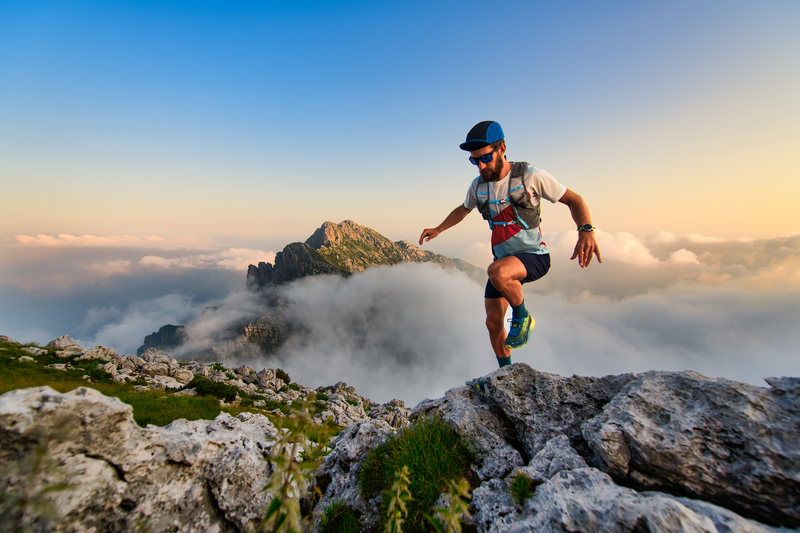
94% of researchers rate our articles as excellent or good
Learn more about the work of our research integrity team to safeguard the quality of each article we publish.
Find out more
ORIGINAL RESEARCH article
Front. Adolesc. Med.
Sec. Addiction in Adolescents
Volume 3 - 2025 | doi: 10.3389/fradm.2025.1549771
This article is part of the Research Topic Cannabis use and neurocognitive functioning in adolescents View all 3 articles
The final, formatted version of the article will be published soon.
You have multiple emails registered with Frontiers:
Please enter your email address:
If you already have an account, please login
You don't have a Frontiers account ? You can register here
Background: Chronic cannabis use (CU) can result in subtle deficits in cognitive performance that may be linked with alterations in underlying neural functioning. However, these network alterations are not well-characterized following monitored abstinence. Here, we evaluate differences in functional brain network activity associated with CU patterns in adolescents/young adults.Methods: Functional connectomes were generated using resting-state fMRI data collected from 83 healthy young adults (44 male) following two weeks of monitored cannabis abstinence. Network topology metrics were calculated for each of the 7 Yeo 2011 intrinsic connectivity networks (ICNs) and on the whole-brain level. Multiple linear regressions were used to evaluate whether CU (regularusers, n=35 vs non-using controls, n=40) was associated with network topology metric differences after controlling for past-year alcohol use, age, sex, and cotinine levels; moderation by sex was also investigated. Regressions were run within CU group to test for associations between cannabis use patterns (lifetime CU, age of CU initiation, and past-year CU) and network topology. Finally, a network-based statistic (NBS) approach was used to search for connectome subcomponents associated with CU group, CU*sex, and patterns of CU.Results: No significant association between CU groups and ICN topology was observed. Sex moderation was observed; within male cannabis users, higher past-year CU was associated with significantly higher frontoparietal and ventral attention network (VAN) efficiency. Within female cannabis users, higher past-year CU was associated with significantly lower Default Mode Network assortativity. Within individuals who initiated CU before the age of 17, males had lower assortativity in the VAN and Somatomotor network. NBS analyses indicated that connectivity strength within a primarily right-lateralized subnetwork distributed throughout the connectome was significantly and reliably associated with past-year CU).The present findings suggest that subtle differences in resting-state network topology associated with CU may persist after an extended period of abstinence in young adults, particularly males, especially those with heavier past-year use and those who initiated CU earlier in life. While further replication is required in larger samples, these findings suggest potential neuroimaging correlates underlying long-term changes in brain network topology associated with CU.
Keywords: Cannabis, networks, fMRI, Young Adult, Abstinence
Received: 21 Dec 2024; Accepted: 11 Mar 2025.
Copyright: © 2025 Baacke, Sullivan, Shankula and Lisdahl. This is an open-access article distributed under the terms of the Creative Commons Attribution License (CC BY). The use, distribution or reproduction in other forums is permitted, provided the original author(s) or licensor are credited and that the original publication in this journal is cited, in accordance with accepted academic practice. No use, distribution or reproduction is permitted which does not comply with these terms.
* Correspondence:
Kyle A. Baacke, University of Wisconsin–Milwaukee, Milwaukee, United States
Krista M. Lisdahl, University of Wisconsin–Milwaukee, Milwaukee, United States
Disclaimer: All claims expressed in this article are solely those of the authors and do not necessarily represent those of their affiliated organizations, or those of the publisher, the editors and the reviewers. Any product that may be evaluated in this article or claim that may be made by its manufacturer is not guaranteed or endorsed by the publisher.
Research integrity at Frontiers
Learn more about the work of our research integrity team to safeguard the quality of each article we publish.