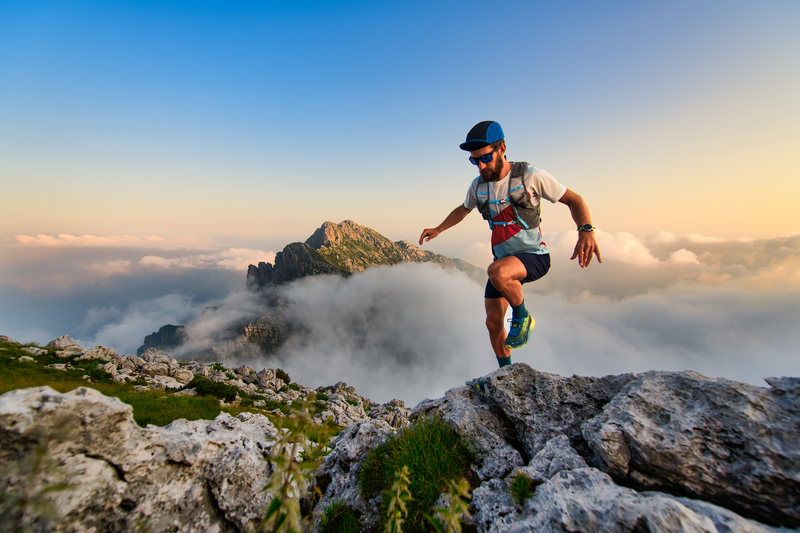
94% of researchers rate our articles as excellent or good
Learn more about the work of our research integrity team to safeguard the quality of each article we publish.
Find out more
ORIGINAL RESEARCH article
Front. Mar. Sci. , 11 December 2018
Sec. Aquatic Physiology
Volume 5 - 2018 | https://doi.org/10.3389/fmars.2018.00465
This article is part of the Research Topic Ecology and Behaviour of Free-Ranging Animals Studied by Advanced Data-Logging and Tracking Techniques View all 23 articles
Instrumenting animals with tags contributes additional resistive forces (weight, buoyancy, lift, and drag) that may result in increased energetic costs; however, additional metabolic expense can be moderated by adjusting behavior to maintain power output. We sought to increase hydrodynamic drag for near-surface swimming bottlenose dolphins, to investigate the metabolic effect of instrumentation. In this experiment, we investigate whether (1) metabolic rate increases systematically with hydrodynamic drag loading from tags of different sizes or (2) whether tagged individuals modulate speed, swimming distance, and/or fluking motions under increased drag loading. We detected no significant difference in oxygen consumption rates when four male dolphins performed a repeated swimming task, but measured swimming speeds that were 34% (>1 m s-1) slower in the highest drag condition. To further investigate this observed response, we incrementally decreased and then increased drag in six loading conditions. When drag was reduced, dolphins increased swimming speed (+1.4 m s-1; +45%) and fluking frequency (+0.28 Hz; +16%). As drag was increased, swimming speed (-0.96 m s-1; -23%) and fluking frequency (-14 Hz; 7%) decreased again. Results from computational fluid dynamics simulations indicate that the experimentally observed changes in swimming speed would have maintained the level of external drag forces experienced by the animals. Together, these results indicate that dolphins may adjust swimming speed to modulate the drag force opposing their motion during swimming, adapting their behavior to maintain a level of energy economy during locomotion.
Summary Statement: Biologging and tracking tags add drag to study subjects. When wearing tags of different sizes, dolphins changed their swimming paths, speed, and movements to modulate power output and energy consumption.
Tagging studies strive to collect novel data in an environment where observations are difficult; tags can measure animal movement as well as environmental and physiological variables to help interpret animal behavior or performance (Johnson et al., 2009; Crossin et al., 2014; Hussey et al., 2015). Tags do, however, contribute additional weight and bulk, and more relevant in the marine realm, perturb the hydrodynamics of highly streamlined animals. It is important to understand the impact of these devices not only on the tagged animal’s vital rates (e.g., Barron et al., 2010; van der Hoop et al., 2014; Best et al., 2015), but also on their behavior which is often assumed to be representative of the untagged population (Ropert-Coudert and Wilson, 2004; Vandenabeele et al., 2011; Broell et al., 2016).
The physiological and behavioral changes associated with handling, attachment, healing, or sedation that may be involved in the tagging procedure can be difficult to separate, especially for short-term studies (Elliott et al., 2012; Jepsen et al., 2015). During swimming, at a given speed, resistive forces (e.g., drag, weight or buoyancy) created by a tag will require additional work from the animal. Additional thrust must be produced to counter drag or more lift to counter the weight of the tag. Drag forces increase with speed-squared and therefore present a steep trade-off (Bannasch et al., 1994; Jones et al., 2013): animals can maintain speed under higher drag conditions but will need to expend more energy to overcome added drag. Diving vertebrates may have several compensatory mechanisms for this added drag; for example, changes in dive behavior (Webb et al., 1998) that do not result in detectable changes in energy requirements (Fahlman et al., 2008). Additionally, animals may compensate for added drag by reducing their speed to modulate power output (van der Hoop et al., 2014). Experimental protocols manipulating drag on swimmers have been effective in quantifying these trade-offs, effects, and compensation strategies (e.g., Webb, 1971a,b).
In a previous study (van der Hoop et al., 2014), bottlenose dolphins (Tursiops truncatus) changed their swimming behavior in response to drag loading from a bio-logging tag (DTAG2; Johnson and Tyack, 2003). Dolphins performing a repeated swimming task slowed down to the point where the tag yielded no increases in drag or power; in doing so, individuals’ energy consumption rates were no different. These results suggested that dolphins modify their behavior to adjust metabolic output and energy expenditure when faced with tag-induced drag. Other strategies exist to reduce the metabolic cost of these conditioned swimming tasks, e.g., changing movement paths between markers would enable individuals to reduce their total distance over time (Alexander, 2003; Ohashi et al., 2007). Building on our previous work, this research extends the experimental protocol monitoring swimming speeds and movement patterns to investigate the impact of multiple levels of drag loading to determine whether (1) metabolic rate increases systematically with greater levels of drag loading or (2) whether individuals employ strategies to adjust power output, such as changing speed, swimming distance or kinematics (fine-scale movements recorded on the tags).
We trained four male bottlenose dolphins (Table 1) with operant conditioning to complete two types of swimming tasks to investigate the effect of drag loading on individuals’ metabolic rates, swimming speeds, behaviors and kinematics. All experiments were by voluntary participation without restraint and the dolphin could refuse to participate or withdraw at any point during an experimental trial. Prior to initiating the study, we desensitized animals to the equipment and trained them for novel research-associated behaviors. All trials were conducted in a man-made lagoon (Figure 1C) approximately 38 m × 42 m, up to 3.5 m deep, at Dolphin Quest Oahu, Honolulu, HI, United States from 23 September–15 October 2013. Experiments were approved by the Woods Hole Oceanographic Institution Animal Care and Use Committee. A portion of the respirometry and energetics data were previously published elsewhere in a different context (Fahlman et al., 2016).
Figure 1. The experimental setup. Top (A) and frontal (B) views of the DTAG (blue) and additional elements that were attached to increase drag loading. Each element has approximately the same cross-sectional area as the tag alone. (C) Dolphins swam between waypoints in the lagoon (stars), and oxygen consumption was measured at the main dock station (yellow star). (D) A bottlenose dolphin equipped with the tag+8 configuration: four elements attached on each side of a DTAG (black). (E) Example of oxygen concentration (O2 %) measured with the respirometer over the duration of a metabolic trial, with 2-min “pre-exercise” and 1-min “post-exercise” and 10-min swim periods highlighted. Sudden drops in O2% reflect inhalations by the dolphin.
We defined drag loading as modifying an animal’s total hydrodynamic drag by perturbing the fluid flow around the animal. Drag loading was increased on the animals by attaching a suction cup biologging tag and additional urethane elements of equivalent frontal area (Drag Conditions, below). The first swimming task (Metabolic Trials, below) involved measuring individuals’ oxygen consumption before and after a continuous 10 min lap swim under three drag conditions (no tag, tag, tag+8; Drag Conditions, below). We did not measure oxygen consumption during the swimming task, but measured recovery rates following exercise as these kinetics can be used as an index for effort and energy stores (Respirometry, below; Royce, 1969; Di Prampero et al., 1970; Cohen-Solal et al., 1995). In the second swimming task (Incremental Loading Trials, below), two of the animals from the first study swam one lap at a time, as drag was adjusted incrementally through six drag conditions (no tag, tag, tag+2, tag+4, tag+6, tag+8) as swimming path and speed were measured by an aerial camera.
The animals performed the swimming tasks with and without the tag loading conditions. Swimming without a drag perturbation was used as the control condition for the experiment. During the tag loading conditions we used a DTAG (version 3; Johnson and Tyack, 2003; Shorter et al., 2013), a suction-cup attached biologging tag approximately 15 cm in length (Figure 1), to record fine-scale movement data from the animal. In addition to the ‘tag’ condition, we also systematically increased the drag loading on the animal by attaching additional urethane elements to either side of the DTAG with zip ties (Figures 1A,B,D). Each drag element had approximately the same cross-sectional area as the tag itself. We refer to the resulting increased drag-loading conditions by the number of elements added, e.g., the tag+4 condition includes the tag and an additional four elements and the tag+8 condition includes the tag and an additional eight elements (Figures 1A,B,D). For all tag loading conditions, we attached the tag to the back of the animal, between the blowhole and dorsal fin to replicate the location of tags placed on wild animals. Metabolic trials were conducted without the tag (control), and with the tag and tag+8 configurations; Incremental Loading trials included all symmetrical drag loading conditions.
In order to estimate the relative drag force between the tag conditions a set of computational fluid dynamics (CFD) simulations using commercial CFD code STAR-CCM+ (Siemens, Munich, Germany) were performed. In these simulations, the tag geometry was modeled on a semi-cylindrical body in a non-confined channel with fully developed flow. Velocity and turbulence quantities at the flow inlet were obtained by performing simulations for flow in an empty channel assuming periodicity at different velocities 1, 2, 3, and 4 m s-1. The Reynolds number, Re, ranged 1.6 × 106–6.6 × 106. Base cell sizes were selected in accordance with previously performed simulations on similar geometries (van der Hoop et al., 2014). The resulting mesh size varied between 20 and 25 million cells depending on the tag geometry. Simulations were performed using the standard k-𝜀 model (Jones and Launder, 1972) in two-layer formulation (Rodi, 1991). While there are limitations associated with the k-𝜀 turbulence model (Lloyd and Espanoles, 2002), this model results in a significant reduction of computational time, and examples in the literature have demonstrated that the k-𝜀 model turbulence mode provides accurate results for geometries and flow conditions comparable to those presented in this work (Sridhar et al., 2010; Kinaci et al., 2015). Further, we were interested in relative differences between the forces created by a range of tag geometries and not absolute estimates of force, a question that lends itself well to an efficient computational approach.
To measure dolphins’ respiratory flow and expired oxygen (O2) and carbon dioxide (CO2), we used a custom-made Fleisch type pneumotachometer and associated protocols as described in Fahlman et al. (2015). We estimated the O2 consumption rate (, mL O2 min-1) as previously described in Fahlman et al. (2015, 2016). We summed the total O2 volume for each breath during the before- and after-exercise periods, and then divided by the duration of those periods to calculate the average before and after exercise. We report as measured (ml O2 min-1) and as mass-specific (s, ml O2 kg-1 min-1) by dividing by the measured body mass of the individuals during the month of the study period (Table 1).
We calibrated the gas analyzers (ML206, Harvard Apparatus, sampling at 200 Hz) before and after the experiment using a commercial mixture of 5% O2, 5% CO2, and 90% N2 (blend accuracy 0.10%; Praxair, Inc., Danbury, CT, United States). We used ambient air to check the calibration before and after each experimental trial. We obtained hourly mean air temperature, relative humidity and atmospheric pressure measurements from the National Weather Service database for the times of the trials (National Weather Service, 2015). All gas volumes were converted to standard temperature and pressure for dry air (STPD, Quanjer et al., 1993). Exhaled air was assumed to be saturated at 37°C, and inhaled air volume was corrected for ambient temperature and relative humidity. CO2 was not removed from the air sample.
We used a specific experimental design to assess the metabolic changes associated with variation in drag, as follows. The dolphin was asked to remain neutrally buoyant at the water surface next to the trainer for at least 5 min immediately prior to exercise while the pneumotachometer was placed over the blow hole allowing the respiratory flow, gas content and metabolic rate to be determined (Figures 1C,E). The ‘pre-exercise’ metabolic rate was determined to be the average over the last 2 min of this period. By this time, the variability in the instantaneous had decreased. After the 10-min swimming task, the dolphin returned immediately to the measurement station, where we continuously measured the respiratory flow and gas composition for a minimum of 5 min. We defined the first 1 min of this period as the ‘post-exercise’ period over which we calculated the . We fitted an exponential function to 30 s averages of measured in the post-exercise period and characterized these kinetics by the recovery half-time (t1/2), defined as the time needed for to decrease from its peak value by half (Di Prampero et al., 1970).
To examine the metabolic impacts of increased drag, four dolphins were trained to swim around the lagoon clockwise with one of three drag conditions: control, Tag, or Tag+8. Four trainers, stationed at the same points for each trial (Figure 1C), directed the animal as it swam continuously between points for a minimum of 10 min (maximum = 10:33 min:sec). We did not control swimming speed or the total number of laps, but recorded swimming paths to estimate speed (see Aerial Video, below). We measured dolphins’ metabolic rate before and after this 10-min swimming task (see Respirometry, above), but animals breathed freely while swimming. None of the breaths during the swim were measured with the respirometer, but were counted from aerial video to calculate the breathing frequency (breaths min-1). We chose the drag condition for each trial at random for each individual; however, Dolphin 99L7 did not perform any trials with tag+8 due to earlier unsuccessful attempts to complete the task with high drag loading. A trial was terminated if the dolphin was not completing the task as directed, or if the animal chose to stop. Each dolphin performed only one swimming trial per day. All metabolic trials were completed 23 September–15 October 2013 between 8:37 and 11:18, when animals were pre-prandial.
To determine the effects of incremental changes in drag loading and unloading on swimming path, speed and kinematics, two bottlenose dolphins swam laps between the same waypoints as above (Figure 1C), but in the counter-clockwise direction. After each lap, the animal stopped and drag loading elements were either added or removed. For both animals, the first lap began with the tag+8 loading condition. After each lap, drag elements were removed: tag+8, tag+6, tag+4, tag+2, tag, control. Drag on the animal was then increased in the reverse order, adding two elements after each lap: control, tag, tag+2, tag+4, tag+6, tag+8. A total of 12 laps were completed by each animal. We measured the time it took the animals to complete each lap, and recorded the swimming paths of the dolphins with a camera mounted above the lagoon (see Aerial Video, below). The time between laps (for the resistance to be adjusted) was on average 2:19 (SD: 2:01, IDs 6JK5) and 2:45 min (SD: 2:26, ID 9FL3) with a range of 0:30–8:38 min overall. We did not measure metabolic rate during this second swimming task. We undertook trials between 12:00 and 15:05 on 14 October (ID 6JK5) and 15 October (ID 9FL3) 2013. Individuals had been fed before the trial, and were reinforced between laps. Approximately, 2 h elapsed between unloading and loading portions of the trial for both individuals.
We installed a wall-mounted GoPro camera (Hero3 5.0 MegaPixel) to record aerial video footage of the lagoon (Figure 1C) during the Metabolic and Incremental Loading trials. Calibration and undistortion of the GoPro video was performed using the OCamCalib Matlab toolbox1 (Scaramuzza et al., 2006; Rufli et al., 2008). Two separate calibration profiles were generated: one for the 16:9 aspect ratio for videos with frames of 1920 pixels by 1080 pixels, and another for the 4:3 aspect ratio for videos with frames of 1280 pixels by 960 pixels. The video data for all trials were undistorted using its applicable calibration profile and the undistortion tool in the toolbox.
Animal locations in the lagoon during the trials were digitized using Tracker (version 4.87; Brown, 2014) video tracking software. Collected track points were converted from the camera frame to the world frame using projective transforms. These transforms were generated by manually matching image pixel locations with known world coordinates of the lagoon, and computing the transformation matrix using the standard direct linear transformation (DLT) method. As the GoPro was not permanently mounted, each video required a separate transformation from image frame to world frame. Manually tracked points of the dolphins were converted to the world frame using these transformation matrices in homogeneous coordinate transformations. Average lap speed was calculated from the tracked camera data for 12 Metabolic trials (one for each animal × condition).
Data recorded by the inertial sensors on the DTAG (three-axis accelerometers and magnetometers) were processed with custom fluke-stroke detection algorithms (Johnson, 2015; Shorter et al., 2017). We resolved the amplitude of the body pitch acceleration (radians) and the fluke stroke rate (f, Hz) for all tag and drag loading conditions in Metabolic trials and Incremental Loading trials as in Shorter et al. (2017).
The results from the CFD simulations were used to estimate the relative increase in drag force created by the different tag conditions (Supplementary Figure S1). We fitted power functions to the relationship between speed (U; m s-1) and simulated drag (D; N):
where a and b are coefficients for the power function derived by least squares (cftool; MATLAB, 2014). The differences in drag forces between the tag and the other loading conditions were then calculated (Supplementary Figure S1).
The net drag force (Fd) created by the body of the animal was modeled as:
Where, Cd is the drag coefficient (0.01), ρ is the density of the water (1029 kg m-3), U is the relative speed of the animal in the water (1–4 m s-1), and Aw is the wetted surface area of the animal (2.3 m2) from (Fish, 1993).
Combining Eqs 2 and 3, we calculated the estimated drag forces for dolphins at their observed mean swimming speeds during the experimental conditions. Additionally, we calculated the swimming speed that would result in drag forces comparable to those in the control condition (Ured; m s-1 has drag forces equal to Dcontrol,Uobs; Supplementary Figure S1, closed circles), and estimated the forces that would be created if the animals maintained the swimming speed selected during the control condition using Eq. 3.
To determine whether metabolic rate differed between individuals or drag conditions, we used two-way ANOVA on three metabolic measures or proxies: post-exercise , τ1/2 (min), and breathing frequency during swimming. Our expectation was that all metabolic indices would be higher with increased drag loading. We used two-way ANOVA with post hoc Tukey’s test to determine whether swimming speed differed significantly between drag loading conditions, while controlling for individual behavior. We used two-way ANOVA to compare the fluke stroke frequency and amplitude between tag and tag+8 conditions during Metabolic trials. Statistical analyses were completed in MATLAB (2014) and R Core Team (2015). Reported values are mean ± SD.
Qualitatively, the CFD simulations illustrate how the tag and the added drag elements increased drag by perturbing the fluid flow around the instrument (Figure 2). The tag alone was relatively hydrodynamic with the flow remaining attached to the tag body, but the cup geometry created drag-inducing areas of stagnate recirculating flow. Adding bluff-bodied drag elements greatly affected flow around the tag, resulting in flow separation and large areas of recirculating flow behind the drag elements. As more drag elements were added this effect was magnified, resulting in increased drag and a growing disturbance to the fluid flow.
Figure 2. Computational fluid dynamics (CFD) simulation results for the different tag configurations in 2 m s-1 flow: (A) Tag, (B) Tag+2, (C) Tag+4, (D) Tag+6, and (E) Tag+8. These results illustrate how the tag and added drag elements affect the velocity of the flow in the simulation. (F) A representative example of how the tag and drag elements modified the flow around the animal during the experiment.
Four dolphins performed 26 metabolic trials under different drag loading conditions (control, tag, tag+8), the number and order of which we list in Table 1. Mean ( ± SD) air temperature and humidity were 27.3 ± 1.0°C (range 25.0–29.4°C) and 62 ± 5% (48–69%) during the times of the trials. The mean atmospheric pressure was 1016.7 ± 1.1 hPa (1014.8–1018.9 hPa) and mean water temperature in the lagoon was 24.6 ± 0.5°C. Different individuals had significantly different (F3,20 = 5.09, p < 0.0088) and s (F3,20 = 4.31; p = 0.0169) in the first minute after exercise; however, we did not detect a significant effect of drag on either or s (Figure 3 and Table 2).
Figure 3. Oxygen consumption rates (VO2; L min-1) of four bottlenose dolphins (represented by different colors) measured in the first minute after a 10-min swimming trial under control (no tag), tag and tag+8 conditions. Data points represent separate trials.
Table 2. Mean ( ± SD) values of mass-specific metabolic rate (sVO2; ml O2 kg-1 min-1), recovery half time (τ1/2; min), breathing frequency, swimming speed (m s-1), fluke stroke rate (FSR; Hz), and pitch amplitude (radians).
There was no significant difference in the τ1/2 with drag condition (Table 2); returned to half its maximum value after 1.97 ± 1.25 min (e.g., Figure 4). τ1/2 was highly variable between individuals (F3,20 = 5.41; p = 0.0073). There was no significant effect of drag loading on breathing frequency during the 10-min swimming task (Table 2 and Supplementary Figure S2). Drag condition significantly affected swimming speed (Figure 5 and Table 2); mean swimming speed was no different between control and tag-only conditions (Tukey HSD; p = 0.9182) but, when instrumented with the tag+8, swimming speed was significantly slower compared to in control and tag conditions (by 34 and 33% respectively; Tukey HSD; p = 0.0007 and 0.0009; Figure 5). Dolphins swam with significantly lower fluke stroke frequencies at higher drag loading, but no difference in pitch amplitude was detected (Table 2).
Figure 4. Measurements of instantaneous (oxygen consumption, L min-1) for one dolphin before and after exercise when wearing a biologging tag. Lighter symbols connected with dotted lines reflect individual measurements. Darker symbols with a solid line represent 30-s averages. The solid line post-exercise is the exponential decay curve from which the recovery half-time is calculated.
Figure 5. Mean swimming speed decreases with drag loading and estimated total body drag was maintained. Drag forces with velocity (lines) estimated from CFD simulations, and at mean swimming velocities (symbols) observed during 12 Metabolic trials (one for each animal × condition).
The swimming path of the dolphins during the different loading conditions is presented in Figure 6. As drag loading was decreased, the animal’s swimming speed and total distance traveled increased. Conversely, as drag loading was increased swimming speed and total distance traveled decreased (Supplementary Figure S3). The decreases in swim speed observed compared to the control were similar to predicted values from CFD simulations, if individuals were changing speed to maintain drag forces (Figure 7). Compared to the control (no tag), the two dolphins slowed on average 14 ± 4% when wearing just the tag, and slowed 24 ± 7, 27 ± 6, 35 ± 10, and 38 ± 6% with the tag+2, +4, +6, and +8 configurations, respectively (Figure 7). CFD simulations predicted a similar pattern but much smaller speed reductions compared to those observed. For example, CFD predicted a 1% speed reduction from the tag only, to 16% speed reduction for the tag+8 (Figure 7 and Table 3). Fluke stroke frequencies increased with swimming speed during Incremental Loading trials (Figure 8A). The overall trend was an increase in f as drag was removed from tag+8 to tag; however, individuals differed in the magnitude of responses between conditions (Figure 8C). As drag was added again, one individual decreased f while the other remained fairly consistent (Figure 8C). Mean fluke stroke amplitude decreased with speed (Figure 8B) and as drag was reduced (Figure 8D). Amplitudes increased as drag was added again, though the shape of this response varied between individuals (Figure 8D).
Figure 6. Dolphins progressively changed swimming paths with drag unloading and loading. Paths of swimming dolphins 9FL3 (A,B) and 6JK5 (C,D) tracked from aerial video footage as they swam counter-clockwise between waypoints. Drag was progressively decreased (A,C) and then increased (B,D) through drag loading conditions without the tag and up to the tag+8 (right). Gaps in paths reflect time periods when the dolphins were not visible for > 2 time steps, ∼1 s. Note the zoomed inset in (D).
Figure 7. Dolphins changed speed with incremental changes in drag loading, in line with what is expected from simulation models. Observed swimming speed (circles) and expected drag force at that speed (from CFD modeling; lines) in each experimental condition as drag is manipulated on two bottlenose dolphins (A: 9FL3 and B: 6JK5) by removing (connected by dotted lines) and adding (connected by solid lines) eurethane elements and a biologging tag (schematic right).
Table 3. Equations for drag with speed from computational fluid dynamics (CFD) simulations of tags of increasing size and a dolphin without a tag and with tags of increasing size, the absolute (N) and percent increase in drag force at 4 m s-1, and the expected absolute (m s-1) and percent reduction in speed required to maintain drag forces when not wearing a tag at 4 m s-1, and the observed speed (m s-1) and change in speed (%) during Incremental Loading trials.
Figure 8. Dolphins changed fine-scale movements with incremental changes in drag loading. (A,B) Mean ± SD fluke stroke frequency (Hz) and amplitude (rad) with speed (m s-1) in all drag loading conditions for two individuals. (C,D) Mean ± SD fluke stroke frequency (Hz) and amplitude (rad) in two animals (Animal 9FL3 connected by dotted lines; 6JK5 connected by dashed lines) as drag elements and a biologging tag (right) are removed (connected by dotted lines) and then added (connected by solid lines) to manipulate drag loading.
Energy economy plays an important part in behavioral strategies animals employ to locomote (Fish, 1998; Williams et al., 2000); as such, tagged animals may compensate for drag from biologging tags by modifying swimming biomechanics or behavior. In this work, we investigated if and how animals modify their behavior in response to hydrodynamic loading created by tags of increasing size, or if and how they accommodate the increased drag created by the tag.
Despite the significant increase in drag created by the largest tag loading condition, the 10-min exercise period did not lead to a detectable difference in the recovery during the post-exercise period for any of the loading conditions (Figures 3, 4). We did not expect a significant effect of the tag-only configuration, given lower drag loading [1.02× total increase, 4 N at 4 m s-1 compared to the larger DTAG2 used in van der Hoop et al. (2014); 1.17× total increase; 20 N at 4 m s-1], which also yielded no detectable metabolic effect. But we did expect an increased cost associated with the tag+8 attachment. The simulations predicted that the tag+8 configuration would increase the drag on the dolphins by 77 N at 4 m s-1, a 1.4× total increase, but we detected no consistent or significant metabolic effect during recovery. This could be because the dolphins decreased their swimming speed by 34% and reduced their fluke stroke rates compared to the control condition. This change in speed reduced the estimated drag on the animal by 40% (125 to 74 N; Figure 5). The effect of the tag without added drag elements was less dramatic, with no detectable difference in swimming speed between the control and tag conditions (Figure 5).
By incrementally changing the tag configuration (Incremental Loading trials), we were able to demonstrate two additional examples where the dolphins modified their behavior in response to small (<20 N) changes in drag. Using a more thorough combination of data types and analysis methods (camera-tracked speed and position data, along with animal-borne tag data) with a subset of the animals (n = 2) we were able to measure other changes in behavior that occurred in response to the drag loading. When drag was reduced by removing elements (Incremental Loading trials), dolphins changed their fluke stroke rate, swimming speed, and modified their swimming path. As drag was increased again, dolphins reduced fluke stroke rate, slowed down, and decreased total distance traveled (Figures 6–8 and Table 3). We did observe differences in the response to the loading between individuals; for example, one individual showed a large increase in fluke stroke rate between Tag+8 and Tag+4 during unloading (Figure 8C) whereas fluke stroke rate was relatively unchanged during re-loading. These differences in response may be related to small changes in amplitude and speed (Fish and Rohr, 1999). We did not detect a difference in fluke stroke amplitude during Metabolic trials, while we did see a decrease in amplitude with speed in the Incremental Loading trials (Figure 8B). This may be due to the higher swimming speeds or sample size across a range of drag scenarios; future work to further quantify the timing and interaction between these parameters, and how they change through time and with drag, would address more fundamental questions in swimming biomechanics. Interestingly, when swimming with the added drag during the Incremental Loading trials the animals slowed to speeds that reduced the overall estimated body drag to levels below what the simulations would predict if the animals were just slowing to maintain a constant body drag (Figure 7). This reduction in speed is likely not due to animals tiring from the exercise protocol as (1) the recovery time (τ1/2) from Metabolic trials was less than the average time between Incremental Loading trials (i.e., animals likely recovered between laps) and (2) the same changes in swimming speed and kinematics were observed in the shorter Incremental Loading and longer Metabolic trials.
In the Metabolic and Incremental loading trials, individuals reduced their speed more than we expected based on drag forces from CFD models (Table 3). CFD modeling predicted a 1–16% reduction in speed across the tag conditions, whereas we observed reductions of 14–38%, significant beyond the tag condition (Table 2 and Supplementary Figure S3). Wearing the tag and additional elements may elicit effects separate from drag (associated with lift forces, handling, the sensation from the suction cups). Improved CFD modeling with full dolphin geometry may also reduce the discrepancy between expected and observed results.
Reduced activity levels have been observed in tagged bottlenose dolphins (Blomqvist and Amundin, 2004) and porpoises (Geertsen et al., 2004), along with significantly lower speeds in instrumented (Lang and Daybell, 1963; Skrovan et al., 1999) or pregnant dolphins (Noren et al., 2011). Other swimmers show similar behavioral changes in response to drag from external tags (Wilson et al., 1986; also reviewed in van der Hoop et al., 2014; Jepsen et al., 2015; Rosen et al., 2017) or other external features (e.g., van der Hoop et al., 2017). The dolphins in this study were able to reduce their speeds as there was no constraint to maintain it. Free-ranging animals face different motivators and constraints; for example, high-speed maneuvers are required to evade predators, pursue prey (Goldbogen et al., 2007; Aguilar Soto et al., 2008) and maintain social cohesiveness (Wursig, 1982). Additional energetic expenses can occur when maintaining speed with higher drag. Fish and eels with external tags show significantly increased metabolic rates when maintaining swimming speeds (reviewed in Jepsen et al., 2015).
It is important to consider how these results in a controlled setting contribute a greater understanding of movement and energy in cetaceans. From an evolutionary standpoint, animals may seek to minimize energetic costs, especially those involved in everyday actions (e.g., routine swimming; Sparrow and Newell, 1998). Movements can be adapted to minimize metabolic energy expenditure with respect to constraints imposed by a task (swim from A to B), the environment (low drag, high drag), and the organism itself (body shape, size). By perceiving a difference in conditions between tasks and adapting behavior to reduce expenditure, the dolphins in this study are economical (Sparrow and Newell, 1998; Halsey, 2016). Beyond economy, these results indicate the sensitivity of these dolphins to small changes in their hydrodynamics, which changed their swimming speed (Figure 7), fluking (Figure 8), and more subtly their swimming paths (Figure 6) in response to small changes in drag loading. These results reflect specific responses of dolphins in this controlled setting, which offers the opportunity to combine measurements of individual energetics and biomechanics to refine our understanding of energetically optimal swimming gaits and help interpret tag data recorded on free-swimming, wild marine mammals.
Our experimental results indicate that even a relatively small increase from a hydrodynamic tag can result in measurable changes in behavior. The DTAG alone did not strongly or significantly affect the drag load on the bottlenose dolphins tested; as such, we expect it elicits little-to-no effect on animals of similar size and shape. The animal responses to the drag elements illustrate that larger tags, or those that are larger relative to the subject’s body size (Portugal et al., 2018), as well as tags that are less hydrodynamically shaped, have the potential to affect measured biomechanics and swimming speed. The DTAG added 4 N at 4 m s-1 (increase of 2%) whereas the tag+2 added 27 N (increase of 14%; Table 3); these blunt drag elements therefore induce more drag than the original tag, and indicate the importance of shape. These experiments were also conducted with the tag in an optimal location for small cetaceans: between the blowhole and dorsal fin, in the forward-facing orientation. Tags deployed in capture-release programs are typically placed in this preferred position; however, pole-based deployments on cetaceans can result in variable attachment orientation and location. Tags placed on different regions of the body may have considerably different hydrodynamic regimes and will therefore contribute different drag and lift forces and pitching moments: when placed ahead of the point of maximal girth tags can lead to early flow separation and large increases in frontal area (Culik and Wilson, 1991; Healy et al., 2004; Vandenabeele et al., 2014), but those placed too far caudally can result in body destabilization. When oriented sideways or in any direction off-axis, hydrodynamic tags will have a greater frontal area; experimental and simulated drag forces on the similar tag bodies vary by ±10 N depending on orientation (Shorter et al., 2013). Based on these results we recommend that hydrodynamic tag housings continue to be designed and/or refined (Pavlov and Rashad, 2012; Balmer et al., 2013; Shorter et al., 2013; Fiore et al., 2017) as well as adopted (even when this affects the relative size of the tag), and that researchers directly acknowledge the potential tag impacts on their measured data, especially on smaller animals.
We sought to quantify the metabolic cost of biologging tags on bottlenose dolphins during a controlled swimming task. A combination of data types (e.g., position, fine-scale movement, speed, and physiological measurements) allowed us to detect and measure alternative or adaptive strategies that tag subjects can use when faced with drag loading. When performing the tasks at their freely-chosen pace, dolphins adjusted their swimming speed and movement patterns, and no change in metabolic cost was detected. Further studies to constrain the task to determine metabolic impact when high-speed behaviors are maintained are required.
All data and code associated with this manuscript have been made available: doi: 10.5281/zenodo.1489118.
JvdH, AF, KS, JR-L, and MM developed concepts and performed fieldwork. JR-L directed animal husbandry and training. JvdH, AF, and JG processed and analyzed data. KS and VP conducted and analyzed simulations. JvdH, AF, KS, VP, JR-L, and MM wrote the manuscript.
Funding for this project was provided by the National Oceanographic Partnership Program (National Science Foundation via the Office of Naval Research N00014-11-1-0113 to MM) and the Office of Naval Research (ONR YIP Award N000141410563 to AF). Dolphin Quest provided in-kind support of animals, crew, and access to resources. JvdH was supported by a Postgraduate Scholarship from the Natural Sciences and Engineering Research Council of Canada.
The authors declare that the research was conducted in the absence of any commercial or financial relationships that could be construed as a potential conflict of interest.
The authors give special thanks to Dolphin Quest Oahu whose dolphin interactive facility at The Kahala Hotel & Resort served as a critically important controlled research environment for this study. They thank JvdH’s thesis committee members for additional comments that improved the manuscript, and D. Zhang and A. Stoldt for assistance with camera and tag data processing.
The Supplementary Material for this article can be found online at: https://www.frontiersin.org/articles/10.3389/fmars.2018.00465/full#supplementary-material
FIGURE S1 | Drag forces on a bottlenose dolphin (black) and a dolphin instrumented with a tag (blue) and tags with additional drag-adding elements as simulated and predicted from computational fluid dynamics (solid lines). Coefficients for the equations for each condition (e.g., Dtag+8 = aUb) are listed in Table 3. With added drag, dolphins can maintain speed (e.g., 4 m s-1) but experience higher drag forces (open symbols) or can reduce speed (e.g., Ured,tag+8) to maintain the drag force they experience when not wearing a tag (e.g., Dcontrol, U = 4; solid symbols).
FIGURE S2 | Mean breathing frequency of four bottlenose dolphins (each represented by different colors) during a 10-min swimming task when wearing no tag (control; circles), a biologging tag (triangles) and a tag with eight extra drag elements (tag+8; squares).
FIGURE S3 | Total distance traveled (m) versus swimming speed (m s-1) of the two dolphins (Animal 9FL3 open symbols; 6JK5 closed symbols) in the Incremental Loading Trials.
Aguilar Soto, N., Johnson, M. P., Madsen, P. T., Diaz, F., Dominguez, I., Brito, A., et al. (2008). Cheetahs of the deep sea: deep foraging sprints in short-finned pilot whales off Tenerife (Canary Islands). J. Anim. Ecol. 77, 936–947. doi: 10.1111/j.1365-2656.2008.01393.x
Alexander, R. M. N. (2003). Principles of Animal Locomotion. Princeton, NJ: Princeton University Press. doi: 10.1515/9781400849512
Balmer, B. C., Wells, R. S., Howle, L. E., Barleycorn, A. A., McLellan, W. A., Ann Pabst, D., et al. (2013). Advances in cetacean telemetry: a review of single-pin transmitter attachment techniques on small cetaceans and development of a new satellite-linked transmitter design. Mar. Mamm. Sci. 30, 656–673. doi: 10.1111/mms.12072
Bannasch, R., Wilson, R. P., and Culik, B. M. (1994). Hydrodynamic aspects of design and attachment of a back-mounted device in penguins. J. Exp. Biol. 194, 83–96.
Barron, D. G., Brawn, J. D., and Weatherhead, P. J. (2010). Meta-analysis of transmitter effects on avian behaviour and ecology. Methods Ecol. Evol. 1, 180–187. doi: 10.1111/j.2041-210X.2010.00013.x
Best, P. B., Mate, B., and Lagerquist, B. (2015). Tag retention, wound healing, and subsequent reproductive history of southern right whales following satellite-tagging. Mar. Mamm. Sci. 31, 520–539. doi: 10.1111/mms.12168
Blomqvist, C., and Amundin, M. (2004). An acoustic tag for recording directional pulsed ultrasounds aimed at free-swimming bottlenose dolphins (Tursiops truncatus) by conspecifics. Aquat. Mamm. 30, 345–356. doi: 10.1578/AM.30.3.2004.345
Broell, F., Burnell, C., and Taggart, C. T. (2016). Measuring abnormal movements in free-swimming fish with accelerometers: implications for quantifying tag and parasite load. J. Exp. Biol. 219, 695–705. doi: 10.1242/jeb.133033
Brown, D. (2014). Tracker Video Analysis and Modeling Tool. Available at: http://www.cabrillo.edu/∼dbrown/tracker/ accessed 8 November 2014
Cohen-Solal, A., Laperche, T., Morvan, D., Geneves, M., Caviezel, B., and Gourgon, R. (1995). Prolonged kinetics of recovery of oxygen consumption after maximal graded exercise in patients with chronic heart failure. analysis with gas exchange measurements and NMR spectroscopy. Circulation 91, 2924–2932. doi: 10.1161/01.CIR.91.12.2924
Crossin, G. T., Cooke, S. J., Goldbogen, J. A., and Phillips, R. A. (2014). Tracking fitness in marine vertebrates: current knowledge and opportunities for future research. Mar. Ecol. Prog. Ser. 496, 1–17. doi: 10.3354/meps10691
Culik, B., and Wilson, R. P. (1991). Swimming energetics and performance of instrumented adelie penguins (Pygoscelis adeliae). J. Exp. Biol. 158, 355–368.
Di Prampero, P. E., Davies, C. T., Cerretelli, P., and Margaria, R. (1970). An analysis of O2 debt contracted in submaximal exercise. J. Appl. Physiol. 29, 547–551. doi: 10.1152/jappl.1970.29.5.547
Elliott, K. H., McFarlane-Tranquilla, L., Burke, C. M., Hedd, A., Montevecchi, W. A., and Anderson, W. G. (2012). Year-long deployments of small geolocators increase corticosterone levels in murres. Mar. Ecol. Prog. Ser. 466, 1–7. doi: 10.3354/meps09975
Fahlman, A., Hastie, G. D., Rosen, D. A. S., Naito, Y., and Trites, A. W. (2008). Buoyancy does not affect diving metabolism during shallow dives in Steller sea lions Eumetopias jubatus. Aquat. Biol. 3, 147–154. doi: 10.3354/ab00074
Fahlman, A., Loring, S. H., Levine, G., Rocho-Levine, J., Austin, T., and Brodsky, M. (2015). Lung mechanics and pulmonary function testing in cetaceans. J. Exp. Biol. 218, 2030–2038. doi: 10.1242/jeb.119149
Fahlman, A., van der Hoop, J., Moore, M. J., Levine, G., Rocho-Levine, J., and Brodsky, M. (2016). Estimating energetics in cetaceans from respiratory frequency: why we need to understand physiology. Biol. Open 5, 436–442. doi: 10.1242/bio.017251
Fiore, G., Anderson, E., Garborg, C. S., Murray, M., Johnson, M., Moore, M. J., et al. (2017). From the track to the ocean: using flow control to improve marine bio-logging tags for cetaceans. PLoS One 12:e0170962. doi: 10.1371/journal.pone.0170962
Fish, F. E. (1993). Power output and propulsive efficiency of swimming bottlenose dolphins (Tursiops truncatus). J. Exp. Biol. 185, 179–193.
Fish, F. E. (1998). Comparative kinematics and hydrodynamics of odontocete cetaceans: morphological and ecological correlates with swimming performance. J. Exp. Biol. 201, 2867–2877.
Fish, F. E., and Rohr, J. J. (1999). Review of Dolphin Hydrodynamics and Swimming Performance. San Diego, CA: US Navy SPAWAR Systems Center Technical Report 1801. doi: 10.21236/ADA369158
Geertsen, B. M., Teilmann, J., Kastelein, R. A., Vlemmix, H. N. J., and Miller, L. A. (2004). Behaviour and physiological effects of transmitter attachments on a captive harbour porpoise (Phocoena phocoena). J. Cetacean Res. Manag. 6, 139–146.
Goldbogen, J. A., Pyenson, N. D., and Shadwick, R. E. (2007). Big gulps require high drag for fin whale lunge feeding. Mar. Ecol. Prog. Ser. 349, 289–301. doi: 10.1098/rsif.2008.0492
Halsey, L. G. (2016). Terrestrial movement energetics: current knowledge and its application to the optimising animal. J. Exp. Biol. 219, 1424–1431. doi: 10.1242/jeb.133256
Healy, M., Chiaradia, A., Kirkwood, R., and Dann, P. (2004). Balance: a neglected factor when attaching external devices to penguins. Mem. Natl. Inst. Polar Res. 58, 179–182.
Hussey, N. E., Kessel, S. T., Aarestrup, K., Cooke, S. J., Cowley, P. D., Fisk, A. T., et al. (2015). Aquatic animal telemetry: a panoramic window into the underwater world. Science 348:1255642. doi: 10.1126/science.1255642
Jepsen, N., Thorstad, E. B., Havn, T., and Lucas, M. C. (2015). The use of external electronic tags on fish: an evaluation of tag retention and tagging effects. Anim. Biotelem. 3:49. doi: 10.1186/s40317-015-0086-z
Johnson, M. (2015). DTAG Toolbox for MATLAB. Available at: http://soundtags.st-andrews.ac.uk/dtags/dtag-toolbox/
Johnson, M., Aguilar de Soto, N., and Madsen, P. T. (2009). Studying the behaviour and sensory ecology of marine mammals using acoustic recording tags: a review. Mar. Ecol. Prog. Ser. 395, 55–73. doi: 10.3354/meps08255
Johnson, M., and Tyack, P. (2003). A digital acoustic recording tag for measuring the response of wild marine mammals to sound. IEEE J. Ocean. Eng. 28, 3–12. doi: 10.1109/JOE.2002.808212
Jones, T. T., Van Houtan, K. S., Bostrom, B. L., Ostafichuk, P., Mikkelsen, J., Tezcan, E., et al. (2013). Calculating the ecological impacts of animal-borne instruments on aquatic organisms. Methods Ecol. Evol. 4, 1178–1186. doi: 10.1111/2041-210X.12109
Jones, W. P., and Launder, B. E. (1972). The prediction of laminarization with a two-equation model of turbulence. Int. J. Heat Mass Transf. 15, 301–314. doi: 10.1016/0017-9310(72)90076-2
Kinaci, O. K., Sukas, O. F., and Bal, S. (2015). Prediction of wave resistance by a reynolds-averaged navier–stokes equation–based computational fluid dynamics approach. Proc. Inst. Mech. Eng. Part M 230, 531–548.
Lang, T. G., and Daybell, D. A. (1963). Porpoise Performance Tests in a Sea-Water Tank. Naval Ordnance Test Station Technical Paper 3063. NAVWEPS Report 8060, Ridgecrest, CA: Naval Ordnance Test Station, 32. doi: 10.21236/AD0298742
Lloyd, G., and Espanoles, A. (2002). Best Practice Guidelines for Marine Applications of Computational Fluid Dynamics. London: WS Atkins Consultants and Members of the NSC, MARNET-CFD Thernatic Network, 84.
MATLAB (2014). MATLAB and Statistics Toolbox Release 2014b. Available at: http://www.mathworks.com/
National Weather Service (2015). Weather Observations, Vol. 2013. Honolulu, HI: Honolulu International Airport.
Noren, S. R., Redfern, J. V., and Edwards, E. F. (2011). Pregnancy is a drag: hydrodynamics, kinematics and performance in pre- and post-parturition bottlenose dolphins (Tursiops truncatus). J. Exp. Biol. 214, 4151–4159. doi: 10.1242/jeb.059121
Ohashi, K., Thomson, J. D., and D’Souza, D. (2007). Trapline foraging by bumble bees: IV. Optimization of route geometry in the absence of competition. Behav. Ecol. 18, 1–11. doi: 10.1093/beheco/arl053
Pavlov, V. V., and Rashad, A. M. (2012). A non-invasive dolphin telemetry tag: computer design and numerical flow simulation. Mar. Mamm. Sci. 28, E16–E27. doi: 10.1111/j.1748-7692.2011.00476.x
Portugal, S. J., White, C. R., and Börger, L. (2018). Miniaturization of biologgers is not alleviating the 5% rule. Methods Ecol. Evol. 9, 1662–1666. doi: 10.1016/j.cbpa.2016.05.025
Quanjer, P. H., Tammeling, G. J., Cotes, J. E., Pedersen, O. F., Peslin, R., and Yernault, J.-C. (1993). Lung volumes and forced ventilatory flows. Eur. Resp. J. 6, 5–40. doi: 10.1183/09041950.005s1693
R Core Team (2015). R: A Language and Environment for Statistical Computing. Available at: https://www.r-project.org/
Rodi, W. (1991). “Experience with two-layer models combining the k-e model with a one-equation model near the wall,” in Proceedings of the 29th Aerospace Sciences Meeting AIAA 91-0216, (Reno, NA: AIAA). doi: 10.2514/6.1991-216
Ropert-Coudert, Y., and Wilson, R. P. (2004). Subjectivity in bio-logging science: do logged data mislead? Mem. Natl. Inst. Polar Res. 58, 23–33.
Rosen, D. A. S., Gerlinsky, C. G., and Trites, A. W. (2017). Telemetry tags increase the costs of swimming in northern fur seals, Callorhinus ursinus. Mar. Mamm. Sci. 34, 385–402. doi: 10.1111/mms.12460
Royce, J. (1969). Active and passive recovery from maximal aerobic capacity work. Int. Z. Angew. Physiol. 28, 1–8. doi: 10.1007/BF00696033
Rufli, M., Scaramuzza, D., and Siegwart, R. (2008). “Automatic detection of checkerboards on blurred and distorted images,” in Proceedings of the IEEE/RSJ International Conference on Intelligent Robots and Systems (IROS 2008), Nice. doi: 10.1109/IROS.2008.4650703
Scaramuzza, D., Martinelli, A., and Siegwart, R. (2006). “A toolbox for easy calibrating omnidirectional cameras,” in Proceedings to IEEE International Conference on Intelligent Robots and Systems (IROS 2006), Beijing. doi: 10.1109/IROS.2006.282372
Shorter, K. A., Murray, M. M., Johnson, M., Moore, M. J., and Howle, L. E. (2013). Drag of suction cup tags on swimming animals: modeling and measurement. Mar. Mamm. Sci. 30, 726–746. doi: 10.1111/mms.12083
Shorter, K. A., Shao, Y., Ojeda, L., Barton, K., Rocho-Levine, J., van der Hoop, J., et al. (2017). A day in the life of a dolphin: using bio-logging tags for improved animal health and well-being. Mar. Mamm. Sci. 33, 785–802. doi: 10.1111/mms.12408
Skrovan, R. C., Williams, T. M., Berry, P. S., Moore, P. W., and Davis, R. W. (1999). The diving physiology of bottlenose dolphins (Tursiops truncatus). II. Biomechanics and changes in buoyancy at depth. J. Exp. Biol. 202, 2749–2761.
Sparrow, W. A., and Newell, K. M. (1998). Metabolic energy expenditure and the regulation of movement economy. Psychon. Bull. Rev. 5, 173–196. doi: 10.3758/BF03212943
Sridhar, D., Bhanuprakash, T. V. K., and Das, H. N. (2010). Frictional resistance calculations on a ship using CFD. Int. J. Comput. Appl. 11, 24–31. doi: 10.5120/1577-2109
van der Hoop, J. M., Fahlman, A., Hurst, T., Rocho-Levine, J., Shorter, K. A., Petrov, V., et al. (2014). Bottlenose dolphins modify behavior to reduce metabolic effect of tag attachment. J. Exp. Biol. 217, 4229–4236. doi: 10.1242/jeb.108225
van der Hoop, J. M., Nowacek, D. P., Moore, M. J., and Triantafyllou, M. S. (2017). Swimming kinematics and efficiency of entangled North Atlantic right whales. Endanger. Species Res. 32, 1–17. doi: 10.3354/esr00781
Vandenabeele, S., Wilson, R., and Grogan, A. (2011). Tags on seabirds: how seriously are instrument-induced behaviours considered? Anim. Welf. 20, 559–571.
Vandenabeele, S. P., Grundy, E., Friswell, M. I., Grogan, A., Votier, S. C., and Wilson, R. P. (2014). Excess baggage for birds: inappropriate placement of tags on gannets changes flight patterns. PLoS One 9:e92657. doi: 10.1371/journal.pone.0092657
Webb, P. M., Crocker, D. E., Blackwell, S. B., Costa, D. P., and Le Boeuf, B. J. (1998). Effects of buoyancy on the diving behavior of northern elephant seals. J. Exp. Biol. 201, 2349–2358.
Webb, P. W. (1971a). The swimming energetics of trout I. Thrust and power output at cruising speeds. J. Exp. Biol. 55, 489–520.
Webb, P. W. (1971b). The swimming energetics of trout II. Oxygen consumptin and swimming efficiency. J. Exp. Biol. 55, 521–540.
Williams, T. M., Davis, R. W., Fuiman, L. A., Francis, J., Le Boeuf, B. J., Horning, M., et al. (2000). Sink or swim: strategies for cost-efficient diving by marine mammals. Science 288, 133–136. doi: 10.1126/science.288.5463.133
Wilson, R. P., Grant, W. S., and Duffy, D. C. (1986). Recording devices on free-ranging marine animals: does measurement affect foraging performance? Ecology 67, 1091–1093. doi: 10.2307/1939832
Keywords: drag, swimming efficiency, adaptive behavior, tag effect, biomechanics, metabolism
Citation: van der Hoop JM, Fahlman A, Shorter KA, Gabaldon J, Rocho-Levine J, Petrov V and Moore MJ (2018) Swimming Energy Economy in Bottlenose Dolphins Under Variable Drag Loading. Front. Mar. Sci. 5:465. doi: 10.3389/fmars.2018.00465
Received: 28 July 2018; Accepted: 20 November 2018;
Published: 11 December 2018.
Edited by:
Cory D. Champagne, National Marine Mammal Foundation, United StatesReviewed by:
Jennifer Maresh, West Chester University, United StatesCopyright © 2018 van der Hoop, Fahlman, Shorter, Gabaldon, Rocho-Levine, Petrov and Moore. This is an open-access article distributed under the terms of the Creative Commons Attribution License (CC BY). The use, distribution or reproduction in other forums is permitted, provided the original author(s) and the copyright owner(s) are credited and that the original publication in this journal is cited, in accordance with accepted academic practice. No use, distribution or reproduction is permitted which does not comply with these terms.
*Correspondence: Julie M. van der Hoop, anZhbmRlcmhvb3BAd2hvaS5lZHU=
Disclaimer: All claims expressed in this article are solely those of the authors and do not necessarily represent those of their affiliated organizations, or those of the publisher, the editors and the reviewers. Any product that may be evaluated in this article or claim that may be made by its manufacturer is not guaranteed or endorsed by the publisher.
Research integrity at Frontiers
Learn more about the work of our research integrity team to safeguard the quality of each article we publish.