- 1Mayo Clinic College of Medicine, Mayo Clinic, Rochester, MN, USA
- 2Department of Neurologic Surgery, Mayo Clinic, Rochester, MN, USA
- 3Department of Neurology, Mayo Clinic, Rochester, MN, USA
- 4Division of Engineering, Mayo Clinic, Rochester, MN, USA
- 5Department of Physiology and Biomedical Engineering, Mayo Clinic, Rochester, MN, USA
Current strategies for optimizing deep brain stimulation (DBS) therapy involve multiple postoperative visits. During each visit, stimulation parameters are adjusted until desired therapeutic effects are achieved and adverse effects are minimized. However, the efficacy of these therapeutic parameters may decline with time due at least in part to disease progression, interactions between the host environment and the electrode, and lead migration. As such, development of closed-loop control systems that can respond to changing neurochemical environments, tailoring DBS therapy to individual patients, is paramount for improving the therapeutic efficacy of DBS. Evidence obtained using electrophysiology and imaging techniques in both animals and humans suggests that DBS works by modulating neural network activity. Recently, animal studies have shown that stimulation-evoked changes in neurotransmitter release that mirror normal physiology are associated with the therapeutic benefits of DBS. Therefore, to fully understand the neurophysiology of DBS and optimize its efficacy, it may be necessary to look beyond conventional electrophysiological analyses and characterize the neurochemical effects of therapeutic and non-therapeutic stimulation. By combining electrochemical monitoring and mathematical modeling techniques, we can potentially replace the trial-and-error process used in clinical programming with deterministic approaches that help attain optimal and stable neurochemical profiles. In this manuscript, we summarize the current understanding of electrophysiological and electrochemical processing for control of neuromodulation therapies. Additionally, we describe a proof-of-principle closed-loop controller that characterizes DBS-evoked dopamine changes to adjust stimulation parameters in a rodent model of DBS. The work described herein represents the initial steps toward achieving a “smart” neuroprosthetic system for treatment of neurologic and psychiatric disorders.
Introduction
Neurologic and psychiatric disorders can be characterized by motor, behavioral, cognitive, affective, or perceptual traits that affect how individuals move, feel, think, and behave (Benabid et al., 2005; Nemeroff, 2007; Williams and Okun, 2013). These disorders affect over 94 million people in the United States alone with health-care related costs exceeding $648 billion (Logothetis, 2003b; Benabid et al., 2005; Speert et al., 2012; Williams and Okun, 2013). Although most individuals suffering from neurologic and psychiatric disorders are successfully treated with a combination of medications and therapy, up to 30% of patients are unable to respond to standard therapeutic interventions (Olanow et al., 2000; Benabid et al., 2005; Hamani et al., 2006; Nemeroff, 2007; Williams and Okun, 2013). For these treatment-resistant patients, high-frequency electrical stimulation of subcortical brain structures, known as deep brain stimulation (DBS), presents a highly successful therapeutic alternative (Benabid et al., 2005; Williams and Okun, 2013). DBS is FDA-approved for the treatment of Parkinson's disease (PD) and essential tremor (ET) (Benabid et al., 1987, 1991; Burchiel et al., 1999; Koller et al., 2001; Obeso and Guridi, 2001; Simuni et al., 2002; Rehncrona et al., 2003; Germano et al., 2004; Rodriguez-Oroz, 2004; Blomstedt and Hariz, 2010; Moro et al., 2010; Weiss et al., 2013). Additionally, DBS has received humanitarian device exemptions for dystonia and obsessive-compulsive disorder, and there are multiple studies underway for the treatment of other neurologic and psychiatric disorders (Benabid et al., 1987, 1991; Burchiel et al., 1999; Obeso and Guridi, 2001; Simuni et al., 2002; Velasco et al., 2005; Lim et al., 2007; Mueller et al., 2008; Blomstedt and Hariz, 2010; Denys et al., 2010; Fisher et al., 2010; Ramasubbu et al., 2013).
Brain stimulation has been an important tool in the field of neurosurgery pioneered by Spiegel and Wycis (Blomstedt and Hariz, 2010). Intra-operative electrical stimulation of neural tissue has been used since the early days of human stereotaxis to identify surgical targets (Gildenberg, 2003, 2005). Application of brain stimulation in modern-day neurosurgery was revolutionized by Benabid and colleagues, who used high frequency stimulation (typically 100–130 Hz) delivered directly into specific brain regions to mimic the effects of surgical lesions without performing any tissue resection (Benabid et al., 1987, 1991; Blomstedt and Hariz, 2010). DBS achieves therapeutic benefits by delivering electrical currents to specific anatomical targets within the brain via multi-contact electrodes connected to implanted pulse generators. In DBS therapy, a balance between maximal clinical improvement and minimal stimulation-induced side effects is typically achieved by adjusting active electrode contacts, stimulus frequency, amplitude, and pulse duration.
Clinical DBS programming is an iterative process in which stimulation parameters are adjusted in order to maximize therapeutic benefits while minimizing side effects (Morishita et al., 2013) Although many DBS patients require minimal stimulation adjustment following surgery, many more require several months of regular parameter adjustments before optimal therapeutic results can be achieved (Okun et al., 2005; Bronstein et al., 2011; Kluger et al., 2011). However, sustaining these therapeutic benefits requires subsequent adjustment of stimulation parameters every few months (Mayberg et al., 2000, 2005; Deuschl et al., 2006; Moro et al., 2006; Frankemolle et al., 2010; Mure et al., 2011). Therefore, existing clinical programming and stimulation paradigms are poorly suited to cope with the dynamic and comorbid nature of most neurologic disorders. This, in turn, highlights the need for dynamic feedback systems that can continually and automatically adjust stimulation parameters in response to changes within the environment of the brain.
Therapeutic Stimulation Paradigms
The therapeutic success of DBS depends not only on accurate surgical targeting and electrode implantation, but also on the ability to optimize stimulation parameters to maximize therapeutic benefits while minimizing side effects. Clinical strategies for therapeutic DBS programming require multiple post-operative visits during which experienced clinicians perform clinical evaluations and corresponding device programming. In each visit, a series of inputs (active contacts, stimulus amplitude, pulse width, and frequency) are adjusted in an attempt to minimize adverse effects while maximizing clinical benefits. Although this strategy has provided significant patient benefit, the results are far from optimal. First, this open loop strategy relies on the subjective experiences of both the patient and clinician, without providing objective feedback to support parameter optimization. Second, the therapeutic response observed in this acute setting does not guarantee sustained therapeutic effects. Disease progression, environmental factors, and behaviorally induced changes in network activity can all render therapeutic stimulation ineffective, requiring additional programming sessions (Obeso and Guridi, 2001; Hunka et al., 2005; Kupsch et al., 2011). Third, the procedure is costly and time consuming. As such, only a fraction of the stimulation parameter space can be practically explored during each session. Fourth, DBS device programming can differ according to the target chosen, the orientation of the electrode relative to the target, the disorder being treated, and the symptoms being treated for a given disorder (Velasco et al., 2007; Ricchi et al., 2012; Min et al., 2013; Miocinovic et al., 2013). Additionally, the timing of programming as well as the waiting time between adjustments can influence when different therapeutic responses can be observed, and these responses also vary between disorders (e.g., Tremor is nearly immediate, whereas depression could take several weeks to observe the effect of a disorder) (Velasco et al., 2007; Ricchi et al., 2012; Min et al., 2013; Miocinovic et al., 2013). Therefore, it is necessary to implement DBS control strategies that can adjust stimulation parameters in real-time according to quantifiable and objective neurochemical, physiological, and behavioral changes while reducing the frequency of clinical interventions. However, before such control strategies can be implemented, it is necessary to improve the understanding of the cellular mechanisms responsible for the network effects of DBS.
The cellular response of single neurons to extracellular electrical fields has been well characterized over short time scales (Smith and Grace, 1992; Benazzouz et al., 2000; Hashimoto et al., 2003; Maurice et al., 2003; Kita et al., 2005; Miocinovic et al., 2006). It is known that excitation of efferent axons or fibers of passage near the site of stimulation results in network changes in neurotransmission and electrical activity (Grill et al., 2004; McIntyre et al., 2004a,b; Johnson et al., 2008; McIntyre and Hahn, 2010; Shah et al., 2010). Furthermore, functional and metabolic imaging studies have shown that successful treatment of neurologic and psychiatric disorders is associated with metabolic normalization in proximal and distal regions of the brain (Mayberg et al., 2000, 2005; Mure et al., 2011). The precise relationships between therapeutic improvement and changes in metabolic patterns remain unknown. As such, current research efforts focus on the use electrophysiology and electrochemistry to elucidate the network effects of DBS (Bledsoe et al., 2009; Lee et al., 2011; Vitek et al., 2012).
Real-Time Monitoring of Neural Activity
Signaling within the brain occurs both electrically and chemically. Technological advances in neural activity monitoring have enabled real-time investigation of cellular and molecular dynamics using electrophysiological and neurochemical probes. While the most used technique involves electrophysiological monitoring of extra-cellular neuronal activity (Smith and Grace, 1992; Benazzouz et al., 2000; Hashimoto et al., 2003; Maurice et al., 2003; Johnson et al., 2005; Kita et al., 2005; Miocinovic et al., 2006) recent advances in electrode technology allow in vivo monitoring of synaptic neurotransmitter activity (Roham et al., 2007; van Gompel et al., 2010).
Electrophysiological analysis has been widely used to study stimulation-evoked changes in brain activity, such as increased pallidal (Hashimoto et al., 2003; Kita et al., 2005; Miocinovic et al., 2006) and nigral activity (Smith and Grace, 1992; Benazzouz et al., 2000; Maurice et al., 2003) during subthalamic nucleus (STN) DBS. This has been accomplished by recording single neuron activity (single unit recordings), activity from local groups of neurons (multi unit activity, local field potentials), and distributed signals representing global brain activity [electrocorticograms (ECoGs), electroencephalograms (EEGs)]. Alternatively, neurochemical analysis techniques such as microdialysis, amperometry, and voltammetry, can detect local changes in neurotransmitter concentration evoked by internal and external mechanical, electrical, and chemical stimuli (Dale et al., 2005; Wightman, 2006). Neurochemical recordings have been used to monitor in vivo release of analytes such as oxygen, dopamine, adenosine, serotonin, and glutamate in small and large animal models of DBS (Agnesi et al., 2009; Bledsoe et al., 2009; Chang et al., 2009; Kimble et al., 2009; Griessenauer et al., 2010; Shon et al., 2010a,b).
Single-Unit Recordings
Single unit recordings capture the activity of distinct neurons in vivo by placing a high-impedance microelectrode within the extracellular space surrounding the cell body. These electrodes, having surface areas under 2 × 10-5 cm2 (Loffler, 2012), record extracellular potentials representative of intracellular action potentials from neurons adjacent to the electrode tip. The high spatial and temporal resolution provided by single unit recordings allows for precise measurements of neuronal spikes (Buzsáki et al., 2012). However, activity from single units can be difficult to isolate due to crosstalk from neighboring cells (Bai and Wise, 2001). Additionally, single unit recordings can be biased toward activity from larger (e.g., pyramidal) cells (Buzsáki et al., 1983). Furthermore, electrode migration, immune responses (e.g., glial scarring), and disruption of surrounding neural tissue interfere with signal quality and limit reliable single unit activity to acute recording conditions (Carter and Houk, 1993; Polikov et al., 2005).
Multi-Unit Recordings
Multi unit recordings capture fast spiking activity from groups of neurons using high-impedance microelectrode arrays. Similar to single unit recordings, this technique provides good spatial and temporal resolution reflecting synaptic events occurring at high frequencies (>800 Hz) (Logothetis, 2003a,b; Mattia et al., 2010). Unfortunately, multi-unit recording arrays suffer from stiff form factors that result in shear-induced inflammation of the surrounding tissue (Cheung, 2007). Furthermore, recording can only occur from the tips of the electrodes, limiting recording selectivity (Maynard et al., 1997).
Local Field Potentials
Local field potential (LFP) analysis is an electrophysiological technique for detecting changes in brain activity that offers great potential for understanding the network effects of DBS (Tsang et al., 2012; Priori et al., 2013). This technique is capable of recording chronic electrical activity directly from single and multiple neural units using micro and macro electrodes implanted within the nucleus of interest (Bronte-Stewart et al., 2009; Giannicola et al., 2012). LFPs are typically used to record low-frequency changes in activity across groups of neurons within a volume of interest (Andersen et al., 2004; Buzsáki et al., 2012; Rosa et al., 2012). These activity changes reflect a weighted average of integrative processes and associations between cells that can be detected over longer distances through extracellular space (Logothetis, 2003a,b; Bronte-Stewart et al., 2009). Unfortunately, the longer recording range of LFP techniques is associated with decreased spatial resolution. Despite this limitation, LFP recordings can be performed in real-time using the same DBS electrode, which eliminates the need for additional electrode penetrations (Rossi et al., 2007). Therefore, local field potentials present a good starting point for establishing closed-loop neurostimulation control systems (Rosin et al., 2011; Santaniello et al., 2011; Berényi et al., 2012; Little et al., 2013).
Global Field Potentials
Analysis of global brain activity can be used to identify both spontaneous and event-related responses from large groups of neurons. Whole-brain electrophysiological brain activity (i.e., global field potentials) is typically measured using far-field sensors located on the scalp (EEG) or directly on the brain surface (ECoG). These global field potentials can be used to identify information regarding high-level sensory processing, perception, and locomotor activity (Issa and Wang, 2013). For example, EEG signals with low spatial resolution can be recorded non-invasively by non-surgically attaching recording electrodes to the scalp. Alternatively, ECoG signals offer increased spatial resolution, but recording electrodes must be surgically attached at the cortical surface (Buzsáki et al., 2012). Despite the advantages of global field potentials, these signals do not provide insight into activity changes within specific subcortical structures. As such, a system that combines activity analysis within cortical (e.g., EcOG) and subcortical (e.g., LFP) networks should provide a better depiction of network dynamics which, in turn, will be required to develop optimal closed-loop stimulation paradigms (Rosa et al., 2012).
Neurochemical Recordings
Neurochemical sensing allows real-time characterization of neural activity with high spatial resolution and signal specificity (Lee et al., 2004). Microdialysis, amperometry, and voltammetry are three widely used techniques for neurochemical monitoring (Blaha and Phillips, 1996).
Microdialysis is a technique for sampling different analytes and determining their concentration in extracellular fluid (Chefer et al., 2009). This technique offers excellent specificity, selectivity, and sensitivity for quantifying neurotransmitter release in a laboratory setting (Watson et al., 2006). However, it suffers from limited temporal resolution (Smolders et al., 1997; Khan et al., 1999). Therefore, microdialysis is not suitable for real-time clinical application in closed-loop systems.
Amperometry is an alternative technique for measuring analytes in the extracellular space. Amperometric recordings involve the application of a fixed electric potential through a carbon fiber microelectrode (CFM) placed in close proximity to the target cells (Gale et al., 2013; Tye et al., 2013). These CFM are coated with specific enzymes known to react with non-electrolytic analytes of interest, resulting in electroactive products that can be electrically measured (Oldenziel et al., 2004). This allows continuous monitoring of changes in electrical currents within the surrounding extracellular fluid. The detected changes in current are caused by oxidative reactions between the applied potential and analyte molecules within the extracellular space (van Gompel et al., 2010). The downfall of this technique is the high complexity associated with chronic in vivo measurements, which require continuous enzyme delivery to detect the breakdown products of the neurotransmitter of interest (Jacobs et al., 2010).
Analogous to amperometry, voltammetry provides real-time high-resolution analyte measurements (Blaha et al., 1990). Specifically, fast scan cyclic voltammetry (FSCV) is a voltammetry technique in which a linearly varying potential is applied to a carbon fiber electrode, allowing for oxidation and reduction of surrounding electroactive molecules to take place (Robinson et al., 2003; Lee et al., 2007). The magnitudes of the analyte oxidation and reduction current peaks are directly proportional to the concentration of analyte oxidized and reduced at the electrode surface (Atcherley et al., 2013). Furthermore, the resulting electrical current vs. applied potential relationships (Figure 1) provide a chemical signature (i.e., voltammogram) that allows identification of specific neurotransmitters or other electroactive analytes (Robinson et al., 2003). FSCV detection of analytes is limited to electroactive molecules such as dopamine, adenosine, and oxygen (van Gompel et al., 2010). Furthermore, the lifetime of CFM is limited to a few months (Kim et al., under review), restricting clinical application of FSCV detection methods to intraoperative approaches.
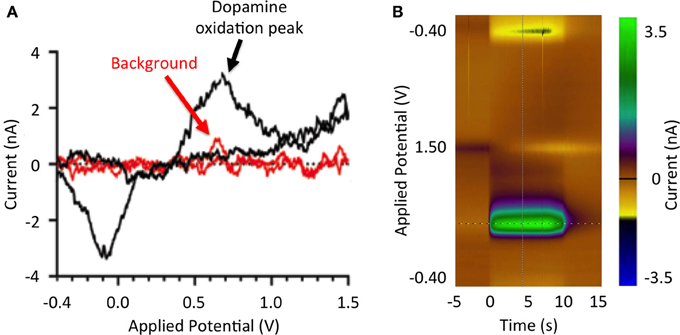
Figure 1. Stimulation-evoked dopamine responses. (A) Dopamine redox reactions at the tip of a carbon fiber microelectrode during fast scan cyclic voltammetry. As the potential applied to the electrode increases from −0.4 to 0.0 V, extracellular dopamine is reduced (reduction peak at −3.5 nA). As the applied potential is further increased from 0.0 to 1.0 V, dopamine is oxidized (oxidation peak at 3.5 nA). Measured current background is shown in red. (B) Pseudo-color representation of dopamine oxidation current at +0.6 V at DBS onset (100 Hz, 2 ms, 300 μA).
Smart DBS Control
Clinical DBS systems follow an open-loop paradigm. That is, stimulation parameters are pre-programmed into the DBS device and held constant until the next programming session, regardless of the internal state of the system or environmental factors (Foltynie and Hariz, 2010). In contrast, closed-loop DBS systems rely on sensor feedback to monitor the environment and internal state of the system in order to adjust stimulation parameters accordingly (Abbott, 2006; Fagg et al., 2007). That is, stimulation parameters (e.g., stimulation frequency, stimulus amplitude, etc.) are automatically adjusted to maintain specific therapeutic outputs such as tremor suppression in the presence of disturbances, environmental perturbations, and internal network changes (Figure 2). To date, development of closed-loop neuroprosthetic devices has largely focused on using electrophysiological activity as feedback signals (Avestruz et al., 2008; Skarpaas and Morrell, 2009; Rosin et al., 2011; Basu et al., 2013; Grant and Lowery, 2013). Neurochemical-based feedback, however, offers the prospect of finer control of stimulation-induced effects, as it allows activity monitoring from individual types of neurons by virtue of their neurotransmitters. The ability to use neurochemical feedback to control DBS has been demonstrated by characterizing glutamate release using mathematical models linking electrical stimulation to glutamate release in a rat model of DBS (Behrend et al., 2009). Thus, chemical sensing presents a unique opportunity for developing closed-loop smart neurocontrol systems that are optimized for specific disorders and targets, and which can account for intra- and inter-patient variability.
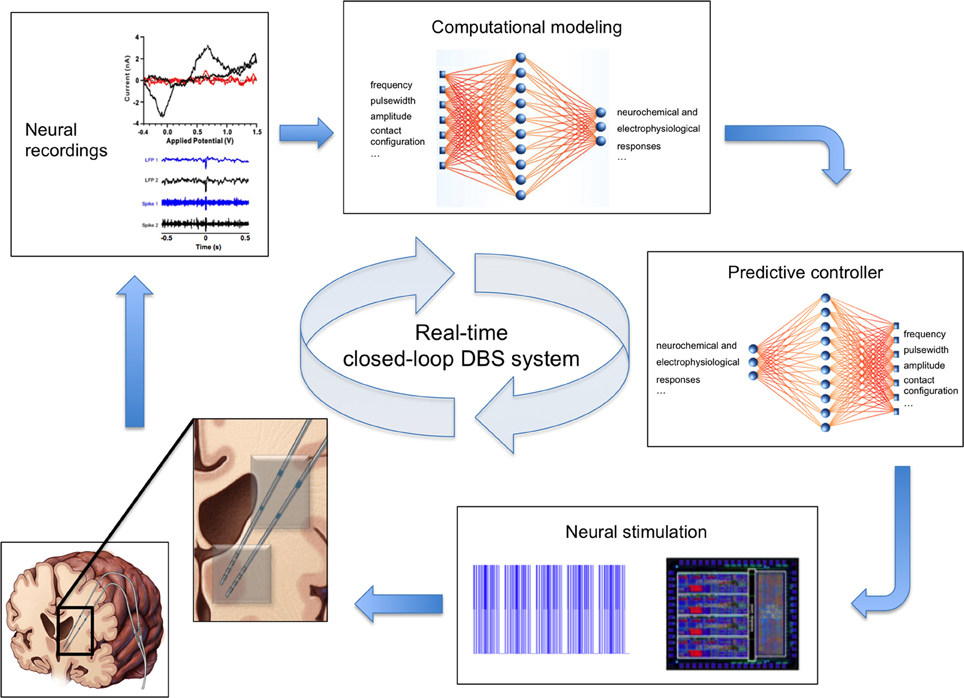
Figure 2. Real-time closed-loop deep brain stimulation system. Clockwise from bottom left: (1) Schematic of the human brain with two electrodes (inset) for simultaneous stimulation (gray contacts) and recording of neural activity (blue contacts). (2) Example voltammogram, local field potentials, and single unit activity signals representing recorded neurochemical and electrophysiological neural activity. (3) Computational model of neurochemical and electrophysiological dynamics allows generation and optimization of data beyond the time constraints imposed by experimental conditions. (4) Smart controller uses existing neural activity to predict stimulation parameters required to achieve therapeutic neuromodulation. (5) Predicted stimulation parameters are applied to the brain using an implanted neurostimulation system.
Neurochemistry of DBS
Studies using small and large animal models suggest that therapeutic DBS coincides with changes in neurotransmitter release (Lee et al., 2004; Shon et al., 2010a,b). It has been established that dopaminergic cell loss in the substantia nigra leads to striatal dopamine deficiency and movement abnormalities in PD patients (MacDonald et al., 2013). It has also been shown that therapeutic STN DBS for treatment of PD decreases the need for exogenous levodopa (Moro et al., 1999; Molinuevo et al., 2000) and has been hypothesized to increase striatal dopamine release (Lee et al., 2009). Complementing findings in electrophysiological and neurochemical sensing studies have shown that STN DBS evokes dopamine release in the striatum of parkinsonian rats (Blaha and Phillips, 1996; Lee et al., 2006). Similarly, stimulation-evoked adenosine release has been recorded intraoperatively in the ventral intermediate nucleus of the thalamus in human patients undergoing DBS for treatment of ET (Chang et al., 2012). However, the specific relationship between DBS and neurochemical activity changes remains unknown. Therefore, understanding the relationships between stimulation parameters and neurotransmitter concentration levels is paramount for developing closed-loop DBS control strategies.
In the following paragraphs, we describe a proof-of-principle approach to closed-loop DBS that automatically adjusts stimulation parameters in order to sustain stable dopamine levels in a rodent model of DBS. The paradigm proposed herein uses FSCV to quantify striatal dopamine release evoked by medial forebrain bundle (MFB) DBS. Additionally, this paradigm relies on non-linear regression, computational modeling, and constrained optimization techniques to parameterize stimulation-evoked dopamine responses. The inverse dynamics of stimulation-evoked dopaminergic responses are modeled using artificial neural networks (ANN), which also predict stimulation parameters required for sustaining target dopaminergic concentration levels. The performance of this closed-loop paradigm was evaluated by comparing target dopaminergic responses to in vivo dopaminergic responses achieved using ANN-predicted stimulation parameters (Figure 4). While focused on DBS of ascending dopaminergic fibers in the MFB for evoking dopamine release in the rat striatum (Agnesi et al., 2009), this closed-loop paradigm is applicable to a variety of analytes, targets, and neurologic disorders.
Experimental Paradigm
To quantify the dynamics of stimulation-evoked dopamine release, recording FSCV CFM and bipolar DBS macroelectrodes were implanted into the striatum and MFB, respectively, in four anesthetized rats. All animal procedures were performed according to the guidelines of the Mayo Clinic Institutional Animal Care and Use Committee (IACUC). Animals were kept on a standard 12 h light-dark cycle with access to food and water ad libitum in conventional housing in accordance with National Institutes of Health (NIH) and US Department of Agriculture guidelines.
Animals were anesthetized and the head was fixed in a Kopf stereotactic frame (David Kopf Instruments, California) for electrode targeting. Following brain exposure, one bipolar stimulating electrode, one FSCV recording electrode, and one silver-chloride reference electrode was inserted into the left MFB, striatum, and contralateral cortex, respectively. Recording electrodes were allowed to stabilize within the tissue environment for 20 min. Finally, the electrodes were connected to a wireless stimulator and neurotransmitter sensor for real-time detection of stimulation-evoked dopamine release (Kimble et al., 2009; Chang et al., 2013).
Following electrode implantation, a comprehensive range of stimulation parameters (Table 1) was used to determine the magnitudes and temporal patterns of stimulation-evoked dopamine release. Stimulation was divided into 65 20-s bins. Each bin corresponded to one combination of stimulation parameters delivered through the active electrode contact. Each stimulation bin was followed by a stabilization and washout period of 180 s.
Stimulation-Evoked Neurochemical Monitoring
Stimulation-evoked dopamine measurements were obtained by changing the CFM potential from a resting potential of −0.4–1.3 V and back, at a rate of 400 V/s. This triangular waveform was repeated at a frequency of 10 Hz (Chang et al., 2012). The CFM was held at the resting potential between scans. We converted the measured oxidation and reduction current peaks to dopamine concentration using post-operative in vitro flow injection analysis calibration of each CFM (Griessenauer et al., 2010). Our preliminary results showed that as MFB DBS amplitude increases, extracellular dopamine levels within the striatum also increase (Figure 3A). A similar response is also observed as pulse duration is increased from 0.1 to 2.0 ms (Figure 3B). Changes in frequency, however, give rise to a different dopaminergic response. Maximum response was observed at 100 Hz, followed by a decrease in dopamine oxidation currents at higher frequencies (Figure 3C).
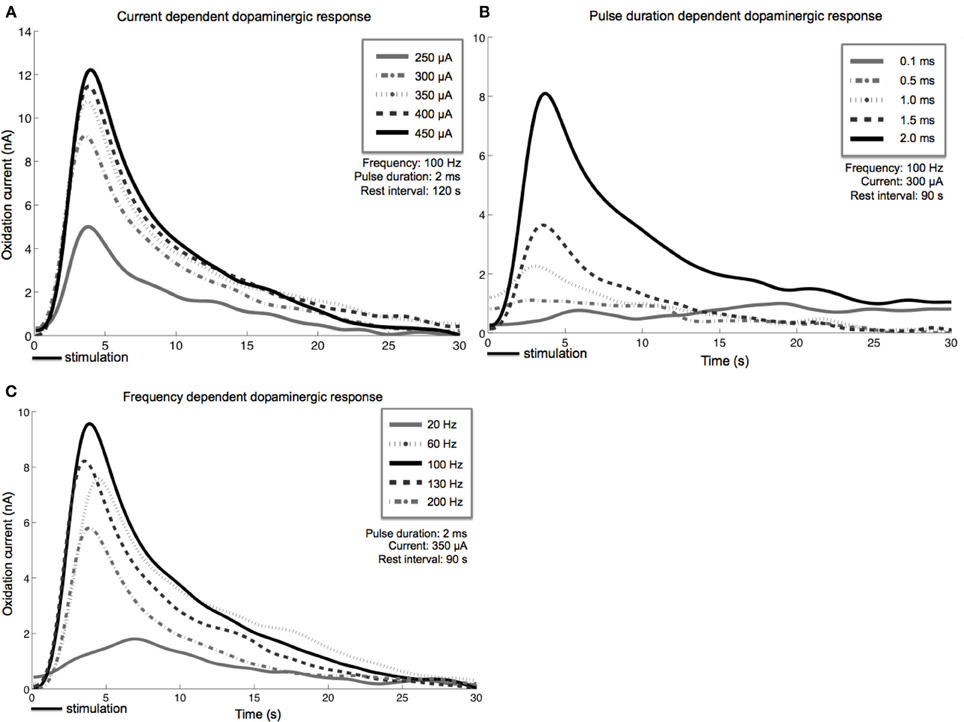
Figure 3. Stimulation-evoked dopamine release characterization in four anesthetized rats. A carbon-fiber recording electrode was implanted into the left striatum and a bipolar stimulating electrode was placed within the ipsilateral medial forebrain bundle. A reference silver-chloride electrode was implanted into the contralateral cortex. Current amplitude (A), pulse duration (B), and frequency (C) were individually varied while the remaining stimulation parameters were held constant. Stimulus duration was set at 2 s for all experiments described above.
Neurochemical Response Modeling
Implementation of neurochemically-driven closed-loop DBS control strategies requires characterization of the relationship between electrical stimulation and neurochemical responses. To characterize this relationship, stimulation-evoked FSCV dopamine signals were low pass filtered (5th-order Butterworth filter, 100 Hz cutoff frequency) to remove signal noise. Additionally, the responses to individual stimuli were characterized using a combination of 7th-degree polynomial and 2nd-order exponential mathematical models. The mathematical model parameters (eight for the polynomial fit and four for the exponential fit) and corresponding stimuli were presented to a double-layer feedforward ANN with sigmoidal and linear transfer functions (Lujan and Crago, 2009). The hidden layer contained 150-hidden neurons. The inputs to the ANN consisted on the stimulation frequency, pulsewidth, and stimulus amplitude, while system outputs corresponded to the 12 model parameters. Initial weights and biases were selected at random for 10 different initial conditions. Ten corresponding ANNs were trained on 80% of the data (selected at random) using the Levenberg-Marquardt algorithm. The trained ANN with the lowest generalization error, calculated using the remaining 20% of data, was selected as a system model. The resulting system model, when combined with constrained optimization for minimization of stimulation energy, can identify and eliminate mathematical redundancies for the optimal design of the closed-loop controller (Lujan and Crago, 2009).
Stimulation Prediction
In order to provide optimal stimulation, a predictive model that characterizes the inverse relationship between stimulation parameters and dopamine levels was created. Similarly to the system model, the predictive model was created using a double-layer ANN with 600 hidden neurons, as well as sigmoidal and linear transfer functions (Lujan and Crago, 2009). The inputs to the predictive model corresponded to the sets of 12 model parameters, while the outputs corresponded to the three stimulation parameters. This inverse model was then used to predict the stimulation parameters required to sustain specific extracellular dopamine levels within the striatum, thus allowing for feedback control. This was followed by stimulation of the MFB using the predicted parameters, and simultaneous recording of extracellular dopamine levels. Root mean squared (RMS) errors between experimentally measured and desired stimulation-evoked dopamine responses were used to determine controller efficacy. Least-squares regression analysis of the dependencies of actual dopamine levels on target levels was used in an effort to identify systematic (e.g., slope, offset) sources of error (Lujan and Crago, 2009).
Closed-Loop Control
Our preliminary results in four anesthetized rats suggest that mathematical models can be used to describe the relationships between stimulation-evoked extracellular dopamine responses and DBS parameters (R2 = 0.8). Furthermore, these results show that adjusting stimulation parameter intensity can modulate dopamine concentration, and that we can use ANN to dynamically predict stimulation parameters required to adjust stimulation-evoked dopamine levels (Figure 4). However, to further understand the network effects of DBS and optimize the therapeutic efficacy of stimulation, it may be necessary to combine electrophysiological (e.g., LFP, ECoG) and neurochemical feedback signals.
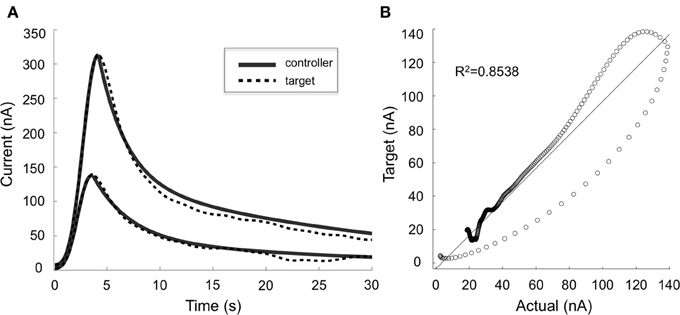
Figure 4. Controller performance. (A) Comparison of target (dotted lines) and actual (solid lines) dopaminergic responses evoked by stimulation parameters predicted by the artificial neural network controller. Two typical responses are shown. (B) Target and actual responses were compared using linear regression and Pearson's correlation (R2 = 0.8538).
Discussion
Frequent adjustment of stimulation settings has been shown to improve the efficacy of DBS therapy (Rosin et al., 2011), which highlights the nature of the changing brain environment. Thus, a smart, automated system capable of dynamically adjusting stimulation parameters in response to a changing environment becomes critical for improving the therapeutic efficacy of DBS therapy. The proof-of-principle closed-loop DBS system proposed above offers the potential for maintaining therapeutic responses during disease progression. By taking advantage of mathematical models, the paradigm presented here can potentially replace the trial-and-error process currently used in clinical programming with deterministic approaches, thereby achieving optimal therapeutic outcomes while minimizing the number of clinical interventions. In turn, this will ultimately reduce required hospital visits and associated healthcare costs (Fraix et al., 2006).
Before automated adjustment of stimulation parameters can be clinically implemented, however, several key clinical questions need to be investigated. Specifically, the relationship between neurotransmitter levels and symptoms of neurologic disease needs further elucidation. For example, there is indirect evidence to suggest that dopamine depletion plays a role in the symptoms of PD and that dopaminergic medications have a therapeutic response. However, precise concentration changes that occur with symptom exacerbation and amelioration are unknown. Additionally, multiple neurotransmitters may play a critical role in the disease (Fitzgerald, 2014). Thus, optimal neurotransmitters and optimal recording locations should be identified for each disorder. Future work should be directed toward validating closed-loop algorithms, correlating neurotransmitter release to clinical benefit in a large animal disease model of Parkinsonism or ET.
Similarly, an important technical barrier that needs to be addressed is that chronic recordings are not possible using current electrode technology. CFMs are subject to electrode fouling due to the charge imbalance of the waveforms required for FSCV. Efforts are underway to develop electrochemical-sensing techniques capable of extending electrode longevity by renewing the electrochemically active surface following adsorption of chemical species (Takmakov et al., 2010). Additionally, it has been reported that diamond coating may potentially prolong the life of recording electrodes (Roham et al., 2007). Once these technologies have been developed, they will need to undergo extensive safety and efficacy testing and validation in pathological animal models before advancing to clinical trials.
Conclusions
Conventional neuromodulation systems have been successful at achieving therapeutic outcomes in patients with neurologic and psychiatric disorders. However, limitations in existing technology make ensuring optimal benefits a difficult and expensive endeavor. Correlation of multi-modal electrophysiological and neurochemical recordings may provide new insight into the cellular and molecular mechanisms of therapeutic neuromodulation. Therefore, development of smart DBS controllers that rely on the relationships between neurochemical and electrophysiological recordings with the clinical effects of DBS offers the potential of replacing the trial-and-error process used in clinical programming with a deterministic approach. Furthermore, the versatility and adaptability of such controllers will allow expansion of the clinical indications that can be treated with DBS while tailoring its application to individual patients and symptoms. In turn, these will likely improve clinical outcomes, reduce the time and frequency of patient visits, and lower overall health care costs.
Conflict of Interest Statement
J. L. Lujan has intellectual property licensed to Boston Scientific. The authors declare that the research was conducted in the absence of any commercial or financial relationships that could be construed as a potential conflict of interest.
Acknowledgments
This work is supported by The Grainger Foundation, NIH grants R01 NS084975 (J. L. Lujan) and R01 NS070872 (Kendall H. Lee). The authors thank Brian Paek, James Baek, and Megan Settel for their assistance with FSCV electrode manufacture and animal surgery.
References
Abbott, A. (2006). Neuroprosthetics: in search of the sixth sense. Nature 442, 125–127. doi: 10.1038/442125a
Agnesi, F., Tye, S. J., Bledsoe, J. M., Griessenauer, C. J., Kimble, C. J., Sieck, G. C., et al. (2009). Wireless Instantaneous Neurotransmitter Concentration System–based amperometric detection of dopamine, adenosine, and glutamate for intraoperative neurochemical monitoring. J. Neurosurg. 111, 701–711. doi: 10.3171/2009.3.JNS0990
Andersen, R. A., Musallam, S., and Pesaran, B. (2004). Selecting the signals for a brain–machine interface. Curr. Opin. Neurobiol. 14, 720–726. doi: 10.1016/j.conb.2004.10.005
Atcherley, C. W., Laude, N. D., Parent, K. L., and Heien, M. L. (2013). Fast-scan controlled-adsorption voltammetry for the quantification of absolute concentrations and adsorption dynamics. Langmuir 29, 14885–14892. doi: 10.1021/la402686s
Avestruz, A.-T., Santa, W., Carlson, D., Jensen, R., Stanslaski, S., Helfenstine, A., et al. (2008). A 5 uW/Channel spectral analysis IC for chronic bidirectional brain-machine interfaces. IEEE J. Solid-State Circuits 43, 3006–3024. doi: 10.1109/JSSC.2008.2006460
Bai, Q., and Wise, K. D. (2001). Single-unit neural recording with active microelectrode arrays. IEEE Trans. Biomed. Eng. 48, 911–920. doi: 10.1109/10.936367
Basu, I., Graupe, D., Tuninetti, D., Shukla, P., Slavin, K. V., Metman, L. V., et al. (2013). Pathological tremor prediction using surface electromyogram and acceleration: potential use in ON-OFF demand driven deep brain stimulator design. J. Neural Eng. 10:036019. doi: 10.1088/1741-2560/10/3/036019
Behrend, C. E., Cassim, S. M., and Pallone, M. J. (2009). Toward feedback controlled deep brain stimulation: dynamics of glutamate release in the subthalamic nucleus in rats. J. Neurosci. Methods 180, 278–289. doi: 10.1016/j.jneumeth.2009.04.001
Benabid, A.-L., Chabardès, S., and Seigneuret, E. (2005). Deep-brain stimulation in Parkinson's disease: long-term efficacy and safety - What happened this year? Curr. Opin. Neurol. 18, 623–630. doi: 10.1097/01.wco.0000186839.53807.93
Benabid, A.-L., Pollak, P., Gervason, C., Hoffmann, D., Gao, D. M., Hommel, M., et al. (1991). Long-term suppression of tremor by chronic stimulation of the ventral intermediate thalamic nucleus. Lancet 337, 403–406. doi: 10.1016/0140-6736(91)91175-T
Benabid, A.-L., Pollak, P., Louveau, A., Henry, S., and de Rougemont, J. (1987). Combined (thalamotomy and stimulation) stereotactic surgery of the VIM thalamic nucleus for bilateral Parkinson disease. Appl. Neurophysiol. 50, 344–346.
Benazzouz, A., Gao, D. M., Ni, Z. G., Piallat, B., Bouali-Benazzouz, R., and Benabid, A.-L. (2000). Effect of high-frequency stimulation of the subthalamic nucleus on the neuronal activities of the substantia nigra pars reticulata and ventrolateral nucleus of the thalamus in the rat. Neuroscience 99, 289–295. doi: 10.1016/S0306-4522(00)00199-8
Berényi, A., Belluscio, M., Mao, D., and Buzsáki, G. (2012). Closed-loop control of epilepsy by transcranial electrical stimulation. Science 337, 735–737. doi: 10.1126/science.1223154
Blaha, C. D., Coury, A., Fibiger, H. C., and Phillips, A. G. (1990). Effects of neurotensin on dopamine release and metabolism in the rat striatum and nucleus accumbens: cross-validation using in vivo voltammetry and microdialysis. Neuroscience 34, 699–705. doi: 10.1016/0306-4522(90)90176-5
Blaha, C. D., and Phillips, A. G. (1996). A critical assessment of electrochemical procedures applied to the measurement of dopamine and its metabolites during drug-induced and species-typical behaviours. Behav. Pharmacol. 7, 675–708. doi: 10.1097/00008877-199611000-00014
Bledsoe, J. M., Kimble, C. J., Covey, D. P., Blaha, C. D., Agnesi, F., Mohseni, P., et al. (2009). Development of the wireless instantaneous neurotransmitter concentration system for intraoperative neurochemical monitoring using fast-scan cyclic voltammetry. J. Neurosurg. 111, 712–723. doi: 10.3171/2009.3.JNS081348
Blomstedt, P., and Hariz, M. I. (2010). Deep brain stimulation for movement disorders before DBS for movement disorders. Parkinsonism Relat. Disord. 16, 429–433. doi: 10.1016/j.parkreldis.2010.04.005
Bronstein, J. M., Tagliati, M., Alterman, R. L., Lozano, A. M., Volkmann, J., Stefani, A., et al. (2011). Deep brain stimulation for Parkinson disease: an expert consensus and review of key issues. Arch. Neurol. 68, 165. doi: 10.1001/archneurol.2010.260
Bronte-Stewart, H., Barberini, C., Koop, M. M., Hill, B. C., Henderson, J. M., and Wingeier, B. (2009). The STN beta-band profile in Parkinson's disease is stationary and shows prolonged attenuation after deep brain stimulation. Exp. Neurol. 215, 20–28. doi: 10.1016/j.expneurol.2008.09.008
Burchiel, K. J., Anderson, V. C., Favre, J., and Hammerstad, J. P. (1999). Comparison of pallidal and subthalamic nucleus deep brain stimulation for advanced Parkinson's disease: results of a randomized, blinded pilot study. Neurosurgery 45, 1375–1382. discussion: 1382–1384.
Buzsáki, G., Anastassiou, C. A., and Koch, C. (2012). The origin of extracellular fields and currents—EEG, ECoG, LFP and spikes. Nat. Rev. Neurosci. 13, 407–420. doi: 10.1038/nrn3241
Buzsáki, G., Leung, L. W., and Vanderwolf, C. H. (1983). Cellular bases of hippocampal EEG in the behaving rat. Brain Res. 287, 139–171. doi: 10.1016/0165-0173(83)90037-1
Carter, R. R., and Houk, J. C. (1993). Multiple single-unit recordings from the CNS using thin-film electrode arrays. IEEE Trans. Rehab. Eng. 1, 175–184. doi: 10.1109/86.279266
Chang, S.-Y., Kim, I., Marsh, M. P., Jang, D. P., Hwang, S.-C., van Gompel, J. J., et al. (2012). Wireless fast-scan cyclic voltammetry to monitor adenosine in patients with essential tremor during deep brain stimulation. Mayo Clin. Proc. 87, 760–765. doi: 10.1016/j.mayocp.2012.05.006
Chang, S.-Y., Kimble, C. J., Kim, I., Paek, S. B., Kressin, K. R., Boesche, J. B., et al. (2013). Development of the mayo investigational neuromodulation control system: toward a closed-loop electrochemical feedback system for deep brain stimulation. J. Neurosurg. 119, 1556–1565. doi: 10.3171/2013.8.JNS122142
Chang, S. Y., Shon, Y. M., and Agnesi, F. (2009). “Microthalamotomy effect during deep brain stimulation: potential involvement of adenosine and glutam,” in 31st Annual International Conference of the IEEE EMBS (Minneapolis, MN).
Chefer, V. I., Thompson, A. C., Zapata, A., and Shippenberg, T. S. (2009). Overview of brain microdialysis. Curr. Protoc. Neurosci. 47, 1–28. doi: 10.1002/0471142301.ns0701s47
Cheung, K. C. (2007). Implantable microscale neural interfaces. Biomed. Microdevices 9, 923–938. doi: 10.1007/s10544-006-9045-z
Dale, N., Hatz, S., Tian, F., and Llaudet, E. (2005). Listening to the brain: microelectrode biosensors for neurochemicals. Trends Biotechnol. 23, 420–428. doi: 10.1016/j.tibtech.2005.05.010
Denys, D., Mantione, M., Figee, M., van den Munckhof, P., Koerselman, F., Westenberg, H., et al. (2010). Deep brain stimulation of the nucleus accumbens for treatment-refractory obsessive-compulsive disorderobsessive-compulsive disorder. Arch. Gen. Psychiatry 67, 1061–1068. doi: 10.1001/archgenpsychiatry.2010.122
Deuschl, G., Herzog, J., Kleiner-Fisman, G., Kubu, C., Lozano, A. M., Lyons, K. E., et al. (2006). Deep brain stimulation: postoperative issues. Mov. Disord. 21, S219–S237. doi: 10.1002/mds.20957
Fagg, A. H., Hatsopoulos, N. G., de Lafuente, V., Moxon, K. A., Nemati, S., Rebesco, J. M., et al. (2007). Biomimetic brain machine interfaces for the control of movement. J. Neurosci. 27, 11842–11846. doi: 10.1523/JNEUROSCI.3516-07.2007
Fisher, R., Salanova, V., Witt, T., Worth, R., Henry, T., Gross, R., et al. (2010). Electrical stimulation of the anterior nucleus of thalamus for treatment of refractory epilepsy. Epilepsia 51, 899–908. doi: 10.1111/j.1528-1167.2010.02536.x
Fitzgerald, P. J. (2014). Is elevated norepinephrine an etiological factor in some cases of Parkinson's disease? Med. Hypotheses 82, 462–469. doi: 10.1016/j.mehy.2014.01.026
Foltynie, T., and Hariz, M. I. (2010). Surgical management of Parkinson's disease. Expert Rev. Neurother. 10, 903–914. doi: 10.1586/ern.10.68
Fraix, V., Houeto, J.-L., Lagrange, C., Le Pen, C., Krystkowiak, P., Guehl, D., et al. (2006). Clinical and economic results of bilateral subthalamic nucleus stimulation in Parkinson's disease. J. Neurol. Neurosurg. Psychiatry 77, 443–449. doi: 10.1136/jnnp.2005.077677
Frankemolle, A. M. M., Wu, J., Noecker, A. M., Voelcker-Rehage, C., Ho, J. C., Vitek, J. L., et al. (2010). Reversing cognitive-motor impairments in Parkinson's disease patients using a computational modelling approach to deep brain stimulation programming. Brain 133, 746–761. doi: 10.1093/brain/awp315
Gale, J. T., Lee, K. H., Amirnovin, R., Roberts, D. W., Williams, Z. M., Blaha, C. D., et al. (2013). Electrical stimulation-evoked dopamine release in the primate striatum. Stereotact. Funct. Neurosurg. 91, 355–363. doi: 10.1159/000351523
Germano, I. M., Gracies, J.-M., Weisz, D. J., Tse, W., Koller, W. C., and Olanow, C. W. (2004). Unilateral stimulation of the subthalamic nucleus in Parkinson disease: a double-blind 12-month evaluation study. J. Neurosurg. 101, 36–42. doi: 10.3171/jns.2004.101.1.0036
Giannicola, G., Rosa, M., Servello, D., Menghetti, C., Carrabba, G., Pacchetti, C., Zangaglia, R., Cogiamanian, F., Scelzo, E., Marceglia, S., et al. (2012). Subthalamic local field potentials after seven-year deep brain stimulation in Parkinson's disease. Experimental Neurology 237, 312–317. doi: 10.1016/j.expneurol.2012.06.012
Gildenberg, P. L. (2003). History repeats itself. Stereotact Funct Neurosurg 80, 61–75. doi: 10.1159/000075162
Gildenberg, P. L. (2005). Evolution of neuromodulation. Stereotact Funct Neurosurg 83, 71–79. doi: 10.1159/000086865
Grant, P. F., and Lowery, M. M. (2013). Simulation of cortico-basal ganglia oscillations and their suppression by closed loop deep brain stimulation. IEEE Trans. Neural Syst. Rehabil. Eng. 21, 584–594. doi: 10.1109/TNSRE.2012.2202403
Griessenauer, C. J., Chang, S.-Y., Tye, S. J., Kimble, C. J., Bennet, K. E., Garris, P. A., et al. (2010). Wireless instantaneous neurotransmitter concentration system: electrochemical monitoring of serotonin using fast-scan cyclic voltammetry-a proof-of-principle study. J. Neurosurg. 113, 656–665. doi: 10.3171/2010.3.JNS091627
Grill, W. M., Snyder, A. N., and Miocinovic, S. (2004). Deep brain stimulation creates an informational lesion of the stimulated nucleus. Neuroreport 15, 1137–1140. doi: 10.1097/00001756-200405190-00011
Hamani, C., Neimat, J., and Lozano, A. M. (2006). Deep brain stimulation for the treatment of Parkinson's disease. J. Neural Transm. Suppl. 70, 393–399. doi: 10.1007/978-3-211-45295-0_59
Hashimoto, T., Elder, C. M., Okun, M. S., Patrick, S. K., and Vitek, J. L. (2003). Stimulation of the subthalamic nucleus changes the firing pattern of pallidal neurons. J. Neurosci. 23, 1916–1923.
Hunka, K., Suchowersky, O., Wood, S., Derwent, L., and Kiss, Z. H. T. (2005). Nursing time to program and assess deep brain stimulators in movement disorder patients. J. Neurosci. Nurs. 37, 204–210. doi: 10.1097/01376517-200508000-00006
Issa, E. B., and Wang, X. (2013). Increased neural correlations in primate auditory cortex during slow-wave sleep. J. Neurophysiol. 109, 2732–2738. doi: 10.1152/jn.00695.2012
Jacobs, C. B., Peairs, M. J., and Venton, B. J. (2010). Review: carbon nanotube based electrochemical sensors for biomolecules. Anal. Chim. Acta. 662, 105–127. doi: 10.1016/j.aca.2010.01.009
Johnson, M., Franklin, R., Scott, K. A., Brown, R., and Kipke, D. (2005). Neural probes for concurrent detection of neurochemical and electrophysiological signals in vivo. Conf. Proc. IEEE Eng. Med. Biol. Soc. 7, 7325–7328. doi: 10.1109/IEMBS.2005.1616203
Johnson, M. D., Miocinovic, S., McIntyre, C. C., and Vitek, J. L. (2008). Mechanisms and targets of deep brain stimulation in movement disorders. Neurotherapeutics 5, 294–308. doi: 10.1016/j.nurt.2008.01.010
Khan, G. M., Smolders, I., Lindekens, H., Manil, J., Ebinger, G., and Michotte, Y. (1999). Effects of diazepam on extracellular brain neurotransmitters in pilocarpine-induced seizures in rats. Eur. J. Pharmacol. 373, 153–161. doi: 10.1016/S0014-2999(99)00209-5
Kimble, C. J., Johnson, D. M., Winter, B., Whitlock, S. V., Kressin, K. R., Horne, A. E., et al. (2009). “Wireless Instantaneous Neurotransmitter Concentration Sensing System (WINCS) for intraoperative neurochemical monitoring,” in 31st Annual International Conference of the IEEE EMBS, 1–4. Available online at: http://ieeexplore.ieee.org/xpls/abs_all.jsp?arnumber=5332773
Kita, H., Tachibana, Y., Nambu, A., and Chiken, S. (2005). Balance of monosynaptic excitatory and disynaptic inhibitory responses of the globus pallidus induced after stimulation of the subthalamic nucleus in the monkey. J. Neurosci. 25, 8611–8619. doi: 10.1523/JNEUROSCI.1719-05.2005
Kluger, B. M., Foote, K. D., Jacobson, C. E., and Okun, M. S. (2011). Lessons learned from a large single center cohort of patients referred for DBS management. Parkinsonism Relat. Disord. 17, 236–239. doi: 10.1016/j.parkreldis.2010.05.003
Koller, W. C., Lyons, K. E., Wilkinson, S. B., and Pahwa, R. (2001). Efficacy of unilateral deep brain stimulation of the vim nucleus of the thalamus for essential head tremor. Mov. Disord. 14, 847–850. doi: 10.1002/1531-8257(199909)14:5%3C847::AID-MDS1021%3E3.0.CO;2-G
Kupsch, A., Tagliati, M., Vidailhet, M., Aziz, T., Krack, P., Moro, E., et al. (2011). Early postoperative management of DBS in dystonia: programming, response to stimulation, adverse events, medication changes, evaluations, and troubleshooting. Mov. Disord. 26 (Suppl. 1), S37–S53. doi: 10.1002/mds.23624
Lee, K. H., Blaha, C. D., Garris, P. A., Mohseni, P., Horne, A. E., Bennet, K. E., et al. (2009). Evolution of deep brain stimulation: human electrometer and smart devices supporting the next generation of therapy. Neuromodulation 12, 85–103. doi: 10.1111/j.1525-1403.2009.00199.x
Lee, K. H., Blaha, C. D., Harris, B. T., Cooper, S., Hitti, F. L., Leiter, J. C., et al. (2006). Dopamine efflux in the rat striatum evoked by electrical stimulation of the subthalamic nucleus: potential mechanism of action in Parkinson's disease. Eur. J. Neurosci. 23, 1005–1014. doi: 10.1111/j.1460-9568.2006.04638.x
Lee, K. H., Chang, S.-Y., Jang, D. P., Kim, I., Goerss, S., van Gompel, J., et al. (2011). Emerging techniques for elucidating mechanism of action of deep brain stimulation. Conf. Proc. IEEE Eng. Med. Biol. Soc. 2011, 677–680. doi: 10.1109/IEMBS.2011.6090152
Lee, K. H., Chang, S.-Y., Roberts, D. W., and Kim, U. (2004). Neurotransmitter release from high-frequency stimulation of the subthalamic nucleus. J. Neurosurg. 101, 511–517. doi: 10.3171/jns.2004.101.3.0511
Lee, K. H., Kristic, K., van Hoff, R., Hitti, F. L., Blaha, C., Harris, B., et al. (2007). High-frequency stimulation of the subthalamic nucleus increases glutamate in the subthalamic nucleus of rats as demonstrated by in vivo enzyme-linked glutamate sensor. Brain Res. 1162, 121–129. doi: 10.1016/j.brainres.2007.06.021
Lim, S.-N., Lee, S.-T., Tsai, Y.-T., Chen, I.-A., Tu, P.-H., Chen, J.-L., et al. (2007). Electrical stimulation of the anterior nucleus of the thalamus for intractable epilepsy: a long-term follow-up study. Epilepsia 48, 342–347. doi: 10.1111/j.1528-1167.2006.00898.x
Little, S., Pogosyan, A., Neal, S., Zavala, B., Zrinzo, L., Hariz, M., et al. (2013). Adaptive deep brain stimulation in advanced Parkinson disease. Ann. Neurol. 74, 449–457. doi: 10.1002/ana.23951
Loffler, S. (2012). Towards Closed Loop Deep Brain Stimulation: An Integrated Approach for Neural Recording and Microstimulation. 1–235.
Logothetis, N. K. (2003a). MR imaging in the non-human primate: studies of function and of dynamic connectivity. Curr. Opin. Neurobiol. 13, 630–642. doi: 10.1016/j.conb.2003.09.017
Logothetis, N. K. (2003b). The underpinnings of the BOLD functional magnetic resonance imaging signal. J. Neurosci. 23, 3963–3971.
Lujan, J. L., and Crago, P. E. (2009). Automated optimal coordination of multiple-DOF neuromuscular actions in feedforward neuroprostheses. IEEE Trans. Biomed. Eng. 56, 179–187. doi: 10.1109/TBME.2008.2002159
MacDonald, A. A., Monchi, O., Seergobin, K. N., Ganjavi, H., Tamjeedi, R., and MacDonald, P. A. (2013). Parkinson's disease duration determines effect of dopaminergic therapy on ventral striatum function. Mov. Disord. 28, 153–160. doi: 10.1002/mds.25152
Mattia, M., Ferraina, S., and del Giudice, P. (2010). Dissociated multi-unit activity and local field potentials: a theory inspired analysis of a motor decision task. Neuroimage 52, 812–823. doi: 10.1016/j.neuroimage.2010.01.063
Maurice, N., Thierry, A.-M., Glowinski, J., and Deniau, J.-M. (2003). Spontaneous and evoked activity of substantia nigra pars reticulata neurons during high-frequency stimulation of the subthalamic nucleus. J. Neurosci. 23, 9929–9936.
Mayberg, H. S., Brannan, S. K., Tekell, J. L., Silva, J. A., Mahurin, R. K., McGinnis, S., et al. (2000). Regional metabolic effects of fluoxetine in major depression: serial changes and relationship to clinical response. Biol. Psychiatry 48, 830–843. doi: 10.1016/S0006-3223(00)01036-2
Mayberg, H. S., Lozano, A. M., Voon, V., McNeely, H. E., Seminowicz, D., Hamani, C., et al. (2005). Deep brain stimulation for treatment-resistant depression. Neuron 45, 651–660. doi: 10.1016/j.neuron.2005.02.014
Maynard, E. M., Nordhausen, C. T., and Normann, R. A. (1997). The Utah intracortical Electrode Array: a recording structure for potential brain-computer interfaces. Electroencephalogr. Clin. Neurophysiol. 102, 228–239. doi: 10.1016/S0013-4694(96)95176-0
McIntyre, C. C., and Hahn, P. J. (2010). Network perspectives on the mechanisms of deep brain stimulation. Neurobiol. Dis. 38, 329–337. doi: 10.1016/j.nbd.2009.09.022
McIntyre, C. C., Savasta, M., Kerkerian-Le Goff, L., and Vitek, J. L. (2004a). Uncovering the mechanism(s) of action of deep brain stimulation: activation, inhibition, or both. Clin. Neurophysiol. 115, 1239–1248. doi: 10.1016/j.clinph.2003.12.024
McIntyre, C. C., Savasta, M., Walter, B. L., and Vitek, J. L. (2004b). How does deep brain stimulation work? Present understanding and future questions. J. Clin. Neurophysiol. 21, 40–50. doi: 10.1097/00004691-200401000-00006
Min, B., Guoming, L., and Jian, Z. (2013). Treatment of mesial temporal lobe epilepsy with amygdalohippocampal stimulation: a case series and review of the literature. Exp. Ther. Med. 5, 1264–1268. doi: 10.3892/etm.2013.968
Miocinovic, S., Parent, M., Butson, C. R., Hahn, P. J., Russo, G. S., Vitek, J. L., et al. (2006). Computational analysis of subthalamic nucleus and lenticular fasciculus activation during therapeutic deep brain stimulation. J. Neurophysiol. 96, 1569–1580. doi: 10.1152/jn.00305.2006
Miocinovic, S., Somayajula, S., Chitnis, S., and Vitek, J. L. (2013). History, applications, and mechanisms of deep brain stimulation. JAMA Neurol. 70, 163–171. doi: 10.1001/2013.jamaneurol.45
Molinuevo, J. L., Valldeoriola, F., Tolosa, E., Rumia, J., Valls-Sole, J., Roldan, H., et al. (2000). Levodopa withdrawal after bilateral subthalamic nucleus stimulation in advanced Parkinson disease. Arch. Neurol. 57, 983–988. doi: 10.1001/archneur.57.7.983
Morishita, T., Fayad, S. M., Goodman, W. K., Foote, K. D., Chen, D., Peace, D. A., et al. (2013). Surgical neuroanatomy and programming in deep brain stimulation for obsessive compulsive disorder. Neuromodulation. doi: 10.1111/ner.12141. [Epub ahead of print].
Moro, E., Lozano, A. M., Pollak, P., Agid, Y., Rehncrona, S., Volkmann, J., et al. (2010). Long-term results of a multicenter study on subthalamic and pallidal stimulation in Parkinson's disease. Mov. Disord. 25, 578–586. doi: 10.1002/mds.22735
Moro, E., Poon, Y.-Y. W., Lozano, A. M., Saint-Cyr, J. A., and Lang, A. E. (2006). Subthalamic nucleus stimulation: improvements in outcome with reprogramming. Arch. Neurol. 63, 1266–1272. doi: 10.1001/archneur.63.9.1266
Moro, E., Scerrati, M., Romito, L. M., Roselli, R., Tonali, P., and Albanese, A. (1999). Chronic subthalamic nucleus stimulation reduces medication requirements in Parkinson's disease. Neurology 53, 85–90. doi: 10.1212/WNL.53.1.85
Mueller, J., Skogseid, I. M., and Benecke, R. (2008). Pallidal deep brain stimulation improves quality of life in segmental and generalized dystonia: results from a prospective, randomized sham-controlled trial. Mov. Disord. 23, 131–134. doi: 10.1002/mds.21783
Mure, H., Hirano, S., Tang, C. C., Isaias, I. U., Antonini, A., Ma, Y., et al. (2011). Parkinson's disease tremor-related metabolic network: characterization, progression, and treatment effects. Neuroimage 54, 1244–1253. doi: 10.1016/j.neuroimage.2010.09.028
Nemeroff, C. B. (2007). The burden of severe depression: a review of diagnostic challenges and treatment alternatives. J. Psychiatr. Res. 41, 189–206. doi: 10.1016/j.jpsychires.2006.05.008
Obeso, J. A., and Guridi, J. (2001). Deep-brain stimulation of the subthalamic nucleus or the pars interna of the globus pallidus in Parkinson's Disease. N. Engl. J. Med. 345, 956–963. doi: 10.1056/NEJMoa000827
Okun, M. S., Tagliati, M., Pourfar, M., Fernandez, H. H., Rodriguez, R. L., Alterman, R. L., et al. (2005). Management of referred deep brain stimulation failuresa retrospective analysis from 2 movement disorders centers. Arch. Neurol. 62, 1250–1255. doi: 10.1001/archneur.62.8.noc40425
Olanow, W., Schapira, A. H., and Rascol, O. (2000). Continuous dopamine-receptor stimulation in early Parkinson's disease. Trends Neurosci. 23, S117–S126. doi: 10.1016/S1471-1931(00)00030-6
Oldenziel, W. H., Beukema, W., and Westerink, B. H. C. (2004). Improving the reproducibility of hydrogel-coated glutamate microsensors by using an automated dipcoater. J. Neurosci. Methods 140, 117–126. doi: 10.1016/j.jneumeth.2004.04.038
Polikov, V. S., Tresco, P. A., and Reichert, W. M. (2005). Response of brain tissue to chronically implanted neural electrodes. J. Neurosci. Methods 148, 1–18. doi: 10.1016/j.jneumeth.2005.08.015
Priori, A., Foffani, G., Rossi, L., and Marceglia, S. (2013). Experimental neurology. Exp. Neurol. 245, 77–86. doi: 10.1016/j.expneurol.2012.09.013
Ramasubbu, R., Anderson, S., Haffenden, A., Chavda, S., and Kiss, Z. H. (2013). Double-blind optimization of subcallosal cingulate deep brain stimulation for treatment-resistant depression: a pilot study. J. Psychiatry Neurosci. 38, 325–332. doi: 10.1503/jpn.120160
Rehncrona, S., Johnels, B., and Widner, H. (2003). Long-term efficacy of thalamic deep brain stimulation for tremor: double-blind assessments. Mov. Disord. 18, 163–170. doi: 10.1002/mds.10309
Ricchi, V., Zibetti, M., Angrisano, S., Merola, A., Arduino, N., Artusi, C. A., et al. (2012). Transient effects of 80 Hz stimulation on gait in STN DBS treated PD patients: a 15 months follow-up study. Brain Stimul. 5, 388–392. doi: 10.1016/j.brs.2011.07.001
Robinson, D. L., Venton, B. J., Heien, M. L. A. V., and Wightman, R. M. (2003). Detecting subsecond dopamine release with fast-scan cyclic voltammetry in vivo. Clin. Chem. 49, 1763–1773. doi: 10.1373/49.10.1763
Rodriguez-Oroz, M. C. (2004). Efficacy of deep brain stimulation of the subthalamic nucleus in Parkinson's disease 4 years after surgery: double blind and open label evaluation. J. Neurol. Neurosurg. Psychiatry 75, 1382–1385. doi: 10.1136/jnnp.2003.031294
Roham, M., Halpern, J. M., Martin, H. B., Chiel, H. J., and Mohseni, P. (2007). “Diamond microelectrodes and CMOS microelectronics for wireless transmission of fast-scan cyclic voltammetry,” in Engineering in Medicine and Biology Society, 2007. EMBS 2007. 29th Annual International Conference of the IEEE, 6044–6047. doi: 10.1109/IEMBS.2007.4353726
Rosa, M., Giannicola, G., Marceglia, S., Fumagalli, M., Barbieri, S., and Priori, A. (2012). “Neurophysiology of deep brain stimulation: emerging horizons in neuromodulation—new frontiers in brain and spine stimulation,” in International Review of Neurobiology, Vol. 107 (Philadelphia, PA), 23–55. doi: 10.1016/B978-0-12-404706-8.00004-8
Rosin, B., Slovik, M., Mitelman, R., Rivlin-Etzion, M., Haber, S. N., Israel, Z., et al. (2011). Closed-loop deep brain stimulationis superior in ameliorating parkinsonism. Neuron 72, 370–384. doi: 10.1016/j.neuron.2011.08.023
Rossi, L., Foffani, G., Marceglia, S., Bracchi, F., Barbieri, S., and Priori, A. (2007). An electronic device for artefact suppression in human local field potential recordings during deep brain stimulation. J. Neural Eng. 4, 96–106. doi: 10.1088/1741-2560/4/2/010
Santaniello, S., Fiengo, G., Glielmo, L., and Grill, W. M. (2011). Closed-loop control of deep brain stimulation: a simulation study. IEEE Trans. Neural Syst. Rehabil. Eng. 19, 15–24. doi: 10.1109/TNSRE.2010.2081377
Shah, R. S., Chang, S.-Y., Min, H.-K., Cho, Z.-H., Blaha, C. D., and Lee, K. H. (2010). Deep brain stimulation: technology at the cutting edge. J. Clin. Neurol. 6, 167–182. doi: 10.3988/jcn.2010.6.4.167
Shon, Y.-M., Chang, S.-Y., Tye, S. J., Kimble, C. J., Bennet, K. E., Blaha, C. D., et al. (2010a). Comonitoring of adenosine and dopamine using the wireless instantaneous neurotransmitter concentration system: proof of principle. J. Neurosurg. 112, 539–548. doi: 10.3171/2009.7.JNS09787
Shon, Y.-M., Lee, K. H., Goerss, S. J., Kim, I. Y., Kimble, C., van Gompel, J. J., et al. (2010b). High frequency stimulation of the subthalamic nucleus evokes striatal dopamine release in a large animal model of human DBS neurosurgery. Neurosci. Lett. 475, 136–140. doi: 10.1016/j.neulet.2010.03.060
Simuni, T., Jaggi, J. L., Mulholland, H., Hurtig, H. I., Colcher, A., Siderowf, A. D., et al. (2002). Bilateral stimulation of the subthalamic nucleus in patients with Parkinson disease: a study of efficacy and safety. J. Neurosurg. 96, 666–672. doi: 10.3171/jns.2002.96.4.0666
Skarpaas, T. L., and Morrell, M. J. (2009). Intracranial stimulation therapy for epilepsy. Neurotherapeutics 6, 238–243. doi: 10.1016/j.nurt.2009.01.022
Smith, I. D., and Grace, A. A. (1992). Role of the subthalamic nucleus in the regulation of nigral dopamine neuron activity. Synapse 12, 287–303. doi: 10.1002/syn.890120406
Smolders, I., van Belle, K., Ebinger, G., and Michotte, Y. (1997). Hippocampal and cerebellar extracellular amino acids during pilocarpine-induced seizures in freely moving rats. Eur. J. Pharmacol. 319, 21–29. doi: 10.1016/S0014-2999(96)00830-8
Speert, D., Bentsen, T., and Fenichel, M. (eds.). (2012). Brain Facts. 7th Edn. Washington, DC: Society for Neuroscience.
Takmakov, P., Zachek, M. K., Keithley, R. B., Walsh, P. L., Donley, C., McCarty, G. S., et al. (2010). Carbon microelectrodes with a renewable surface. Anal. Chem. 82, 2020–2028. doi: 10.1021/ac902753x
Tsang, E. W., Hamani, C., Moro, E., Mazzella, F., Saha, U., Lozano, A. M., et al. (2012). Subthalamic deep brain stimulation at individualized frequencies for Parkinson disease. Neurology 78, 1930–1938. doi: 10.1212/WNL.0b013e318259e183
Tye, S. J., Miller, A. D., and Blaha, C. D. (2013). Ventral tegmental ionotropic glutamate receptor stimulation of nucleus accumbens tonic dopamine efflux blunts hindbrain-evoked phasic neurotransmission: implications for dopamine dysregulation disorders. Neuroscience 252, 337–345. doi: 10.1016/j.neuroscience.2013.08.010
van Gompel, J. J., Chang, S.-Y., Goerss, S. J., Kim, I. Y., Kimble, C., Bennet, K. E., et al. (2010). Development of intraoperative electrochemical detection: wireless instantaneous neurochemical concentration sensor for deep brain stimulation feedback. Neurosurg. Focus 29, E6. doi: 10.3171/2010.5.FOCUS10110
Velasco, F., Carrillo-Ruiz, J. D., Brito, F., Velasco, M., Velasco, A. L., Marquez, I., et al. (2005). Double-blind, randomized controlled pilot study of bilateral cerebellar stimulation for treatment of intractable motor seizures. Epilepsia 46, 1071–1081 doi: 10.1111/j.1528-1167.2005.70504.x
Velasco, F., Velasco, A. L., Velasco, M., Jiménez, F., Carrillo-Ruiz, J. D., and Castro, G. (2007). Deep brain stimulation for treatment of the epilepsies: the centromedian thalamic target. Acta Neurochir. Suppl. 97, 337–342. doi: 10.1007/978-3-211-33081-4_38
Vitek, J. L., Zhang, J., Hashimoto, T., Russo, G. S., and Baker, K. B. (2012). External pallidal stimulation improves parkinsonian motor signs and modulates neuronal activity throughout the basal ganglia thalamic network. Exp. Neurol. 233, 581–586. doi: 10.1016/j.expneurol.2011.09.031
Watson, C. J., Venton, B. J., and Kennedy, R. T. (2006). In vivo measurements of neurotransmitters by microdialysis sampling. Anal. Chem. 78, 1391–1399. doi: 10.1021/ac0693722
Weiss, D., Walach, M., Meisner, C., Fritz, M., Scholten, M., Breit, S., et al. (2013). Nigral stimulation for resistant axial motor impairment in Parkinson's disease? A randomized controlled trial. Brain 136, 2098–2108. doi: 10.1093/brain/awt122
Wightman, R. M. (2006). Detection technologies. Probing cellular chemistry in biological systems with microelectrodes. Science 311, 1570–1574. doi: 10.1126/science.1120027
Keywords: deep brain stimulation (DBS), feedback control systems, local field potentials (LFP), fast scan cyclic voltammetry (FSCV), machine learning, individualized medicine
Citation: Grahn PJ, Mallory GW, Khurram OU, Berry BM, Hachmann JT, Bieber AJ, Bennet KE, Min H-K, Chang S-Y, Lee KH and Lujan JL (2014) A neurochemical closed-loop controller for deep brain stimulation: toward individualized smart neuromodulation therapies. Front. Neurosci. 8:169. doi: 10.3389/fnins.2014.00169
Received: 04 April 2014; Accepted: 02 June 2014;
Published online: 25 June 2014.
Edited by:
Mitsuhiro Hayashibe, University of Montpellier, FranceReviewed by:
Matthew Johnson, University of Minnesota, USAChristian J. Hartmann, Heinrich Heine University Duesseldorf, Germany
Copyright © 2014 Grahn, Mallory, Khurram, Berry, Hachmann, Bieber, Bennet, Min, Chang, Lee and Lujan. This is an open-access article distributed under the terms of the Creative Commons Attribution License (CC BY). The use, distribution or reproduction in other forums is permitted, provided the original author(s) or licensor are credited and that the original publication in this journal is cited, in accordance with accepted academic practice. No use, distribution or reproduction is permitted which does not comply with these terms.
*Correspondence: J. L. Lujan, Departments of Neurologic Surgery and Physiology and Biomedical Engineering, Mayo Clinic, 200 First Street SW, Rochester, MN 55905, USA e-mail: lujan.luis@mayo.edu