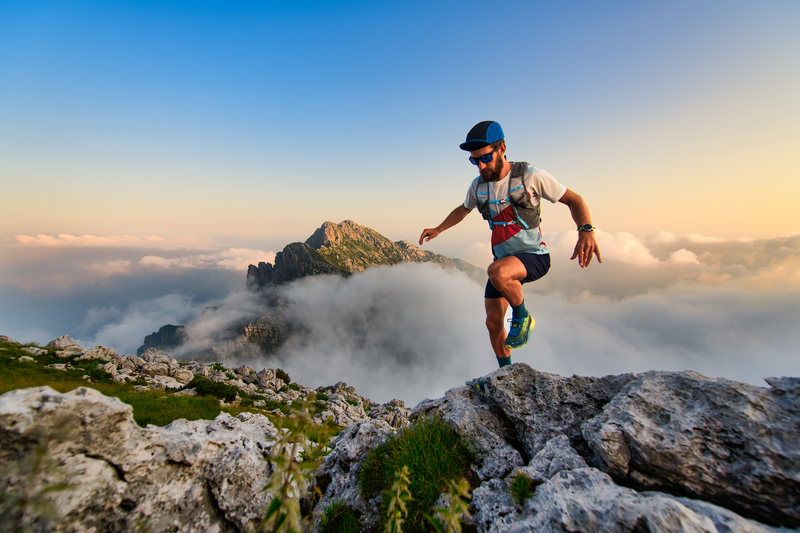
94% of researchers rate our articles as excellent or good
Learn more about the work of our research integrity team to safeguard the quality of each article we publish.
Find out more
ORIGINAL RESEARCH article
Front. Acoust.
Sec. Ultrasound Technologies
Volume 3 - 2025 | doi: 10.3389/facou.2025.1545057
This article is part of the Research Topic Insights in Ultrasound Technologies: 2024 View all 5 articles
The final, formatted version of the article will be published soon.
You have multiple emails registered with Frontiers:
Please enter your email address:
If you already have an account, please login
You don't have a Frontiers account ? You can register here
In this in silico, in vitro and in vivo study, we propose metrics for the characterization of highly scattering media using backscattered acoustic waves in the MHz range, for application to the characterization of biological media. Multi-element array transducers are used to record the ultrasonic Inter-element Response Matrix (IRM) of scattering phantoms and of lungs tissue in rodent models of pulmonary fibrosis. The distribution of singular values of the IRM in the frequency domain is then studied to quantify the multiple scattering contribution. Numerical models of scattering media, as well as Gelatin-Glass bead and PDMS phantoms with different scatterer densities are used as a first step to demonstrate the proof of concept. The results show that changes in microstructure of a complex random medium affect parameters associated with the distribution of singular values. Two metrics are proposed: E(X), which is the expected value of the singular value distribution and λ max , the maximum value of the probability density function of the singular value distribution, ie the most represented singular value. After validation of the methods in silico and in phantoms, we show that these metrics are relevant to evaluate pulmonary fibrosis in an in vivo rodent study on six control rats and eighteen rats with varying degrees of severity of pulmonary fibrosis. In rats, a moderate correlation was found between the severity of pulmonary fibrosis and metrics E(X) and λ max . These results suggest that such parameters could be used as metrics to estimate the amount of multiple scattering in highly heterogeneous media, and that these parameters could contribute to the evaluation of structural changes in lung microstructure.
Keywords: ultrasound, Tissue characterization, random media, multiple scattering, scattering, Quantitative ultrasound
Received: 13 Dec 2024; Accepted: 14 Feb 2025.
Copyright: © 2025 Yousefian, Dashti, Karbalaeisadegh, Yao, Blackwell, Montgomery, Zhu, Egan and MULLER. This is an open-access article distributed under the terms of the Creative Commons Attribution License (CC BY). The use, distribution or reproduction in other forums is permitted, provided the original author(s) or licensor are credited and that the original publication in this journal is cited, in accordance with accepted academic practice. No use, distribution or reproduction is permitted which does not comply with these terms.
* Correspondence:
MARIE MULLER, North Carolina State University, Raleigh, United States
Disclaimer: All claims expressed in this article are solely those of the authors and do not necessarily represent those of their affiliated organizations, or those of the publisher, the editors and the reviewers. Any product that may be evaluated in this article or claim that may be made by its manufacturer is not guaranteed or endorsed by the publisher.
Research integrity at Frontiers
Learn more about the work of our research integrity team to safeguard the quality of each article we publish.