- 1Department of Computer Science, University of Colorado Boulder, Boulder CO, United States
- 2Department of Aerospace Engineering Sciences, University of Colorado Boulder, Boulder CO, United States
- 3Draper, Cambridge MA, United States
Introduction: Future concepts for airborne autonomy point toward human operators moving out of the cockpit and into supervisory roles. Urban air mobility, airborne package delivery, and military intelligence, surveillance, and reconnaissance (ISR) are all actively exploring such concepts or currently undergoing this transition. Supervisors of these systems will be faced with many challenges, including platforms that operate outside of visual range and the need to decipher complex sensor or telemetry data in order to make informed and safe decisions with respect to the platforms and their mission. A central challenge to this new paradigm of non-co-located mission supervision is developing systems which have explainable and trustworthy autonomy and internal decision-making processes.
Methods: Competency self-assessments are methods that use introspection to quantify and communicate important information pertaining to autonomous system capabilities and limitations to human supervisors. We first discuss a computational framework for competency self-assessment: factorized machine self-confidence (FaMSeC). Within this framework, we then define the generalized outcome assessment (GOA) factor, which quantifies an autonomous system’s ability to meet or exceed user-specified mission outcomes. As a relevant example, we develop a competency-aware learning-based autonomous uncrewed aircraft system (UAS) and evaluate it within a multi-target ISR mission.
Results: We present an analysis of the computational cost and performance of GOA-based competency reporting. Our results show that our competency self-assessment method can capture changes in the ability of the UAS to achieve mission critical outcomes, and we discuss how this information can be easily communicated to human partners to inform decision-making.
Discussion: We argue that competency self-assessment can enable AI/ML transparency and provide assurances that calibrate human operators with their autonomous teammate’s ability to meet mission goals. This in turn can lead to informed decision-making, appropriate trust in autonomy, and overall improvements to mission performance.
1 Introduction
Humans stand to benefit greatly from working cooperatively with autonomous systems that can operate in potentially high-risk situations or perform complex and repetitive tasks. However, reliance on robots and autonomous systems should only occur when the human operator is confident that the system can adequately perform the tasks at hand. This willingness to rely is referred to as trust, a subjective measure which, in part, is a function of human belief in an agent’s competency, as well as belief in the predictability and “normality″ of the tasking situation (among many other factors) (Israelsen and Ahmed, 2019). In operational contexts, it has been established that the trust developed by a user in an autonomous system may potentially result in an inaccurate understanding of that system’s capabilities (Dzindolet et al., 2003). Such misunderstanding raises the potential for the improper tasking of the agent, and subsequent misuse, abuse, or disuse of autonomy in deployment scenarios. One strategy for encouraging appropriate human trust is for an autonomous robot to report its own perspective on tasks at hand (McGuire et al., 2018; McGuire et al., 2019). If done correctly, a user could better judge whether the robot is sufficiently capable of completing a task within desired delegation parameters, thus adjusting user expectations of performance in a manner suitable to the situation at hand. This idea lies at the core of a wide spectrum of algorithmic strategies for generating soft assurances, which are collectively aimed at “trust management” (Israelsen and Ahmed, 2019).
Remote information gathering tasks represent an increasingly important use case for autonomous systems, spanning diverse domains such as deep space exploration, scientific data collection, environmental monitoring, agriculture, infrastructure inspection, and security and defense. In this study, we focus on the use case of intelligence, surveillance, and reconnaissance (ISR), a term commonly used to characterize missions that employ sensors to gather specifically valuable information. It is often subdivided depending on the intended use of the data gathered by the mission, such as in the defense domain—theater ISR, tactical ISR, and human-portable or small-unit ISR (OASD, 2018). ISR at all levels is becoming increasingly automated and autonomous and is proliferating across domains. Uncrewed aircraft systems (UAS) are being used to surveil battlefields and target locations (Chua, 2012; Cook, 2007), help detect forest fires (Yuan et al., 2015; Sudhakar et al., 2020; Julian and Kochenderfer, 2019), and assess areas after a natural disaster (Ezequiel et al., 2014; Erdelj et al., 2017). In these applications, the human operator is given the role of a supervisor or teammate to one or more ISR platforms.
Of particular interest is small-unit ISR utilizing autonomous UAS. Here, the ISR platforms are generally person-portable and are either launched from a small runway, thrown, or catapulted into the air. They can be fixed- or rotary-wing and are capable of carrying small payloads. They have basic onboard autonomy, which enables path planning and waypoint following. They may also be limited in flight due to size, weight, and power (SWaP) constraints and can be susceptible to inclement weather. Despite some limitations, these platforms can be invaluable in many applications—with little prep time, a small UAS can be deployed to help a fire crew search for the closest fire or help a squad of soldiers safely recon beyond the next hill.
The challenge with small-unit ISR is that, compared to commercial or military pilots (or even pilots of larger platforms like the MQ-1 Predator or MQ-9 Reaper), small UAS operators may receive less training on the operation of their platforms, which could impact trust. For example, soldiers operating the MQ-7 RAVEN require only 10 days of training1. Because the operators of these smaller ISR platforms receive less training prior to deployment, they may have limited understanding of the capabilities and characteristics of the aircraft in off-nominal situations (for example, in poor weather conditions). Additionally, these platforms may not possess soft assurances to calibrate trust. Any misunderstanding of capabilities could be amplified by the high stress and workload environments in which soldiers or disaster responders may find themselves, where mis-calibrated trust in a system can be costly, if not mission-ending. A competency-aware autonomous ISR platform could prevent these misunderstandings by calibrating operator trust through outcome assessments and competency reporting.
We here describe an application of the factorized machine self-confidence (FaMSeC) framework, which allows autonomous algorithmic decision-making agents to generate soft assurances in the form of introspective competency reports based on the concept of machine self-confidence (i.e., machine self-trust). We then discuss the generalized outcome assessment (GOA) factor within the FaMSeC framework and how it can be formulated for reinforcing learning-based autonomous small UAS performing simulated ISR missions. As an extension of our previous research (Conlon et al., 2022a), which only considered limited single-target mission scenarios and narrow sets of competencies, we here present a deeper analysis of the performance of GOA for a broader range of mission-relevant competencies as well as its ability to quantify an autonomous aircraft’s competencies in a multi-target ISR mission under varying weather conditions featuring a stochastic wind model that must be accounted for in a learned probabilistic world model. We close with a discussion of several challenges in bringing competency-awareness to live platforms and propose directions for future work.
2 Background and related work
This section first reviews reinforcement learning and probabilistic world modeling as a framework for autonomous decision making. It then provides a brief overview of the approach to mission competency assessment for probabilistic decision-making agents. These ideas are developed further in the next section to describe their application for small UAS ISR mission contexts.
2.1 Autonomous decision making and probabilistic world modeling
Probabilistic algorithms for decision-making under uncertainty have been attracting wide attention within the aerospace community (Kochenderfer, 2015). In addition to their deep connections to conventional state-space optimal control and estimation strategies for guidance, navigation, and control (GNC), probabilistic decision-making algorithms based on Markov decision processes (MDPs), partially observable MDPs, and reinforcement learning (RL) offer an attractive and unified framework for enabling autonomy onboard vehicles which must respond to off-nominal events while coping with complex and uncertain dynamics, observations, and model parameters. RL is of particular interest in many autonomous vehicle applications for its ability to simultaneously optimize control/guidance laws and learn complex dynamics models for non-trivial tasks online via repeated experiences (i.e., learning episodes which can incorporate simulated as well as real data). For instance, in relation to the USA ISR domain considered here, much recent research has considered RL-based training of UAS vehicle guidance and control laws to optimize various mission-level objective functions including high-quality information gathering, low-error target tracking, opportunistic communications, and minimum energy consumption (Abedin et al., 2020; Goecks and Valasek, 2019; Jagannath et al., 2021; Mosali et al., 2022; van Wijk et al., 2023)
The objective in RL (Sutton and Barto, 2018) is to select actions that maximize the total reward during a given learning episode. It is formalized as an MDP consisting of the following model components. Let
The methods in RL can be separated into model-free and model-based according to what is being learned by the agent. While model-free methods directly learn the optimal policy
To parameterize the world model
The planning process follows a model predictive control (MPC) paradigm, which allows the agent to plan over some prespecified time horizon while continuously incorporating newly received observations. The MPC also provides the flexibility to use any algorithm, such as a tree-based planner, that can aggregate all the possible actions or a simple random-sampling-based method like random shooting (Nagabandi et al., 2019) to generate action sequences. The focus of this work will be on using a simple random-sampling-based planning algorithm which, while likely to introduce uncertainty into the planning process, alleviates the need to carefully design the planner. This planner is used with the learned world model to predict the future states and generate action sequences. Then, a user-defined, task-specific reward function is used to rank the action sequences from best to worst in maximizing the reward, and typically only the first action from the best action sequence is executed in the real environment, resulting in the next real observation from the environment.
The selection and tuning of the reward function
2.2 Mission competency assessment
The introduction of sophisticated learning-based decision-making autonomy ostensibly yields many benefits for human end-users of small UAS. Well-designed decision-making autonomy can not only significantly enhance overall mission performance by using rigorous data-driven optimization to fully utilize vehicle and sensor platform capabilities but can also alleviate human users of “dull, dirty, and dangerous” tasks that are physically and mentally demanding. For example, UAS pilots and sensor specialists in search-and-rescue missions often need to work together to remotely pilot a single vehicle while paying attention to data returns from a variety of onboard sensor payloads and also routinely monitor intelligence feeds and other communication channels to adapt mission strategies in dynamic time-critical situations across multiple hours or days (Ray et al., 2024).
Nevertheless, the issue of trust in autonomy has been widely noted as an important barrier to the wider adoption of vehicle autonomy for such applications (Devitt, 2018; Shahrdar et al., 2019). While the topic of trust in human–machine interaction is far too complex to fully discuss here, it is worth noting that the perceived situation normality, predictability, and competency of autonomous systems play a key role in calibrating end-user trust; this in turn has motivated research on a wide range of soft algorithmic assurances for trust calibration and management in user–autonomous system interactions (Israelsen and Ahmed, 2019). Whereas end-users will typically not be technology experts in topics such as vehicle systems, RL, and deep learning, they often will still have valuable domain knowledge that can be usefully leveraged to design soft assurances. Of particular interest here is how soft assurances can be developed to provide a more accurate representation of a learning-based UAS’s actual mission capabilities so that end-users can better calibrate their trust in a system and assign tasks that remain within its competency limits.
This study focuses on one particular type of soft assurance for learning-based UAS decision-making autonomy known as machine self-confidence. This is defined as an autonomous agent’s own perceived degree of competency to execute tasks within desired parameters while accounting for uncertainties in its environment, states, and limited reasoning/execution capabilities (Aitken, 2016; Israelsen, 2019). Note that this definition not only captures “irreducible” uncertainties that naturally arise in particular tasks (i.e., aleatoric uncertainties such as sensor noise) and “reducible” uncertainties that stem from ignorance of model details (i.e., epistemic uncertainties from lack of available data), but importantly, it also considers “meta-uncertainties” related to the agent’s ability to process, acquire, and act on (uncertain) information. Thus, machine self-confidence not only assesses the degree to which an agent is uncertain about its own or the environment’s state but also the degree to which its models of uncertainty and actions derived from these are suited to the task at hand (Hutchins et al., 2015; Sweet et al., 2016). Colloquially, this may be thought of as an expression of “machine self-trust”, akin to the self-trust/self-confidence expressed to a supervisor by a human subordinate who has been delegated with executing particular tasks.
Aitken (2016), Israelsen et al. (2019), and Israelsen (2019) developed the factorized machine self-confidence (FaMSeC) framework to consider the computation of several interrelated (and non-exhaustive/asymmetric) “problem-solving meta-factors” that enable autonomous decision-making agents to generate machine self-confidence assessments in the context of executing tasks described by Markov decision processes (MDPs). For instance, for agents that reason according to policies governed by standard model-based MDPs, three key meta-factors can be quantitatively evaluated relative to user expectations:
1. Outcome assessment (OA): do the sets of possible events, rewards, costs, utilities, etc. for decisions governed by a policy lead to a desirable landscape of outcomes under uncertainty?
2. Solver quality: are the approximations used by the system for solving decision-making problems appropriate for the given task and model?
3. Model validity: are the agent’s learned/assumed models and training data used for decision-making sufficient for operating in the real world?
Computed scores for each factor can be mapped to notional scales with upper/lower bounds, where the lower bound gives a shorthand indication of “complete lack of confidence” (i.e., some aspect of task, environment, or operational context falls completely outside the agent’s competency boundaries) and the upper bound indicates “complete confidence” (i.e., all aspects are well within system’s competency boundaries). In Israelsen et al. (2019) and Israelsen (2019), a human user study showed that FaMSeC self-confidence computation and reporting improved the ability of human supervisors to assign or reject tasks within/outside the competency boundaries of simulated autonomous vehicles conducting delivery tasks in uncertain adversarial environments. More recent experiments have provided similar findings using the FaMSeC framework with non-learning-based stochastic planning algorithms in both software and hardware simulations of autonomous ground robots performing navigation tasks in uncertain environments (Conlon et al., 2024; Conlon et al., 2022b).
The problem of applying machine self-confidence concepts to learning agents remains open and challenging. The FaMSeC formulation for MDPs naturally extends to a variety of autonomous agents, such as those that must rely on reinforcement learning (RL) to learn optimal policies and behaviors by interacting with uncertain environments. Mellinkoff et al. (2020) considered a simple model-based RL agent, leveraging the FaMSeC outcome assessment metric to modulate exploration/exploitation in sparse reward environments based on degree of confidence in completing tasks. While promising, this approach requires the agent to have well-defined and reasonably small, computationally tractable a priori probabilistic models of its task environment and dynamics. This is not feasible for many autonomous learning applications that must rely on more complex black-box models, such as widely used state-of-the-art deep learning neural network models.
In this study, we restrict attention to probabilistic model-based reinforcement learning (MBRL) using deep neural networks. Despite the theoretical connections between MBRL and MDPs, the extension of FaMSeC to deep RL applications is still not entirely obvious or straightforward. Among the most notable issues, current strategies for computing FaMSeC metrics within MDPs assume the availability of (1) tractable, closed-form state transition probability distributions, (2) fixed policy functions or tables, and (3) static well-defined reward functions and utilities.
In RL applications, assumption (1) is invalid for continuous state spaces with complex dynamics or a large number of states, such that state transition densities cannot be expressed in closed form. Assumption (2) is not valid when considering online or constraint-based decision-making strategies, such as model predictive control. Lastly, assumption (3) is invalid since reward functions for RL problems are often highly tuned to achieve desirable behaviors. Arbitrarily rescaling reward values can drastically alter the interpretation and sensitivity of metrics such as outcome assessment and solver quality, which Aitken (2016) and Israelsen (2019) originally defined via the reward distribution associated with a given policy. In this study, we consider how principles underlying the FaMSeC metrics can be extended to bridge these (and other) gaps.
Self-assessment of an autonomous agent’s capabilities for the given task is critical for collaborative efforts with a human supervisor. The self-assessment approach leveraged focuses on outcome assessment (OA), one of five FaMSeC factors developed by Aitken (2016), Israelsen (2019), and Israelsen et al. (2019), which seeks to encourage appropriate human trust in an autonomous system. For MDP-based problems, OA describes the confidence that a user-specified margin of success can be achieved based on a set of potential rewards governed by a policy
This formulation results in OA
Notwithstanding, there are some notable weaknesses to outcome assessment that can be addressed in this MBRL framework. Cumulative rewards do not contain information relative to the specific or intermediary outcomes of a trajectory.
GOA is computed by running
Given a
Next, the value for GOA is computed through a logistic function, such that GOA
Finally, these GOA values
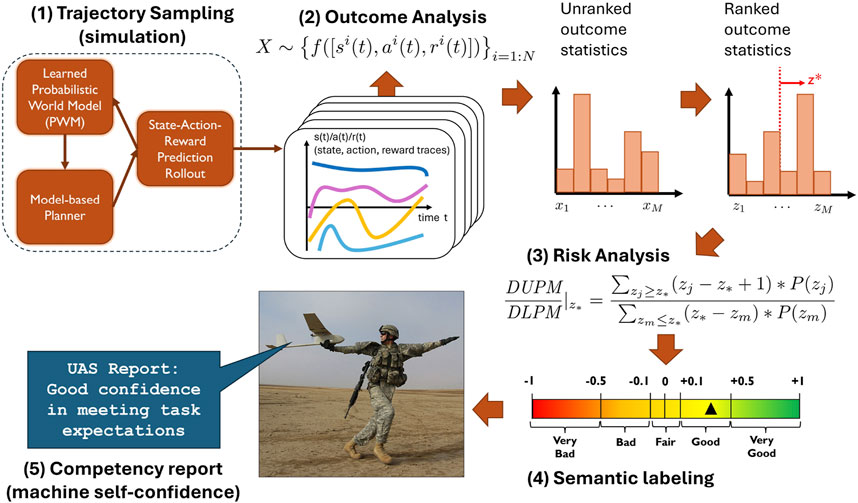
Figure 1. Diagram of GOA computation. (1) Simulation traces sampled from a learned world model. (2) Outcome statistics gathered and converted into ranked equivalence classes. (3) DUPM/DLPM statistic is calculated based on
3 Development of competency assessment in ISR missions
To explore our approach of UAS competency self-assessment for ISR missions, we developed a simulated scenario where a human–UAS team was tasked with surveying several target sites within a broader mission area. The team’s collective goal was for the UAS to visit and collect data on as many sites as possible across two missions. The UAS itself is a lightweight, portable, and easily launched platform, similar to the MQ-7 RAVEN. However, due to its small size, it can be adversely impacted by off-nominal environmental conditions, particularly winds at altitude. The first mission’s daily weather report predicts calm (nominal) winds, while the second mission’s daily forecast is for adverse (off-nominal) winds. Before deploying the UAS, the human supervisor needs to know whether the UAS is capable of achieving their desired outcomes of mission success.
In this section, we discuss the development of a learning-based autonomous UAS capable of self-assessing its mission competencies using GOA. While we note that the MQ-7 RAVEN is capable of flight times in excess of 60 min and operating in a variety of environmental conditions (Pomranky, 2006), we constrain our experiment to a fixed 60 s mission length under both calm and adverse constant wind conditions. This shorter mission duration and limited experimental conditions were chosen to balance model training complexity while still being able to demonstrate GOA analysis of outcome statistics targeted at an ISR platform operating within a realistic mission environment. We believe this contribution to be an important first step in developing methods for future human–UAS teams to utilize competency reports derived from GOA to inform decision-making and improve mission performance in high-risk and uncertain environments.
3.1 Simulation and modeling
Gazebo is a highly customizable, open-source, 3D dynamic multi-robot environment (Koenig and Howard, 2004). It supports a wide variety of platform and sensor models, customized environments, and interfaces with the Robot Operating System (ROS). In addition to modeling the dynamics of our UAS platform, we leverage Gazebo’s high-fidelity modeling of terrain, lighting, and various man-made structures to create the realistic mission area for our experiments. Much of our customization took the form of Gazebo plugins, which are shared libraries loaded into Gazebo that enable fine-grained control of most aspects of the simulator. Our plugins modeled the platform’s dynamics, its battery, and environmental winds. All communication to and from the plugin was via ROS messaging. Upon start-up, the plugin paused Gazebo and initialized the models with initial UAS state
3.1.1 Platform model
Our platform was modeled as a Techpod fixed-wing UAS from the RotorS package (Furrer et al., 2016). This UAS has a wingspan of 2.6 m, a body length of 1.1 m, and a mass of 2.6 kg. We commanded control surface deflections
3.1.2 Battery model
The battery level at time
—where
3.1.3 Wind model
The wind model simulates the wind disturbances affecting the platform. The model generates wind velocity
3.1.4 Probabilistic world model
To develop learning-based autonomous UAS, we formulated the problem of predicting UAS state dynamics given previous states, battery information, and wind information through model-based reinforcement learning (Figure 2). The UAS state consists of both translational
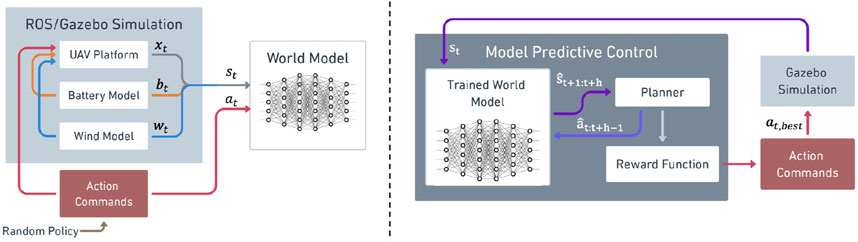
Figure 2. Training procedure (left) showing random data being generated and used to train the world model. The planning procedure (right) shows the trained model being used in conjunction with model predictive control to choose optimal actions for the UAS to execute.
We model the UAS action space as the instantaneous change in control surfaces and thrust vector. Our six-dimensional action space is shown in Equation 3.
One of the main challenges with training neural network world models is ensuring that the input and output data are continuous, normalized, and generalizable to previously unseen data drawn from the training distribution. The absolute positions and Euler angles in the state representation are particularly challenging for these reasons. If using the body frame instead, the gravity vector would have to be explicitly included. We thus formulate the inputs and outputs to the world model in what we call the model frame (Figure 3), where gravity is constant. We predict the changes in pose rather than the absolute vehicle pose and then integrate the neural network output. As the coordinate frame changes and integration is fully differentiable, the loss function used for training can still be based on the error in the main state representation. By carefully crafting these data representations for the neural network, we enable the optimization to result in reasonable backpropagated gradients, allowing for stable training and avoiding issues of vanishing or exploding gradients (Bengio et al., 1994; Pascanu et al., 2013).
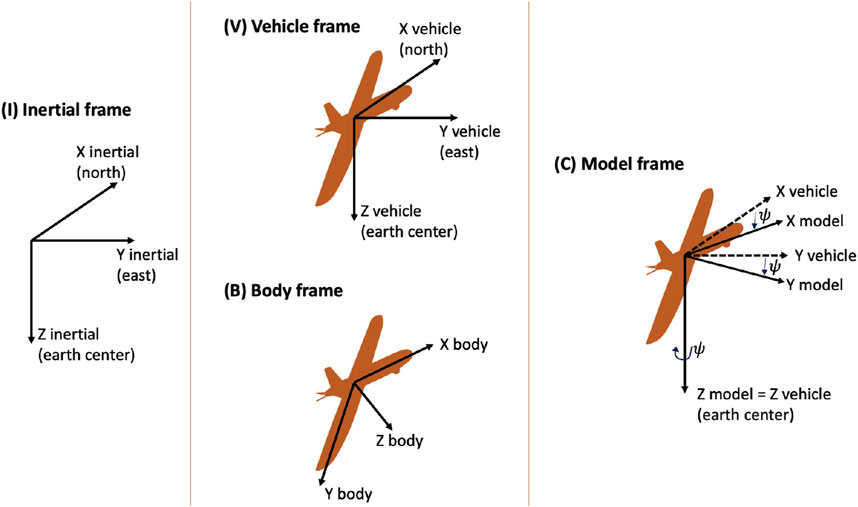
Figure 3. Different coordinate frames: (left) inertial frame with origin at Earth’s center; (middle, top) vehicle frame with same axes as inertial frame but origin at UAS center of mass; (middle, bottom) body frame with origin at UAS center of mass; (right) model frame, used in our representation, which is an intermediate rotation between vehicle and body frame with rotation only along the yaw axis.
The pre-processing step for the data input to the RNN world model involves first transforming the vehicle pose and velocity into the model frame and then normalizing the model input representation to have a mean of 0 and standard deviation of 1 over all trajectories.
The post-processing step for the data output from the RNN world model consists of first transforming the change in vehicle pose back into the inertial frame. We then integrate the change in vehicle pose to determine the predicted pose in the inertial frame. Next, we transform the vehicle pose velocity back into the body frame. Finally, we look up the predicted vehicle pose in a wind map to determine the predicted wind vector.
As training data, we first collect 40,000 trajectories by executing random actions in the Gazebo simulation. These trajectories are collected at 1 Hz for a total of 1 min, resulting in 60 length sequences that consist of information on all of the states and actions. Following data collection, we train an RNN that uses a GRU cell to carry through the information from one time step to the next. The model is trained with a depth of 10, meaning that for any given state, the network is asked to predict ten time-steps ahead. The loss then takes on the form shown in Equation 4, where the total loss is the sum of individual losses
where
is the mean absolute error of the following components of the predicted and true states.
During the planning phase, we use our trained world model in conjunction with the filtering and reward-weighted refinement planner (Nagabandi et al., 2019) to predict a sequence of states given a sequence of actions. Within our MPC setup, the re-planning occurs at every time-step so that only the first action out of the best action sequence as chosen by the reward function is applied to the environment.
3.1.5 Reward modeling
We modeled reward
Each individual reward function
rewards the agent for capturing a target. A target is considered captured if the UAS moves within a 25 m radius of the given target; once a target is captured, it cannot be captured again (i.e., no additional reward is given). The safely reward penalizes the UAS for crashing into the ground plane (altitude = 0) and takes the form
The battery level reward
rewards the UAS for conserving the battery life which is expended by the control actions. For our experiments, we tuned our reward parameters to
4 Experimental design
We designed two experiments to investigate the ability of generalized outcome assessment (GOA) to evaluate the competency of our autonomous UAS in a time-constrained multi-target visit task under differing wind conditions. Wind speed and its impact on the platform’s dynamics is an important factor a user must consider when deciding if and how to deploy a fixed-wing UAS for an ISR mission. Here, we investigated the ability of GOA to assess the UAS competency across two wind-speed experiments. Calm wind represents a nominal operating environment with a constant wind velocity of
4.1 Multi-target ISR task
We designed a relevant ISR mission where we tasked our autonomous fixed-wing UAS to fly to different target areas within a larger mission area under varying wind conditions. There were six targets available to the platform to visit. Each target had a fixed
In each episode, the UAS began at a fixed
The goal of the task was for the UAS to maximize the number of targets visited (visiting between zero and six targets) while maintaining safe altitude and conserving battery level. We decomposed this high-level goal into three mission outcomes of interest to a potential operator: (1) battery-level conservation, (2) time to the first visit of any of the six targets, and (3) total targets visited of out the six. Given these outcomes of interest, an operator may, before deploying the UAS for this mission, want to know whether the UAS can (1) complete the mission with
4.2 Competency assessment
To quantify the autonomous UAS’s predicted competency in achieving each outcome, we implemented the GOA method covered in Section 2.2. For each experiment (calm or adverse wind), GOA sampled 25 rollouts from the Docker-based Gazebo simulation of the UAS executing the mission. This resulted in a set of 25 60-second trajectory traces that the algorithm then analyzed with respect to each of the three outcomes of interest.
We mapped the raw battery levels to GOA
4.3 Hardware resources
All experiments and data analyses were performed on a Dell Precision Laptop running Ubuntu 20.04. The laptop was equipped with an Intel i7 4 core processor, 16 GB RAM, and Nvidia RTX A3000 GPU. All simulation software (code, simulator, and associated scripts) were run within a Docker container. We used the World Model within the Model Predictive Control framework as covered in 3.1.4. For all simulations, we used a 60 s mission time where each simulation step was 1.0 s.
5 Results
We analyzed the generalized outcome assessment (GOA) for both calm and adverse wind conditions. With respect to the algorithm’s computational cost, the two main processes underlying GOA are (1) trajectory sampling (simulation) and (2) analysis to include both outcome analysis and risk analysis (Figure 1). Across both experiments, we found that the time taken to sample the set of trajectories was the primary contributor to computational cost
5.1 Wind conditions
Our calm wind experiment simulated wind speeds of
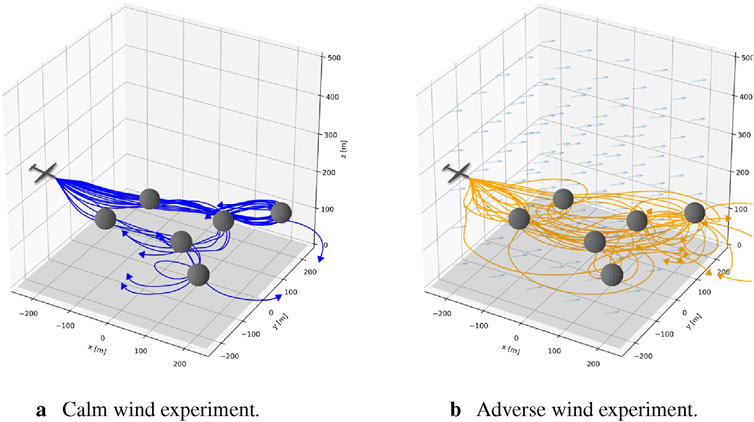
Figure 4. Figures of the calm wind experiment (blue trajectories (A)) and adverse wind experiment (orange trajectories (B)) showing the predicted paths of the UAS as it attempts to capture as many of the six targets (gray orbs) as possible within the mission time limit. UAS silhouette denotes the platform’s starting position, while the trajectory arrows show its direction of travel at the end of the task. (A) Calm wind experiment. (B) Calm wind experiment.
5.2 Comparison of outcome distributions and outcome assessments
In this section, we present an analysis of the outcome distributions for each of the three outcomes of interest: battery level, target timing, and targets visited. For each outcome of interest, we discuss the UAS-predicted outcome distributions and how they each translate to the GOA across a range of potential
5.2.1 Battery level outcome
Our first outcome of interest is battery level. Because a UAS may have to contend with uncertainties in task, environment, weather, and/or potential adversaries, keeping a battery reserve is critical to mission success. An operator may want to know whether the UAS is capable of completing the mission with
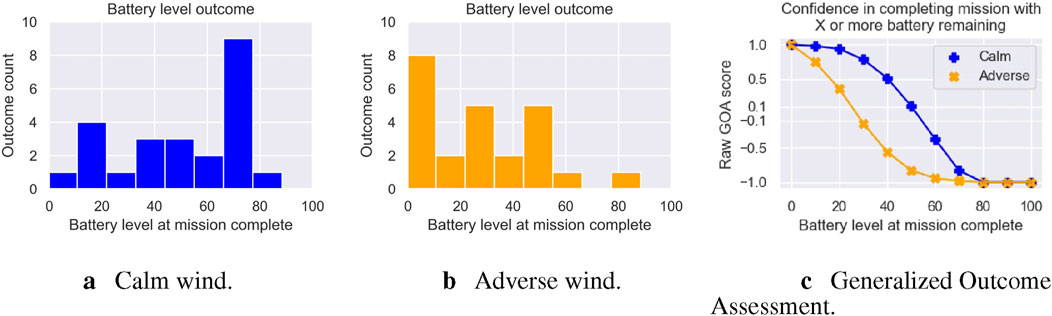
Figure 5. Figures showing battery level outcome distributions across both calm (blue (A)) and adverse (orange (B)) wind experiments. (C) GOA response across all possible ending battery levels, estimating generally higher confidence in the UAS completing the mission with more battery remaining in the calm experiment compared to the adverse experiment. (A) Calm wind. (B) Adverse wind. (C) Generalized outcome assessment.
Figure 5C shows how these two outcome distributions translate to raw GOA scores. We can see that in both experiments, GOA estimated very good confidence
5.2.2 Target timing outcome
Our second outcome of interest is target timing. For both mission planning and collaborative tasking, it is critical to understand the platform’s time on target. We measure time-on-target, or target timing, as the time in seconds for the UAS to visit its first target. Here, an operator may ask whether the UAS is capable of arriving at the first target within
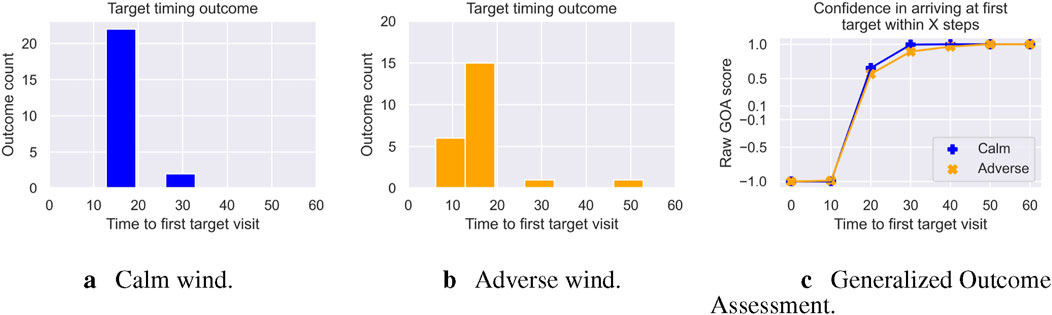
Figure 6. Figures showing the time to first target outcome distributions across both calm (blue (A)) and adverse (orange (B)) wind experiments. (C) GOA response across all possible times to arrive at the first target; UAS has generally higher confidence in visiting the first target faster in the calm experiment than in the adverse experiment. (A) Calm wind. (B) Adverse wind. (C) Generalized outcome assessment.
Figure 6C shows the GOA plot for this outcome of interest. We can see both experiments track a similar curve, with the calm experiment showing slightly higher confidence. In both the calm and adverse wind experiments, GOA would estimate very bad confidence
5.2.3 Targets visited outcome
Our third outcome of interest is total targets visited. This outcome gives users a sense of how well the UAS will be able to maximize target visits. We measured this as the raw count of targets visited during the 60-s mission. Once a target was visited, it was not counted again. Here, an operator could ask whether the UAS could visit
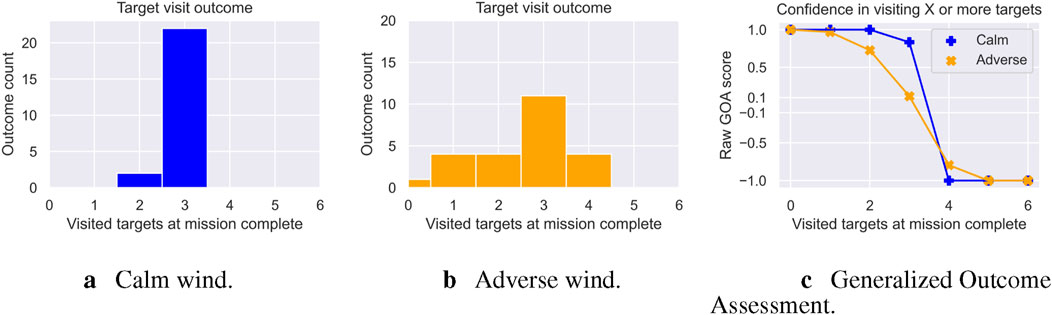
Figure 7. Total targets visited outcome distributions across both calm (blue (A)) and adverse (orange (B)) wind experiments. (C) GOA response across all possible targets, showing that the UAS has generally higher confidence in visiting more targets in the calm experiment than in the adverse experiment. (A) Calm wind. (B) Adverse wind. (C) Generalized outcome assessment.
This difference in variance impacts the shape of the GOA curve, and in turn, the level of confidence reported to the operator (Figure 7C). Under the calm wind experiment, GOA estimates very good confidence
6 Discussion and future work
Our results indicate that the FaMSeC generalized outcome assessment can be used to assess the competency of an autonomous UAS executing tasks characterized by environmental uncertainty and mission time pressure. We observed that a UAS tasked with operating in calm wind conditions outperformed the same UAS operating in adverse wind conditions across three outcomes of interest to a human supervisor: battery level, target time, and targets visited. The fact that the UAS showed superior performance in the calm wind conditions is expected and obvious. However, given these outcome distributions, we then showed that they can be analyzed using GOA to compute a metric of machine self-confidence in achieving each outcome of interest. We demonstrated that this competency quantification is impacted by both location and the shape of the predicted outcome distributions—GOA captured uncertainties in predicted outcomes that can both add risk and impact performance. We found that the high performance of the UAS during the calm wind experiment led to overall higher GOA confidence in achieving each mission outcome of interest than the UAS during the adverse wind experiment.
As an example of how this may translate to a human–machine team, where the human is supervising the autonomous UAS, consider the human supervisor requesting the following assessments from the UAS in each experiment:
1. “Will the UAS complete the mission with
2. “Will the UAS visit the first target within 20 seconds?” Both the calm wind UAS and the adverse wind UAS would thus report back very good confidence
3. “Will the UAS visit 4 or more targets?” Both the calm and adverse wind UAS would thus report back very bad confidence (
While we are investigating a small set of potential outcomes in a simplistic ISR mission, reported GOA self-assessments should help calibrate human users with their autonomous system’s capabilities and lead to improved decision-making with respect to mission execution and platform employment. Previous research has found evidence that communicating competency self-assessments in the ground domain can lead to improvements in user decision-making, with downstream improvements to performance and calibrated trust in the system (Israelsen et al., 2019; Conlon et al., 2022b); however, it is not obvious how such information should be presented to autonomous UAS supervisors, who may be managing far faster and more dynamic platforms. One potential direction for future research is to validate competency self-assessments with GOA in the aerial domain using live platforms with humans in the loop.
Moving toward competency self-assessments for live aerial platforms poses several challenges. The first is the need to quantify and report in situ changes to competency. Once a platform is in flight and executing the mission, any a priori self-assessments, such as those presented in this work, could be invalidated by changes in the environment (e.g., weather) or the mission (e.g., additional tasking) or the addition of cooperative and non-cooperative platforms (e.g., other aircraft in the area, adversaries). In order to keep human supervisors calibrated to the platform’s ability to achieve favorable mission outcomes, the platform should be able to update the assessment as new information becomes available. While such competency updating has been shown to be effective for ground platforms that can safely stop and re-assess (Conlon et al., 2024), the air domain presents a challenge in that fixed-wing platforms that rely on forward motion to generate lift do not have the luxury of temporarily stopping operation while the system and the supervisor decide how to proceed.
This leads to an additional challenge, in that competency self-assessments, particularly those executed in situ, need to be computationally fast. We found in this study that the computational bottleneck is in simulating mission rollouts to generate the outcome distributions. We found that this is a function of several parameters, including task time horizon, mission complexity, and the requested number of rollouts. With respect to efficient sampling, research has shown that these distributions can be generated by approximating and intelligently “reusing” rollouts (McGinley, 2022). However, the proposed method has not been directly applied to the aerial domain and may not directly translate to online assessments where previous rollouts can easily be invalidated due to in situ changes. Given this, we believe there is ample opportunity to investigate efficient sampling of probabilistic world models, simulations, and digital twins such as those used in this study.
7 Conclusion
As a step toward developing competency-aware decision-making autonomous agents, we developed and analyzed a simulated learning-based autonomous UAS that leverages model-based reinforcement learning to execute a multi-target intelligence surveillance, and reconnaissance (ISR) task. Our UAS used a learned probabilistic world model of its operating environment in conjunction with a stochastic model-based planner to choose optimal actions given a reward function that prioritizes visiting targets, conserving battery, and maintaining a safe altitude. The simulated trajectories capture uncertainties that emerge from both the planner, task, and modeled environment. We showed that we can analyze the trajectories to quantify the system’s competency using Factorize Machine Self-Confidence GOA. In particular, we evaluated an autonomous UAS operating in both calm and adverse wind conditions and showed that GOA can capture both performance differences as well as uncertainties across three mission outcomes of interest to potential human supervisors. Additionally, we identified several challenges and directions for future work in translating competency self-assessments to live aerial platforms with humans in the loop. The communication of competency self-assessments to human partners should lead to a safer deployment of the autonomous system and additionally provide UAS operators with the ability to make informed decisions based on the platform’s GOA, thus improving the ability to calibrate user trust and understand the system’s capabilities.
Data availability statement
The raw data supporting the conclusions of this article will be made available by the authors, without undue reservation.
Author contributions
NC: conceptualization, data curation, formal analysis, investigation, methodology, software, validation, visualization, writing–original draft, and writing–review and editing. AA: conceptualization, formal analysis, investigation, methodology, software, validation, writing–original draft, and writing–review and editing. JM: conceptualization, formal analysis, investigation, methodology, software, validation, writing–original draft, and writing–review and editing. TS: conceptualization, formal analysis, investigation, methodology, software, validation, writing–original draft, and writing–review and editing. CH: conceptualization, formal analysis, investigation, methodology, software, validation, writing–original draft, and writing–review and editing. MD’A: conceptualization, formal analysis, investigation, methodology, software, validation, writing–original draft, and writing–review and editing. MH: conceptualization, formal analysis, investigation, methodology, software, validation, writing–original draft, and writing–review and editing. CR: conceptualization, formal analysis, investigation, methodology, software, validation, writing–original draft, and writing–review and editing. EF: conceptualization, funding acquisition, investigation, supervision, writing–original draft, and writing–review and editing. RR: conceptualization, formal analysis, funding acquisition, investigation, methodology, software, supervision, validation, writing–original draft, and writing–review and editing. NA: conceptualization, funding acquisition, investigation, methodology, supervision, writing–original draft, and writing–review and editing.
Funding
The authors declare that financial support was received for the research, authorship, and/or publication of this article. This work was partially supported by the Defense Advanced Research Projects Agency (DARPA) under Contract No. HR001120C0032.
Acknowledgments
This manuscript represents an extension to our previously presented work (Conlon et al., 2022a).
Conflict of interest
MD’A, MH, CR, and RR were employed by the company Draper.
The remaining authors declare that the research was conducted in the absence of any commercial or financial relationships that could be construed as a potential conflict of interest.
Publisher’s note
All claims expressed in this article are solely those of the authors and do not necessarily represent those of their affiliated organizations, or those of the publisher, the editors and the reviewers. Any product that may be evaluated in this article, or claim that may be made by its manufacturer, is not guaranteed or endorsed by the publisher.
Author disclaimer
Any opinions, findings and conclusions or recommendations expressed in this material are those of the author(s) and do not necessarily reflect the views of DARPA.
Footnotes
1https://asc.army.mil/web/portfolio-item/aviation_raven-suas/
References
Abedin, S. F., Munir, M. S., Tran, N. H., Han, Z., and Hong, C. S. (2020). Data freshness and energy-efficient uav navigation optimization: a deep reinforcement learning approach. IEEE Trans. Intelligent Transp. Syst. 22, 5994–6006. doi:10.1109/tits.2020.3039617
Aitken, M. (2016). Assured human-autonomy interaction through machine self-confidence. Boulder: University of Colorado. Master’s thesis.
Bengio, Y., Simard, P., and Frasconi, P. (1994). Learning long-term dependencies with gradient descent is difficult. IEEE Trans. Neural Netw. 5, 157–166. doi:10.1109/72.279181
Chua, C. N. (2012). Integration of multiple UAVs for collaborative ISR missions in an urban environment. Monterey, California, USA: Naval Postgraduate School. Master’s thesis.
Conlon, N., Acharya, A., McGinley, J., Slack, T., Hirst, C. A., Hebert, M., et al. (2022a). “Generalizing competency self-assessment for autonomous vehicles using deep reinforcement learning,” in AIAA SciTech forum (AIAA).
Conlon, N., Ahmed, N., and Szafir, D. (2024). Event-triggered robot self-assessment to aid in autonomy adjustment. Front. Robotics AI 10, 1294533. doi:10.3389/frobt.2023.1294533
Conlon, N., Szafir, D., and Ahmed, N. (2022b). ““I’m confident this will end poorly”: robot proficiency self-assessment in human-robot teaming,” in 2022 IEEE/RSJ International Conference on Intelligent Robots and Systems (IROS), 2127–2134. doi:10.1109/IROS47612.2022.9981653
Cook, K. L. B. (2007). “The silent force multiplier: the history and role of uavs in warfare,” in 2007 IEEE aerospace conference, 1–7. doi:10.1109/AERO.2007.352737
Devitt, S. (2018). Trustworthiness of autonomous systems. Found. Trust. Aut. Stud. Syst. Decis. Control 117, 161–184. doi:10.1007/978-3-319-64816-3_9
Dzindolet, M. T., Peterson, S. A., Pomranky, R. A., Pierce, L. G., and Beck, H. P. (2003). The role of trust in automation reliance. Int. J. human-computer Stud. 58, 697–718. doi:10.1016/s1071-5819(03)00038-7
Ebert, F., Finn, C., Dasari, S., Xie, A., Lee, A., and Levine, S. (2018). Visual foresight: model-based deep reinforcement learning for vision-based robotic control. arXiv preprint arXiv:1812.00568
Erdelj, M., Król, M., and Natalizio, E. (2017). Wireless sensor networks and multi-uav systems for natural disaster management. Comput. Netw. 124, 72–86. doi:10.1016/j.comnet.2017.05.021
Ezequiel, C. A. F., Cua, M., Libatique, N. C., Tangonan, G. L., Alampay, R., Labuguen, R. T., et al. (2014). “Uav aerial imaging applications for post-disaster assessment, environmental management and infrastructure development,” in 2014 International Conference on Unmanned Aircraft Systems (ICUAS), 274–283. doi:10.1109/ICUAS.2014.6842266
Furrer, F., Burri, M., Achtelik, M., and Siegwart, R. (2016). “RotorS—a modular gazebo MAV simulator framework,” in Robot operating system (ROS): the complete reference (Springer International Publishing), 1, 595–625. chap. RotorS. doi:10.1007/978-3-319-26054-9_23
Goecks, V. G., and Valasek, J. (2019). Deep reinforcement learning on intelligent motion video guidance for unmanned air system ground target tracking. AIAA Scitech 2019 Forum, 0137. doi:10.2514/6.2019-0137
Hutchins, A. R., Cummings, M. L., Draper, M., and Hughes, T. (2015). Representing autonomous systems’ self-confidence through competency boundaries. Proc. Hum. Factors Ergonomics Soc. Annu. Meet. 59, 279–283. doi:10.1177/1541931215591057
Israelsen, B. (2019). Algorithmic assurances and self-assessment of competency boundaries in autonomous systems. Boulder: University of Colorado at Boulder. Ph.D. thesis.
Israelsen, B., Ahmed, N., Frew, E., Lawrence, D., and Argrow, B. (2019). “Machine self-confidence in autonomous systems via meta-analysis of decision processes,” in International conference on applied human factors and ergonomics (Springer), 213–223.
Israelsen, B. W., and Ahmed, N. R. (2019). Dave I can assure you that it’s going to be all right a definition, case for, and survey of algorithmic assurances in human-autonomy trust relationships. ACM Comput. Surv. (CSUR) 51, 1–37. doi:10.1145/3267338
Jagannath, J., Jagannath, A., Furman, S., and Gwin, T. (2021). Deep learning and reinforcement learning for autonomous unmanned aerial systems: roadmap for theory to deployment. Deep Learn. Unmanned Syst., 25–82. doi:10.1007/978-3-030-77939-9_2
Julian, K. D., and Kochenderfer, M. J. (2019). Distributed wildfire surveillance with autonomous aircraft using deep reinforcement learning. J. Guid. Control, Dyn. 42, 1768–1778. doi:10.2514/1.G004106
Koenig, N., and Howard, A. (2004). “Design and use paradigms for gazebo, an open-source multi-robot simulator,” in 2004 IEEE/RSJ International Conference on Intelligent Robots and Systems (IROS) (IEEE), (Cat. No.04CH37566.
McGinley, J. (2022). Approaches for the computation of generalized self-confidence statements for autonomous vehicles. Boulder: University of Colorado. Master’s thesis.
McGuire, S., Furlong, P. M., Fong, T., Heckman, C., Szafir, D., Julier, S. J., et al. (2019). Everybody needs somebody sometimes: Validation of adaptive recovery in robotic space operations. IEEE Robotics Automation Lett. 4, 1216–1223. doi:10.1109/lra.2019.2894381
McGuire, S., Furlong, P. M., Heckman, C., Julier, S., Szafir, D., and Ahmed, N. (2018). Failure is not an option: policy learning for adaptive recovery in space operations. IEEE Robotics Automation Lett. 3, 1639–1646. doi:10.1109/lra.2018.2801468
Mellinkoff, B., Ahmed, N. R., and Burns, J. (2020). “Towards self-confidence-based adaptive learning for lunar exploration,” in AIAA scitech 2020 forum. doi:10.2514/6.2020-1378
Moerland, T. M., Broekens, J., and Jonker, C. M. (2020). Model-based reinforcement learning: a survey. arXiv preprint arXiv:2006.16712
Mosali, N. A., Shamsudin, S. S., Alfandi, O., Omar, R., and Al-Fadhali, N. (2022). Twin delayed deep deterministic policy gradient-based target tracking for unmanned aerial vehicle with achievement rewarding and multistage training. IEEE Access 10, 23545–23559. doi:10.1109/access.2022.3154388
Nagabandi, A., Konoglie, K., Levine, S., and Kumar, V. (2019). Deep dynamics models for learning dexterous manipulation. arXiv e-prints. arXiv:1909.11652
OASD (2018). “Unmanned systems integrated roadmap 2017-2042,” in Office of the assistant secretary of defense for acquisition Washington United States.
Pascanu, R., Mikolov, T., and Bengio, Y. (2013). “On the difficulty of training recurrent neural networks,” in Proceedings of the 30th International Conference on International Conference on Machine Learning (JMLR.org) (Atlanta, GA, USA: ICML’), III–1310–III–1318.
Pomranky, R. A. (2006). “Human robotics interaction army technology objective raven small unmanned aerial vehicle task analysis and modeling,”. Adelphi, MD, USA: US Army Research Laboratory.
Ray, H. M., Laouar, Z., Sunberg, Z., and Ahmed, N. (2024). “Human-centered autonomy for autonomous suas target searching,” in 2024 IEEE/RAS international conference on robotics and automation (ICRA 2024).
Shahrdar, S., Menezes, L., and Nojoumian, M. (2019). “A survey on trust in autonomous systems,” in Intelligent Computing: Proceedings of the 2018 Computing Conference (Springer).
Sudhakar, S., Vijayakumar, V., Sathiya Kumar, C., Priya, V., Ravi, L., and Subramaniyaswamy, V. (2020). Unmanned aerial vehicle (uav) based forest fire detection and monitoring for reducing false alarms in forest-fires. Comput. Commun. 149, 1–16. doi:10.1016/j.comcom.2019.10.007
Sutton, R. S., and Barto, A. G. (2018). Reinforcement learning: an introduction. second edn. The MIT Press.
Sweet, N., Ahmed, N. R., Kuter, U., and Miller, C. (2016). “Towards self-confidence in autonomous systems,” in AIAA infotech @ aerospace. doi:10.2514/6.2016-1651
van Wijk, D., Eves, K. J., and Valasek, J. (2023). “Deep reinforcement learning controller for autonomous tracking of evasive ground target,” in AIAA SciTech 2023 forum, 0128.
Wang, T., Bao, X., Clavera, I., Hoang, J., Wen, Y., Langlois, E., et al. (2019). Benchmarking model-based reinforcement learning. arXiv preprint arXiv:1907.02057
Keywords: machine self-confidence, human-autonomy teaming, intelligent aerospace systems, trustworthy AI, uncrewed aerial vehicles
Citation: Conlon N, Acharya A, McGinley J, Slack T, Hirst CA, D’Alonzo M, Hebert MR, Reale C, Frew EW, Russell R and Ahmed NR (2025) Competency self-assessment for a learning-based autonomous aircraft system. Front. Aerosp. Eng. 4:1454832. doi: 10.3389/fpace.2025.1454832
Received: 25 June 2024; Accepted: 02 January 2025;
Published: 14 February 2025.
Edited by:
Zhaodan Kong, University of California, Davis, United StatesReviewed by:
John Page, University of New South Wales, AustraliaKrishna Kalyanam, National Aeronautics and Space Administration, United States
Copyright © 2025 Conlon, Acharya, McGinley, Slack, Hirst, D’Alonzo, Hebert, Reale, Frew, Russell and Ahmed. This is an open-access article distributed under the terms of the Creative Commons Attribution License (CC BY). The use, distribution or reproduction in other forums is permitted, provided the original author(s) and the copyright owner(s) are credited and that the original publication in this journal is cited, in accordance with accepted academic practice. No use, distribution or reproduction is permitted which does not comply with these terms.
*Correspondence: Nicholas Conlon, bmljaG9sYXMuY29ubG9uQGNvbG9yYWRvLmVkdQ==