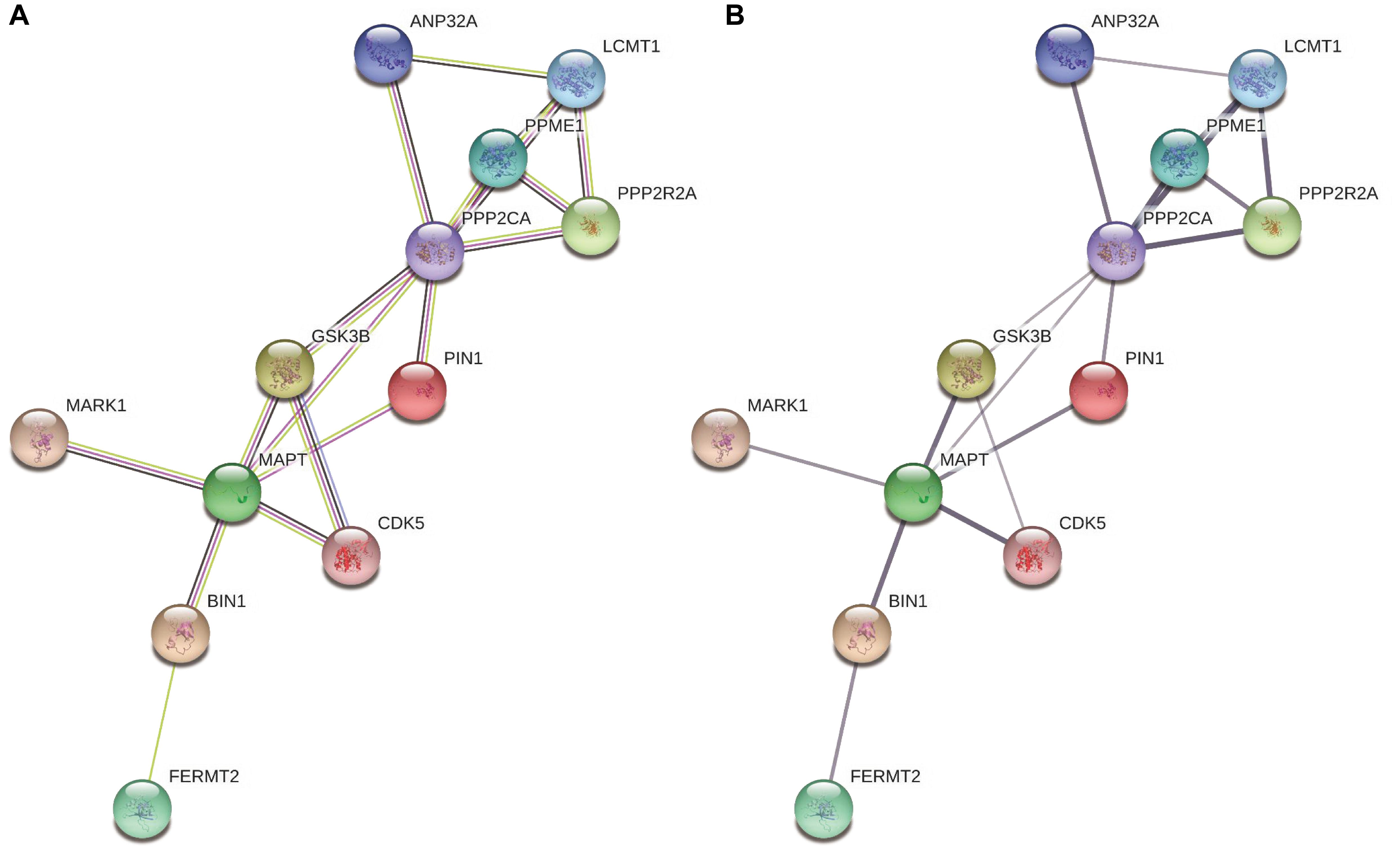
Figure 4. The interaction between BIN1 and other proteins involving the metabolic pathways of tau. (A) Colors represent different evidence sources (the experimental results, pink line; text mining sources, yellow line; gene co-expression, black line). (B) The thickness indicates the strength of protein interaction.
Discussion
In this study, we systematically investigated whether the genes associated with Aβ and tau metabolism conferred genetic susceptibility to sLOAD patients in the southern Han Chinese population using targeted panel sequencing. The common variant association test revealed that SNP rs11682128 of BIN1 increased the risk of sLOAD after adjusting for age, sex, and APOE ε4 status and performing the Bonferroni correction, which could be replicated in a European population from the IGAP. Furthermore, the rare variants of NEP and FERMT2 as well as the Aβ degradation, tau pathology, and tau phosphatase pathways were nominally associated with the risk of developing sLOAD.
The abnormal accumulations of Aβ and tau proteins are pathological hallmarks of AD and contribute to the neurodegenerative process in the brains of patients with AD. Although tau deposition was considered a downstream event in the Aβ hypothesis, increasing evidence indicated that Aβ and tau protein accumulations lead to neurotoxicity in parallel (Gomes et al., 2019). Previous studies have identified that three pathogenic genes (APP, PSEN1, and PSEN2) are involved in the pathogenesis of EOAD, suggesting the significant role of Aβ metabolism in EOAD (Jiao et al., 2014). A recent GWAS found that several novel genome-wide risk loci are implicated in Aβ and tau metabolism in LOAD, suggesting that the genes involved in the metabolism of Aβ and tau were associated with both EOAD and LOAD (Kunkle et al., 2019). Given the potentially important roles of genes in the metabolism of Aβ and tau, several studies investigated the relationship of some of these genes with AD. SNP rs1816558 of NEP was associated with an increased risk of AD in the Han Chinese population (Wang et al., 2016a). In the Northern Han Chinese population, rs6665 of NEP elevated the risk of LOAD patients (Liu et al., 2017). SNP rs3781239 of IDE conferred susceptibility to AD in Han Chinese (Wang et al., 2012). BIN1 is the second most important genetic risk factor in AD. SNP rs744373 of BIN1 increased the risk of developing AD in populations from East China (Wang et al., 2016b). In young Chinese healthy individuals, BIN1 rs744373 was linked with reduced functional connectivity and worse high-load working memory performance (Zhang et al., 2015). Nevertheless, in the Chinese population, studies on genes involved in the metabolic pathways of Aβ and tau remain limited. Most of the genetic data related to the metabolism of Aβ and tau are from the Caucasian population (Clarimon et al., 2003; Helisalmi et al., 2004; Wood et al., 2007; Miners et al., 2012; Sassi et al., 2016). Moreover, most of these studies focused on some SNPs by using array-based SNP genotyping. Thus, it is necessary to systematically investigate the association of genes involved in Aβ and tau metabolism with sLOAD in the Chinese population.
BIN1 is located on chromosome 2q14.3 and encodes BIN1, a membrane deforming protein expressed primarily in the brain and muscle. BIN1 has a significant role in endocytosis, cytoskeleton regulation, DNA repair, and apoptosis (Prokic et al., 2014). BIN1 is also associated with tau pathology because it regulates endocytosis of the tau protein (Calafate et al., 2016). The BIN1 ortholog, Amph, mediates the risk of AD due to its involvement in tau neurotoxicity (Chapuis et al., 2013). A three-stage analysis of 8,371 AD cases identified that SNP rs744373 in BIN1 attained genome-wide statistical significance (OR = 1.13; 95% CI:1.06–1.21; P = 1.6 × 10–11), suggesting that the BIN1 gene is a risk factor for AD (Seshadri et al., 2010). In our previous study, we also found that the BIN1 SNP rs744373 was associated with the risk of developing AD (Jiao et al., 2015). In addition, some GWASs showed that SNP rs6733839 in BIN1 conferred susceptibility to AD (Lambert et al., 2013; Kunkle et al., 2019). A study revealed that BIN1 SNP rs744373 was associated with memory deficit and higher tau deposits rather than amyloid deposition across brain regions using positron emission tomography–computed tomography scans, thus demonstrating that BIN1 may lead to cognitive impairment through tau pathology in vivo (Franzmeier et al., 2019). In the present study, we found that SNP rs11682128 in BIN1 was significantly associated with a higher risk of AD development and was replicated in the IGAP, which suggested that it can contribute to the risk of AD across different populations and may have a significant role in the development of AD. BIN1 rs11682128 is located in the non-coding region. With the use of GenoCanyon, SNP rs11682128 in BIN1 was predicted to be functional with a score of 1 (the cutoff value of the functional variant is 0.5) (Lu et al., 2015). Prioritization And Functional Assessment, a non-coding variant assessment software, showed that rs11682128 may be a functional variant (Zhou and Zhao, 2018). Additionally, by using another coding and non-coding variant assessment algorithm, Eigen-PC, the score of BIN1 rs11682128 was 3.156, confirming that it is likely to be functional (Ionita-Laza et al., 2016). Subsequently, BIN1 rs11682128 may be involved in the pathogenesis of AD via exhibiting regulatory activity in the expression of BIN1. Furthermore, there was high confidence regarding the interaction between BIN1 and MAPT, showing that BIN1 might exert a detrimental effect on AD by interacting with tau protein. Moreover, in our study, five common variants of the NEP gene were nominally significantly associated with sLOAD. Based on our data and those of the previously reported studies, the meta-analysis showed that NEP rs701109 and rs6665 did not differ significantly between AD patients and normal controls (Clarimon et al., 2003; Blomqvist et al., 2010; Miners et al., 2012; Wang et al., 2016a; Liu et al., 2017). Larger studies are warranted to elucidate the role of these two SNPs in the pathogenesis of AD.
High-throughput sequencing has made it possible to ascertain nearly all genetic variations, including rare variants, which are defined as variants with a MAF < 1–5%. Despite its potential importance, a rare variant would have a limited impact on a certain disease owing to its low allele frequency (Neale et al., 2011). Thus, there is a need to develop new methods to analyze rare variants by combining information on rare variants in genes or pathways. The burden test was the commonly used method for evaluating rare variants, in which all rare variants were summarized as a single genetic variable and subsequently tested for association with the disease. However, the burden test is limited in cases where either several neutral variants or both protective and risk variants are present (Morris and Zeggini, 2010). To overcome these limitations, another method, the SKAT, was proposed to evaluate the associations for rare variants (Wu et al., 2011). Nevertheless, the power of the SKAT is lower than that of the burden test if the rare variants impact a certain disease in the same direction. Lee et al. (2012) established the SKAT-O test, a linear combination of the burden test and SKAT, thus maximizing the power for analyzing rare variants. SKAT-O is widely used in neurological diseases, including AD (Nho et al., 2017), Parkinson’s disease (Shu et al., 2019), and cerebrovascular disease (Liao et al., 2019).
The imbalance between production and degradation of Aβ leads to the formation of senile plaques, which have deleterious effects on brain regions, especially the temporal cortex (Blomqvist et al., 2010). Previous studies have identified that variants of genes involved in the degradation of Aβ were associated with AD. SNPs rs989692 and rs3736187 in NEP differed significantly between AD and cognitively healthy individuals in a Finnish population, suggesting that NEP could increase the risk of AD (Helisalmi et al., 2004). The rare variants of ECE1 were nominally associated with AD after Bonferroni correction, indicating that ECE1 may increase the susceptibility to AD (Sassi et al., 2016). We previously identified that another novel Aβ degradation gene, ECE2, was a risk factor for AD development (Liao et al., 2020). In this study, the rare variants of NEP and Aβ degradation pathway were nominally significantly different between sLOAD and cognitively healthy individuals, which indicates the potentially significant roles of the rare variants of Aβ degradation pathway in the pathogenesis of sLOAD.
The fly orthologs of FERMT2 (fit1/fit2) were identified as tau modifiers in a Drosophila model of AD (Shulman et al., 2014). SNP rs17125944 in FERMT2 was associated with tau pathology in patients with AD (Farfel et al., 2016). The gene-based association test revealed that the non-synonymous variants of FERMT2 were also associated with LOAD (Beecham et al., 2018). The loci of the BIN1 gene were significantly associated with cerebrospinal fluid T-tau and P-tau levels, suggesting that BIN1 plays an important role in tau pathology (Wang et al., 2016c). Moreover, different GWASs identified that BIN1 and FERMT2 increased the susceptibility to LOAD (Sun et al., 2017). The rare variants of FERMT2 and the pathway of tau pathology reached the nominal significance in our study, further suggesting the significance of genes involved in tau pathology in the pathogenesis of sLOAD.
A decrease in PP2A levels is associated with tau hyperphosphorylation. Immunoblotting analysis of AD autopsy cases revealed a significant decrease of PP2A levels in the frontal and temporal cortices (Sontag et al., 2004). Vázquez-Higuera et al. (2011) found that several SNPs of genes involved in the PP2A pathway, including PPP2CA, PPP2R2A, ANP32A, LCMT1, PPME1, and PIN1, were not related to the risk of AD. However, in the Chinese population, SNP rs2287839 in the PIN1 promoter was significantly associated with the delayed onset of AD (Ma et al., 2012). Our study revealed that the rare variants in the pathway of tau phosphatase were nominally significantly correlated with sLOAD, suggesting their potentially important role in the development of sLOAD. In addition, we found that the frequencies of rare variants associated with Aβ and tau metabolism were similar between our study and the “Chinese AD Exome” (Xu et al., 2018; Zhang et al., 2019a). This indicates that rare variants exhibited high consistency in the Chinese population.
In summary, our study revealed that SNP rs11682128 in BIN1 was significantly associated with the risk of sLOAD, suggesting its significance in sLOAD development. Besides, the rare variants of NEP and FERMT2 as well as the pathways of Aβ degradation, tau pathology, and tau phosphatase were nominally associated with sLOAD, indicating that they may be the important risk factors for sLOAD.
Data Availability Statement
The raw data supporting the conclusions of this article will be made available by the authors, without undue reservation.
Ethics Statement
The studies involving human participants were reviewed and approved by the Ethics Committee of Xiangya Hospital of the Central South University. The patients/participants provided their written informed consent to participate in this study.
Author Contributions
XX, BJ, and LS: study design, acquisition of data, analysis and interpretation of data, and drafting/revising the manuscript. XLia, WZ, ZY, and LG: analysis and interpretation of data. XW, LZ, and XLiu: collecting patients and clinical assessment. XY and BT: review and editing the manuscript. All authors contributed to the article and approved the submitted version.
Funding
This study was supported by the National Natural Science Foundation of China (Nos. 81671075 and 81971029 to LS; No. 81701134 to BJ; and No. 81901171 to XLia), the National Key R&D Program of China (Nos. 2017YFC0840100 and 2017YFC0840104 to LS), Provincial Technology Innovation Guidance Plan Project of Hunan (No. 2018SK52601 to BJ), and The Youth Science Foundation of Xiangya Hospital (2018Q020 to XLia). The i-Select chips was funded by the French National Foundation on AD and related disorders. EADI was supported by the LABEX (Laboratory of Excellence Program Investment for the Future) DISTALZ grant, Inserm, Institut Pasteur de Lille, Université de Lille 2, and the Lille University Hospital. GERAD was supported by the Medical Research Council (Grant No. 503480), Alzheimer’s Research UK (Grant No. 503176), the Wellcome Trust (Grant No. 082604/2/07/Z), and German Federal Ministry of Education and Research (BMBF): Competence Network Dementia (CND) Grant Nos. 01GI0102, 01GI0711, and 01GI0420. CHARGE was partly supported by the NIH/NIA grant R01 AG033193 and the NIA AG081220 and AGES contract N01-AG-12100, the NHLBI grant R01 HL105756, the Icelandic Heart Association, and the Erasmus Medical Center and Erasmus University. ADGC was supported by the NIH/NIA grants U01 AG032984, U24 AG021886, and U01 AG016976, and the Alzheimer’s Association grant ADGC-10-196728.
Conflict of Interest
The authors declare that the research was conducted in the absence of any commercial or financial relationships that could be construed as a potential conflict of interest.
Acknowledgments
We are grateful to all subjects for their participation in our study. We thank Yuping Liu and Yanbo Liu for technical assistance. We also thank the IGAP for providing summary results data for these analyses. The investigators within IGAP contributed to the design and implementation of IGAP and/or provided data but did not participate in analysis or writing of this report. IGAP was made possible by the generous participation of the control subjects, the patients, and their families.
Supplementary Material
The Supplementary Material for this article can be found online at: https://www.frontiersin.org/articles/10.3389/fnagi.2020.584801/full#supplementary-material
Footnotes
- ^ http://bio-bwa.sourceforge.net
- ^ http://broadinstitute.github.io/picard/
- ^ https://software.broadinstitute.org/gatk/
- ^ https://hpc.nih.gov/apps/ANNOVAR.html
- ^ http://www.hgvs.org/
- ^ https://training.cochrane.org/online-learning/core-software-cochrane-reviews/revman/revman-5-download
References
Baranello, R. J., Bharani, K. L., Padmaraju, V., Chopra, N., Lahiri, D. K., Greig, N. H., et al. (2015). Amyloid-beta protein clearance and degradation (ABCD) pathways and their role in Alzheimer’s disease. Curr. Alzheimer Res. 12, 32–46. doi: 10.2174/1567205012666141218140953
Barrett, J. C., Fry, B., Maller, J., and Daly, M. J. (2005). Haploview: analysis and visualization of LD and haplotype maps. Bioinformatics 21, 263–265. doi: 10.1093/bioinformatics/bth457
Beecham, G. W., Vardarajan, B., Blue, E., Bush, W., Jaworski, J., Barral, S., et al. (2018). Rare genetic variation implicated in non-Hispanic white families with Alzheimer disease. Neurol. Genet. 4:e286. doi: 10.1212/nxg.0000000000000286
Blomqvist, M. E., McCarthy, S., Blennow, K., Andersson, B., and Prince, J. A. (2010). Evaluation of neprilysin sequence variation in relation to CSF beta-Amyloid levels and Alzheimer disease risk. Int. J. Mol. Epidemiol. Genet. 1, 47–52.
Calafate, S., Flavin, W., Verstreken, P., and Moechars, D. (2016). Loss of Bin1 promotes the propagation of tau pathology. Cell Rep. 17, 931–940. doi: 10.1016/j.celrep.2016.09.063
Chapuis, J., Hansmannel, F., Gistelinck, M., Mounier, A., Van Cauwenberghe, C., Kolen, K. V., et al. (2013). Increased expression of BIN1 mediates Alzheimer genetic risk by modulating tau pathology. Mol. Psychiatry 18, 1225–1234. doi: 10.1038/mp.2013.1
Clarimon, J., Munoz, F. J., Boada, M., Tarraga, L., Sunyer, J., Bertranpetit, J., et al. (2003). Possible increased risk for Alzheimer’s disease associated with neprilysin gene. J. Neural Transm. 110, 651–657. doi: 10.1007/s00702-002-0807-3
Cruchaga, C., Del-Aguila, J. L., Saef, B., Black, K., Fernandez, M. V., Budde, J., et al. (2018). Polygenic risk score of sporadic late-onset Alzheimer’s disease reveals a shared architecture with the familial and early-onset forms. Alzheimers Dement. 14, 205–214. doi: 10.1016/j.jalz.2017.08.013
den Dunnen, J. T., Dalgleish, R., Maglott, D. R., Hart, R. K., Greenblatt, M. S., McGowan-Jordan, J., et al. (2016). HGVS recommendations for the description of sequence variants: 2016 update. Hum. Mutat. 37, 564–569. doi: 10.1002/humu.22981
Dorszewska, J., Prendecki, M., Oczkowska, A., Dezor, M., and Kozubski, W. (2016). Molecular basis of familial and sporadic Alzheimer’s disease. Curr. Alzheimer Res. 13, 952–963. doi: 10.2174/1567205013666160314150501
Farfel, J. M., Yu, L., Buchman, A. S., Schneider, J. A., De Jager, P. L., and Bennett, D. A. (2016). Relation of genomic variants for Alzheimer disease dementia to common neuropathologies. Neurology 87, 489–496. doi: 10.1212/wnl.0000000000002909
Franzmeier, N., Rubinski, A., Neitzel, J., and Ewers, M. (2019). The BIN1 rs744373 SNP is associated with increased tau-PET levels and impaired memory. Nat. Commun. 10:1766.
Gomes, L. A., Hipp, S. A., Rijal Upadhaya, A., Balakrishnan, K., Ospitalieri, S., Koper, M. J., et al. (2019). Abeta-induced acceleration of Alzheimer-related tau-pathology spreading and its association with prion protein. Acta Neuropathol. 138, 913–941. doi: 10.1007/s00401-019-02053-5
Hardy, J., and Selkoe, D. J. (2002). The amyloid hypothesis of Alzheimer’s disease: progress and problems on the road to therapeutics. Science 297, 353–356. doi: 10.1126/science.1072994
Helisalmi, S., Hiltunen, M., Vepsalainen, S., Iivonen, S., Mannermaa, A., Lehtovirta, M., et al. (2004). Polymorphisms in neprilysin gene affect the risk of Alzheimer’s disease in Finnish patients. J. Neurol. Neurosurg. Psychiatry 75, 1746–1748. doi: 10.1136/jnnp.2004.036574
Ionita-Laza, I., McCallum, K., Xu, B., and Buxbaum, J. D. (2016). A spectral approach integrating functional genomic annotations for coding and noncoding variants. Nat. Genet. 48, 214–220. doi: 10.1038/ng.3477
Jiao, B., Liu, X., Zhou, L., Wang, M. H., Zhou, Y., Xiao, T., et al. (2015). Polygenic analysis of late-onset Alzheimer’s disease from Mainland China. PLoS One 10:e0144898. doi: 10.1371/journal.pone.0144898
Jiao, B., Tang, B., Liu, X., Xu, J., Wang, Y., Zhou, L., et al. (2014). Mutational analysis in early-onset familial Alzheimer’s disease in Mainland China. Neurobiol. Aging 35, 1957.e1–1957.e6. doi: 10.1016/j.neurobiolaging.2014.02.014
Kametani, F., and Hasegawa, M. (2018). Reconsideration of amyloid hypothesis and tau hypothesis in Alzheimer’s disease. Front. Neurosci. 12:25. doi: 10.3389/fnins.2018.00025
Kunkle, B. W., Grenier-Boley, B., Sims, R., Bis, J. C., Damotte, V., Naj, A. C., et al. (2019). Genetic meta-analysis of diagnosed Alzheimer’s disease identifies new risk loci and implicates Abeta, tau, immunity and lipid processing. Nat. Genet. 51, 414–430.
Lambert, J. C., Ibrahim-Verbaas, C. A., Harold, D., Naj, A. C., Sims, R., Bellenguez, C., et al. (2013). Meta-analysis of 74,046 individuals identifies 11 new susceptibility loci for Alzheimer’s disease. Nat. Genet. 45, 1452–1458. doi: 10.1038/ng.2802
Lee, S., Emond, M. J., Bamshad, M. J., Barnes, K. C., Rieder, M. J., Nickerson, D. A., et al. (2012). Optimal unified approach for rare-variant association testing with application to small-sample case-control whole-exome sequencing studies. Am. J. Hum. Genet. 91, 224–237. doi: 10.1016/j.ajhg.2012.06.007
Li, H., and Durbin, R. (2010). Fast and accurate long-read alignment with Burrows-Wheeler transform. Bioinformatics 26, 589–595. doi: 10.1093/bioinformatics/btp698
Li, K. C., Luo, X., Zeng, Q. Z., Xu, X. J., Huang, P. Y., Shen, Z. J., et al. (2018). Distinct patterns of interhemispheric connectivity in patients with early- and late-onset Alzheimer’s disease. Front. Aging Neurosci. 10:261. doi: 10.3389/fnagi.2018.00261
Liao, X., Cai, F., Sun, Z., Zhang, Y., Wang, J., Jiao, B., et al. (2020). Identification of Alzheimer’s disease-associated rare coding variants in the ECE2 gene. JCI Insight 5:e135119. doi: 10.1172/jci.insight.135119
Liao, X., Zhang, T., Li, B., Hu, S., Liu, J., Deng, J., et al. (2019). Rare RNF213 variants and the risk of intracranial artery stenosis/occlusion disease in Chinese population: a case-control study. BMC Med. Genet. 20:55. doi: 10.1186/s12881-019-0788-9
Liu, C. D., Wang, Q., Zong, D. K., Pei, S. C., Yan, Y., Yan, M. L., et al. (2016). Knockdown of microRNA-195 contributes to protein phosphatase-2A inactivation in rats with chronic brain hypoperfusion. Neurobiol. Aging 45, 76–87. doi: 10.1016/j.neurobiolaging.2016.05.010
Liu, C. X., Tan, L., Sun, F. R., Zhang, W., Miao, D., Tan, M. S., et al. (2017). The association of MME microRNA binding site polymorphism with the risk of late onset Alzheimer’s disease in Northern Han Chinese. Curr. Neurovasc. Res. 14, 90–95. doi: 10.2174/1567202614666170313110301
Lu, Q., Hu, Y., Sun, J., Cheng, Y., Cheung, K. H., and Zhao, H. (2015). A statistical framework to predict functional non-coding regions in the human genome through integrated analysis of annotation data. Sci. Rep. 5:10576. doi: 10.1038/srep10576
Ma, S. L., Tang, N. L., Tam, C. W., Lui, V. W., Lam, L. C., Chiu, H. F., et al. (2012). A PIN1 polymorphism that prevents its suppression by AP4 associates with delayed onset of Alzheimer’s disease. Neurobiol. Aging 33, 804–813. doi: 10.1016/j.neurobiolaging.2010.05.018
Martin, L., Latypova, X., Wilson, C. M., Magnaudeix, A., Perrin, M. L., Yardin, C., et al. (2013). Tau protein kinases: involvement in Alzheimer’s disease. Ageing Res. Rev. 12, 289–309. doi: 10.1016/j.arr.2012.06.003
Mawuenyega, K. G., Sigurdson, W., Ovod, V., Munsell, L., Kasten, T., Morris, J. C., et al. (2010). Decreased clearance of CNS beta-amyloid in Alzheimer’s disease. Science 330:1774. doi: 10.1126/science.1197623
McKenna, A., Hanna, M., Banks, E., Sivachenko, A., Cibulskis, K., Kernytsky, A., et al. (2010). The genome analysis toolkit: a MapReduce framework for analyzing next-generation DNA sequencing data. Genome Res. 20, 1297–1303. doi: 10.1101/gr.107524.110
McKhann, G. M., Knopman, D. S., Chertkow, H., Hyman, B. T., Jack, C. R. Jr., Kawas, C. H., et al. (2011). The diagnosis of dementia due to Alzheimer’s disease: recommendations from the national institute on aging-Alzheimer’s association workgroups on diagnostic guidelines for Alzheimer’s disease. Alzheimers Dement. 7, 263–269. doi: 10.1016/j.jalz.2011.03.005
Miners, S., van Helmond, Z., Barker, R., Passmore, P. A., Johnston, J. A., Todd, S., et al. (2012). Genetic variation in MME in relation to neprilysin protein and enzyme activity, Abeta levels, and Alzheimer’s disease risk. Int. J. Mol. Epidemiol. Genet. 3, 30–38.
Morris, A. P., and Zeggini, E. (2010). An evaluation of statistical approaches to rare variant analysis in genetic association studies. Genet. Epidemiol. 34, 188–193. doi: 10.1002/gepi.20450
Natunen, T., Helisalmi, S., Vepsäläinen, S., Sarajärvi, T., Antikainen, L., Mäkinen, P., et al. (2012). Genetic analysis of genes involved in amyloid-β degradation and clearance in Alzheimer’s disease. J. Alzheimers Dis. 28, 553–559. doi: 10.3233/jad-2011-111109
Neale, B. M., Rivas, M. A., Voight, B. F., Altshuler, D., Devlin, B., Orho-Melander, M., et al. (2011). Testing for an unusual distribution of rare variants. PLoS Genet. 7:e1001322. doi: 10.1371/journal.pgen.1001322
Nho, K., Kim, S., Horgusluoglu, E., Risacher, S. L., Shen, L., Kim, D., et al. (2017). Association analysis of rare variants near the APOE region with CSF and neuroimaging biomarkers of Alzheimer’s disease. BMC Med. Genomics 10(Suppl. 1):29. doi: 10.1186/s12920-017-0267-0
Oliveira, J., Costa, M., de Almeida, M. S. C., da Cruz, E. S. O. A. B., and Henriques, A. G. (2017). Protein Phosphorylation is a Key Mechanism in Alzheimer’s Disease. J. Alzheimers Dis. 58, 953–978. doi: 10.3233/jad-170176
Patterson, C. (2018). World Alzheimer Report 2018. The State of the Art of Dementia Research: New Frontiers. An Analysis of Prevalence, Incidence, Cost and Trends. London: Alzheimer’s Disease International.
Prokic, I., Cowling, B. S., and Laporte, J. (2014). Amphiphysin 2 (BIN1) in physiology and diseases. J. Mol. Med. 92, 453–463. doi: 10.1007/s00109-014-1138-1
Purcell, S., Neale, B., Todd-Brown, K., Thomas, L., Ferreira, M. A., Bender, D., et al. (2007). PLINK: a tool set for whole-genome association and population-based linkage analyses. Am. J. Hum. Genet. 81, 559–575. doi: 10.1086/519795
Saez-Atienzar, S., and Masliah, E. (2020). Cellular senescence and Alzheimer disease: the egg and the chicken scenario. Nat. Rev. Neurosci. 21, 433–444. doi: 10.1038/s41583-020-0325-z
Saido, T., and Leissring, M. A. (2012). Proteolytic degradation of amyloid beta-protein. Cold Spring Harb. Perspect. Med. 2:a006379. doi: 10.1101/cshperspect.a006379
Sassi, C., Ridge, P. G., Nalls, M. A., Gibbs, R., Ding, J., Lupton, M. K., et al. (2016). Influence of coding variability in APP-Abeta metabolism genes in sporadic Alzheimer’s disease. PLoS One 11:e0150079. doi: 10.1371/journal.pone.0150079
Scheltens, P., Blennow, K., Breteler, M. M., de Strooper, B., Frisoni, G. B., Salloway, S., et al. (2016). Alzheimer’s disease. Lancet 388, 505–517. doi: 10.1016/S0140-6736(15)01124-1
Seshadri, S., Fitzpatrick, A. L., Ikram, M. A., DeStefano, A. L., Gudnason, V., Boada, M., et al. (2010). Genome-wide analysis of genetic loci associated with Alzheimer disease. JAMA 303, 1832–1840. doi: 10.1001/jama.2010.574
Shentu, Y. P., Huo, Y., Feng, X. L., Gilbert, J., Zhang, Q., Liuyang, Z. Y., et al. (2018). CIP2A causes Tau/APP phosphorylation, synaptopathy, and memory deficits in Alzheimer’s disease. Cell Rep. 24, 713–723. doi: 10.1016/j.celrep.2018.06.009
Shu, L., Qin, L., Min, S., Pan, H., Zhong, J., Guo, J., et al. (2019). Genetic analysis of DNA methylation and hydroxymethylation genes in Parkinson’s disease. Neurobiol. Aging 84, 242.e13–242.e16. doi: 10.1016/j.neurobiolaging.2019.02.025
Shulman, J. M., Imboywa, S., Giagtzoglou, N., Powers, M. P., Hu, Y., Devenport, D., et al. (2014). Functional screening in Drosophila identifies Alzheimer’s disease susceptibility genes and implicates Tau-mediated mechanisms. Hum. Mol. Genet. 23, 870–877. doi: 10.1093/hmg/ddt478
Sontag, E., Luangpirom, A., Hladik, C., Mudrak, I., Ogris, E., Speciale, S., et al. (2004). Altered expression levels of the protein phosphatase 2A ABalphaC enzyme are associated with Alzheimer disease pathology. J. Neuropathol. Exp. Neurol. 63, 287–301. doi: 10.1093/jnen/63.4.287
Stanevich, V., Jiang, L., Satyshur, K. A., Li, Y., Jeffrey, P. D., Li, Z., et al. (2011). The structural basis for tight control of PP2A methylation and function by LCMT-1. Mol. Cell 41, 331–342. doi: 10.1016/j.molcel.2010.12.030
Sun, Q., Xie, N., Tang, B., Li, R., and Shen, Y. (2017). Alzheimer’s disease: from genetic variants to the distinct pathological mechanisms. Front. Mol. Neurosci. 10:319. doi: 10.3389/fnmol.2017.00319
Szklarczyk, D., Gable, A. L., Lyon, D., Junge, A., Wyder, S., Huerta-Cepas, J., et al. (2019). STRING v11: protein-protein association networks with increased coverage, supporting functional discovery in genome-wide experimental datasets. Nucleic Acids Res. 47, D607–D613. doi: 10.1093/nar/gky1131
Vázquez-Higuera, J. L., Mateo, I., Sanchez-Juan, P., Rodriguez-Rodriguez, E., Pozueta, A., Calero, M., et al. (2011). Genetic variation in the tau protein phosphatase-2A pathway is not associated with Alzheimer’s disease risk. BMC Res. Notes 4:327. doi: 10.1186/1756-0500-4-327
Wang, F., Shu, C., Jia, L., Zuo, X., Zhang, Y., Zhou, A., et al. (2012). Exploration of 16 candidate genes identifies the association of IDE with Alzheimer’s disease in Han Chinese. Neurobiol. Aging 33, 1014.e1–1014.e9. doi: 10.1016/j.neurobiolaging.2010.08.004
Wang, H. Z., Bi, R., Zhang, D. F., Li, G. D., Ma, X. H., Fang, Y., et al. (2016a). Neprilysin confers genetic susceptibility to Alzheimer’s disease in Han Chinese. Mol. Neurobiol. 53, 4883–4892. doi: 10.1007/s12035-015-9411-z
Wang, H. Z., Bi, R., Hu, Q. X., Xiang, Q., Zhang, C., Zhang, D. F., et al. (2016b). Validating GWAS-identified risk loci for Alzheimer’s disease in Han Chinese populations. Mol. Neurobiol. 53, 379–390. doi: 10.1007/s12035-014-9015-z
Wang, H. F., Wan, Y., Hao, X. K., Cao, L., Zhu, X. C., Jiang, T., et al. (2016c). Bridging integrator 1 (BIN1) genotypes mediate Alzheimer’s disease risk by altering neuronal degeneration. J. Alzheimers Dis. 52, 179–190. doi: 10.3233/jad-150972
Wang, K., Li, M., and Hakonarson, H. (2010). ANNOVAR: functional annotation of genetic variants from high-throughput sequencing data. Nucleic Acids Res. 38:e164. doi: 10.1093/nar/gkq603
Wang, Z., Xu, Q., Cai, F., Liu, X., Wu, Y., and Song, W. (2019). BACE2, a conditional beta-secretase, contributes to Alzheimer’s disease pathogenesis. JCI Insight 4:e123431. doi: 10.1172/jci.insight.123431
Wood, L. S., Pickering, E. H., McHale, D., and Dechairo, B. M. (2007). Association between neprilysin polymorphisms and sporadic Alzheimer’s disease. Neurosci. Lett. 427, 103–106. doi: 10.1016/j.neulet.2007.09.019
Wu, M. C., Lee, S., Cai, T., Li, Y., Boehnke, M., and Lin, X. (2011). Rare-variant association testing for sequencing data with the sequence kernel association test. Am. J. Hum. Genet. 89, 82–93. doi: 10.1016/j.ajhg.2011.05.029
Xu, M., Zhang, D. F., Luo, R., Wu, Y., Zhou, H., Kong, L. L., et al. (2018). A systematic integrated analysis of brain expression profiles reveals YAP1 and other prioritized hub genes as important upstream regulators in Alzheimer’s disease. Alzheimers Dement. 14, 215–229. doi: 10.1016/j.jalz.2017.08.012
Zhang, D. F., Fan, Y., Xu, M., Wang, G., Wang, D., Li, J., et al. (2019a). Complement C7 is a novel risk gene for Alzheimer’s disease in Han Chinese. Natl. Sci. Rev. 6, 257–274. doi: 10.1093/nsr/nwy127
Zhang, D. F., Xu, M., Bi, R., and Yao, Y. G. (2019b). Genetic analyses of Alzheimer’s disease in China: achievements and perspectives. ACS Chem. Neurosci. 10, 890–901. doi: 10.1021/acschemneuro.8b00435
Zhang, X., Yu, J. T., Li, J., Wang, C., Tan, L., Liu, B., et al. (2015). Bridging integrator 1 (BIN1) genotype effects on working memory, hippocampal volume, and functional connectivity in young healthy individuals. Neuropsychopharmacology 40, 1794–1803. doi: 10.1038/npp.2015.30
Zhou, L., and Zhao, F. (2018). Prioritization and functional assessment of noncoding variants associated with complex diseases. Genome Med. 10:53. doi: 10.1186/s13073-018-0565-y
Keywords: Alzheimer’s disease, Chinese, amyloid-β, tau, metabolism
Citation: Xiao X, Jiao B, Liao X, Zhang W, Yuan Z, Guo L, Wang X, Zhou L, Liu X, Yan X, Tang B and Shen L (2020) Association of Genes Involved in the Metabolic Pathways of Amyloid-β and Tau Proteins With Sporadic Late-Onset Alzheimer’s Disease in the Southern Han Chinese Population. Front. Aging Neurosci. 12:584801. doi: 10.3389/fnagi.2020.584801
Received: 18 July 2020; Accepted: 13 October 2020;
Published: 06 November 2020.
Edited by:
Ramesh Kandimalla, Texas Tech University Health Sciences Center, United StatesReviewed by:
Yong-Gang Yao, Kunming Institute of Zoology, ChinaJianping Jia, Capital Medical University, China
Deng-Feng Zhang, Kunming Institute of Zoology, Chinese Academy of Sciences, China
Copyright © 2020 Xiao, Jiao, Liao, Zhang, Yuan, Guo, Wang, Zhou, Liu, Yan, Tang and Shen. This is an open-access article distributed under the terms of the Creative Commons Attribution License (CC BY). The use, distribution or reproduction in other forums is permitted, provided the original author(s) and the copyright owner(s) are credited and that the original publication in this journal is cited, in accordance with accepted academic practice. No use, distribution or reproduction is permitted which does not comply with these terms.
*Correspondence: Lu Shen, shenlu@csu.edu.cn