- 1Georgia Cancer Center, Augusta University, Augusta, GA, United States
- 2Department of Pediatrics, Medical College of Georgia, Augusta University, Augusta, GA, United States
- 3School of Computing, University of Georgia, Athens, GA, United States
- 4Institute of Public and Preventive Health, Augusta University, Augusta, GA, United States
Objective: To analyze the impact of social determinants of health (SDH) on cancer outcomes of children and adolescents and young adults (AYA) treated for cancer.
Study design: The protocol for this study was registered at PROSPERO (CRD402022346854). A search strategy was implemented across six databases over the last two decades. The focus narrowed to 31 studies conducted in the United States, involving patients between the ages of 15 and 39, assessing survival outcomes based on SDH factors. The Joanna Briggs Institute (JBI) Critical Appraisal Checklist for Analytical Cross-Sectional Studies was employed for risk of bias assessment.
Results: The most extensively examined SDH factors were neighborhood socioeconomic status (nSES) and health insurance status. Other variables investigated were location of care (6/31), poverty level (5/31), education level (3/31), marital status (4/31), median income (3/31), travel distance to medical facility (3/31), language isolation (2/31), and unemployment (1/31). The primary outcome evaluated was overall survival (OS) and cancer-specific survival (CSS). Meta-analyses focusing on hematological malignancies revealed statistically significant associations, such as lowest nSES correlating with worse OS [hazard ratio (HR):1.46, 95%-CI:1.29–1.66] and CSS (HR:1.43, 95%-CI:1.20–1.72), Medicaid/public insurance linked to worse OS (HR: 1.21, 95%-CI:1.16–1.26), and no insurance associated with worse OS (HR:1.35, 95%-CI:1.17–1.55).
Conclusion: The study highlights the fragmented and incomplete nature of research on SDH in cancer treatment in this age group. Health insurance coverage and nSES were the most studied, revealing significant impacts on patient survival. Identifying vulnerable patients through such analyses could inform policy decisions and address existing gaps in SDH research more effectively.
Systematic Review Registration: https://www.crd.york.ac.uk/prospero/, PROSPERO (CRD402022346854).
Introduction
Cancer is among the leading causes of death for children and for adolescents and young adults (AYA) (1, 2). In the United States (US), it is anticipated that 5,280 adolescents in addition to 9,910 children will be diagnosed with cancer by the end of 2023 (1). Among those, 1,040 and 550, respectively, will die from the disease. In the last few decades, remarkable progress in the medical field has resulted in improved prognosis of cancer affecting this specific population (2). However, barriers remain to access advanced diagnostic methods and therapeutic modalities.
Social medicine is traced to the middle of the 20th century, when scientists began to study the root of the disease rather than focusing only on its biological mechanisms (3). In 1948, the World Health Organization (WHO) included “social well-being” in its definition of health for the first time (4). Currently, WHO recognizes the pivotal role of factors other than biological on the diseases and defines the Social Determinants of Health (SDH) as “conditions in which people are born, grow, work, live and age, and the wider set of forces and systems shaping the conditions of daily life” (5). SDH has been grouped into 5 domains: (i) education access and quality, (ii) health care access and quality, (iii) economic stability, (iv) neighborhood and built environment, and (v) social and community according to the Healthy People 2030 (6, 7). Over the past few decades, the population's lifestyle has dramatically changed with the increase in urbanization and industrialization (8), leading to disparities across all levels of quality of life.
It is a consensus that a broad array of SDH plays a significant role in determining survival and treatment-related outcomes in pediatric and AYA patients with cancer. However, the precise effect of each SDH domain and the connection between these domains has to be defined (9). Several studies have analyzed the effects of SDH on children and AYA diagnosed with cancer, but the number of studies that were able to utilize SDH measures at individual-level and tailored for medical research is still scarce. In fact, most studies with higher number of patients rely on public registries such as SEER and NCDB. Although such databases represented a revolution in terms of representation of the US population's clinical features, they were not initially designed to address SDH. In this context, recent initiatives by the National Health Institute's National Institute on Minority Health and Health Disparities (NIMHD) have been focused on building consensus measures on SDH (10) and the hope is that the prospective studies will benefit from this tool.
Therefore, we designed the present study to identify what SDH have been studied in the US, how they were evaluated, and what impact they have on the survival of children and AYA with cancer. Our goal was to provide a resource to inform policy decisions and identify the gaps in SDH research so they can be addressed.
Methods
Eligibility criteria, information sources, and search strategy
This systematic review and meta-analysis were registered at the Prospective Register of Systematic Reviews (PROSPERO) under the registration number CRD42022346854. This study is part of a series of systematic reviews including all types of cancer and followed the same protocol (11–13). In this particular study, we covered tumors arising exclusively in children and AYA. We conducted a thorough search encompassing manuscripts published from January 2001 to September 2022, utilizing six databases, namely PubMed, Scopus, Web of Science, Embase, Cochrane, and Google Scholar (grey literature). Patient, intervention, comparison, outcome, and study design (PICOS) strategy was employed as follows: Population- Patients diagnosed with any type of cancer (specifically for this study, the population was restricted to children and AYA within the age range of 0–39 years) treated for cancer in the United States; Intervention- Any SDH according to the five major domains by the Healthy People 2030 (6, 7): economic stability; neighborhood and built environment; education access and quality; social and community context; health care access and quality; Comparison—None; Outcome measures- Cancer treatment outcomes related to the survival of the patients; Types of studies- Observational studies. We excluded studies in which (i) results were not stratified according to cancer type, (ii) survival-related outcomes were not presented, (iii) outcomes of the patients were assessed exclusively according to their geospatial location (for example, living in a rural or metropolitan setting; comparison between two or more cities, counties or states), (iv) cancer survival was evaluated over time only, not considering any SDH; (v) outcome was analyzed only according to the race and/or ethnicity of the patients, not considering any directly modifiable SDH, and (vi) pre-clinical studies, case reports, reviews of the literature, conference reports, letters, personal opinions, book chapters (vii) studies written in a language other than English.
Study selection
In Phase 1 of the study selection, two authors independently screened all titles and abstracts identified through the search strategy. Studies that did not fulfill the inclusion criteria were eliminated. In Phase 2, the full text of the manuscripts selected in Phase 1 were examined independently by each author. Disagreements in all phases were resolved through discussion and an expert in Pediatric Oncology was consulted when necessary.
Data collection and data items
We collected all information using the qualitative analysis software NVivo (Lumivero, Denver, CO, USA), as previously described (11, 12). A second author cross-checked the data collection. Data about the study characteristics, interventions, results, main findings, and main conclusion was collected. Survival outcomes collected included overall survival (OS) and cancer-specific survival (CSS) hazard ratio (HR) or in months.
Assessment of bias in individual studies
The risk of bias in individual studies was assessed using the Joanna Briggs Institute Critical Appraisal Checklist for analytical cross-sectional studies (11). Two authors independently applied the checklist, and any disagreements were resolved through consultation with a clinical expert. The risk of bias was categorized into three levels: High (0%–50% of items scored as “Yes”), Moderate (50%–70% of items scored as “Yes”), and Low (70%–100% of items scored as “Yes”).
Synthesis methods
SDH were grouped according to the five domains as defined by the World Health Organization: economic stability, neighborhood and built environment, education access and quality, social and community context, and health care access and quality.
The significance of the results was assessed based on p-value (significant if p < 0.05) when it was available, by the authors’ statement or 95%-confidence intervals (95%-CI). The study encompassed quantitative and qualitative analysis. For the qualitative analysis, MS collected the author's name, year of publication, sample size, age group, social determinants studied, survival outcomes effect size (HR and 95%-CI), and p-value if available. Authors crosschecked the retrieved information. Any disagreement was solved by discussion and mutual agreement, and experts were consulted when required.
Statistical analysis
Meta-analysis was performed depending on data availability. Hazard ratios were pooled for SDH and health insurance. Subgroup analysis for hematological malignancies was performed for both variables. Fifteen studies were chosen for the quantitative analysis based on having similar reference and/or measures to assess nSES. The heterogeneity of the analysis was verified using Cochran's Q (x2 test) and I2 test I2 value ranging from 50 to 90% was interpreted as indicative of significant heterogeneity. Meta-analyses were presented using forest plots to determine associations between SDH and either OS or CSS. HR was used to estimate the outcome effect on the meta-analysis. RevMan 5.3 review manager software was used for all statistical analyses.
Results
Study selection
We identified 38,654 manuscripts across the 6 databases queried and 23,335 duplicates were excluded. After screening the title and abstract (phase 1), 44 records were eligible for full-text analysis. Fifteen of these studies were excluded in phase 2 (Supplementary Table S1) (14–26) and 2 studies were added from the reference lists (27, 28). In summary, 31 studies were examined in this systematic review, and 15 manuscripts were eligible for the meta-analysis (Supplementary Figure S1A).
Studies characteristics
Despite the search covering the period from 2001 to 2022, all included studies were published between 2008 and 2022. Leukemias (n = 9, 29%) (29–37), lymphomas (n = 7, 23%) (27, 38–44) and central nervous system (CNS) tumors (n = 5, 16%) (28, 45–48) were the most extensively researched cancer types. Most studies focused on children and adolescents (0–20 years-old, n = 14, 45%) followed by AYA (15–39 years-old) (n = 9, 26%). Sample size ranged between 235 and 80,855 patients and most studies were based on state or national registries. Noteworthy, the California cancer registry (n = 10, 32%) and Surveillance, Epidemiology, and End Results Program (SEER, n = 7, 23%) were the most frequently cited.
Risk of bias of included studies
The risk of bias (ROB) assessment showed that 1 study had high ROB (27), 1 study had moderate ROB (42), and 29 studies had low ROB (Supplementary Figure 1B). All the included studies defined their inclusion criteria while some 18 did not state strategies to deal with any confounder (27, 28, 31, 32, 35, 39, 42, 43, 45, 46, 49–56).
Results of individual studies
Ten SDH variables were investigated across the 31 studies. In terms of the five SDH domains by Healthy People 2023, healthcare access and quality (n = 3, 30%) and economic stability (n = 4, 40%) had the most studied variables, followed by the social and community context (n = 2, 20%), and the education access and quality (n = 1, 10%). Notably, no SDH within neighborhood and built environment domain was examined in the studies included in this review.
The most frequently studied variables were socioeconomic status (SES, n = 19) and health insurance status (n = 18). Other variables included: type of treatment facility (n = 6), poverty (n = 5), median income (n = 4), marital status (n = 4), education (n = 3), travel distance to treatment facility (n = 3), language isolation (n = 2) and unemployment (n = 1). Overall survival was the main outcome in all studies while the secondary outcome CSS was evaluated in 32% (n = 10, 32%).
Health care access and quality
Within the health care access and quality domain, three SDH variables, all at patient level, were explored in the included studies: health insurance (18/31), type of treatment facility (6/31), and travel distance to the treatment facility (3/31, Table 1). Health insurance was captured at the diagnosis and at patient level in all studies. There was a direct impact on children and AYA cancer outcomes in 12 (63%) of the 18 studies that investigated this variable. Being uninsured (29, 31, 34, 36, 40, 42, 57–59) or covered by Medicaid or public insurance (34, 40, 42, 44, 49, 50, 57, 58, 60) was associated with shorter OS (32, 36, 48, 49). Four studies discussed the association between health insurance coverage and CSS. Two studies found a significant association between public insurance or uninsured status and worse CSS (29, 40). Derouen et al. stratified the risk of death depending on the type of cancer. They found a significant association between non-private insurance and worse OS in 11 types of cancer (57).
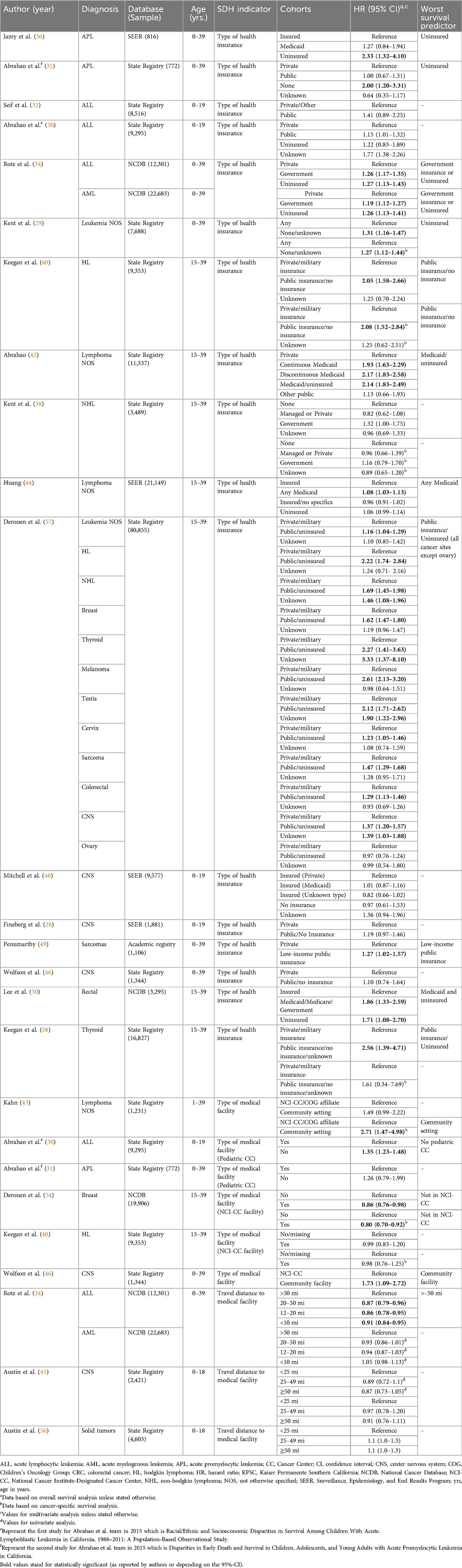
Table 1. Key findings from studies assessing the influence of social determinants of health (SDH) within the “health care access and quality” domain on treatment-related outcomes in pediatric and adolescent and young adult (AYA) patients with cancer.
Meta-analysis was employed to assess the impact of health insurance status on the survival of children and AYA with cancer. Pooling data from six studies examining hematological malignancies, we found that Medicaid/Public insurance (HR = 1.21, 95%-CI:1.16–1.26) and lack of insurance (HR = 1.35, 95%-CI: 1.17–1.55) emerged as significant predictors of poorer OS (p < 0.0001; Figure 1).
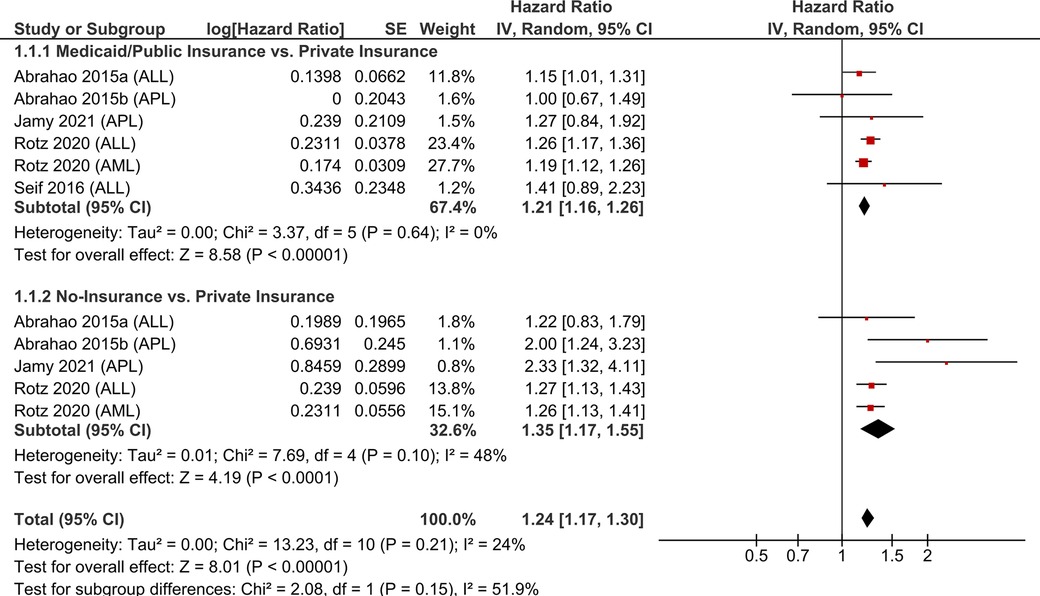
Figure 1. Pooled hazard ratios for overall survival according to the health insurance coverage of children and AYA with hematological malignancies.
Types of health care facility impacted survival in 4 (66%) of the 6 studies that examined this variable. Noteworthy, patients treated in specialized cancer centers demonstrated improved outcomes (31, 43, 46, 54) (Table 1). Only 1 study (Rotz et al) reported that travelling more than 50 miles to the treatment facility adversely affected survival in ALL patients (34). However, no significant association was found in other studies for patients with AML, CNS tumors, or non-CNS solid tumors (34, 45, 56).
Economic stability
Four SDH variables were found in the economic stability domain, including: SES (19/31), poverty (5/31), median income (4/31), and employment (1/31, Table 2). All SDH were reported at community-level. Various approaches were employed to measure the SES, with none based on individual data; instead, county, neighborhood, or zip code-level data were utilized. It is worth mentioning that, although the indices used to assess SES of the population are also based on variables falling in domains other than Economic stability continuum, we described these measurements under this subgroup as most variables pertain to the economic status of the local community. The nSES (Yost index) was the most frequently used approach (11/19, 57.9%) (29–31, 38, 40, 42, 48, 51, 54, 57, 58).

Table 2. Key findings from studies assessing the influence of social determinants of health (SDH) within the “Economic stability” domain on treatment-related outcomes in pediatric and adolescent and young adult (AYA) patients with cancer.
Six studies (32%) revealed no significant association between OS and SES. Four studies (21%) showed a significant association between CSS and nSES, where the lowest nSES correlated with the worst CSS. Derouen et al. stratified according to cancer type and showed a higher HR with Hodgkin, non-Hodgkin lymphoma, and colorectal cancer (27). Considering these findings collectively, nSES had a significant association with OS and CSS. However, the precise effect of nSES varies according to cancer type.
For the meta-analysis, we included eight studies for the examination of OS. Focusing particularly on hematological malignancies, we observed a more pronounced impact on OS in the lowest quartile compared to the highest quartile (HR = 1.46, 95%-CI:1.29–1.66) Notably, there was low heterogeneity across all groups, except for the comparison between the lowest and highest quartiles (Figure 2). The analysis of CSS in hematological malignancies, we found a significant association between nSES and CSS in all subgroup analyses. The strongest association was evident in the comparison between the lowest and highest quartiles for nSES (HR = 1.43, 95%-CI: 1.20–1.72) (Supplementary Figure S2).
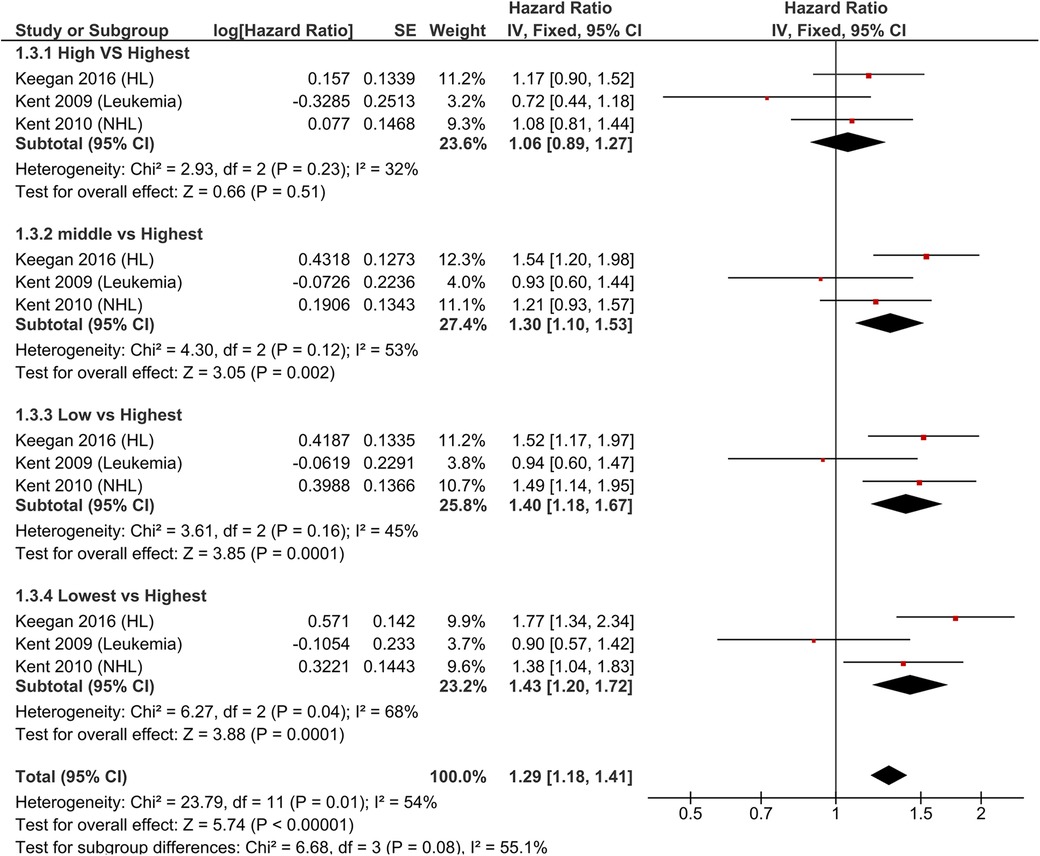
Figure 2. Pooled hazard ratios for overall survival according to nSES in children and AYA with hematological malignancies.
The effects of poverty on OS were examined in five manuscripts (Table 2) (28, 33, 39, 44, 53), and three of these studies (60%) found a significant association between poverty and OS (28, 39, 53). Four studies investigated the impact of household/community median income on hematological malignancies patients (27, 34, 41, 44), and 2 of these manuscripts (50%) showed a significant association between incomes level and OS. Huang et al. employed age-stratification and observed a consistent result for patients aged 15–17 years; however, the effect diminished in older age groups (44). There was no significant association between unemployment and survival (44).
Education access and quality
The association between education level at community level and OS was explored in three of the 31 included studies (Table 3) (28, 41, 44). In addition to OS, Chao et al. investigated CSS as a secondary outcome to assess the correlation with census block-level college graduate percentage as a measure of education level; however, neither outcome showed statistical significance (41). Huang et al. studied lymphoma patients using county-level percentages of people with a high school education or less, stratified into three age groups, but once again, the results were not statistically significant (44).
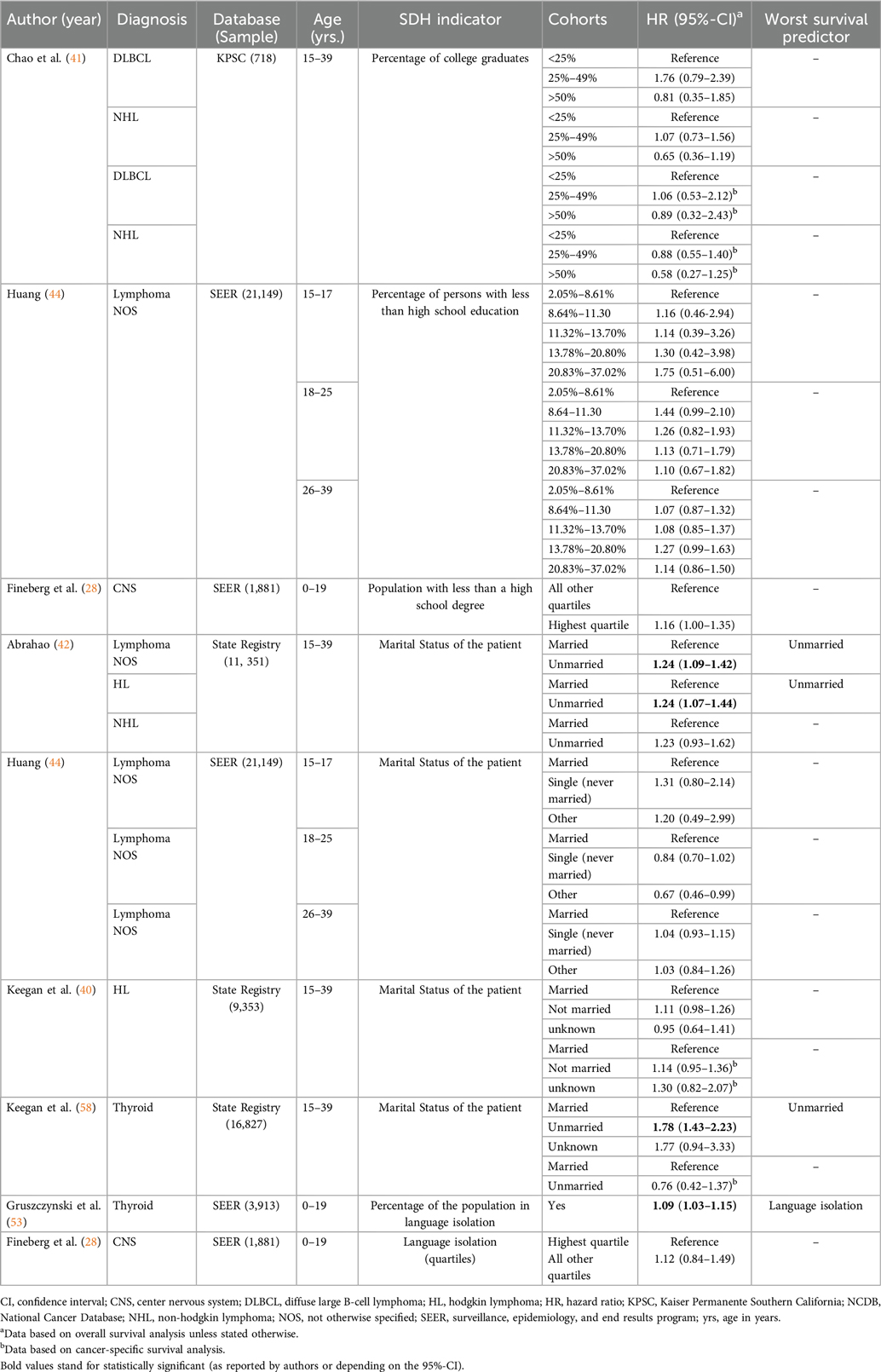
Table 3. Key findings from studies assessing the influence of social determinants of health (SDH) within the “Education access and quality” and “Social and community context” domains on treatment-related outcomes in pediatric and adolescent and young adult (AYA) patients with cancer.
Social and community context
Marital status at individual level and language isolation at community level were the only 2 variables measured in this age group in the manuscripts analyzed. For the marital status, 4 studies (Table 3) used OS as the main outcome (40, 42, 44, 58), and 2 of those used the CSS as a secondary outcome (40, 58). Three reports (75%) assessed lymphoma patients, and one manuscript examined thyroid cancer patients (58). Two of these manuscripts (50%) showed a statistically significant correlation between being married and longer OS (42, 58). For language isolation, one manuscript showed a significant association with OS (53).
Discussion
Our study uncovered several risk factors associated with suboptimal outcomes in pediatric and AYA patients. Notably, individuals with non-private insurance, residing in areas marked by low median income, high poverty rates, limited educational resources, lower SES, and who were unmarried (AYA) tended to exhibit the poorest outcomes. Clinicians must be attentive to these factors while evaluating the patients and adapt their therapeutic strategies and prognostic assessments accordingly. Additionally, it is also essential to acknowledge the importance of the multidisciplinary management of these patients and incorporate social professionals in the workflow during the treatment journey of children and AYAs with cancer.
The primary focus of existing research on the role of SDH in the cancer outcomes of children and AYA has been predominantly centered on variables falling within the economic stability and healthcare access domains, i.e., nSES and health insurance. These variables are widely available in most public registries such as SEER and NCDB and, although important, they were not designed to be assessed in patients with cancer. In addition, we also observed that there is a noticeable gap in the study of other SDH domains, namely, neighborhood and built environment, social and community context, and education access and quality.
Medical insurance had a significant association with OS and CSS in several types of cancer; however, it is important to point out that the insurance status is normally registered at the diagnosis, especially in public registries. Changes may occur during the patient's progress through their cancer journey. For example, uninsured patients who become eligible for Medicaid and receive treatment under its coverage might be captured as uninsured. On the other hand, the type of insurance at the diagnosis provides important information about the healthcare access of the patient (or their parents) before their eligibility for Medicaid. In our analysis, individuals with non-private insurance faced the highest risk of early death, suggesting a potential correlation with delayed presentation and advanced disease state among under-insured patients (24, 61). This is particularly concerning given the higher likelihood of AYA, aged 18–34, not having health insurance (62). This demographic may be at an elevated risk of facing increased economic burdens and limited access to care (63). Moreover, beyond insurance status, additional factors may contribute to adverse outcomes, such as the quality of healthcare facilities providing patient care and the distance patients must travel to access care. These considerations highlight the multifaceted nature of challenges faced by individuals with inadequate insurance coverage.
Meta-analyses have revealed statistically significant associations between lower nSES and poorer OS; between lower nSES and worse CSS; between Medicaid/public insurance and worse OS; and between no-insurance and worse OS. These findings underscore the substantial impact of SDH on pediatric age groups. Notably, the influence of SES on survival remains evident even when patients share the same insurance coverage, emphasizing the profound impact of financial burden on survival outcomes (57). On the other hand, it is important to point out that all SES measurements were assessed at community level and there is evidence of poor correlation between the patient and area level SDH measurements (64). Community level measures are indicators of the environment, independently of the individual (64), and should be analyzed with caution. Further investigation is imperative to establish comprehensive criteria for assessing SES. Such criteria should meticulously capture individual and familial resources, as well as the surrounding environment, to enhance our understanding of the nuanced factors influencing health outcomes in these age groups.
This study has some limitations. All examined publications were retrospective studies predominantly utilizing SEER and California Cancer Registry databases. This choice restricts the range of outcomes and variables that can be explored. To comprehensively study all SDH domains and assess other treatment-related outcomes impacted by SDH, there is a clear need for prospective studies encompassing a more diverse representation of the general U.S. population. As a recurrent limitation of systematic reviews, there was a small number of studies that fulfilled the selection criteria, preventing us from performing a quantitative analysis of most SDH analyzed. Furthermore, the majority of analyzed manuscripts focused on hematological cancers within the specified age group. While these cancers are prevalent in this demographic, it results in an underrepresentation of patients with other cancer types, limiting the generalizability of the findings. In the context of meta-analyses, it is important to acknowledge limitations stemming from heterogeneity in SDH measures and/or reference points across manuscripts. These variations may introduce complexities in comparing and synthesizing results. As such, future studies should strive for standardized approaches in measuring and reporting SDH factors to facilitate more robust meta-analyses. Indeed, this issue has been recognized and in 2018 the National Institute on Minority Health and Health Disparities (NIMHD) launched the initiative to build a consensus toolbox of measures on SDH (10). The goal of the PhenX measures for SDH project is “to establish a collection of Common Data Elements (CDEs) to improve the quality and consistency of data acquisition and facilitate collaboration” (10).
Prominent avenues for future research include the exploration of additional SDH variables and a nuanced understanding of the proportional contribution of each SDH domain, along with their interactions, in influencing objective patient outcomes. Recognizing that quality of life (QOL) outcomes may also be impacted by SDH factors, it becomes crucial to consider the potential ascertainment bias introduced by SDH in the measurement of QOL. For children, particularly in the social and community context domain, factors such as school bullying, domestic violence, parents not living together, nontraditional family arrangements, religious beliefs, nutrition status, and access to healthy food merit thorough investigation. Future studies should aim to identify outcome predictive SDH variables that are unique to specific age strata for pediatric patients. This will not only strengthen the evidence base but also provide insights for tailored interventions aimed at improving health outcomes across diverse patient populations.
Conclusion
This study provided the panorama of how the SDH have been measured in children and AYA patients diagnosed with cancer and highlights the need for improvement in this critical field. The most common SDH variables evaluated were health insurance coverage and nSES, as these are broadly available in most public registries. On the other hand, SDH within the social and community context domain, neighborhood and built environment domain, and education access and quality domains were the least explored. We detected the following factors as predictors of poor outcomes of children and AYA patient diagnosed with cancer: non-private insurance, living in areas with low median income, high poverty rates, limited educational resources, lower SES, and being unmarried (for AYA). Developing tailored methods to measure the SDH and, consequently, identify vulnerable children and AYA diagnosed with cancer is a critical need to inform policy decisions and physicians and, ultimately, decrease the disparities in the outcomes of underserved patients.
Data availability statement
The original contributions presented in the study are included in the article/Supplementary Material, further inquiries can be directed to the corresponding author.
Author contributions
MS: Conceptualization, Data curation, Formal Analysis, Investigation, Methodology, Visualization, Writing – original draft. KT: Formal Analysis, Investigation, Project administration, Writing – review & editing. MM-G: Conceptualization, Data curation, Formal Analysis, Investigation, Writing – review & editing. BS: Conceptualization, Writing – review & editing. GA: Formal Analysis, Software, Supervision, Writing – review & editing. EB: Conceptualization, Investigation, Supervision, Writing – review & editing. TJ: Conceptualization, Investigation, Supervision, Writing – review & editing. JC: Conceptualization, Investigation, Supervision, Writing – review & editing.
Funding
The author(s) declare that no financial support was received for the research, authorship, and/or publication of this article.
Acknowledgments
We express our gratitude to Rhea-Beth Markowitz, PhD, for her valuable assistance in reviewing the manuscript.
Conflict of interest
The authors declare that the research was conducted in the absence of any commercial or financial relationships that could be construed as a potential conflict of interest.
Publisher's note
All claims expressed in this article are solely those of the authors and do not necessarily represent those of their affiliated organizations, or those of the publisher, the editors and the reviewers. Any product that may be evaluated in this article, or claim that may be made by its manufacturer, is not guaranteed or endorsed by the publisher.
Supplementary material
The Supplementary Material for this article can be found online at: https://www.frontiersin.org/articles/10.3389/fradm.2024.1441776/full#supplementary-material
Abbreviations
ADI, area deprivation index; ALL, Acute lymphoblastic leukemia; AML, Acute myeloid leukemia; APL, Acute promyelocytic leukemia; AYA, adolescents and young adults; CI, Confidence interval; CSS, cancer-specific survival; HR, hazard ratio; OS, overall survival; SDH, social determinants of health; SEER, Surveillance, Epidemiology, and End Results Program; SES, socioeconomic status; nSES, neighborhood socioeconomic status.
References
1. Siegel RL, Miller KD, Wagle NS, Jemal A. Cancer statistics, 2023. CA Cancer J Clin. (2023) 73(1):17–48. doi: 10.3322/caac.21763
2. Elmore LW, Greer SF, Daniels EC, Saxe CC, Melner MH, Krawiec GM, et al. Blueprint for cancer research: critical gaps and opportunities. CA Cancer J Clin. (2021) 71(2):107–39. doi: 10.3322/caac.21652
3. Taylor R, Rieger A. Medicine as social science: Rudolf Virchow on the typhus epidemic in upper silesia. Int J Health Serv. (1985) 15(4):547–59. doi: 10.2190/XX9V-ACD4-KUXD-C0E5
5. Graham H, White PCL. Social determinants and lifestyles: integrating environmental and public health perspectives. Public Health. (2016) 141:270–8. doi: 10.1016/j.puhe.2016.09.019
6. 2030 Happy People. U.S. Department of Health and Human Services, Office of Disease Prevention and Health Promotion. Available online at: https://health.gov/healthypeople/objectives-and-data/social-determinants-health (Accessed May 5, 2024).
7. World Health Organization. Social determinants of health. (2023). Available from: https://www.who.int/health-topics/social-determinants-of-health#tab=tab_1 (Accessed May 5, 2024).
8. Marmot M, Friel S, Bell R, Houweling TA, Taylor S. Closing the gap in a generation: health equity through action on the social determinants of health. Lancet. (2008) 372(9650):1661–9. doi: 10.1016/S0140-6736(08)61690-6
9. Tran Y H, Coven SL, Park S, Mendonca EA. Social determinants of health and pediatric cancer survival: a systematic review. Pediatr Blood Cancer. (2022) 69(5):e29546. doi: 10.1002/pbc.29546
10. Hamilton CM, Strader LC, Pratt JG, Maiese D, Hendershot T, Kwok RK, et al. The PhenX toolkit: get the most from your measures. Am J Epidemiol. (2011) 174(3):253–60. doi: 10.1093/aje/kwr193
11. Miranda-Galvis M, Tjioe K, Balas EA, Agrawal G, Cortes JE. Disparities in survival of hematologic malignancies in the context of social determinants of health: a systematic review. Blood Adv. (2023) 7(21):6466–91. doi: 10.1182/bloodadvances.2023010690
12. Tjioe KC, Miranda-Galvis M, Johnson MS, Agrawal G, Balas EA, Cortes JE. The interaction between social determinants of health and cervical cancer survival: a systematic review. Gynecol Oncol. (2024) 181:141–54. doi: 10.1016/j.ygyno.2023.12.020
13. Maloney ME, Bacak C, Tjioe K, Davis LS, Andrew Balas E, Agrawal G, et al. The intersection of melanoma survival and social determinants of health in the United States: a systematic review. JAAD Int. (2024) 1:106–8. doi: 10.1016/j.jdin.2024.07.006
14. Neuzil K, Apple A, Sybenga A, Chen H, Zhao S, Whiteside M, et al. Health disparities among Tennessee pediatric renal tumor patients. J Pediatr Surg. (2020) 55(6):1081–7. doi: 10.1016/j.jpedsurg.2020.02.029
15. Kehm RD, Spector LG, Poynter JN, Vock DM, Altekruse SF, Osypuk TL. Does socioeconomic status account for racial and ethnic disparities in childhood cancer survival? Cancer. (2018) 124(20):4090–7. doi: 10.1002/cncr.31560
16. Roth M, Berkman A, Andersen CR, Cuglievan B, Andrew Livingston J, Hildebrandt M, et al. Improved survival of young adults with cancer following the passage of the affordable care act. Oncologist. (2022) 27(2):135–43. doi: 10.1093/oncolo/oyab049
17. Collins CL, Peng J, Singh S, Hamilton AS, Freyer DR. Patterns of cancer care and association with survival among younger adolescents and young adults: a population-based retrospective cohort study. Cancer Epidemiol Biomarkers Prev. (2021) 30(11):2105–13. doi: 10.1158/1055-9965.EPI-21-0530
18. Schraw JM, Peckham-Gregory EC, Hughes AE, Scheurer ME, Pruitt SL, Lupo PJ. Residence in a hispanic enclave is associated with inferior overall survival among children with acute lymphoblastic leukemia. Int J Environ Res Public Health. (2021) 18(17):9273. doi: 10.3390/ijerph18179273
19. Murphy CC, Lupo PJ, Roth ME, Winick NJ, Pruitt SL. Disparities in cancer survival among adolescents and young adults: a population-based study of 88 000 patients. J Natl Cancer Inst. (2021) 113(8):1074–83. doi: 10.1093/jnci/djab006
20. Colton MD, Goulding D, Beltrami A, Cost C, Franklin A, Cockburn MG, et al. A US population-based study of insurance disparities in cancer survival among adolescents and young adults. Cancer Med. (2019) 8(10):4867–74. doi: 10.1002/cam4.2230
21. Tai EW, Ward KC, Bonaventure A, Siegel DA, Coleman MP. Survival among children diagnosed with acute lymphoblastic leukemia in the United States, by race and age, 2001 to 2009: findings from the CONCORD-2 study. Cancer. (2017) 123:5178–89. doi: 10.1002/cncr.30899
22. Garner EF, Maizlin II, Dellinger MB, Gow KW, Goldfarb M, Goldin AB, et al. Effects of socioeconomic status on children with well-differentiated thyroid cancer. Surgery. (2017) 162(3):662–9. doi: 10.1016/j.surg.2017.04.008
23. Ryan JL, Eddington AR, Hullmann SE, Ramsey RR, Wolfe-Christensen C, Chaney JM, et al. An examination of parenting capacity variables and child adjustment outcomes across socioeconomic level in pediatric cancer. Children’s Health Care. (2013) 42(3):281–93. doi: 10.1080/02739615.2013.816617
24. Smith EC, Ziogas A, Anton-Culver H. Association between insurance and socioeconomic status and risk of advanced stage hodgkin lymphoma in adolescents and young adults. Cancer. (2012) 118(24):6179–87. doi: 10.1002/cncr.27684
25. Foliart D, Pollock B, Mezei G, Iriye R, Silva J, Ebi K, et al. Magnetic field exposure and long-term survival among children with leukaemia. Br J Cancer. (2006) 94(1):161–4. doi: 10.1038/sj.bjc.6602916
26. Tai E, Hallisey E, Peipins LA, Flanagan B, Lunsford NB, Wilt G, et al. Geographic access to cancer care and mortality among adolescents. J Adolesc Young Adult Oncol. (2018) 7(1):22–9. doi: 10.1089/jayao.2017.0066
27. Puckett Y, Ta A. Socioeconomic factors impact inpatient mortality in pediatric lymphoma patients. Cureus. (2016) 8(5):e624. doi: 10.7759/cureus.624
28. Fineberg R, Zahedi S, Eguchi M, Hart M, Cockburn M, Green AL. Population-based analysis of demographic and socioeconomic disparities in pediatric CNS cancer survival in the United States. Sci Rep. (2020) 10(1):4588. doi: 10.1038/s41598-020-61237-2
29. Kent EE, Sender LS, Largent JA, Anton-Culver H. Leukemia survival in children, adolescents, and young adults: influence of socioeconomic status and other demographic factors. Cancer Causes Control. (2009) 20(8):1409–20. doi: 10.1007/s10552-009-9367-2
30. Abrahao R, Lichtensztajn DY, Ribeiro RC, Marina NM, Keogh RH, Marcos-Gragera R, et al. Racial/ethnic and socioeconomic disparities in survival among children with acute lymphoblastic leukemia in California, 1988–2011: a population-based observational study. Pediatr Blood Cancer. (2015) 62(10):1819–25. doi: 10.1002/pbc.25544
31. Abrahao R, Ribeiro RC, Medeiros BC, Keogh RH, Keegan TH. Disparities in early death and survival in children, adolescents, and young adults with acute promyelocytic leukemia in California. Cancer. (2015) 121(22):3990–7. doi: 10.1002/cncr.29631
32. Seif AE, Fisher BT, Li Y, Torp K, Rheam DP, Huang YS, et al. Patient and hospital factors associated with induction mortality in acute lymphoblastic leukemia. Pediatr Blood Cancer. (2014) 61(5):846–52. doi: 10.1002/pbc.24855
33. Bona K, Blonquist TM, Neuberg DS, Silverman LB, Wolfe J. Impact of socioeconomic Status on timing of relapse and overall survival for children treated on dana-farber cancer institute ALL consortium protocols (2000–2010). Pediatr Blood Cancer. (2016) 63(6):1012–8. doi: 10.1002/pbc.25928
34. Rotz SJ, Wei W, Thomas SM, Hanna R. Distance to treatment center is associated with survival in children and young adults with acute lymphoblastic leukemia. Cancer. (2020) 126(24):5319–27. doi: 10.1002/cncr.33175
35. Knoble NB, Alderfer MA, Hossain MJ. Socioeconomic status (SES) and childhood acute myeloid leukemia (AML) mortality risk: analysis of SEER data. Cancer Epidemiol. (2016) 44:101–8. doi: 10.1016/j.canep.2016.07.007
36. Jamy OH, Godby R, Dhir A, Costa LJ, Xavier AC. Impact of insurance status on the survival of younger patients diagnosed with acute promyelocytic leukemia in the United States. Cancer. (2021) 127(16):2966–73. doi: 10.1002/cncr.33593
37. Schraw JM, Peckham-Gregory EC, Rabin KR, Scheurer ME, Lupo PJ, Oluyomi A. Area deprivation is associated with poorer overall survival in children with acute lymphoblastic leukemia. Pediatr Blood Cancer. (2020) 67(9):e28525. doi: 10.1002/pbc.28525
38. Kent EE, Morris RA, Largent JA, Ziogas A, Sender LS, Anton-Culver H. Socioeconomic impacts on survival differ by race/ethnicity among adolescents and young adults with non-hodgkin’s lymphoma. J Cancer Epidemiol. (2010) 2010:824691. doi: 10.1155/2010/824691
39. Metzger ML, Castellino SM, Hudson MM, Rai SN, Kaste SC, Krasin MJ, et al. Effect of race on the outcome of pediatric patients with hodgkin’s lymphoma. J Clin Oncol. (2008) 26(8):1282–8. doi: 10.1200/JCO.2007.14.0699
40. Keegan TH, DeRouen MC, Parsons HM, Clarke CA, Goldberg D, Flowers CR, et al. Impact of treatment and insurance on socioeconomic disparities in survival after adolescent and young adult hodgkin lymphoma: a population-based study. Cancer Epidemiol Biomarkers Prev. (2016) 25(2):264–73. doi: 10.1158/1055-9965.EPI-15-0756
41. Chao C, Chiu V, Xu L, Cooper RM. Survival differences by race/ethnicity and neighborhood socioeconomic Status in adolescents and young adults diagnosed with non-hodgkin lymphoma. J Adolesc Young Adult Oncol. (2015) 4(2):76–83. doi: 10.1089/jayao.2014.0037
42. Abrahao R, Cooley JJP, Maguire FB, Parikh-Patel A, Morris CR, Schwarz EB, et al. Stage at diagnosis and survival among adolescents and young adults with lymphomas following the affordable care act implementation in California. Int J Cancer. (2022) 150(7):1113–22. doi: 10.1002/ijc.33880
43. Kahn JM, Zhang X, Kahn AR, Castellino SM, Neugut AI, Schymura MJ, et al. Racial disparities in children, adolescents, and young adults with hodgkin lymphoma enrolled in the New York state medicaid program. J Adolesc Young Adult Oncol. (2022) 11(4):360–9. doi: 10.1089/jayao.2021.0131
44. Huang C, Hu H, Jia L, Liu H, Hu S. Outcomes of lymphoma among American adolescent and young adult patients varied by health insurance-a SEER-based study. J Pediatr Hematol Oncol. (2022) 44(2):e403–12. doi: 10.1097/MPH.0000000000002314
45. Austin MT, Hamilton E, Zebda D, Nguyen H, Eberth JM, Chang Y, et al. Health disparities and impact on outcomes in children with primary central nervous system solid tumors. J Neurosurg Pediatr. (2016) 18(5):585–93. doi: 10.3171/2016.5.PEDS15704
46. Wolfson J, Sun CL, Kang T, Wyatt L, D'Appuzzo M, Bhatia S. Impact of treatment site in adolescents and young adults with central nervous system tumors. J Natl Cancer Inst. (2014) 106(8):dju166. doi: 10.1093/jnci/dju166
47. Adel Fahmideh M, Schraw JM, Chintagumpala M, Lupo PJ, Oluyomi AO, Scheurer ME. Neighborhood socioeconomic deprivation and mortality in children with central nervous system tumors. Cancer Epidemiol Biomarkers Prev. (2021) 30(12):2278–85. doi: 10.1158/1055-9965.EPI-21-0368
48. Mitchell HK, Morris M, Ellis L, Abrahao R, Bonaventure A. Racial/ethnic and socioeconomic survival disparities for children and adolescents with central nervous system tumours in the United States, 2000–2015. Cancer Epidemiol. (2020) 64:101644. doi: 10.1016/j.canep.2019.101644
49. Penumarthy NL, Goldsby RE, Shiboski SC, Wustrack R, Murphy P, Winestone LE. Insurance impacts survival for children, adolescents, and young adults with bone and soft tissue sarcomas. Cancer Med. (2020) 9(3):951–8. doi: 10.1002/cam4.2739
50. Lee DY, Teng A, Pedersen RC, Tavangari FR, Attaluri V, McLemore EC, et al. Racial and socioeconomic treatment disparities in adolescents and young adults with stage II-III rectal cancer. Ann Surg Oncol. (2017) 24(2):311–8. doi: 10.1245/s10434-016-5626-0
51. Lara J, Brunson A, Keegan TH, Malogolowkin M, Pan CX, Yap S, et al. Determinants of survival for adolescents and young adults with urothelial bladder cancer: results from the California cancer registry. J Urol. (2016) 196(5):1378–82. doi: 10.1016/j.juro.2016.05.082
52. Hamilton EC, Nguyen HT, Chang YC, Eberth JM, Cormier J, Elting LS, et al. Health disparities influence childhood melanoma stage at diagnosis and outcome. J Pediatr. (2016) 175:182–7. doi: 10.1016/j.jpeds.2016.04.068
53. Gruszczynski NR, Low CM, Choby G, Meister KD, Smith BH, Balakrishnan K. Effects of social determinants of health care on pediatric thyroid cancer outcomes in the United States. Otolaryngol Head Neck Surg. (2022) 166(6):1045–54. doi: 10.1177/01945998211032901
54. Derouen MC, Gomez SL, Press DJ, Tao L, Kurian AW, Keegan TH. A population-based observational study of first-course treatment and survival for adolescent and young adult females with breast cancer. J Adolesc Young Adult Oncol. (2013) 2(3):95–103. doi: 10.1089/jayao.2013.0004
55. Chalfant V, Riveros C, Stec AA. Effect of social disparities on 10 year survival in pediatric patients with Wilms’ tumor. Cancer Med. (2023) 12(3):3452–9. doi: 10.1002/cam4.5124
56. Austin MT, Nguyen H, Eberth JM, Chang Y, Heczey A, Hughes DP, et al. Health disparities are important determinants of outcome for children with solid tumor malignancies. J Pediatr Surg. (2015) 50(1):161–6. doi: 10.1016/j.jpedsurg.2014.10.037
57. DeRouen MC, Parsons HM, Kent EE, Pollock BH, Keegan THM. Sociodemographic disparities in survival for adolescents and young adults with cancer differ by health insurance status. Cancer Causes Control. (2017) 28(8):841–51. doi: 10.1007/s10552-017-0914-y
58. Keegan TH, Grogan RH, Parsons HM, Tao L, White MG, Onel K, et al. Sociodemographic disparities in differentiated thyroid cancer survival among adolescents and young adults in California. Thyroid. (2015) 25(6):635–48. doi: 10.1089/thy.2015.0021
59. Lee J-Y, Kim S, Kim YT, Lim MC, Lee B, Jung K-W, et al. Changes in ovarian cancer survival during the 20 years before the era of targeted therapy. BMC Cancer. (2018) 18(1):1–8. doi: 10.1186/s12885-017-3892-2
60. Rosenberg AR, Kroon L, Chen L, Li CI, Jones B. Insurance status and risk of cancer mortality among adolescents and young adults. Cancer. (2015) 121(8):1279–86. doi: 10.1002/cncr.29187
61. Wang J, Ha J, Lopez A, Bhuket T, Liu B, Wong RJ. Medicaid and uninsured hepatocellular carcinoma patients have more advanced tumor stage and are less likely to receive treatment. J Clin Gastroenterol. (2018) 52(5):437–43. doi: 10.1097/MCG.0000000000000859
62. Martin S, Ulrich C, Munsell M, Taylor S, Lange G, Bleyer A. Delays in cancer diagnosis in underinsured young adults and older adolescents. Oncologist. (2007) 12(7):816–24. doi: 10.1634/theoncologist.12-7-816
63. Morrison VA, Bell JA, Hamilton L, Ogbonnaya A, Shih H-C, Hennenfent K, et al. Economic burden of patients with diffuse large B-cell and follicular lymphoma treated in the USA. Future Oncology. (2018) 14(25):2627–42. doi: 10.2217/fon-2018-0267
Keywords: social determinants of health, hematological malignancies, pediatric, adolescents, survival, young adults, cancer, hematology
Citation: Sharara M, Tjioe KC, Miranda-Galvis M, Santellano Juarez B, Agrawal G, Balas EA, Johnson TS and Cortes JE (2024) Social determinants of health impact on cancer affecting children, adolescents, and young adults: systematic review and meta-analysis. Front. Adolesc. Med. 2:1441776. doi: 10.3389/fradm.2024.1441776
Received: 31 May 2024; Accepted: 30 August 2024;
Published: 13 September 2024.
Edited by:
Thomas G. Knight, Levine Cancer Institute, United StatesReviewed by:
Rana Bayakly, Georgia Department of Public Health, United StatesPinki Prasad, Louisiana State University, United States
Susanne Schmidt, The University of Texas Health Science Center at San Antonio, United States
Copyright: © 2024 Sharara, Tjioe, Miranda-Galvis, Santellano Juarez, Agrawal, Balas, Johnson and Cortes. This is an open-access article distributed under the terms of the Creative Commons Attribution License (CC BY). The use, distribution or reproduction in other forums is permitted, provided the original author(s) and the copyright owner(s) are credited and that the original publication in this journal is cited, in accordance with accepted academic practice. No use, distribution or reproduction is permitted which does not comply with these terms.
*Correspondence: Jorge E. Cortes, am9yZ2UuY29ydGVzQGF1Z3VzdGEuZWR1