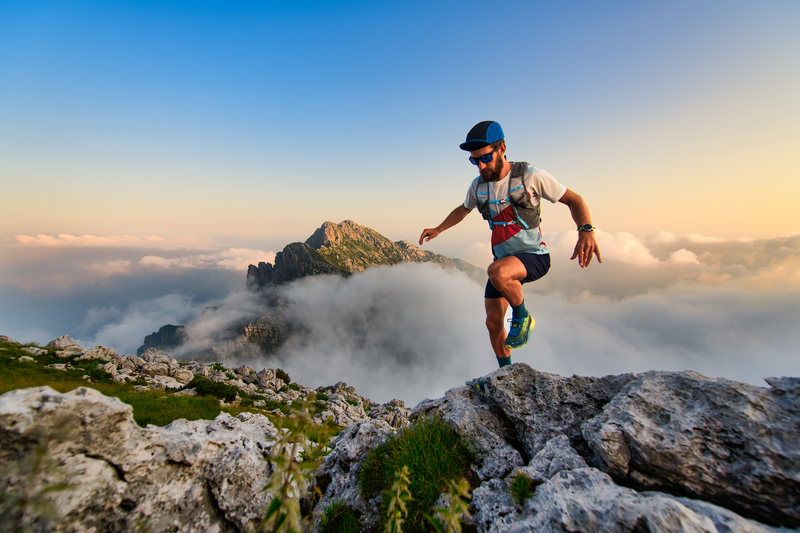
94% of researchers rate our articles as excellent or good
Learn more about the work of our research integrity team to safeguard the quality of each article we publish.
Find out more
SPECIALTY GRAND CHALLENGE article
Front. Aerosp. Eng. , 12 September 2023
Sec. Intelligent Aerospace Systems
Volume 2 - 2023 | https://doi.org/10.3389/fpace.2023.1281522
We are in the midst of the fourth industrial revolution, and artificial intelligence (AI) is one of its driving technologies. AI refers to a system that can emulate human-level intelligence. During the past century, ever since the advent of aviation, humans have played a central role in military and commercial applications. It is anticipated that advances in AI will bring about an augmentation of human performance by advancing automation coupled with enhanced functionality, efficiency, safety, and decision making. In August 2020, the DARPA (Defense Advanced Research Projects Agency) ACE program’s AlphaDogfight Trials demonstrated an AI defeating an experienced F-16 flight pilot in a simulator. In the civilian aviation application arena, Morgan Stanley estimates that advanced air mobility (AAM), which is very dependent on assured autonomy, will have an addressable market of $4.4 tn by 2040 and $18.9 tn by 2050.
The application areas for intelligent aerospace systems are ever growing and include the following. 1) Autonomous Aircraft for Advanced Air Mobility, which operate without human intervention for duties such as task planning, obstacle avoidance, data collection, and decision making. 2) Flight control and navigation systems driven by AI which enable precise navigation while analyzing real-time data for optimal path planning, energy efficient flight paths, and augmented safety. 3) Maintenance and diagnostics systems that use health monitoring algorithms to predict potential issues, thereby circumventing operational problems by scheduling maintenance when needed and reducing downtime. 4) Mission planning and execution assisted by AI, which can offer optimized trajectories and make real-time changes to adapt to changing conditions—also referred to as “unknown unknowns”. 5) Air traffic management enabled by AI which can manage an increasing number of flights and assure safe and efficient aircraft routing.
Although these AI-driven developing capabilities have drawn much attention, a new can of worms has also been opened. There is a flow of important questions oozing out such as: Is AI reliable, safe, trustworthy, ethical, responsible, verifiable, robust, dependable, etc.?. With increased interest and the potential impact of AI on the aeronautical industry in terms of new capabilities and economic benefits, there is a clear and immediate need for research. The grand challenges that advance autonomy, described in part here, offer a unique set of opportunities for researchers across academia, federal agencies, and industry. The Intelligent Aerospace Systems section of Frontiers in Aerospace Engineering looks forward to supporting and disseminating research that addresses the current and future challenges on the path to realizing this immense potential.
The aerospace industry has made extensive use of automation in systems and sub-systems. Automation uses technology to perform tasks without human intervention and may be based on linguistic rules or more complex control actions. Automation works well for repetitive tasks or sequencing complex tasks by breaking them down into simpler sub-tasks. One shortcoming of automated processes is limited adaptability—their inability to learn, adapt, and make decisions beyond the scope of the programmed instructions. On the other hand, AI emulates human reasoning by enabling machines to perform tasks that require human intelligence. The following features are often associated with AI: 1) learning and adaptation; 2) complex decision making; 3) cognitive ability; 4) autonomy. AI can enhance automation by incorporating optimal decision-making capabilities. One of the challenges of integrating AI into aerospace systems is its lack of trustworthiness. We have developed a complex system of establishing trust in human actions within the aerospace industry. If AI were to replace some of that human action, we need to trust it to perform its tasks effectively and reliably while assuring safety. The field of trustworthy AI is in its infancy, with many gaps between where we desire to be versus where we currently are. As a result of these gaps, there is well-founded fear that AI systems may cause harm to their users and to society (Kaur et al., 2022). Trustworthiness may comprise several requirements, such as fairness, explicability, certifiability, accountability, responsibility, verifiability, reliability, and acceptance. Kaur et al. (2022) analyze the aforementioned requirements through a literature survey and provide insights into approaches that mitigate AI risks and increase trust, as well as strategies for validating and verifying (V&V) these systems. Chatila R. et al. (2021) emphasize the need for the following attributes for trustworthy AI as vital to building operational governance frameworks and aligning their application with core human values and rights: security, robustness, transparency, verifiability, explicability, and safety. Moreover, Gartner provides an estimate that 30% of products based on AI will require the use of a trustworthy AI framework by 2025 (Burke et al., 2019), and, importantly, that as many as 86% of users will remain loyal to (and trust) companies that use ethical AI principles (Edelman, 2019). The report produced by the European Commission titled ‘Ethics guidelines for trustworthy AI presents an interesting and useful approach to evaluate the responsible development of AI systems while encouraging international collaboration on AI solutions that are beneficial for humanity (Floridi, 2019).
Li et al. (2023) provide a set of principles and a useful set of practices for implementing trustworthy AI. Baron et al. (2018) discuss a framework for trustworthiness requirements and models for aviation and aerospace systems.
The need for trustworthiness in AI has led to the requirement for eXplainable AI (referred to as XAI), which provides transparent and understandable explanations for decisions and predictions (Adadi and Berrada, 2018). A majority of AI models such as deep learning are anticipated as a black box (Chennam et al., 2023). Furthermore, it is believed that XAI will assist in bridging the trust gap and enable acceptance by human subject matter experts. DARPA (Gunning, 2017) launched the XAI program, which aims to make AI systems explainable and trustworthy. This initiative has been well internalized by researchers, thus creating a major shift in AI research, especially for safety-critical applications such as aerospace, defense, and medical. Sutthithatip et al. (2022) explore the application of XAI for safety-critical aerospace by surveying various techniques, such as model agnostic, fuzzy logic, white-box AI, black-box AI, and knowledge graphs. In addition, the XAI requirements are presented for safety-critical systems from the point of view of developers, guarantors, and interpreters. Degas et al. (2022) surveyed the application of XAI within the aviation/ATM domain and provided a conceptual framework named the DPP (descriptive, predictive, and prescriptive) model, along with a potential scenario in 2030. Youness and Aalah (2023) demonstrated the interpretability of deep learning prognosis using XAI for remaining useful life (RUL) prediction in a simulated turbofan engine.
As we transition from automation to AI driven, safety-critical, autonomous aerospace systems, assured autonomy (AA) is a quintessential concept that concerns the need for high levels of confidence and reliability. In addition to aerospace, AA is also relevant in industries such as defense, transportation, healthcare, and industrial automation, where the cost of failure can be catastrophic. AA incorporates AI/ML, sensor fusion, control systems, and a robust framework of V&V, as well as testing methodologies, coupled with autonomy to guarantee that the system will perform safely and effectively and at an acceptable risk level. Typically, AA includes the following components in addition to autonomy and assurance: safety; reliability, risk management, ethics, and accountability. Collins (2015) reports on NASA’s vision for safe, autonomous systems operations and the central role that AA plays in realizing that vision. Moreover, it is projected that increasing autonomy, while assuring safety and reliability, will revolutionize the business models that drive aviation. Topcu et al. (2020) report on the importance of AA to the future of autonomous systems and the corresponding revolution; they list the negative outcomes that may result from a lack of assurance. Clarke et al. (2020) discuss the critical steps needed to achieve AA for AAM that include learning-enabled perception, human–machine teaming, and verification of nondeterministic systems. Bartlett et al. (2023) provide specific autonomy functions for AA and corresponding design assurance strategies. Fidi (2023) advocates the use of a digital representative—a “digital twin” of the vehicle to further advance state-of-the-art AA research—and that using digital twins will enable new applications and better planning.
Human–AI teaming (HAT) or human–AI collaboration represents the integration of humans and AI systems to cooperatively perform tasks and achieve goals. HAT provides synergy between humans and AI, creating a relationship that utilizes the unique strengths of each entity. Collaboration may occur between one or more humans on one side and one or more different AI systems on the other. The main idea is that the human partner overcomes the typical shortcomings of AI such as brittleness, perceptual limitations, hidden biases, and lack of ability to predict future events (Endsley et al., 2022a). Furthermore, in the short- and mid-terms, due to these inadequacies, AI requires careful human supervision and management. The National Academy of Sciences, Engineering and Medicine report (Endsley et al., 2022a) presents the current capability and the research gaps concerning the design and implementation of coupled human-AI operations, with the aim of augmenting overall performance beyond that of either entity. Endsley (2023) suggests methods for supporting team situational awareness (SA) within HAT and provides a framework for understanding the types of information that need to be shared within HAT. Moreover, AI transparency and explicability play an important role in supporting SA, and mental models in HAT and the SA-Oriented Design (SAOD) process are detailed.
Textor et al. (2022) explore the aspect of AI’s conformity with ethical norms and the impact that may have on human trust, and that typical human responses to ethical violations could not repair the loss of trust. Ezenyilimba et al. (2023) utilize the case of search-and-rescue to investigate the relationship between robot explicability and transparency on SA and trust, showing that trust improved when detailed explanations were provided, as opposed to transparency alone. Dorton and Harper (2022) provide a naturalistic exploration of trust and AI from the perspective of intelligence analysts. They identify that the performance of the system coupled with its explicability and perceived utility were important elements in enabling them to achieve their mission (Endsley et al., 2022b).
The field of intelligent aerospace systems is growing in impact, bringing about impressive capabilities and new use cases. As highlighted here, there are several important scientific and technological challenges that will require focused research efforts for decades to come. The Intelligent Aerospace Systems section within Frontiers in Aerospace Engineering seeks to advance research and development in AI-enabled areas such as advanced air mobility, flight control and navigation, maintenance and diagnostics, mission planning and execution, and air traffic management by publishing high-quality contributions in these areas that address challenges at the forefront of aerospace engineering.
KC: writing–original draft.
The author declares that the research was conducted in the absence of any commercial or financial relationships that could be construed as a potential conflict of interest.
The author declared that they were an editorial board member of Frontiers at the time of submission. This had no impact on the peer review process and the final decision.
All claims expressed in this article are solely those of the authors and do not necessarily represent those of their affiliated organizations, or those of the publisher, the editors, and the reviewers. Any product that may be evaluated in this article, or claim that may be made by its manufacturer, is not guaranteed or endorsed by the publisher.
Adadi, A., and Berrada, M. (2018). “Peeking inside the black-box: A survey on explainable artificial intelligence (XAI)”, IEEE Access 6, 52138–52160. doi:10.1109/access.2018.2870052
Baron, A., Babiceanu, R. F., and Seker, R. (October 2018). "Trustworthiness requirements and models for aviation and aerospace systems," Proc. 2018 Integr. Commun. Navigation, Surveillance Conf. (ICNS), Herndon, VA, USA
Bartlett, P., Chamberlain, L., Singh, S., and Coblenz, L. (2023). A near-term path to assured aerial autonomy. SAE Int. J. Aerosp. 16 (3). doi:10.4271/01-16-03-0020
Burke, B., Cearley, D., Jones, N., Smith, D., Chandrasekaran, A., Lu, C. K., et al. (2019). Gartner top 10 strategic technology trends for 2020-smarter with gartner. Retrieved from https://www.gartner.com/smarterwithgartner/gartner-top-10-strategic-technology-trends-for-2020/.
Chatila, R., (2021). “Reflections on artificial intelligence for humanity,” in Lecture notes in computer science. Editors B. Braunschweig, and M. Ghallab (Cham, Germany: Springer). doi:10.1007/978-3-030-69128-8_2
Chennam, K. K., Mudrakola, S., Maheswari, V. U., Aluvalu, R., and Rao, K. G. (2023). “Black box models for eXplainable artificial intelligence,” in Explainable AI: Foundations, methodologies and applications. Intelligent systems reference library. Editors M. Mehta, V. Palade, and I. Chatterjee (Cham, Germany: Springer). doi:10.1007/978-3-031-12807-3_1
Clarke, J-P., and Tomlin, C. J. (2020). Some steps toward autonomy in aeronautics. Retrieved from https://www.nae.edu/234438/Some-Steps-toward-Autonomy-in-Aeronautics.
Collins, C. (2015). Nasa: assured autonomy for aviation transformation: NASA's aerospace research mission directorate on NACA's 100th anniversary. Retrieved from: https://www.defensemedianetwork.com/stories/nasa-assured-autonomy-for-aviation-transformation/4/.
Degas, A., Islam, M. R., Hurter, C., Barua, S., Rahman, H., Poudel, M., et al. (2022). A survey on artificial intelligence (AI) and eXplainable AI in air traffic management: current trends and development with future research trajectory. Appl. Sci. 12 (3), 1295. doi:10.3390/app12031295
Dorton, S., and Harper, S. (2022). A naturalistic investigation of trust, AI, and intelligence work. J. Cognitive Eng. Decis. Mak. 16 (4), 222–236. doi:10.1177/15553434221103718
Edelman, (2019). Edelman trust barometer. Retrieved from https://www.edelman.com/sites/g/files/aatuss191/files/2019-02/2019_Edelman_Trust_Barometer_Global_Report.pdf.
Endsley, M. R., Cooke, N., McNeese, N., Bisantz, A., Militello, L., and Roth, E. (2022b). Special issue on human-AI teaming and special issue on AI in healthcare. J. Cognitive Eng. Decis. Mak. 16 (4), 179–181. doi:10.1177/15553434221133288
Endsley, M. R., (2022a). “National academies of Sciences, engineering, and medicine,” in Human-AI teaming: State-of-the-Art and research needs (Washington, DC: The National Academies Press). doi:10.17226/26355
Endsley, M. R. (2023). Supporting human-AI teams: transparency, explainability, and situation awareness. Comput. Hum. Behav. 140, 107574. doi:10.1016/j.chb.2022.107574
Ezenyilimba, A., Wong, M., Hehr, A., Demir, M., Wolff, A., Chiou, E., et al. (2023). Impact of transparency and explanations on trust and situation awareness in human–robot teams. J. Cognitive Eng. Decis. Mak. 17 (1), 75–93. doi:10.1177/15553434221136358
Fidi, C. (2023). The future of safe and secure aerospace systems. Retrieved from https://www.eetimes.eu/the-future-of-safe-and-secure-aerospace-systems/.
Floridi, L. (2019). Establishing the rules for building trustworthy AI. Nat. Mach. Intell. 1, 261–262. doi:10.1038/s42256-019-0055-y
Gunning, D. (2017). Explainable artificial intelligence (XAI). Defense Advanced Research Projects Agency.
Kaur, D., Uslu, S., Rittichier, K. J., and Durresi, A. (2022). “Trustworthy artificial intelligence: A review”, ACM Comput. Surv., 55, 2, 1-38. doi:10.1145/3491209
Li, B., Qi, P., Liu, B., Di, S., Liu, J., Pei, J., et al. (2023). “Trustworthy AI: from principles to practices”, ACM Comput. Surv., 55, 9, 1-46. doi:10.1145/3555803
Sutthithatip, S., Perinpanayagam, S., and Aslam, S., (October 2022). "The changing face of HR professionals' expectations amidst COVID-19: A comparison in between Sri Lanka and foreign context," Proceedings of the 2022 IEEE Aerosp. Conf. (AERO), Big Sky, MT, USA, doi:10.1007/s11135-022-01533-3
Textor, C., Zhang, R., Lopez, J., Schelble, B. G., McNeese, N. J., Freeman, G., et al. (2022). Exploring the relationship between ethics and trust in human–artificial intelligence teaming: A mixed methods approach. J. Cognitive Eng. Decis. Mak. 16 (4), 252–281. doi:10.1177/15553434221113964
Topcu, U., Bliss, N., Cooke, N., Cummings, M., Llorens, A., Shrobe, H., et al. (2020). Assured autonomy: path toward living with autonomous systems we can trust. https://doi.org/10.48550/arXiv.2010.14443.
Keywords: intelligent systems, artificial intelligence, assured autonomy, trustworthy AI, advanced automation, soft computing, human–AI teaming, Industry 4.0
Citation: Cohen K (2023) Grand challenges in intelligent aerospace systems. Front. Aerosp. Eng. 2:1281522. doi: 10.3389/fpace.2023.1281522
Received: 22 August 2023; Accepted: 25 August 2023;
Published: 12 September 2023.
Edited and reviewed by:
Ramesh K. Agarwal, Washington University in St. Louis, United StatesCopyright © 2023 Cohen. This is an open-access article distributed under the terms of the Creative Commons Attribution License (CC BY). The use, distribution or reproduction in other forums is permitted, provided the original author(s) and the copyright owner(s) are credited and that the original publication in this journal is cited, in accordance with accepted academic practice. No use, distribution or reproduction is permitted which does not comply with these terms.
*Correspondence: Kelly Cohen, a2VsbHkuY29oZW5AdWMuZWR1
Disclaimer: All claims expressed in this article are solely those of the authors and do not necessarily represent those of their affiliated organizations, or those of the publisher, the editors and the reviewers. Any product that may be evaluated in this article or claim that may be made by its manufacturer is not guaranteed or endorsed by the publisher.
Research integrity at Frontiers
Learn more about the work of our research integrity team to safeguard the quality of each article we publish.