- 1Research Imaging Centre, Campbell Family Mental Health Research Institute, Centre for Addiction and Mental Health, University of Toronto, Toronto, ON, Canada
- 2Division of Brain, Imaging and Behaviour – Systems Neuroscience, Krembil Research Institute, University Health Network, University of Toronto, Toronto, ON, Canada
- 3Division of Neurosurgery, Toronto Western Hospital, University Health Network, University of Toronto, Toronto, ON, Canada
- 4Morton and Gloria Shulman Movement Disorder Unit & E. J. Safra Parkinson Disease Program, Neurology Division, Department of Medicine, Toronto Western Hospital, University Health Network, University of Toronto, Toronto, ON, Canada
The recent application of graph theory to brain networks promises to shed light on complex diseases such as Parkinson’s disease (PD). This study aimed to investigate functional changes in sensorimotor and cognitive networks in Parkinsonian patients, with a focus on inter- and intra-connectivity organization in the disease-associated nodal and hub regions using the graph theoretical analyses. Resting-state functional MRI data of a total of 65 participants, including 23 healthy controls (HCs) and 42 patients, were investigated in 120 nodes for local efficiency, betweenness centrality, and degree. Hub regions were identified in the HC and patient groups. We found nodal and hub changes in patients compared with HCs, including the right pre-supplementary motor area (SMA), left anterior insula, bilateral mid-insula, bilateral dorsolateral prefrontal cortex (DLPFC), and right caudate nucleus. In general, nodal regions within the sensorimotor network (i.e., right pre-SMA and right mid-insula) displayed weakened connectivity, with the former node associated with more severe bradykinesia, and impaired integration with default mode network regions. The left mid-insula also lost its hub properties in patients. Within the executive networks, the left anterior insular cortex lost its hub properties in patients, while a new hub region was identified in the right caudate nucleus, paralleled by an increased level of inter- and intra-connectivity in the bilateral DLPFC possibly representing compensatory mechanisms. These findings highlight the diffuse changes in nodal organization and regional hub disruption accounting for the distributed abnormalities across brain networks and the clinical manifestations of PD.
Introduction
The clinical phenotypes of Parkinson’s disease (PD) include cardinal motor symptoms such as tremor, rigidity, bradykinesia, and loss of postural stability, along with a set of non-motor symptoms such as depression, sleep disturbances, autonomic dysfunction and cognitive impairment (Barnum and Tansey, 2012; Bonnet et al., 2012). Cognitive impairment is one of the most common non-motor symptoms in PD. It ranges from mild cognitive impairment in different cognitive domains (e.g., attention, executive, visuospatial, and memory) to dementia, which is thought to be derived from dysfunction of different neurotransmitter systems/brain networks (Gratwicke et al., 2015) associated with Lewy pathology and Alzheimer’s disease pathology in cortical and subcortical regions (Halliday et al., 2014). The recent graph theoretical approaches may be suitable to capture such neuropathology involving diffuse area of brain as well as complex cognitive function involving interactions of multiple regions and networks, as it allows for characterization of functional interactions such as integration and segregation on multiple levels such as nodes, modules, and entire brain as well as for identification of hub regions (Bullmore and Sporns, 2009), revealing more information about brain network changes compared with other methods such as seed-based analysis and independent component analysis.
The graph theoretical approaches have revealed that human brains show the following topological characteristics (Bullmore and Sporns, 2009, 2012). First, individual brain regions termed ‘nodes’ typically have disproportionate connections with other nodes. Highly connected nodes are considered as hubs, which play a crucial role in integrating information. The anterior and posterior cingulate cortices, insula, and superior frontal and parietal cortex are identified as both structural and functional hubs (van den Heuvel and Sporns, 2013; Sporns, 2014). These are heteromodal areas that are involved in a broad range of cognitive processes (Achard et al., 2006). Second, some nodes are more closely connected to one another and form modules, which promote specialized processing and functional segregation. Large-scale modules correspond to well-established resting-state functional networks (Engel et al., 2013; Stam, 2014). Third, these modules are also interconnected via hubs with long-distance pathways and thereby promote functional integration. Brain networks display small-world properties of efficient global and local parallel information transfer (i.e., integration and segregation) at relatively low connection cost (Achard and Bullmore, 2007), which reflects an optimal organization through evolution and development (Gong et al., 2009; Bullmore and Sporns, 2012). The coexistence and balance of segregation and integration of modules are fundamental for brain function (Bullmore and Sporns, 2012; Sporns, 2013).
There is evidence that the pathological course of neurodegenerative diseases, such as Alzheimer’s disease, target network hubs (Seeley et al., 2009; Stam et al., 2009), and selective damage to hubs can significantly change the integration of brain networks (Bullmore and Sporns, 2012). With the dysfunction of multiple neurotransmitters (Gratwicke et al., 2015; Pagonabarraga et al., 2015) and deposition of alpha-synuclein (Braak et al., 2005), network hubs are almost certainly vulnerable in PD (Stam, 2014), and the selective deterioration of these network hubs may account for the distributed abnormalities across the brain in PD (McColgan et al., 2015) with distinct clinical correlations.
The premotor area and pre-supplementary motor area (SMA) have been identified as key regions connecting multiple networks in healthy adults (Spreng et al., 2013). These regions are important for self-initiated movements and preparation for actions (Passingham et al., 2010). The SMA consisting of SMA proper and pre-SMA, is part of the basal ganglia thalamo-cortical motor circuit (Alexander et al., 1986) associated with dopamine and the changes in functional connectivity have been well documented in PD patients, along with other cortical motor areas such as the primary motor cortex and premotor area (Wu et al., 2009a).
The dorsolateral prefrontal cortex (DLPFC) has been implicated as an anatomical and a functional node associated with executive functions (Spreng et al., 2013; van den Heuvel and Sporns, 2013). This brain region is part of fronto-parietal network (FPN) or executive control network (Dosenbach et al., 2007; Seeley et al., 2007), as well as the fronto-striatal dopamine network (Gratwicke et al., 2015). PD patients often show executive dysfunction due to a disruption of the fronto-striatal dopamine system (Gratwicke et al., 2015). In addition, structural (Koshimori et al., 2015) and functional (Trujillo et al., 2015; Wu et al., 2015) changes in this cortical area and their association with cognitive impairment have been reported consistently in these patients (Koshimori et al., 2015).
Another important disease-associated hub in PD is the insular cortex, which connects and integrates several functional systems: the anterior area is involved in cognitive and behavioral/emotional functions, and the mid to posterior area involved in sensorimotor functions (Kurth et al., 2010). The insular cortex has extensive connections with the striatum in a connectivity gradient from posterior to anterior (Christopher et al., 2014a). In post-mortem PD brains, alpha-synuclein depositions become evident throughout the insula at Braak’s stage V (Braak et al., 2005). In addition, the level of CSF alpha-synuclein concentration was significantly associated with anterior insular network disruption (Madhyastha et al., 2015). The anterior insula is a core region of the salience network that guides behavior (Menon and Uddin, 2010), and this brain network can affect other cognitive networks such as the default mode network (DMN) (Bonnelle et al., 2012; Chiong et al., 2013). Graph theoretical analyses have revealed a decreased hub role in the left dorsal anterior insula in PD patients compared to healthy controls (HCs) (Tinaz et al., 2016). Furthermore, recent data from our group have demonstrated that D2 receptor availability in the anterior insula was associated with executive and memory dysfunction in PD patients with mild cognitive impairment (Christopher et al., 2014b, 2015). Thus, the anterior insula, in particular, seems to be a critical “hub” for cognitive impairment in Parkinsonian patients (Christopher et al., 2014a; Criaud et al., 2016).
The present study aimed to investigate whether nodal connections and network hubs were affected in PD patients, and whether these changes were associated with motor and non-motor symptoms employing graph theoretical approaches to resting-state functional MRI (rsfMRI) data. We hypothesized that (1) PD would affect the nodal and hub organization of regions such as the SMA, insula, DLPFC, as well as the striatum, and (2) the nodal and hub changes would account for some of the motor and cognitive symptoms of PD.
Materials and Methods
Participants
Forty-five patients meeting the UK Brain Bank criteria for the diagnosis of idiopathic PD and 25 HCs participated in the study. During the screening session, all participants provided written informed consent following a full explanation of the study procedures. Subsequently, they were assessed for their global cognitive performance using the Montreal Cognitive Assessment (MoCA) and their level of depression using the Beck Depression Inventory (BDI) II. Additionally, patients were assessed for their motor severity of the disease using the motor subset of the Unified Parkinson Disease Rating Scale (UPDRS) III and for disease stage using the Hoehn and Yahr. The Levodopa Equivalent Daily Dose (LEDD) was also calculated by Levodopa (mg/day) + Controlled Levodopa (Levodopa × 0.75 mg/day) + Entacapone/Comtan (Levodopa × 0.33 mg/day) + Pramipexole/Mirapex (mg/day) + Ropinirole/Requiq × 20 (mg/day) + Rasagiline/Azilect × 100 (mg/day) + Selegiline × 10 (mg) + Amantadine (mg/day) (Tomlinson et al., 2010). All the participants underwent a structural and rsfMRI scan. Patients underwent the study procedures in an on-medication state. We reasoned that (1) scanning patients in an on-medication state would minimize participants’ motion effects on rsfMRI data (Tahmasian et al., 2015), and (2) studying chronically medicated patients would permit us to capture the complex neural substrate of cognitive impairment because cognitive impairment in patients is mostly likely to be derived from dysfunction of multiple neurotransmitters (Gratwicke et al., 2015). Exclusion criteria for the participants included: (1) history of a head injury, psychiatric or neurological diseases (except PD for the patients), (2) alcohol or drug dependency or abuse, (3) contraindications for MRI scanning, (4) dyskinesias and dystonia for PD patients, and (5) for HCs, MoCA scores <26 and BDI II scores >13. The study was approved by the Centre for Addiction and Mental Health Research Ethics Board.
MRI Image Acquisition
MR images were acquired with a General Electric Discovery MR 750 3T scanner with 8-channel head coil. The protocol included whole-brain anatomic T1-weighted MRI images (Fast Spoiled Gradient Echo pulse sequence; 200 sagittal slices; matrix of 256 × 256; Repetition Time: 6.7 ms; Echo Time: 3.0 ms; slice thickness: 0.9 mm; Field of View: 23 cm; Inversion Time: 650 ms; flip angle = 8°) and rsfMRI images (Gradient Echo/Fast Gradient Echo pulse sequence; 31 axial slices; matrix of 64 × 64; Repetition Time: 2000 ms; Echo Time: 30 ms; flip angle: 60°; Field of View: 22 cm; slice thickness: 5 mm; duration: approximately 8 min). During the rsfMRI scan, the participants were instructed to keep their eyes open, let their mind wander, not to think about anything in particular, and not to fall asleep. The first two volumes of the rsfMRI images were removed to allow for magnetization equilibrium, resulting in the preprocessing of 240 volumes.
rsfMRI Preprocessing
The functional data were preprocessed for each subject using the Conn functional connectivity toolbox version 15a (Whitfield-Gabrieli and Nieto-Castanon, 2012) including slice timing correction, motion correction using a 6° rigid spatial transformation, which provided the spatial deviation for each time point for translational (x, y, z) and rotational (roll, pitch, yaw) directions of movement, normalization into standard Montreal Neurological Institute space using non-linear transformations, and smoothing with a Gaussian smoothing kernel of 6 mm full-width half maximum. Outliers in the global signal of brain activation and movement were examined using the artifact detection toolbox. Time points were considered as outliers if the global signal of brain activation exceeded three standard deviations of the mean or if movement exceeded 0.5 mm across translational and rotational directions of scan-to-scan deviation. Participants were excluded from the analyses if outliers accounted for greater than 20% of the entire dataset. Among the 70 participants, five participants (two HCs and three patients) were excluded due to excessive head motion and artifacts, resulting in 23 HCs and 42 patients included in the graph theoretical analysis. Realignment parameters for head motion including x, y, z, roll, pitch, yaw as well as the total number of outliers did not show significant group differences between HCs and patients. Nuisance signals including six motion parameters as well as the signal from white matter and CSF voxels were regressed using aCompCor, the component–based noise correction method (Behzadi et al., 2007). The residual datasets were then temporally filtered (0.008 < f < 0.09).
Network Nodes
Among 160 regions of interest of six different neural networks, derived from a series of five meta-analyses of fMRI activation studies combined with cognitive control nodes identified during error-processing (Dosenbach et al., 2010), we selected 120 regions of interest in spheres with a radius of 5 mm in four networks (Figures 1A–D) to investigate the hub regions associated with motor and cognitive symptoms of PD. These included 34 nodes of the DMN, 21 nodes of the FPN, 32 nodes of the cingulo-opercular network (CON), and 33 nodes of the sensorimotor network (SMN).
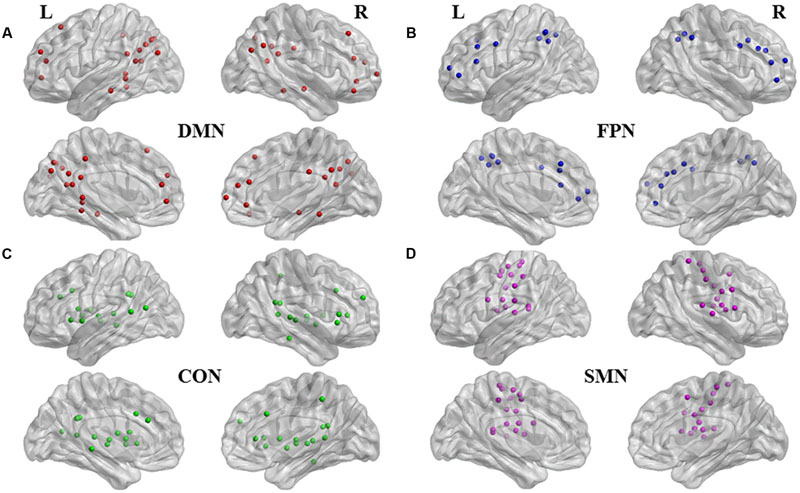
FIGURE 1. Functional nodes investigated. Medial and lateral views of brain images with 120 regions of interest of four subnetworks (Dosenbach et al., 2010). (A) 34 nodes in red are affiliated with default mode network (DMN); (B) 21 nodes in blue, with fronto-parietal network (FPN); (C) 32 nodes in green, with cinculo-opercular network (CON); and (D) 33 nodes in purple, with sensorimotor network (SMN). Brain image and nodes are visualized using the BrainNet Viewer (NKLCNL, Beijing Normal University).
Computation of Network Measures
Network measures were computed with age as a covariate using a Graph-Theoretical Analysis Toolbox (Hosseini et al., 2012). This toolbox constructs a binary undirected graph G that has a network degree of E equal to the number of edges, and a network density (cost) of D = E/[N × (N-1)]/2 representing the ratio of existing edges relative to all possible edges (Bruno et al., 2012). It also identifies network fragment. Network measures were computed over a range of connection densities. We examined those graphs where all nodes were fully connected in the networks for both groups, which ensured that network comparison between groups was meaningful with the same number of nodes in each module, and where small-world properties were displayed for both groups. Network fragment was observed at the density of 40%. Animal research has indicated that densities above 50% are unlikely to be biological (Kaiser and Hilgetag, 2006). Therefore, the densities ranging from 41 to 50% with an increment of 1% were investigated. Both HCs and patients displayed small worldness properties where normalized characteristic path length is close to one and normalized clustering coefficient is greater than one. Our interest was to detect changes in nodal characteristics including hubness. Therefore, we investigated the graph measures of local efficiency, as well as of nodal degree and betweenness centrality (BC) (Bullmore and Sporns, 2009; Rubinov and Sporns, 2010). Local efficiency measures the efficiency of local communication of a given node, taking account for the number of the shortest paths between its neighboring nodes (Latora and Marchiori, 2001; Rubinov and Sporns, 2010). Nodal degree is defined as the total number of connections that a node has with other nodes in the network, and BC is defined as the fraction of all shortest paths in the network that pass through a given node. Bridging nodes that connect disparate parts of the network often have high BC, making a potential hub role of these nodes in the network (van den Heuvel and Hulshoff Pol, 2010), that interact with many other regions and facilitate functional integration (Rubinov and Sporns, 2010).
Seed-Based Functional Connectivity Analysis
The only nodes showing group differences were further investigated with seed-based functional connectivity analyses to elucidate changes in connectivity with other nodes in the network using the Conn functional connectivity toolbox version 15a.
Statistical Analysis
Statistical analyses for demographic measures were performed using SPSS Statistics 20.0.01 (Chicago, IL, USA). Statistical significance threshold was set at P ≤ 0.05. Independent student’s t-test was used to compare the means in head motion parameters and demographic measures between patients and HCs.
Area under the curve (AUC) analyses were performed to test group differences in graph measures using a two-tailed non-parametric permutation test with 1000 repetitions over the density range without network fragmentation using Graph-Theoretical Analysis Toolbox. The AUC analyses are less sensitive for thresholding processes as they yield a summarized scalar independent of single threshold selection. Statistical significance threshold was set at P ≤ 0.005.
As a hub is characterized both by high connectivity and centrality (Sporns et al., 2007), the nodes whose degree and BC were one standard deviation above the mean network degree and BC were considered hubs. Spearman correlation analyses were also performed between the only network measures that yielded significant group differences, and different disease measures (UPDRS III total scores, rigidity, bradykinesia, and resting tremor sub-scores, LEDD, MoCA and BDI II scores) using SPSS Statistics 20.0.02 (Chicago, IL, USA) Statistical significance threshold was set at P ≤ 0.05. General linear model was used to obtain between-subjects contrasts of seed-based functional connectivity analysis. Statistical significance threshold was set at P ≤ 0.005.
Results
Demographic and Clinical Characteristics
Table 1 shows the demographic and clinical characteristics of the patients and HCs. There were significant differences in MoCA (t = 3.216, P = 0.002), and BDI II scores (t = -4.27, P < 0.001) between the two groups. PD patients showed lower global cognitive performance and higher depression level than HCs. There was no significant difference regarding age (t = 0.588, P = 0.56), or education (t = 0.179, P = 0.86) between the two groups.
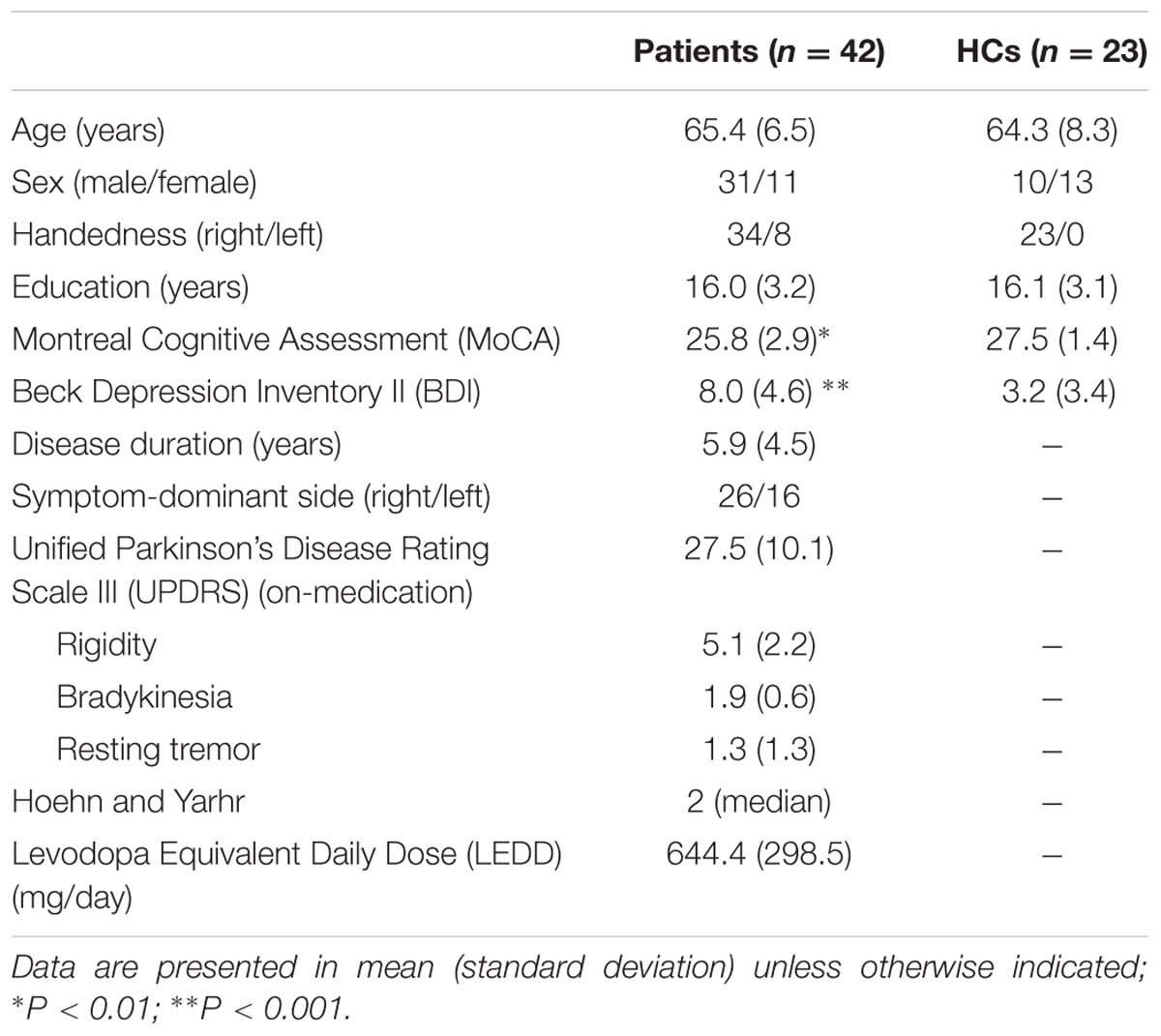
TABLE 1. Demographic and clinical characteristics of patients with Parkinson’s disease (PD) and healthy controls (HCs).
Group Comparisons of Graph Measures
Compared to HCs, patients showed increased numbers of connections in the right and left DLPFC measured by nodal degree (P = 0.005 and P = 0.003, respectively) (Table 2; Figure 2). In contrast, patients showed a reduction in intra-connectivity of the right mid-insula measured by local efficiency (P = 0.005), as well as a reduction in bridging role of the right pre-SMA (P = 0.005) measured by nodal BC.
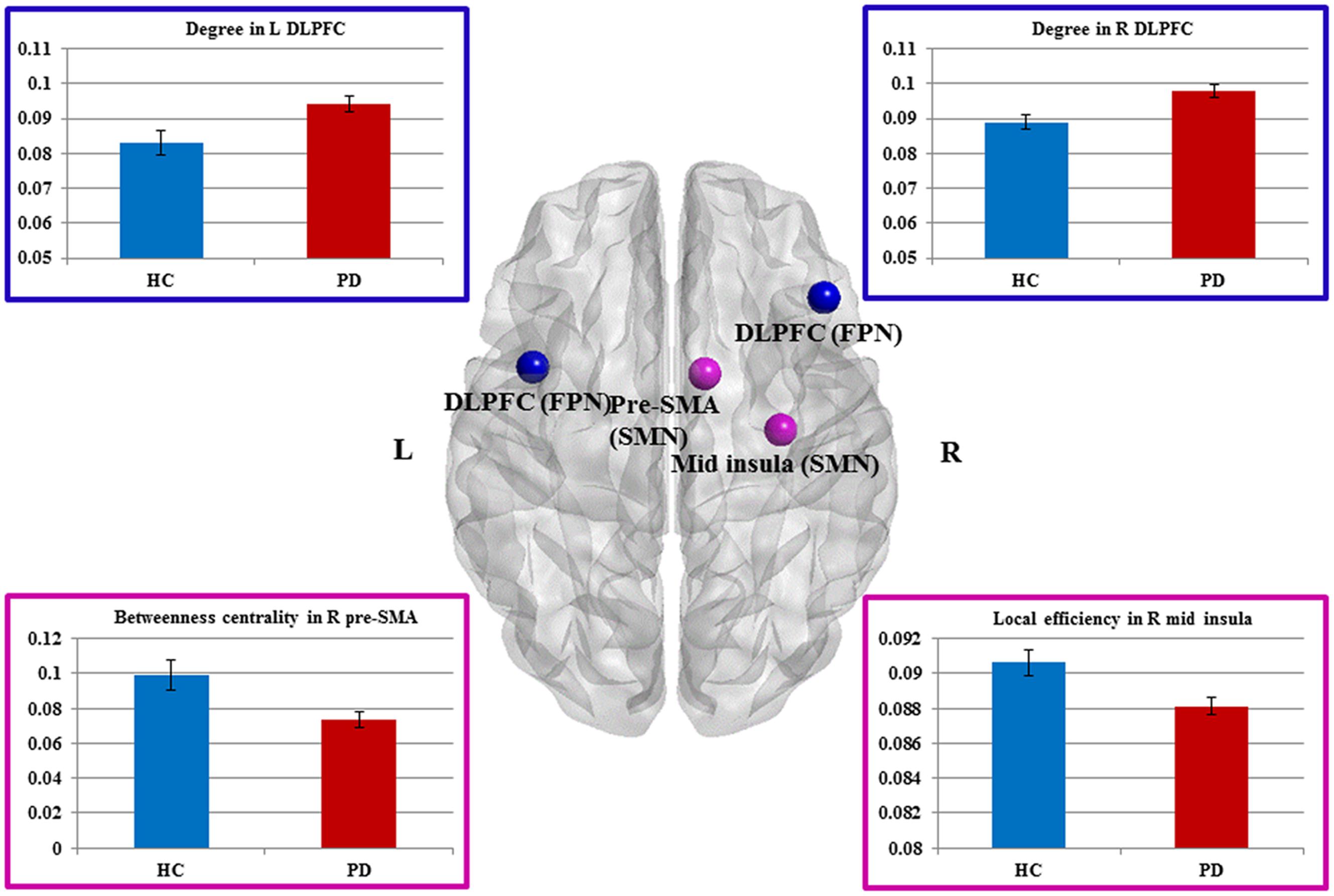
FIGURE 2. Sensorimotor and fronto-parietal nodes showing group differences. Dorsal view of brain image presenting four nodes showing group differences. Increased degree in the bilateral DLPFC of FPN, as well as decreased betweeness centrality in the right pre-SMA and decreased local efficiency in the right mid-insula of SMN in Parkinson’s disease (PD) patients compared with healthy controls (HCs) (P ≤ 0.005, uncorrected). The nodes are presented in equal size for visualization purposes. Brain image and nodes are visualized using the BrainNet Viewer (NKLCNL, Beijing Normal University). Bar graphs show the averaged values of each graph measure for HCs and PD (patients with Parkinson’s disease). Error bars represent standard error of the mean.
We interrogated whether the graph measures in these four nodes would account for any of the clinical measures. We found that BC of the right pre-SMA showed a significant negative correlation with bradykinesia scores (r = -0.31, P = 0.048), suggesting that the diminished bridging role (i.e., impaired integration) of the right pre-SMA may contribute to more severe bradykinesia. In addition, we conducted exploratory correlation analyses between the bilateral DLPFC and MoCA subscores for executive function and attention because the DLPFC is involved in both functions. Although we did not find any correlations in PD patients, interestingly, PD patients performed as well as HCs on these subtests.
We then applied the seed-based functional connectivity analyses to explore how these four affected areas changed their connectivity with other nodes of the network. The results are summarized in Table 3. First, nodes in the DLPFC of FPN showed increased intra-connectivity with one another. This increased regional inter-connectivity may occur as a compensatory mechanism. Second, the right pre-SMA of the SMN showed reduced inter-connectivity with nodes in the DMN, suggesting its bridging and integrative role between cognitive and motor functions.
Hub Analysis
Patients presented eight regions acting as hubs while HCs presented nine regions (Table 4). Seven of these regions were shared by both patients and HCs. The patients lost hub properties in the left anterior insula of the CON and the left mid-insula of the SMN, while the right caudate nucleus of the CON acted as a new hub in the patients (Figure 3). We explored whether any of these three hub regions were associated with the clinical measures. We found a significant positive correlation between hubness (i.e., mean of degree and BC) in the left mid-insula and dopaminergic medication (r = 0.3, P = 0.05), suggesting that dopaminergic medication may influence hubness in the mid-insula in these patients. We conducted further exploratory correlation analyses between the left anterior insula and the right caudate nucleus, and executive function and memory as the insula was associated with both executive and memory function in PD patients. No correlations were found in PD patients. However, PD patients performed significantly worse than HCs on the memory subtest (PD: 2.9 ± 1.4 and HC: 3.7 ± 1.3, t = 2.1, P = 0.038).
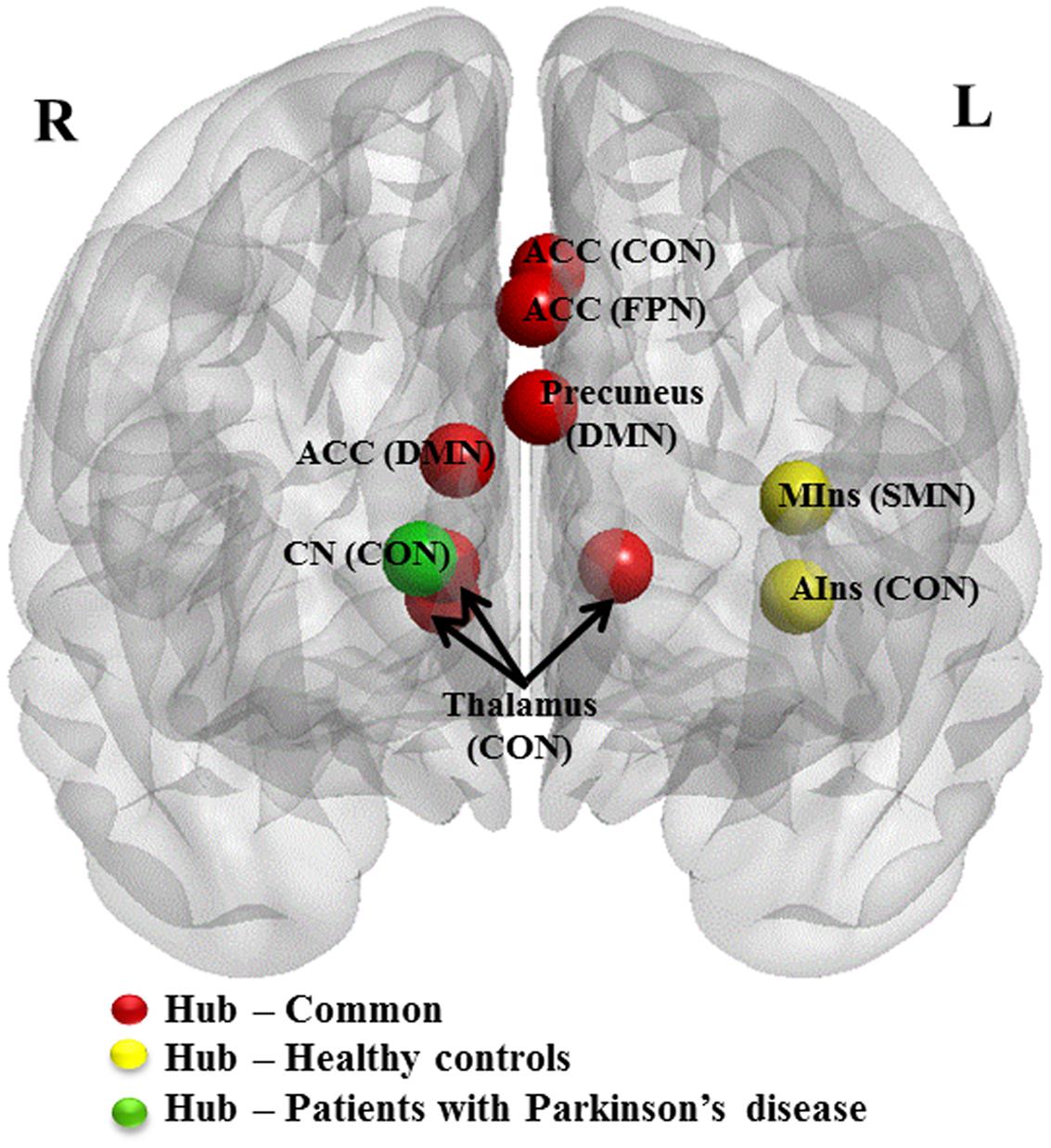
FIGURE 3. Hub regions in patients with PD and HCs. Caudal view of brain image presenting 10 hub regions. ACC, anterior cingulate cortex; AIns, anterior insula; CN, caudate nuculeus; CON, cingulo-opercular network; DMN, default mode network; FPN, fronto-parietal network; MIns, mid-insula. Seven hubs in red represent common hubs to both HCs and patients with PD. Two in yellow represent hubs identified only in HCs and one in green, only in patients. Brain image and hubs are visualized using the BrainNet Viewer (NKLCNL, Beijing Normal University).
Discussion
In the current study, we aimed to investigate functional changes in the SMN and cognitive networks including the DMN, FPN, and CON in PD with a particular focus on changes in the disease-associated nodes and hubs using graph theoretical analyses. In general, patients showed weakened connectivity in nodes of the SMN and enhanced connectivity in nodes associated with cognitive networks. Patients showed functional changes in the right pre-SMA and right mid-insula, as well as the bilateral DLPFC (BA9) compared with HCs. PD also affected hub functions and organization. In particular, the left anterior and left mid-insula cortex lost their hub properties and a node in the caudate nucleus was identified as a new hub in these patients.
Changes in the SMN
As hypothesized, we found functional changes in nodes of the SMN, which is consistent with previous studies using the graph theoretical approaches (Wu et al., 2009b; Skidmore et al., 2011; Nagano-Saito et al., 2014; Wei et al., 2014; Tinaz et al., 2016). Specifically, patients showed a reduction in intra-connectivity of the right mid-insula and impaired integration of the right pre-SMA with nodes of the DMN. The diminished bridging role of the right pre-SMA was associated with more severe bradykinesia. The left mid-insula lost its hub properties in the patients and showed a positive interaction with dopaminergic medication.
The pre-SMA is the anterior part of the SMA and is separated from the SMA proper by the vertical anterior commissural line (Picard and Strick, 2001). These two areas are different in terms of anatomical connections and functions. The SMA proper is connected to the primary motor cortex, projected to the spinal cord and is involved in movement generation, while the pre-SMA has extensive connections with the prefrontal cortex (Wang et al., 2001) and is involved in cognition (Picard and Strick, 2001). For example, the pre-SMA has been implicated to play an important role in cognitive motor control involving integration of sensory information and decision-making about actions or motor selection (Ikeda et al., 1999).
It is not surprising that the decreased inter-connectivity of the pre-SMA with a cognitive network can account for the slowness of movement, as it is postulated that bradykinesia could arise from slowness in formulating the instructions to move before the onset of and during the actions (Berardelli et al., 2001). As the right pre-SMA, but not the left pre-SMA has functional connectivity with the DMN (Ter Minassian et al., 2014), the weakened connectivity between the right pre-SMA and the nodes of the DMN, in the context of dopamine deficiency, may affect the redirection of attentional processes from self-reflection (i.e., internal reference) to goal-directed behavior, thus contributing to bradykinesia.
We found that the left mid-insula correlated with LEDD, but not with the UPDRS motor scores or subscores. This might be because the mid-insula is implicated in the processing of position, movement, and sensation of the body (Cerasa et al., 2006; Chang et al., 2013). The UPDRS-III and the motor subscores may not closely reflect the function of mid-insula when patients are in an on medication state. In addition, the presence or absence of correlation between the right and left mid-insula of SMN and LEDD might be due to the differences in their network measures (i.e., local efficiency vs. hubness).
Changes in the Cognitive Networks
We also found functional changes in the DLPFC (BA9) associated with the FPN and the left anterior insula of the CON in the patients compared with HCs. The patients showed an increased level of connectivity in bilateral DLPFC (BA9). The subsequent functional connectivity analysis revealed that the enhanced connectivity occurred within and between cognitive networks (i.e., FPN and CON). The hub analysis revealed that while the left anterior insula lost its hub properties, a new hub region was identified in the striatal region (i.e., caudate nucleus) associated with the same network (i.e., CON) in the Parkinsonian patients.
In aging brains, hyper-activations are interpreted as compensatory responses when older adults perform at the same level as younger adults or when increased brain activity is positively correlated with cognitive performance in older adults, but not in younger adults (Grady, 2012). Our patients had significantly lower MoCA scores compared with HCs, and there were no positive correlations between the network measures and MoCA scores, suggesting that the enhanced connectivity of the bilateral DLPFC and the new hubness of the caudate nucleus may be parallel “attempted compensation” (Grady, 2012) for trying to maintain an efficient utilization of neural resources (Grady, 2008) in the context of dopamine deficiency. On the other hand, the fact that MoCA subscores for executive function and attention (Nasreddine et al., 2005) in PD patients were not significantly different from those in HCs suggests that the enhanced connectivity may be successful instead of “attempted” compensatory responses for the particular cognitive functions.
The emergence of the right caudate nucleus as a new hub may be explained by the “hub failure” (Stam, 2014) of the left anterior insula. This model postulates that affected hubs reroute their connections to nodes at lower levels of hierarchy, resulting in the local emergence of new connections, which may also be an emergence of new hubs in the same network as observed in our data. The underpinning of the left lateralization of the affected anterior insular is unknown. However, the verbal memory dysfunction (Christopher et al., 2015) in our patients might be associated with the left lateralization. Because of the extensive connections between the anterior insula and the caudate nucleus, the deteriorating left anterior insula may have rerouted some of the connections to the right caudate nucleus.
Dopaminergic Modulation on Resting-State Functional Connectivity
Our PD patients underwent all study procedures in an on medication state in order to minimize the motion effects on the rsfMRI data. However, we acknowledge the confounding effects of dopaminergic medication on rsfMRI in PD patients. Dopamine replacement therapy typically normalizes aberrant functional connectivity patterns in sensorimotor nodes and network (Tahmasian et al., 2015) although there are differential effects of the medication on the normalization (Wu et al., 2009b). On the other hand, dopaminergic medications can have differential effects on cognitive nodes and networks. Our findings of reduced functional connectivity in the SMN nodes including the pre-SMA and mid-insula likely reflected the abnormalities that were not completely restored by dopaminergic medication while other more overt sensorimotor nodes were normalized. In addition, the pre-SMA that involves in cognitive control for action may be affected by dopamine replacement therapy and dopamine agonists differently from other sensorimotor nodes.
The failing hub function of the left anterior insula in the medicated patients suggests contribution of other pathology and/or inadequate effects of dopaminergic medication. Increased functional connectivity in the bilateral DLPFC that were likely compensatory responses may be associated with the on medication state as functional connectivity in the DLPFC is generally affected by dopaminergic medication. However, we did not find the association between the brain changes and LEDD. Further studies are needed to investigate the modulating effects of dopaminergic medication on network measures by contrasting them in the same cohort of PD patients in on and off medication states.
Heterogeneity of PD Patients
PD patients in the current study were clinically heterogeneous. Therefore, our findings may have reflected functional changes derived from those with certain clinical and cognitive/behavioral characteristics. For example, 13 of the patients (31%) had MoCA scores lower than 26. PD patients had significantly higher BDI II scores than HCs although only three patients had the scores higher than 13, and the depression levels of our patients were below the cur-off scores on average. The duration of disease ranges from 1 to 18 years. There were one patient at Hoehn and Yarhr stage 1, nine at stage 1.5, twenty-seven at stage 2, four at stage 2.5, and one at stage 3. We did not find any correlations between the graph measures and these clinical and cognitive/behavioral measures. However, further studies are needed to elucidate specific network changes in PD with clinical and cognitive/behavioral subtypes.
Summary and Conclusion
In summary, these findings highlight the diffuse changes in the nodal organization along with the regional hub disruption, which accounts for the distributed abnormalities across brain networks and clinical manifestations of PD. Some of these network alterations may be adaptive and counteracting the influence of neurotransmitter dysfunction and deposition of alpha-synuclein, resulting in a blurring of network boundaries required for distinct cerebral functions.
Author Contributions
YK, S-SC, and AS: study conception and design; YK: data acquisition: YK, S-SC, MC; data analysis; YK, S-SC, LC, MJ, CG, SC, MH, RM, CH, AL, SH, and AS: date interpretation; YK: manuscript drafting; S-SC, LC, MJ, CG, SC, MH, RM, CH, AL, SH, and AS: manuscript review and critique; YK, S-SC, LC, MJ, CG, SC, MH, RM, CH, AL, SH, and AS: manuscript final approval.
Funding
This study was supported by Canadian Institutes of Health Research (MOP 136778). AS is supported by the Canada Research Chair program. YK is supported by Canadian Institute of Health Research Doctoral Research Award.
Conflict of Interest Statement
The authors declare that the research was conducted in the absence of any commercial or financial relationships that could be construed as a potential conflict of interest.
Footnotes
References
Achard, S., and Bullmore, E. (2007). Efficiency and cost of economical brain functional networks. PLoS Comput. Biol. 3:e17. doi: 10.1371/journal.pcbi.0030017
Achard, S., Salvador, R., Whitcher, B., Suckling, J., and Bullmore, E. (2006). A resilient, low-frequency, small-world human brain functional network with highly connected association cortical hubs. J. Neurosci. 26, 63–72. doi: 10.1523/JNEUROSCI.3874-05.2006
Alexander, G. E., DeLong, M. R., and Strick, P. L. (1986). Parallel organization of functionally segregated circuits linking basal ganglia and cortex. Annu. Rev. Neurosci. 9, 357–381. doi: 10.1146/annurev.ne.09.030186.002041
Barnum, C. J., and Tansey, M. G. (2012). Neuroinflammation and non-motor symptoms: the dark passenger of Parkinson’s disease? Curr. Neurol. Neurosci. Rep. 12, 350–358. doi: 10.1007/s11910-012-0283-6
Behzadi, Y., Restom, K., Liau, J., and Liu, T. T. (2007). A component based noise correction method (CompCor) for BOLD and perfusion based fMRI. Neuroimage 37, 90–101. doi: 10.1016/j.neuroimage.2007.04.042
Berardelli, A., Rothwell, J. C., Thompson, P. D., and Hallett, M. (2001). Pathophysiology of bradykinesia in Parkinson’s disease. Brain 124, 2131–2146. doi: 10.1093/brain/124.11.2131
Bonnelle, V., Ham, T. E., Leech, R., Kinnunen, K. M., Mehta, M. A., Greenwood, R. J., et al. (2012). Salience network integrity predicts default mode network function after traumatic brain injury. Proc. Natl. Acad. Sci. U.S.A. 109, 4690–4695. doi: 10.1073/pnas.1113455109
Bonnet, A. M., Jutras, M. F., Czernecki, V., Corvol, J. C., and Vidailhet, M. (2012). Nonmotor symptoms in Parkinson’s disease in : relevant clinical aspects. Parkinsons Dis. 2012:198316.
Braak, H., Rub, U., Jansen Steur, E. N., Del Tredici, K., and de Vos, R. A. (2005). Cognitive status correlates with neuropathologic stage in Parkinson disease. Neurology 64, 1404–1410. doi: 10.1212/01.WNL.0000158422.41380.82
Bruno, J., Hosseini, S. M., and Kesler, S. (2012). Altered resting state functional brain network topology in chemotherapy-treated breast cancer survivors. Neurobiol. Dis. 48, 329–338. doi: 10.1016/j.nbd.2012.07.009
Bullmore, E., and Sporns, O. (2009). Complex brain networks: graph theoretical analysis of structural and functional systems. Nat. Rev. Neurosci. 10, 186–198. doi: 10.1038/nrn2575
Bullmore, E., and Sporns, O. (2012). The economy of brain network organization. Nat. Rev. Neurosci. 13, 336–349.
Cerasa, A., Hagberg, G. E., Peppe, A., Bianciardi, M., Gioia, M. C., Costa, A., et al. (2006). Functional changes in the activity of cerebellum and frontostriatal regions during externally and internally timed movement in Parkinson’s disease. Brain Res. Bull. 71, 259–269. doi: 10.1016/j.brainresbull.2006.09.014
Chang, L. J., Yarkoni, T., Khaw, M. W., and Sanfey, A. G. (2013). Decoding the role of the insula in human cognition: functional parcellation and large-scale reverse inference. Cereb. Cortex 23, 739–749. doi: 10.1093/cercor/bhs065
Chiong, W., Wilson, S. M., D’Esposito, M., Kayser, A. S., Grossman, S. N., Poorzand, P., et al. (2013). The salience network causally influences default mode network activity during moral reasoning. Brain 136, 1929–1941. doi: 10.1093/brain/awt066
Christopher, L., Duff-Canning, S., Koshimori, Y., Segura, B., Boileau, I., Chen, R., et al. (2015). Salience network and parahippocampal dopamine dysfunction in memory-impaired Parkinson disease. Ann. Neurol. 77, 269–280. doi: 10.1002/ana.24323
Christopher, L., Koshimori, Y., Lang, A. E., Criaud, M., and Strafella, A. P. (2014a). Uncovering the role of the insula in non-motor symptoms of Parkinson’s disease. Brain 137, 2143–2154. doi: 10.1093/brain/awu084
Christopher, L., Marras, C., Duff-Canning, S., Koshimori, Y., Chen, R., Boileau, I., et al. (2014b). Combined insular and striatal dopamine dysfunction are associated with executive deficits in Parkinson’s disease with mild cognitive impairment. Brain 137, 565–575. doi: 10.1093/brain/awt337
Criaud, M., Christopher, L., Boulinguez, P., Ballanger, B., Lang, A. E., Cho, S. S., et al. (2016). Contribution of insula in Parkinson’s disease: a quantitative meta-analysis study. Hum. Brain Mapp. 37, 1375–1392. doi: 10.1002/hbm.23109
Dosenbach, N. U., Fair, D. A., Miezin, F. M., Cohen, A. L., Wenger, K. K., Dosenbach, R. A., et al. (2007). Distinct brain networks for adaptive and stable task control in humans. Proc. Natl. Acad. Sci. U.S.A. 104, 11073–11078. doi: 10.1073/pnas.0704320104
Dosenbach, N. U., Nardos, B., Cohen, A. L., Fair, D. A., Power, J. D., Church, J. A., et al. (2010). Prediction of individual brain maturity using fMRI. Science 329, 1358–1361. doi: 10.1126/science.1194144
Engel, A. K., Gerloff, C., Hilgetag, C. C., and Nolte, G. (2013). Intrinsic coupling modes: multiscale interactions in ongoing brain activity. Neuron 80, 867–886. doi: 10.1016/j.neuron.2013.09.038
Gong, G., Rosa-Neto, P., Carbonell, F., Chen, Z. J., He, Y., and Evans, A. C. (2009). Age- and gender-related differences in the cortical anatomical network. J. Neurosci. 29, 15684–15693. doi: 10.1523/JNEUROSCI.2308-09.2009
Grady, C. L. (2008). Cognitive neuroscience of aging. Ann. N. Y. Acad. Sci. 1124, 127–144. doi: 10.1196/annals.1440.009
Grady, C. L. (2012). Trends in neurocognitive aging. Nat. Rev. Neurosci. 13, 491–505. doi: 10.1038/nrn3256
Gratwicke, J., Jahanshahi, M., and Foltynie, T. (2015). Parkinson’s disease dementia: a neural networks perspective. Brain 138, 1454–1476. doi: 10.1093/brain/awv104
Halliday, G. M., Leverenz, J. B., Schneider, J. S., and Adler, C. H. (2014). The neurobiological basis of cognitive impairment in Parkinson’s disease. Mov. Disord. 29, 634–650. doi: 10.1002/mds.25857
Hosseini, S. M., Hoeft, F., and Kesler, S. R. (2012). GAT: a graph-theoretical analysis toolbox for analyzing between-group differences in large-scale structural and functional brain networks. PLoS ONE 7:e40709. doi: 10.1371/journal.pone.0040709
Ikeda, A., Yazawa, S., Kunieda, T., Ohara, S., Terada, K., Mikuni, N., et al. (1999). Cognitive motor control in human pre-supplementary motor area studied by subdural recording of discrimination/selection-related potentials. Brain 122(Pt 5), 915–931. doi: 10.1093/brain/122.5.915
Kaiser, M., and Hilgetag, C. C. (2006). Nonoptimal component placement, but short processing paths, due to long-distance projections in neural systems. PLoS Comput. Biol. 2:e95. doi: 10.1371/journal.pcbi.0020095
Koshimori, Y., Segura, B., Christopher, L., Lobaugh, N., Duff-Canning, S., Mizrahi, R., et al. (2015). Imaging changes associated with cognitive abnormalities in Parkinson’s disease. Brain Struct. Funct. 220, 2249–2261. doi: 10.1007/s00429-014-0785-x
Kurth, F., Zilles, K., Fox, P. T., Laird, A. R., and Eickhoff, S. B. (2010). A link between the systems: functional differentiation and integration within the human insula revealed by meta-analysis. Brain Struct. Funct. 214, 519–534. doi: 10.1007/s00429-010-0255-z
Latora, V., and Marchiori, M. (2001). Efficient behavior of small-world networks. Phys. Rev. Lett. 87:198701. doi: 10.1103/PhysRevLett.87.198701
Madhyastha, T. M., Askren, M. K., Zhang, J., Leverenz, J. B., Montine, T. J., and Grabowski, T. J. (2015). Group comparison of spatiotemporal dynamics of intrinsic networks in Parkinson’s disease. Brain 138, 2672–2686. doi: 10.1093/brain/awv189
McColgan, P., Seunarine, K. K., Razi, A., Cole, J. H., Gregory, S., Durr, A., et al. (2015). Selective vulnerability of Rich Club brain regions is an organizational principle of structural connectivity loss in Huntington’s disease. Brain 138, 3327–3344. doi: 10.1093/brain/awv259
Menon, V., and Uddin, L. Q. (2010). Saliency, switching, attention and control: a network model of insula function. Brain Struct. Funct. 214, 655–667. doi: 10.1007/s00429-010-0262-0
Nagano-Saito, A., Martinu, K., and Monchi, O. (2014). Function of basal ganglia in bridging cognitive and motor modules to perform an action. Front. Neurosci. 8:187. doi: 10.3389/fnins.2014.00187
Nasreddine, Z. S., Phillips, N. A., Bédirian, V., Charbonneau, S., Whitehead, V., Collin, I., et al. (2005). The montreal cognitive assessment, MoCA: a brief screening tool for mild cognitive impairment. J. Am. Geriatr. Soc. 53, 695–699.
Pagonabarraga, J., Kulisevsky, J., Strafella, A. P., and Krack, P. (2015). Apathy in Parkinson’s disease: clinical features, neural substrates, diagnosis, and treatment. Lancet Neurol. 14, 518–531. doi: 10.1016/S1474-4422(15)00019-8
Passingham, R. E., Bengtsson, S. L., and Lau, H. C. (2010). Medial frontal cortex: from self-generated action to reflection on one’s own performance. Trends Cogn. Sci. 14, 16–21. doi: 10.1016/j.tics.2009.11.001
Picard, N., and Strick, P. L. (2001). Imaging the premotor areas. Curr. Opin. Neurobiol. 11, 663–672. doi: 10.1016/S0959-4388(01)00266-5
Rubinov, M., and Sporns, O. (2010). Complex network measures of brain connectivity: uses and interpretations. Neuroimage 52, 1059–1069. doi: 10.1016/j.neuroimage.2009.10.003
Seeley, W. W., Crawford, R. K., Zhou, J., Miller, B. L., and Greicius, M. D. (2009). Neurodegenerative diseases target large-scale human brain networks. Neuron 62, 42–52. doi: 10.1016/j.neuron.2009.03.024
Seeley, W. W., Menon, V., Schatzberg, A. F., Keller, J., Glover, G. H., Kenna, H., et al. (2007). Dissociable intrinsic connectivity networks for salience processing and executive control. J. Neurosci. 27, 2349–2356. doi: 10.1523/JNEUROSCI.5587-06.2007
Skidmore, F., Korenkevych, D., Liu, Y., He, G., Bullmore, E., and Pardalos, P. M. (2011). Connectivity brain networks based on wavelet correlation analysis in Parkinson fMRI data. Neurosci. Lett. 499, 47–51. doi: 10.1016/j.neulet.2011.05.030
Sporns, O. (2013). Network attributes for segregation and integration in the human brain. Curr. Opin. Neurobiol. 23, 162–171. doi: 10.1016/j.conb.2012.11.015
Sporns, O. (2014). Contributions and challenges for network models in cognitive neuroscience. Nat. Neurosci 17, 652–660. doi: 10.1038/nn.3690
Sporns, O., Honey, C. J., and Kotter, R. (2007). Identification and classification of hubs in brain networks. PLoS ONE 2:e1049. doi: 10.1371/journal.pone.0001049
Spreng, R. N., Sepulcre, J., Turner, G. R., Stevens, W. D., and Schacter, D. L. (2013). Intrinsic architecture underlying the relations among the default, dorsal attention, and frontoparietal control networks of the human brain. J. Cogn. Neurosci. 25, 74–86. doi: 10.1162/jocn_a_00281
Stam, C. J. (2014). Modern network science of neurological disorders. Nat. Rev. Neurosci. 15, 683–695. doi: 10.1038/nrn3801
Stam, C. J., de Haan, W., Daffertshofer, A., Jones, B. F., Manshanden, I., van Cappellen van Walsum, A. M., et al. (2009). Graph theoretical analysis of magnetoencephalographic functional connectivity in Alzheimer’s disease. Brain 132, 213–224. doi: 10.1093/brain/awn262
Tahmasian, M., Bettray, L. M., van Eimeren, T., Drzezga, A., Timmermann, L., Eickhoff, C. R., et al. (2015). A systematic review on the applications of resting-state fMRI in Parkinson’s disease: does dopamine replacement therapy play a role? Cortex 73, 80–105. doi: 10.1016/j.cortex.2015.08.005
Ter Minassian, A., Ricalens, E., Nguyen The Tich, S., Dinomais, M., Aubé, C., and Beydon, L. (2014). The presupplementary area within the language network. A resting state functional magnetic resonance imaging functional connectivity analysis. Brain Connect. 4, 440–453.
Tinaz, S., Lauro, P., Hallett, M., and Horovitz, S. G. (2016). Deficits in task-set maintenance and execution networks in Parkinson’s disease. Brain Struct. Funct. 1, 1414–1425.
Tomlinson, C. L., Stowe, R., Patel, S., Rick, C., Gray, R., and Clark, C. E. (2010). Systematic review of Levodopa Dose Equivalent reporting in Parkinson’s disease. Mov. Disord. 25, 2649–2653. doi: 10.1002/mds.23429
Trujillo, J. P., Gerrits, N. J., Vriend, C., Berendse, H. W., van den Heuvel, O. A., and van der Werf, Y. D. (2015). Impaired planning in Parkinson’s disease is reflected by reduced brain activation and connectivity. Hum. Brain Mapp. 36, 3703–3715. doi: 10.1002/hbm.22873
van den Heuvel, M. P., and Hulshoff Pol, H. E. (2010). Exploring the brain network: a review on resting-state fMRI functional connectivity. Eur. Neuropsychopharmacol. 20, 519–534. doi: 10.1016/j.euroneuro.2010.03.008
van den Heuvel, M. P., and Sporns, O. (2013). Network hubs in the human brain. Trends Cogn. Sci. 17, 683–696. doi: 10.1016/j.tics.2013.09.012
Wang, Y., Shima, K., Sawamura, H., and Tanji, J. (2001). Spatial distribution of cingulate cells projecting to the primary, supplementary, and pre-supplementary motor areas: a retrograde multiple labeling study in the macaque monkey. Neurosci. Res. 39, 39–49. doi: 10.1016/S0168-0102(00)00198-X
Wei, L., Zhang, J., Long, Z., Wu, G. R., Hu, X., Zhang, Y., et al. (2014). Reduced topological efficiency in cortical-basal Ganglia motor network of Parkinson’s disease: a resting state fMRI study. PLoS ONE 9:e108124. doi: 10.1371/journal.pone.0108124
Whitfield-Gabrieli, S., and Nieto-Castanon, A. (2012). Conn: a functional connectivity toolbox for correlated and anticorrelated brain networks. Brain Connect. 2, 125–141. doi: 10.1089/brain.2012.0073
Wu, T., Liu, J., Zhang, H., Hallett, M., Zheng, Z., and Chan, P. (2015). Attention to automatic movements in Parkinson’s disease: modified automatic mode in the striatum. Cereb. Cortex 25, 3330–3342. doi: 10.1093/cercor/bhu135
Wu, T., Long, X., Zang, Y., Wang, L., Hallett, M., Li, K., et al. (2009a). Regional homogeneity changes in patients with Parkinson’s disease. Hum. Brain Mapp. 30, 1502–1510. doi: 10.1002/hbm.20622
Keywords: Parkinson’s disease, bradykinesia, cognitive impairment, resting-state functional MRI (rsfMRI), brain network
Citation: Koshimori Y, Cho S-S, Criaud M, Christopher L, Jacobs M, Ghadery C, Coakeley S, Harris M, Mizrahi R, Hamani C, Lang AE, Houle S and Strafella AP (2016) Disrupted Nodal and Hub Organization Account for Brain Network Abnormalities in Parkinson’s Disease. Front. Aging Neurosci. 8:259. doi: 10.3389/fnagi.2016.00259
Received: 25 July 2016; Accepted: 19 October 2016;
Published: 10 November 2016.
Edited by:
Isidre Ferrer, University of Barcelona, SpainReviewed by:
Philippe Boulinguez, Claude Bernard University Lyon 1, FranceSimon J. G. Lewis, Brain and Mind Research Institute, Australia
Roberto Cilia, Parkinson Institute, Italy
Copyright © 2016 Koshimori, Cho, Criaud, Christopher, Jacobs, Ghadery, Coakeley, Harris, Mizrahi, Hamani, Lang, Houle and Strafella. This is an open-access article distributed under the terms of the Creative Commons Attribution License (CC BY). The use, distribution or reproduction in other forums is permitted, provided the original author(s) or licensor are credited and that the original publication in this journal is cited, in accordance with accepted academic practice. No use, distribution or reproduction is permitted which does not comply with these terms.
*Correspondence: Yuko Koshimori, eXVrby5rb3NoaW1vcmlAbWFpbC51dG9yb250by5jYQ==; eWtvc2hlZTMyMUBnbWFpbC5jb20=