- 1Department of Psychiatry and Psychotherapy, University Medical Center Mainz, Mainz, Germany
- 2Center for Mental Health in Old Age & the German AgeGain Study Group, Mainz, Germany
- 3German Sport University Cologne, Institute of Movement and Neurosciences, Cologne, Germany
- 4Leibnitz Institute for Resilience Research (LIR), Mainz, Germany
- 5German Center for Neurodegenerative Diseases (DZNE), Rostock, Germany
- 6Department for Psychosomatic and Psychotherapeutical Medicine, University Hospital Rostock, Rostock, Germany
- 7Institute of Medical Biometry and Statistics (IMBI), University of Freiburg, Freiburg, Germany
- 8Department of Exercise and Sport Science, LUNEX International University of Health, Exercise and Sports, Differdange, Luxembourg
Cognition emerges from coordinated processing among distributed cortical brain regions, enabled through interconnected white matter networks. Cortical disconnection caused by age-related decline in white matter integrity (WMI) is likely to contribute to age-related cognitive decline. Physical activity (PA) has been suggested to have beneficial effects on white matter structure. However, its potential to counteract age-related decline in WMI is not yet well established. The present explorative study analyzed if PA was associated with WMI in cognitively healthy older adults and if this association was modulated by age. Forty-four cognitively healthy older individuals (aged 60–88 years) with diffusion-tensor imaging (DTI) and PA measurements were included from the AgeGain study. Voxelwise analysis using Tract-Based Spatial Statistics (TBSS) demonstrated that PA was associated with WMI in older adults. However, results emphasized that this association was restricted to high age. The association between PA and WMI was found in widespread white matter regions suggesting a global rather than a regional effect. Supplementary analyses demonstrated an association between the integrity of these regions and the performance in memory [verbal learning and memory test (VLMT)] and executive functioning (Tower of London).Results of the present explorative study support the assumption that PA is associated with WMI in older adults. However, results emphasize that this association is restricted to high age. Since cognitive decline in the elderly is typically most pronounced in later stages of aging, PA qualifies as a promising tool to foster resilience against age-related cognitive decline, via the preservation of the integrity of the brains WM.
Introduction
Normal aging is characterized by a cognitive decline in several cognitive domains, including memory, information processing speed, reasoning, spatial orientation, and numeric abilities (Salthouse, 2010; Park and Schwarz, 2012). However, the interindividual variability of age-related cognitive changes is high, ranging from clinically apparent reduction to far-reaching preservation of cognitive functioning (Wilson et al., 2002). Cognitive health has been quoted as a major factor for life quality in the elderly and contributes greatly to late-life functioning and independence (Depp and Jeste, 2006; Reichstadt et al., 2007). This significance of cognitive health highlights the importance of a profound understanding of the preservation of cognitive functioning in aging.
Cognition relies on the coordinated processing among distributed cortical brain regions, enabled through interconnected white matter networks. Cortical disconnection has been shown to lead to cognitive dysfunction (Catani and ffytche, 2005; Filley, 2005). Cortical disconnection is also regarded as a key concept in age-related cognitive decline since several diffusion-tensor imaging (DTI) studies indicate that the integrity of white matter networks typically declines in healthy aging and that cognitive abilities in aging are associated with the integrity of white matter networks (Madden et al., 2009, 2012; Bennett and Madden, 2014). In line with the variance in age-related cognitive decline, studies have also highlighted substantial between-subject variance in age-related changes in white matter integrity (WMI; Madden et al., 2012; Bennett and Madden, 2014; Sexton et al., 2014). Thus, the preservation of WMI is a promising approach to prevent cortical disconnection and maintain cognitive abilities within the aging process.
Concerning this, higher levels of physical activity (PA) have been shown to have beneficial effects on various structural properties in the brain in older adults, especially on gray matter volume (Bherer et al., 2013; Erickson et al., 2014). A growing number of studies now focuses on the investigation of the effects of PA on white matter properties (Sexton et al., 2016). In addition to repeated findings of an association between PA and white matter volume as well as white matter lesions (Sexton et al., 2016), many studies reported a positive effect of PA on WMI (Gow et al., 2012; Johnson et al., 2012; Liu et al., 2012; Tseng et al., 2013a). PA thus qualifies as a promising tool to counteract the age-related decline in WMI and to preserve cognition functioning via the association with WMI. However, several negative findings on the association between PA and WMI have also been published (Voss et al., 2013; Burzynska et al., 2014a; Tian et al., 2014). Hence, the effect of PA on WMI has not yet been well established.
It has been suggested that greater levels of PA may particularly be beneficial in later life since a decline in white matter microstructure is most pronounced in high age. This may contribute to the heterogeneity in study results and highlights the necessity of a better understanding of the trajectory of the association between PA and WMI with age (Sexton et al., 2016). The present explorative study aimed at investigating if PA is associated with WMI in healthy elderly and if this association is modulated by age. In supplementary analyses, the study also analyzed if the integrity of white matter regions that were associated with PA was also related to cognitive performance.
Materials and Methods
Data Source and Subjects
Data used in the present article were obtained from the AgeGain study (Wolf et al., 2018). The multicenter trial AgeGain primarily aims at the investigation of mechanisms and modulators of transfer of cognitive training gains in cognitively healthy elderly. The main exclusion criteria were current (or history of) psychiatric, cognitive, neurological, or cardiovascular diseases, brain lesions, or secondary disorders restricting individuals’ physical capacity (i.e., chronic obstructive pulmonary disease). A full description of AgeGain, including details on the comprehensive database of the study as well as detailed information on in-and exclusion criteria, can be found in the study protocol (Wolf et al., 2018). Data were taken from an interim subset of data. Baseline DTI-scans, actigraphy-based measures of PA, and cognitive data of 44 cognitively healthy older adults (age range: 60–88 years) were included.
Physical Activity
Physical activity was assessed using triaxial accelerometers (GENEActiv, ActivInsights Limited, Kimbolton, Cambridgeshire, UK). Participants were asked to wear the accelerometers on their non-dominant wrists 24 h a day. The devices were set to collect data at 100 Hz for seven consecutive days. The extracted raw data were processed using R package GGIR version 1.9-1 (Migueles et al., 2019).
The analyses included autocalibration (Van Hees et al., 2014), detection and imputation of non-wear time as well as the extraction of the mean Euclidean Norm minus 1 (ENMO) for the acquisition period of seven consecutive days (Van Hees et al., 2013). The ENMO metric is computed from the resultant vector of the measured orthogonal acceleration and adjusts for gravity via subtracting a fixed offset of one gravitational unit from the Euclidean Norm of the three raw acceleration signals. The ENMO metric reflects the mean overall physical activity (Migueles et al., 2019) and correlates with physical activity-related energy expenditure (Van Hees et al., 2013). Its application has become common even in large scale studies (Doherty et al., 2017; Menai et al., 2017; Sanders et al., 2019).
Neuropsychology
The performance of the cognitive domains memory, executive function, and information processing speed was assessed. All three domains are typically affected in aging.
Memory performance was measured using the verbal learning and memory test (VLMT), a German version of the auditory verbal learning test, which requires learning of a list of 15 words in five consecutive trials, free recall of these words after each trial, and recognition after 20 min. The performance was rated according to the sum of correct remembered words of all five trials (VLMT 1–5) and correct recognized words after 20 min (VLMT recognition).
Executive function was measured using the Tower of London task (ToL), which is a measure of planning and problem-solving. The ToL test consists of a set of three pegs on a wooden base and three differently colored balls which can be moved on the pegs. A start configuration of the balls has to be transformed into a given goal configuration while considering the following rules: only one ball can be moved at a time, a ball is not allowed to be placed next to a peg, and only the topmost ball of each peg can be moved. One peg can hold a maximum of three balls, one takes a maximum of two balls and the third takes only one ball. Subjects are instructed to solve a given problem in the minimum number of moves. Trials included problems, where three- to six moves were required to solve the task. The performance was rated based on the number of correctly solved problems.
Information processing speed was assessed by the trail-making-test A (TMT-A). The test requires an individual to draw lines sequentially connecting 25 encircled numbers distributed on a sheet of paper. Participants were instructed to complete the task as quickly and accurately as possible. In case of an error, participants were instructed to return to the circle where the error occurred and continue. The performance was rated according to the response time.
Imaging Data Acquisition and Processing
DWI imaging data were acquired on Siemens Magnetom Trio and Verio 3T scanners using a multiband sequence with a voxel size of 2 × 2 × 2 mm3, 64 diffusion gradients, a multiband factor of 3, and b-values of 0 and 2,000 s/mm2. For distortion correction purposes, an additional b = 0 image with inverted phase encoding, i.e., posterior-anterior, was acquired. DWI data were corrected for eddy current and susceptibility induced artifacts and distortions using “FSL” (Jenkinson et al., 2012) and the included software eddy (Andersson and Sotiropoulos, 2016) as well as top-up (Andersson et al., 2003), which estimates a susceptibility induced off-resonance field using the phase inverted b = 0 image. Subsequently, we used “mrtrix” (Tournier et al., 2019) to fit a diffusion tensor to the data with a weighted linear least squares estimator. Finally, fractional anisotropy (FA) and mean diffusivity (MD) images were calculated from the eigensystem of the estimated diffusion tensors.
Data Analyses
We applied whole-brain voxel-wise regression analyses using Tract-Based Spatial Statistics (TBSS; Smith et al., 2006) to investigate the association between WMI (as quantified by FA and MD) and physical activity. TBSS preprocessing included the following steps: (i) nonlinear registration to the FMRIB58_FA template of all subjects’ FA data using FMRIB’s nonlinear image registration tool (Rueckert et al., 1999); (ii) creation and thinning of a mean FA image with a threshold of 0.2 to obtain a mean FA skeleton that represents the centers of white matter trajectories; and (iii) projection of each subjects’ aligned data onto the skeleton. To achieve skeletonized MD data, the nonlinear warps and skeleton projection vectors of the FA images were applied to the MD data. Regression analyses were performed using the randomize tool, which tested the t-value at each voxel against a null distribution that was obtained from 5,000 random permutations. To minimize the probability of false-positive voxels, analyses were corrected for multiple comparisons across voxels using the threshold-free cluster-enhancement option.
Initially, we tested the general association between WMI and physical activity (mean ENMO). Age was added as a control variable since it is associated with both, WMI and physical activity (Bennett and Madden, 2014; McPhee et al., 2016). Thereafter, we added the interaction term PA × age to the regression model to investigate if the association between physical activity and WMI was modulated by age. Significant regions on the TBSS skeleton were extracted as masks (separately for each regression model) and mean WMI was calculated for each mask. Mean WMI values were then used to characterize potential interaction effects.
Moreover, in explorative analyses, the association between mean WMI values and cognitive performance was investigated using robust regression analyses (based on an MM estimator). Age was added as a control variable since it is known to be associated with WMI and cognition (Salthouse, 2010; Bennett and Madden, 2014). Statistical analyses outside the TBSS framework were carried out using the statistical software package R 3.0.2.
Results
Descriptive Characteristics
Descriptive characteristics of the study group are summarized in Table 1. The mean age of the study group was 69.07 (SD, 7.3) years, 29 individuals (65.9%) were female. Participants were highly educated with a mean educational attainment of 15.43 (SD, 2.2) years. There was no difference in educational attainment between males and females. Education was not related to age. Age was negatively associated with PA (rPerason = −0.32, p = 0.04). Education and gender were not associated with PA. Moreover, age was negatively associated with short-term memory (rPearson = −0.31, p = 0.04) and executive functioning (rPearson = −0.34, p = 0.02), but not with long-term memory and information processing speed. Education and gender were not associated with any cognitive measure.
Association Between WMI and PA
Whole-brain voxel-wise TBSS regression analyses demonstrated no general association between PA and FA- or MD values. However, regression analyses demonstrated an interaction between PA and age on MD. This result indicates that the association between PA and MD is age-dependent. The interaction was found in widespread white matter regions suggesting a global rather than a regional effect (see Figure 1). A comparable interaction pattern between PA and age on FA values has been observed. However, results were less strong and did not reach statistical significance at p ≤ 0.05, corrected for multiple comparisons.
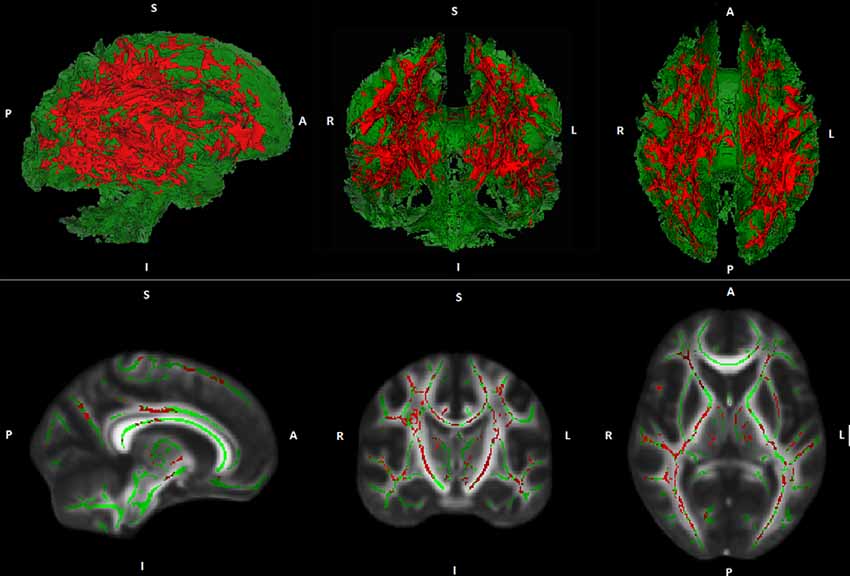
Figure 1. Illustration of Tract-based spatial statistic (TBSS) results. Interaction effects (red) between age and physical activity (PA) on white matter mean diffusivity (MD) are projected on a cerebral white matter skeleton (green; 3D above, 2D below).
Regions demonstrating significant interaction effects between PA and age on MD have been extracted as a separate mask and mean MD values within this mask have been calculated. A scatter plot to characterize the observed interaction indicated a stronger association between PA and mean MD with increasing age (see Figure 2; of note: for convenient visualization of the interaction effect, the association between PA and MD values has been plotted separately for younger- and advanced elderly, as defined by a median split. The median split has only been applied for illustration purposes. All statistical analyses were calculated based on continuous data).
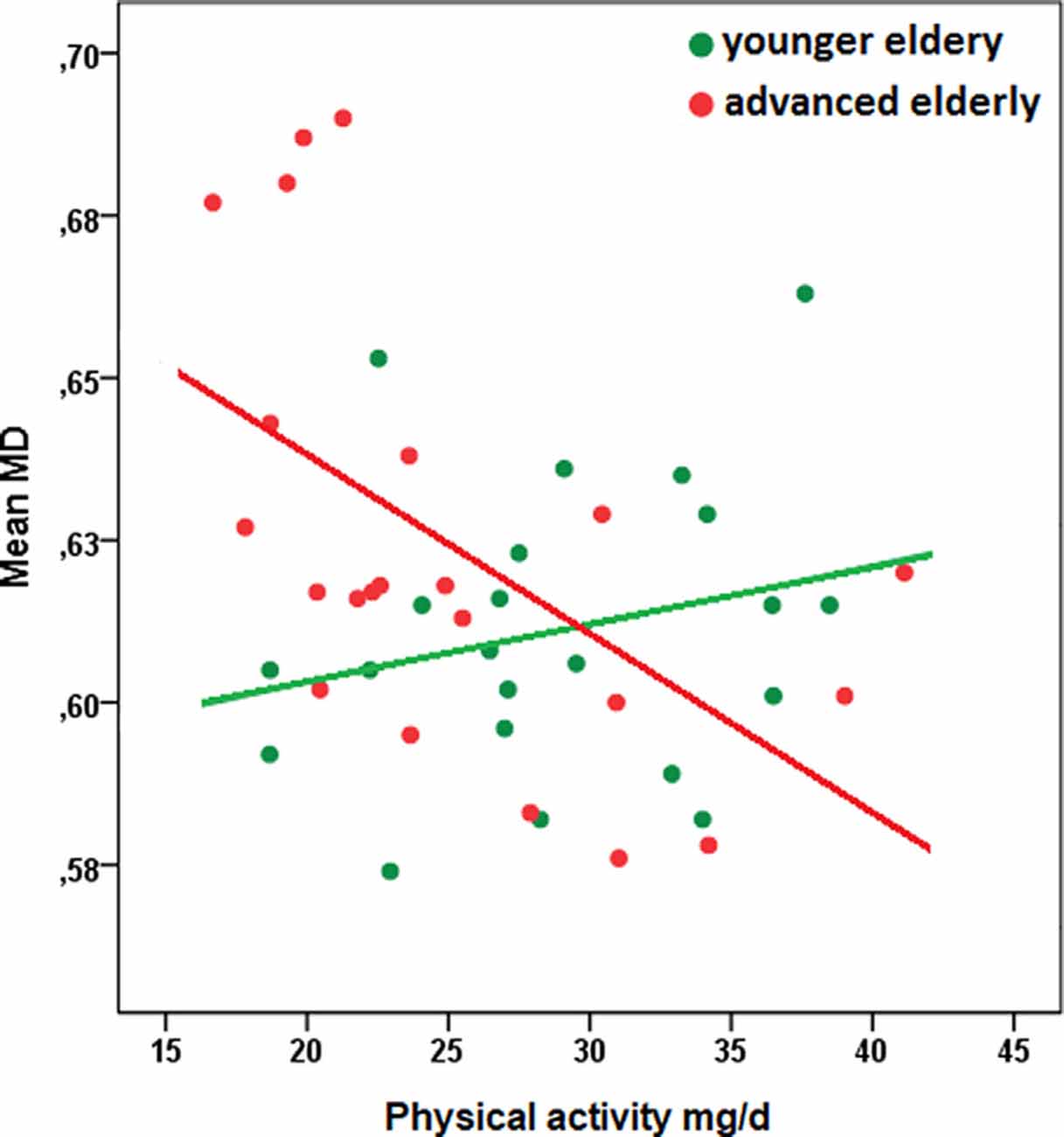
Figure 2. Scatterplot of the relationship between physical activity and mean MD of white matter regions that were associated with the interaction term PA × age, separated for younger- and advanced elderly defined by a median split (green: younger elderly, n = 22; red: advanced elderly, n = 22). The median split has been applied only for illustration purposes. All statistical analyses were calculated based on continuous data. Descriptive characteristics of subgroups: younger elderly, mean age in years (SD): 64.5 (1.7), woman (%): 13 (59.1), education in years: 15.5 (1.5), physical activity in mg/d: 28.8 (6.0); advanced elderly: mean age (SD): 74.6 (6.5), woman (%): 16 (72.7), education in years (SD): 15.4 (2.8), physical activity in mg/d: 25.2 (6.7); Pearson correlation coefficient between PA and mean MD in subgroups: younger elderly, r = 0.24, p = 0.28; advanced elderly, r = −.55, p = 0.01. Abbreviations: MD, mean diffusivity; ENMO, Euclidean Norm minus 1 in milligravity units per day.
Association Between Cognition and WMI of Regions Associated With PA
Supplementary robust regression analyses were applied to investigate the association between cognitive performance and mean MD values of the extracted mask (with age added as a covariate of no interest). A negative association could be observed between mean MD values and executive functioning (ToL: ß = −0.29, p = 0.03). Negative associations could also be observed between mean MD values and short- as well as long term memory. However, these associations did not reach statistical significance (VLMT 1–5: ß = −0.34, p = 0.09; VLMT recognition: ß = −0.18, p = 0.07). Information processing speed was not related to mean MD of the extracted mask (TMT-A: ß = −0.07, p = 0.59).
Discussion
The present explorative study aimed at increasing the understanding of the association between PA and WMI in cognitively healthy elderly. The study indicates that PA is associated with WMI in older adults. However, results emphasize that this association is restricted to higher age.
Over the last years, an increasing number of studies investigated the association between PA and WM measures in cognitively healthy elderly. Many of these studies found that higher levels of PA were related to greater WM volumes (Colcombe et al., 2006; Ho et al., 2011; Gow et al., 2012; Benedict et al., 2013; Tseng et al., 2013b) as well as reduced volume and severity of WM lesions (Sen et al., 2012; Wirth et al., 2014). Although some negative findings have also been published, meta-analyses could confirm the described results (Torres et al., 2015; Sexton et al., 2016). Studies on the relation between PA and WM microstructure are less frequent. In contrast to the mostly homogeneous results on the association between PA and WM volume as well as WM lesions, results on the relationship between PA and WM microstructure are heterogeneous. On the one hand, studies found positive associations between PA and global as well as regional WM FA in cognitively healthy elderly (Gow et al., 2012; Johnson et al., 2012; Liu et al., 2012; Tseng et al., 2013a). Moreover, negative associations between PA and regional WM MD have been reported (Tseng et al., 2013a). On the other hand, several studies did not find any correlations between PA and measures of WM microstructure (Voss et al., 2013; Burzynska et al., 2014b; Tian et al., 2014). Since advancing age is associated with accelerated decline in WM microstructure, it has been suggested that the association between PA and WM microstructure may be more pronounced in (or restricted to) advanced stages of aging (Sexton et al., 2016). The current study supports this hypothesis and thus provides important information for a better understanding of the largely unknown trajectory of the relationship between PA and WM microstructure in the process of aging. Of note, our results imply that the association between PA and WM microstructure is rather global instead of being locally restricted (see Figure 1).
PA as assessed using triaxial accelerometers over several days in everyday life can be seen as a measure of general activity level in the sense of a trait marker. The association between the general PA level and WMI may be mediated by various neurobiological mechanisms. First, a higher level of PA leads to a persistent upregulation of neurotrophic factors, especially of brain-derived neurotrophic factor (BDNF; Huang et al., 2014). BDNF has been shown to support synaptic plasticity and normal axonal pruning (Cao et al., 2007; Singh et al., 2008). Moreover, it has been observed that BDNF has a neuroprotective influence on white matter in both rodents and humans (Husson et al., 2005; Weinstock-Guttman et al., 2007). The association between PA and WMI may thus be mediated by upregulated BDNF in response to PA. Second, there is some evidence that suggests that higher levels of PA may lead to the proliferation of oligodendrocyte progenitor cells, which continue to form new oligodendrocytes and allow for continued myelination (Krityakiarana et al., 2010). Thus, the extent of axonal myelination and therewith the integrity of WM pathways may alter in response to PA, mediated by increased oligodendrocyte proliferation. Third, higher levels of PA have been shown to have beneficial effects on the vascular system, including the preservation of arterial elasticity and wall integrity, as well as a reduction in arterial stiffness and blood pressure (McDonnell et al., 2013). Moreover, some animal studies reported increased capillary density in the consequence of PA (Swain et al., 2003; Ding et al., 2006). Improved vascular health caused by PA may thus contribute to increased WMI via improved oxygen and nutrient delivery (Sexton et al., 2016). Although positive associations between PA and brain structure have typically been interpreted as the result of positive effects of PA on brain structure it is also possible that better cerebral function, especially in old age, may lead to higher PA. A better cerebral function in old age may be associated with a more independent lifestyle, which in turn might be associated with higher PA.
Although a comparable interaction pattern between PA and age on FA and MD values has been observed, results between PA and FA values were less strong and did not reach statistical significance (corrected for multiple comparisons). Fa reflects non-isotropic diffusion. Although FA is very sensitive to microstructural changes, it is not thought to be specific to any particular tissue characteristic (Uddin et al., 2019). MD is a measure of the average magnitude of water diffusion and is used to infer the overall density of tissue barriers. It is thought to reflect the density of synapses, capillaries, and macromolecular proteins as well as changes in cell proliferation and the shapes of neurons or glia (Uddin et al., 2019). Many of these microstructural properties are related to PA (as described above), which might explain the stronger interaction pattern between PA and MD values compared to FA values.
PA has repeatedly been shown to be associated with cognitive functioning in older adults and with a reduced risk for developing Alzheimer’s disease (Kramer et al., 2006; Hillman et al., 2008; Buchman et al., 2012). A high level of PA thus qualifies as an important lifestyle factor to increase resilience against age-related cognitive decline. The neurobiological mechanisms underlying the association between PA and cognition in the elderly are not fully understood. It has been discussed that the positive effects of PA on cognition may be mediated by changes in brain structure and function induced by PA (Kramer and Erickson, 2007; Hillman et al., 2008). However, the role of WMI in the association between PA and cognition is not yet clear. Exploratory regression analyses of the current study demonstrated that WMI of regions that were associated with PA was also related to memory and executive functioning (see “Results” section). These results suggest that changes in WMI caused by PA may mediate the association between PA and cognition in older adults. This assumption is in line with the fact that cognition relies on coordinated processing among distributed cortical brain regions, which is enabled through interconnected white matter networks and that cortical disconnection has been shown to lead to cognitive dysfunction (Catani and ffytche, 2005; Filley, 2005). However, specific mediation analyses in larger study samples are necessary to confirm and specify the role of WMI in the association between PA and cognition in older adults.
An advantage of this study is the assessment of the general PA level using triaxial accelerometers. Triaxial accelerometers allow an objective measurement of PA that does not require any input from the participant and that is not associated with disadvantages typically seen in commonly used self-reports, such as recall bias and fluctuations in answers caused by health status, depression, anxiety, or cognitive ability (Rikli, 2000). Moreover, in comparison to self-reports, stronger relationships between objective accelerometer data and health characteristics, such as obesity or depressive symptoms, have been shown (Koolhaas et al., 2017; Guo et al., 2019). These results imply a higher validity of accelerometer data compared to self-reported data. Triaxial accelerometers are particularly appropriate for the assessment of PA in older adults since bias to the subjective recall of past events is typically more pronounced in advanced age (Barnett et al., 2016).
Methodological Considerations/Limitations
The study has several limitations. First, most of the study participants were highly educated, which might have been associated with a more active lifestyle. This in turn might be accompanied by a small variance in the amount of PA. Moreover, PA-related variations in brain structure might have been underestimated given a potentially homogeneous high level of PA within the study group. Future studies should confirm the observed results in subjects with a broad range of educational attainment and should investigate if the observed association between PA and WMI in older adults is independent of the general activity level. Second, the cross-sectional nature of the analysis does not allow the inference of causality. Third, due to the limited sample size, analyses might have been sensitive to type 2 errors. A power calculation has not been applied since this study is explorative. Future studies should confirm the findings of this study based on a larger study cohort.
Conclusion
Cortical disconnection caused by an age-related decline in WMI is likely to contribute to age-related cognitive decline. Hence, the prevention of white matter degeneration in aging is a promising approach to preserve cognitive functioning and late-life independency. The results of the present study demonstrate that PA is associated with WMI in older adults. Of note, results emphasize that this association is restricted to advanced stages of aging. Moreover, exploratory analyses demonstrated that WMI of regions that were associated with PA was also related to memory and executive functioning. Since cognitive decline in older adults is typically most pronounced in later stages of aging, PA qualifies as a promising tool to foster resilience against age-related cognitive decline, via the preservation of the integrity of the brains WM.
Data Availability Statement
The datasets generated for this study are available on request to the corresponding author.
Ethics Statement
The studies involving human participants were reviewed and approved by Ethics commissions of the Landesärztekammer Rheinland-Pfalz Ethics commissions of the Cologne University’s Faculty of Medicine and Ethics commissions of the Rostock University’s Faculty of Medicine. The patients/participants provided their written informed consent to participate in this study.
Author Contributions
DW: study design, data analyses, interpretation, and preparation of the manuscript. FF: data analyses and interpretation. DR: data acquisition and data analyses. KK, BK, MK, and KB: data acquisition. ST and OT: revision of the manuscript. HB: statistical support. AF: study design, interpretation, and revision of the manuscript. All authors contributed to the article and approved the submitted version.
Funding
This work was supported by the German Federal Ministry of Education and Research (Bundesministerium für Bildung und Forschung, BMBF; grant number: 01GQ1425A).
Conflict of Interest
The authors declare that the research was conducted in the absence of any commercial or financial relationships that could be construed as a potential conflict of interest.
The reviewer GG-E declared a shared affiliation, with no collaboration, with several of the authors, DW, FF, BK, MK, OT, AF, to the handling editor at the time of review.
Acknowledgments
We thank MK for the efforts in recruitment and data acquisition for the AgeGain study, on which the current study is based. Furthermore, we thank all participants for being part of the AgeGain study. AgeGain study group: University Medical Center Mainz: AF, OT, Bernhard Baier, DW, BK, FF, and Alexandra Sebastian; German Sport University Cologne: Heiko Strüder, AM, KK, and DR; University Clinic Cologne: Alexander Drzezga; University Medical Center Rostock: ST, KB, Judith Henf, and Esther Lau; University of Freiburg: HB.
References
Andersson, J. L., and Sotiropoulos, S. N. (2016). An integrated approach to correction for off-resonance effects and subject movement in diffusion MR imaging. NeuroImage 125, 1063–1078. doi: 10.1016/j.neuroimage.2015.10.019
Andersson, J. L., Skare, S., and Ashburner, J. (2003). How to correct susceptibility distortions in spin-echo echo-planar images: application to diffusion tensor imaging. NeuroImage 20, 870–888. doi: 10.1016/S1053-8119(03)00336-7
Barnett, A., van den Hoek, D., Barnett, D., and Cerin, E. (2016). Measuring moderate-intensity walking in older adults using the actigraph accelerometer. BMC Geriatr. 16:211. doi: 10.1186/s12877-016-0380-5
Benedict, C., Brooks, S. J., Kullberg, J., Nordenskjöld, R., Burgos, J., Le Grevès, M., et al. (2013). Association between physical activity and brain health in older adults. Neurobiol. Aging 34, 83–90. doi: 10.1016/j.neurobiolaging.2012.04.013
Bennett, I. J., and Madden, D. J. (2014). Disconnected aging: cerebral white matter integrity and age-related differences in cognition. Neuroscience 276, 187–205. doi: 10.1016/j.neuroscience.2013.11.026
Bherer, L., Erickson, K. I., and Liu-Ambrose, T. (2013). A review of the effects of physical activity and exercise on cognitive and brain functions in older adults. J. Aging Res. 2013:657508. doi: 10.1155/2013/657508
Buchman, A., Boyle, P., Yu, L., Shah, R., Wilson, R., and Bennett, D. (2012). Total daily physical activity and the risk of AD and cognitive decline in older adults. Neurology 78, 1323–1329. doi: 10.1212/WNL.0b013e3182535d35
Burzynska, A. Z., Chaddock-Heyman, L., Voss, M. W., Wong, C. N., Gothe, N. P., Olson, E. A., et al. (2014a). Physical activity and cardiorespiratory fitness are beneficial for white matter in low-fit older adults. PLoS One 9:e107413. doi: 10.1371/journal.pone.0107413
Burzynska, A. Z., Chaddock-Heyman, L., Voss, M. W., Wong, C. N., Gothe, N. P., Olson, E. A., et al. (2014b). Physical activity and cardiorespiratory fitness are beneficial for white matter in low-fit older adults. PLoS One 9:e107413. doi: 10.1371/journal.pone.0107413
Cao, L., Dhilla, A., Mukai, J., Blazeski, R., Lodovichi, C., Mason, C. A., et al. (2007). Genetic modulation of BDNF signaling affects the outcome of axonal competition in vivo. Curr. Biol. 17, 911–921. doi: 10.1016/j.cub.2007.04.040
Catani, M., and ffytche, D. H. (2005). The rises and falls of disconnection syndromes. Brain 128, 2224–2239. doi: 10.1093/brain/awh622
Colcombe, S. J., Erickson, K. I., Scalf, P. E., Kim, J. S., Prakash, R., McAuley, E., et al. (2006). Aerobic exercise training increases brain volume in aging humans. J. Gerontol. A Biol. Sci. Med. Sci. 61, 1166–1170. doi: 10.1093/gerona/61.11.1166
Depp, C. A., and Jeste, D. V. (2006). Definitions and predictors of successful aging: a comprehensive review of larger quantitative studies. Am. J. Geriatr. Psychiatry 14, 6–20. doi: 10.1097/01.JGP.0000192501.03069.bc
Ding, Y.-H., Li, J., Zhou, Y., Rafols, J. A., Clark, J. C., and Ding, Y. (2006). Cerebral angiogenesis and expression of angiogenic factors in aging rats after exercise. Curr. Neurovasc. Res. 3, 15–23. doi: 10.2174/156720206775541787
Doherty, A., Jackson, D., Hammerla, N., Plötz, T., Olivier, P., Granat, M. H., et al. (2017). Large scale population assessment of physical activity using wrist worn accelerometers: the UK biobank study. PLoS One 12:e0169649. doi: 10.1371/journal.pone.0169649
Erickson, K. I., Leckie, R. L., and Weinstein, A. M. (2014). Physical activity, fitness and gray matter volume. Neurobiol. Aging 35, S20–S28. doi: 10.1016/j.neurobiolaging.2014.03.034
Filley, C. M. (2005). White matter and behavioral neurology. Ann. N Y Acad. Sci. 1064, 162–183. doi: 10.1196/annals.1340.028
Gow, A. J., Bastin, M. E., Maniega, S. M., Hernández, M. C. V., Morris, Z., Murray, C., et al. (2012). Neuroprotective lifestyles and the aging brain: activity, atrophy and white matter integrity. Neurology 79, 1802–1808. doi: 10.1212/WNL.0b013e3182703fd2
Guo, W., Key, T. J., and Reeves, G. K. (2019). Accelerometer compared with questionnaire measures of physical activity in relation to body size and composition: a large cross-sectional analysis of UK Biobank. BMJ open 9:e024206. doi: 10.1136/bmjopen-2018-024206
Hillman, C. H., Erickson, K. I., and Kramer, A. F. (2008). Be smart, exercise your heart: exercise effects on brain and cognition. Nat. Rev. Neurosci. 9, 58–65. doi: 10.1038/nrn2298
Ho, A. J., Raji, C. A., Becker, J. T., Lopez, O. L., Kuller, L. H., Hua, X., et al. (2011). The effects of physical activity, education and body mass index on the aging brain. Hum. Brain Mapp. 32, 1371–1382. doi: 10.1002/hbm.21113
Huang, T., Larsen, K., Ried-Larsen, M., Møller, N., and Andersen, L. B. (2014). The effects of physical activity and exercise on brain-derived neurotrophic factor in healthy humans: a review. Scand. J. Med. Sci. Sports 24, 1–10. doi: 10.1111/sms.12069
Husson, I., Rangon, C.-M., Lelièvre, V., Bemelmans, A.-P., Sachs, P., Mallet, J., et al. (2005). BDNF-induced white matter neuroprotection and stage-dependent neuronal survival following a neonatal excitotoxic challenge. Cereb. Cortex 15, 250–261. doi: 10.1093/cercor/bhh127
Jenkinson, M., Beckmann, C. F., Behrens, T. E., Woolrich, M. W., and Smith, S. M. (2012). FSL. NeuroImage 62, 782–790. doi: 10.1016/j.neuroimage.2011.09.015
Johnson, N. F., Kim, C., Clasey, J. L., Bailey, A., and Gold, B. T. (2012). Cardiorespiratory fitness is positively correlated with cerebral white matter integrity in healthy seniors. NeuroImage 59, 1514–1523. doi: 10.1016/j.neuroimage.2011.08.032
Koolhaas, C. M., van Rooij, F. J., Cepeda, M., Tiemeier, H., Franco, O. H., and Schoufour, J. D. (2017). Physical activity derived from questionnaires and wrist-worn accelerometers: comparability and the role of demographic, lifestyle and health factors among a population-based sample of older adults. Clin. Epidemiol. 10, 1–16. doi: 10.2147/CLEP.S147613
Kramer, A. F., and Erickson, K. I. (2007). Capitalizing on cortical plasticity: influence of physical activity on cognition and brain function. Trends Cogn. Sci. 11, 342–348. doi: 10.1016/j.tics.2007.06.009
Kramer, A. F., Erickson, K. I., and Colcombe, S. J. (2006). Exercise, cognition and the aging brain. J. Appl. Physiol. 101, 1237–1242. doi: 10.1152/japplphysiol.00500.2006
Krityakiarana, W., Espinosa-Jeffrey, A., Ghiani, C., Zhao, P., Topaldjikian, N., Gomez-Pinilla, F., et al. (2010). Voluntary exercise increases oligodendrogenesis in spinal cord. Int. J. Neurosci. 120, 280–290. doi: 10.3109/00207450903222741
Liu, Z., Farzinfar, M., M Katz, L., Zhu, H., B Goodlett, C., Gerig, G., et al. (2012). Automated voxel-wise brain DTI analysis of fitness and aging. Open Med. Imaging J. 6, 80–88. doi: 10.2174/1874347101206010080
Madden, D. J., Bennett, I. J., and Song, A. W. (2009). Cerebral white matter integrity and cognitive aging: contributions from diffusion tensor imaging. Neuropsychol. Rev. 19, 415–435. doi: 10.1007/s11065-009-9113-2
Madden, D. J., Bennett, I. J., Burzynska, A., Potter, G. G., Chen, N.-K., and Song, A. W. (2012). Diffusion tensor imaging of cerebral white matter integrity in cognitive aging. Biochim. Biophys. Acta 1822, 386–400. doi: 10.1016/j.bbadis.2011.08.003
McDonnell, B. J., Maki-Petaja, K. M., Munnery, M., Wilkinson, I. B., Cockcroft, J. R., and McEniery, C. M. (2013). Habitual exercise and blood pressure: age dependency and underlying mechanisms. Am. J. Hypertens. 26, 334–341. doi: 10.1093/ajh/hps055
McPhee, J. S., French, D. P., Jackson, D., Nazroo, J., Pendleton, N., and Degens, H. (2016). Physical activity in older age: perspectives for healthy ageing and frailty. Biogerontology 17, 567–580. doi: 10.1007/s10522-016-9641-0
Menai, M., Van Hees, V. T., Elbaz, A., Kivimaki, M., Singh-Manoux, A., and Sabia, S. (2017). Accelerometer assessed moderate-to-vigorous physical activity and successful ageing: results from the whitehall II study. Sci. Rep. 7:45772. doi: 10.1038/srep45772
Migueles, J. H., Rowlands, A. V., Huber, F., Sabia, S., and van Hees, V. T. (2019). GGIR: a research community-driven open source R package for generating physical activity and sleep outcomes from multi-day raw accelerometer data. J. Meas. Phys. Behav. 2, 188–196. doi: 10.1123/jmpb.2018-0063
Reichstadt, J., Depp, C. A., Palinkas, L. A., and Jeste, D. V. (2007). Building blocks of successful aging: a focus group study of older adults’ perceived contributors to successful aging. Am. J. Geriatr. Psychiatry 15, 194–201. doi: 10.1097/JGP.0b013e318030255f
Rikli, R. E. (2000). Reliability, validity and methodological issues in assessing physical activity in older adults. Res. Q. Exerc. Sport 71, 89–96. doi: 10.1080/02701367.2000.11082791
Rueckert, D., Sonoda, L. I., Hayes, C., Hill, D. L., Leach, M. O., and Hawkes, D. J. (1999). Nonrigid registration using free-form deformations: application to breast MR images. IEEE Trans. Med. Imaging 18, 712–721. doi: 10.1109/42.796284
Salthouse, T. A. (2010). Selective review of cognitive aging. J. Int. Neuropsychol. Soc. 16, 754–760. doi: 10.1017/S1355617710000706
Sanders, G. J., Boddy, L. M., Sparks, S. A., Curry, W. B., Roe, B., Kaehne, A., et al. (2019). Evaluation of wrist and hip sedentary behaviour and moderate-to-vigorous physical activity raw acceleration cutpoints in older adults. J. Sports Sci. 37, 1270–1279. doi: 10.1080/02640414.2018.1555904
Sen, A., Gider, P., Cavalieri, M., Freudenberger, P., Farzi, A., Schallert, M., et al. (2012). Association of cardiorespiratory fitness and morphological brain changes in the elderly: results of the austrian stroke prevention study. Neurodegener. Dis. 10, 135–137. doi: 10.1159/000334760
Sexton, C. E., Betts, J. F., Demnitz, N., Dawes, H., Ebmeier, K. P., and Johansen-Berg, H. (2016). A systematic review of MRI studies examining the relationship between physical fitness and activity and the white matter of the ageing brain. NeuroImage 131, 81–90. doi: 10.1016/j.neuroimage.2015.09.071
Sexton, C. E., Walhovd, K. B., Storsve, A. B., Tamnes, C. K., Westlye, L. T., Johansen-Berg, H., et al. (2014). Accelerated changes in white matter microstructure during aging: a longitudinal diffusion tensor imaging study. J. Neurosci. 34, 15425–15436. doi: 10.1523/JNEUROSCI.0203-14.2014
Singh, K. K., Park, K. J., Hong, E. J., Kramer, B. M., Greenberg, M. E., Kaplan, D. R., et al. (2008). Developmental axon pruning mediated by BDNF-p75NTR-dependent axon degeneration. Nat. Neurosci. 11, 649–658. doi: 10.1038/nn.2114
Smith, S. M., Jenkinson, M., Johansen-Berg, H., Rueckert, D., Nichols, T. E., Mackay, C. E., et al. (2006). Tract-based spatial statistics: voxelwise analysis of multi-subject diffusion data. NeuroImage 31, 1487–1505. doi: 10.1016/j.neuroimage.2006.02.024
Swain, R. A., Harris, A. B., Wiener, E. C., Dutka, M. V., Morris, H. D., Theien, B. E., et al. (2003). Prolonged exercise induces angiogenesis and increases cerebral blood volume in primary motor cortex of the rat. Neuroscience 117, 1037–1046. doi: 10.1016/s0306-4522(02)00664-4
Tian, Q., Erickson, K. I., Simonsick, E. M., Aizenstein, H. J., Glynn, N. W., Boudreau, R. M., et al. (2014). Physical activity predicts microstructural integrity in memory-related networks in very old adults. J. Gerontol. A Biol. Sci. Med. Sci. 69, 1284–1290. doi: 10.1093/gerona/glt287
Torres, E. R., Strack, E. F., Fernandez, C. E., Tumey, T. A., and Hitchcock, M. E. (2015). Physical activity and white matter hyperintensities: a systematic review of quantitative studies. Pre. Med. Rep. 2, 319–325. doi: 10.1016/j.pmedr.2015.04.013
Tournier, J.-D., Smith, R., Raffelt, D., Tabbara, R., Dhollander, T., Pietsch, M., et al. (2019). MRtrix3: a fast, flexible and open software framework for medical image processing and visualisation. NeuroImage 202:116137. doi: 10.1016/j.neuroimage.2019.116137
Tseng, B. Y., Gundapuneedi, T., Khan, M., Diaz-Arrastia, R., Levine, B., Lu, H., et al. (2013a). White matter integrity in physically fit older adults. NeuroImage 82, 510–516. doi: 10.1016/j.neuroimage.2013.06.011
Tseng, B. Y., Uh, J., Rossetti, H. C., Cullum, C. M., Diaz-Arrastia, R. F., Levine, B. D., et al. (2013b). Masters athletes exhibit larger regional brain volume and better cognitive performance than sedentary older adults. J. Magn. Reson. Imaging 38, 1169–1176. doi: 10.1002/jmri.24085
Uddin, M. N., Figley, T. D., Solar, K. G., Shatil, A. S., and Figley, C. R. (2019). Comparisons between multi-component myelin water fraction, T1w/T2w ratio and diffusion tensor imaging measures in healthy human brain structures. Sci. Rep. 9:2500. doi: 10.1038/s41598-019-39199-x
Van Hees, V. T., Fang, Z., Langford, J., Assah, F., Mohammad, A., da Silva, I. C., et al. (2014). Autocalibration of accelerometer data for free-living physical activity assessment using local gravity and temperature: an evaluation on four continents. J. Appl. Physiol. 117, 738–744. doi: 10.1152/japplphysiol.00421.2014
Van Hees, V. T., Gorzelniak, L., Leon, E. C. D., Eder, M., Pias, M., Taherian, S., et al. (2013). Separating movement and gravity components in an acceleration signal and implications for the assessment of human daily physical activity. PLoS One 8:e61691. doi: 10.1371/journal.pone.0061691
Voss, M. W., Heo, S., Prakash, R. S., Erickson, K. I., Alves, H., Chaddock, L., et al. (2013). The influence of aerobic fitness on cerebral white matter integrity and cognitive function in older adults: results of a one-year exercise intervention. Hum. Brain Mapp. 34, 2972–2985. doi: 10.1002/hbm.22119
Weinstock-Guttman, B., Zivadinov, R., Tamaño-Blanco, M., Abdelrahman, N., Badgett, D., Durfee, J., et al. (2007). Immune cell BDNF secretion is associated with white matter volume in multiple sclerosis. J. Neuroimmunol. 188, 167–174. doi: 10.1016/j.jneuroim.2007.06.003
Wilson, R. S., Beckett, L. A., Barnes, L. L., Schneider, J. A., Bach, J., Evans, D. A., et al. (2002). Individual differences in rates of change in cognitive abilities of older persons. Psychol. Aging 17, 179–193. doi: 10.1037/0882-7974.17.2.179
Wirth, M., Haase, C. M., Villeneuve, S., Vogel, J., and Jagust, W. J. (2014). Neuroprotective pathways: lifestyle activity, brain pathology and cognition in cognitively normal older adults. Neurobiol. Aging 35, 1873–1882. doi: 10.1016/j.neurobiolaging.2014.02.015
Keywords: healthy aging, physical activity, actigraphy, white matter integrity, cognition
Citation: Wolf D, Fischer FU, Riedel D, Knaepen K, Kollmann B, Kocabayoglu M, Brüggen K, Teipel S, Tüscher O, Binder H, Mierau A and Fellgiebel A (2020) The Impact of Age on the Association Between Physical Activity and White Matter Integrity in Cognitively Healthy Older Adults. Front. Aging Neurosci. 12:579470. doi: 10.3389/fnagi.2020.579470
Received: 02 July 2020; Accepted: 05 October 2020;
Published: 05 November 2020.
Edited by:
Yong Liu, Chinese Academy of Sciences, ChinaReviewed by:
Ilona Dekkers, Leiden University, NetherlandsGabriel Gonzalez-Escamilla, Johannes Gutenberg University Mainz, Germany
Copyright © 2020 Wolf, Fischer, Riedel, Knaepen, Kollmann, Kocabayoglu, Brüggen, Teipel, Tüscher, Binder, Mierau and Fellgiebel. This is an open-access article distributed under the terms of the Creative Commons Attribution License (CC BY). The use, distribution or reproduction in other forums is permitted, provided the original author(s) and the copyright owner(s) are credited and that the original publication in this journal is cited, in accordance with accepted academic practice. No use, distribution or reproduction is permitted which does not comply with these terms.
*Correspondence: Dominik Wolf, dominik.wolf@unimedizin-mainz.de