- 1Science for Life Laboratory, KTH Royal Institute of Technology, Stockholm, Sweden
- 2Department of Bioengineering, Istanbul Medeniyet University, Istanbul, Turkey
- 3Department of Bioengineering, Marmara University, Istanbul, Turkey
- 4Department of Chemistry and Molecular Biology, University of Gothenburg, Gothenburg, Sweden
- 5Department of Molecular and Clinical Medicine, Sahlgrenska University Hospital, University of Gothenburg, Gothenburg, Sweden
- 6Department of Biology and Biological Engineering, Chalmers University of Technology, Gothenburg, Sweden
Drug repositioning has gained attention from both academia and pharmaceutical companies as an auxiliary process to conventional drug discovery. Chemotherapeutic agents have notorious adverse effects that drastically reduce the life quality of cancer patients so drug repositioning is a promising strategy to identify non-cancer drugs which have anti-cancer activity as well as tolerable adverse effects for human health. There are various strategies for discovery and validation of repurposed drugs. In this review, 25 repurposed drug candidates are presented as result of different strategies, 15 of which are already under clinical investigation for treatment of prostate cancer (PCa). To date, zoledronic acid is the only repurposed, clinically used, and approved non-cancer drug for PCa. Anti-cancer activities of existing drugs presented in this review cover diverse and also known mechanisms such as inhibition of mTOR and VEGFR2 signaling, inhibition of PI3K/Akt signaling, COX and selective COX-2 inhibition, NF-κB inhibition, Wnt/β-Catenin pathway inhibition, DNMT1 inhibition, and GSK-3β inhibition. In addition to monotherapy option, combination therapy with current anti-cancer drugs may also increase drug efficacy and reduce adverse effects. Thus, drug repositioning may become a key approach for drug discovery in terms of time- and cost-efficiency comparing to conventional drug discovery and development process.
Introduction
The nature presented itself as a potential drug resource for scientists enabling serendipitous discoveries for centuries. The venture of drug discovery has started with random screening of crude plants such as reserpine, lavender, and opium, and latterly created the drug industry, which is the one of the most advanced industries in our society (Giridhar, 2012). As a part of this industry, the drug discovery process had enormous investments into infrastructures. Research and development (R&D) require huge amounts of time and money. Further, translation of the promising molecule to an approved drug has major problems including high failure rates and withdrawal risks by reason of safety or efficacy problems (Shim and Liu, 2014; Würth et al., 2016).
Drug repositioning (DR), which is an auxiliary process to conventional drug discovery has gained attention from both academia and pharmaceutical companies. Drug repositioning, also named “drug repurposing or drug recycling,” is a promising approach to overcome hurdles in discovering and developing new drugs via identification of the new therapeutic applications of known drugs. Repurposed drug candidates have established formulations and manufacturing methods, extensive pharmacokinetic properties, known adverse effects, clinical trial information, and post-marketing surveillance safety data which make a beeline for the drug development with less chance of failure (Deotarse et al., 2015). To date, there are many success stories about repositioned drugs. The first and most well-known example of DR was discovered serendipitously. Sildenafil was originally produced to be used in coronary artery disease but after the failure on phase 2 trials, it was directed to the treatment of erectile dysfunctions because of its side effect in inducing penile erections (Li and Jones, 2012; Deotarse et al., 2015). Thalidomide, everolimus, nelfinavir, minoxidil, and more drugs have been proven effective in treating another disease than intended at first indications (Huang et al., 2011; Li and Jones, 2012; Pantziarka et al., 2014; Deotarse et al., 2015).
Cancer is one of the most complex diseases as well as the leading cause of death worldwide. Epidemiologic studies pointed out 14.1 million new cancer cases and 8.2 million deaths globally in 2012. Among all cancer types, prostate cancer (PCa) is the fourth most prevalent cancer without distinction of gender and the second most prevalent cancer among men. An approximate 1.1 million cases were diagnosed with PCa in 2012, accounting for 15% of the cancers diagnosed worldwide in men (Ferlay et al., 2015). A recent report showed that PCa in the United States is the first and second leading cancer type considering estimated new cases and deaths, respectively (Siegel et al., 2018).
Most of the conventional anti-cancer chemotherapeutic agents may have notorious adverse effects that drastically reduce the life quality of cancer patients. Therefore, DR is an encouraging strategy to identify non-cancer drugs having anti-cancer activity as well as little or tolerable adverse effects for human health. Hence, DR and any progression on workflows for efficient DR may ameliorate the professed inefficiency of conventional drug development process as previously discussed (Shim and Liu, 2014; Vanhaelen et al., 2017).
In the context of drug discovery, this review focuses on non-cancer drugs that are repurposed to be used as potential PCa therapeutics. We begin by discussing the different DR approaches which enable the researchers to not only discover new candidates but also validate their results as a part of the study. Second, a comprehensive overview is given on the repurposed non-cancer drugs against PCa which have been under clinical investigation until now. Finally, we discuss the gaps and future challenges on drug repositioning approaches and the concepts to propel the field forward for treating PCa as well as other cancer types. Drugs repositioned for PCa treatment may potentially be used in other cancer types since common pathways or targets might be shared in different cancer types regardless of tissue types.
Drug Repositioning Approaches for the Treatment of PCa
Drug repositioning approaches are applicable when discovering new therapeutics and also validating the candidate drugs. We evaluated methods used for discovery of new therapeutics in three main categories (Figure 1) and explained them in terms of PCa treatment. Activity-based DR and in silico-based DR were mentioned previously (Shim and Liu, 2014). However, a notable number of current drug repositioning studies for PCa were established based on observations from previous studies. Therefore, we aimed to consolidate this categorization with knowledge-based DR. We provide examples of DR for PCa comprising different discovery and validation methods (Table 1). We also discuss alternative/complementary approaches and their advantages/disadvantages for new drug discovery (Table 2).
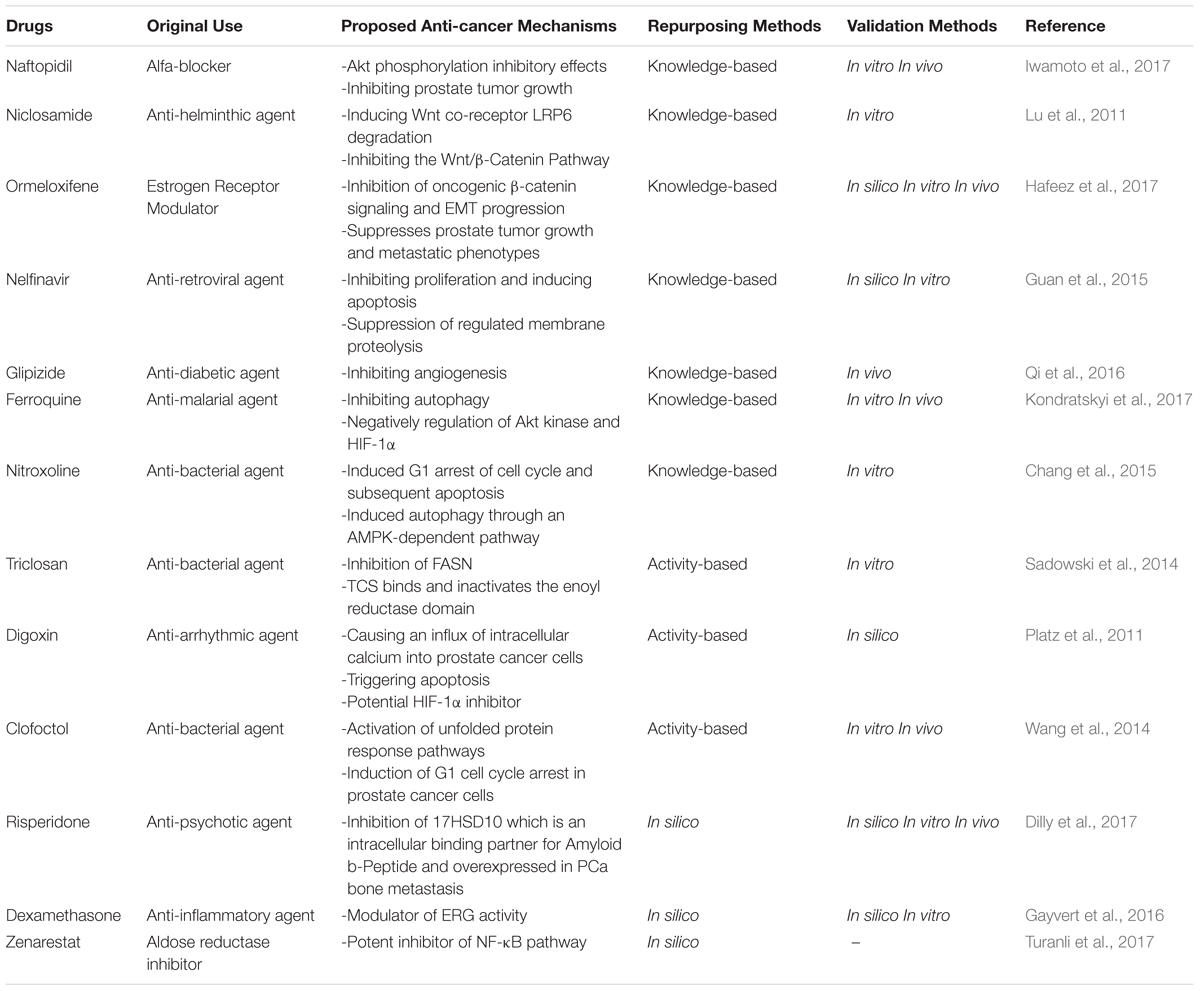
TABLE 1. Non-cancer drug repositioning candidates for prostate cancer and their repurposing strategies.
Knowledge-Based Drug Repositioning
The term “knowledge-based drug repositioning” represents drug repositioning based on knowledge of the medical doctors or researchers and their ability to interpret scientific observations or just coincidences. The first examples of drug repositioning were found serendipitously while working on another disease. Afterward, similar disease indications, sharing altered pathways or the need of combination therapies, led researchers to look for observations and repurpose drugs for a disease rather than the originally targeted one. Hence, these success stories have become prominent and still practicing in the context of drug repositioning. Recent knowledge-based DR studies on PCa have presented new candidates such as ormeloxifene (Hafeez et al., 2017), naftopidil (Iwamoto et al., 2017), ferroquine (FQ) (Kondratskyi et al., 2017), nelfinavir (Guan et al., 2015), nitroxoline (Chang et al., 2015), and glipizide (Qi et al., 2016).
Previous studies showed that loss of E-cadherin accompanying abundancy of N-cadherin is involved in epithelial-to-mesenchymal transition (EMT), leading to PCa being metastatic and more aggressive. Moreover, aberrant expression of β-catenin which plays a major role in EMT is a part of the major oncogenic signaling pathways (Jaggi et al., 2005, 2006). Hafeez et al. (2017) suggested that the clinically approved selective estrogen receptor modulator, ormeloxifene, may inhibit EMT by repressing N-cadherin and β-catenin/TCF-4 transcriptional activity. Ormeloxifene has already been reported to demonstrate anti-cancer activity in different carcinoma such as ovarian, head and neck, and breast. However, Hafeez et al. (2017) studied ormeloxifene for the treatment of PCa and explained its effects on EMT processes and Wnt/β-catenin signaling. To validate their hypothesis, they used molecular docking as an in silico validation method and ormeloxifene showed proficient docking with β-catenin and GSK-3β. In addition, in vitro cell culture studies showed that ormeloxifene induced apoptosis, and reduced tumorigenic, metastatic, and invasive potential of PCa cells. Moreover, treatment remarkably reduced the prostate tumor growth in the xenograft mouse models as in vivo validation for effects of ormeloxifene.
Radiotherapy (RT) is an alternative option to surgery for the treatment of localized PCa. However, cancer cells develop resistance to RT, since RT induces upregulation of anti-oxidant enzymes which decompose free radicals inside the cancer cell and protect them. To increase efficiency of therapy, RT has been investigated in combination with Akt inhibitors (Xu et al., 2010) and, in another study, with a Hedgehog inhibitor (Gonnissen et al., 2016). The selective adrenoreceptor A1D antagonist naftopidil is used for treating lower urinary tract symptoms triggered by benign prostatic hyperplasia (Masumori, 2011). Iwamoto et al. (2017) proposed naftopidil therapy in combination with RT to increase treatment efficiency. They reported significant growth inhibitory effects in addition to Akt phosphorylation-inhibitory effects of naftopidil.
Targeting autophagy was presented as promising direction to repurpose anti-cancer therapeutics and therefore discussed in detail (Piao and Amaravadi, 2016; Rebecca and Amaravadi, 2016), since autophagy is upregulated in many cancers and promotes survival of cancer cells in particular on advanced stages that are under metabolic stress. In addition, lysosomes have a major role in autophagy and effective lysosomal function plays a critical role in tumor invasion, resistance to apoptosis and angiogenesis. In addition to two anti-malarial drugs, chloroquine (CQ) and hydroxychloroquine (HCQ), which have already been under clinical investigation for further use in cancer therapy, Kondratskyi et al. (2017) investigated FQ, another anti-malarial agent. They reported that FQ effectively inhibits autophagy, agitates lysosomal functions, and negatively affects tumor growth in vivo. Results showed that even in extreme conditions such as starvation and hypoxia observed in advanced solid cancers, FQ negatively regulates Akt kinase and hypoxia-inducible factor-1α (HIF-1α).
Nitroxoline is a widely used antibiotic for treating urinary tract infections due to its pharmacokinetically long retention time in urine (Zhang et al., 2016). Several target-based and cell-based assays demonstrated nitroxoline as an anti-cancer agent leading to the inhibition of endothelial cell proliferation for different cancer types including lymphoma, leukemia, pancreatic cancer, ovarian cancer (Jiang et al., 2012), bladder cancer (Shim et al., 2010), and breast cancer (Sosiè et al., 2013). Furthermore, nitroxoline could potentially be useful for therapeutic development against PCa, since the drug results in AMPK-dependent inhibition of the mTOR signaling pathway and cyclin D1-Rb-Cdc25A axis, leading to apoptosis (Chang et al., 2015).
Nelfinavir is a FDA-approved human immunodeficiency virus (HIV) protease inhibitor which was used in combination therapy for HIV infected patients. Nelfinavir has also been reported to exhibit distinct anti-cancer mechanisms such as endoplasmic reticulum (ER) stress-unfolded protein response pathway and Akt inhibition in various carcinomas such as ovarian, pancreatic, and breast (Koltai, 2015; Bhattarai et al., 2016) in addition to PCa (Guan et al., 2015). However, even though nelfinavir has been investigated in phase 1/2 clinical trials for solid tumors, Kaposi’s sarcoma and others, it is yet to be evaluated for PCa in clinical trials. The mechanisms of action for anti-tumor properties of nelfinavir appear numerous including inhibition of (PI3K)/Akt signaling pathway, the proteasome and HIF-1α which inhibits angiogenesis, and induction of ER stress, autophagy, and apoptosis (Gantt et al., 2013).
Activity-Based Drug Repositioning
The need of repurposed drugs is increased to compensate the low success rate of conventional or de novo drug discovery processes. On the other hand, the availability of established drug libraries led researchers to create more rational designs compared to knowledge-based drug repositioning. The term “activity-based drug repositioning” stands for testing actual drugs in assays.
To date, besides the ones provided by commercial companies, there are two publicly available comprehensive clinical compound libraries, established through support of government and private agencies (Padhy and Gupta, 2011). The first initiative, Johns Hopkins Clinical Compound Library (JHCCL), was launched in early 2000s as a joint collaboration between Johns Hopkins Pharmacology and Johns Hopkins Bloomberg School of Public Health. JHCCL is the largest publicly accessible collection of existing drugs with approximately 3,100 available compounds, many of which are FDA-approved or approved by its foreign counterparts (Padhy and Gupta, 2011; Shim and Liu, 2014). Second collection was built by NIH Chemical Genomics Center (NCGC) and called NCGC Pharmaceutical Collection (NPC) which possess 2,500 small compounds that have been approved for clinical use by the United States (FDA), European (EMA), Japanese (NHI), and Canadian (HC) authorities (Huang et al., 2011). NPC is also comprehensive, publicly accessible collection of approved compounds along with approximately 1,000 additional investigational compounds for high-throughput screening. There are also commercially available clinical drug collections on the market. These drug collections have been recognized as valuable resources for DR purposes in many academic studies. To get a comprehensive drug library, the compounds (i.e., off-patent drugs) that are easy to obtain in terms of price and patent restrictions are generally collected first. Drugs with patent that covers drug synthesis or use will be the most difficult and expensive to obtain, concerning price per compound. Major drug metabolites should be included into collections to increase the size of the drug library, as most of them often have diverse pharmacological properties. For instance, fexofenadine, which is a non-sedating anti-histamine, lacks the cardiotoxic side effects of its parent terfenadine (Sussman et al., 1999). These comprehensive drug libraries can be used for both protein target-based and cell-based screens which shed a light on clinical trials (Shim and Liu, 2014).
Despite the great potential of the activity-based DR, even the largest publicly available drug collection, JHCCL, covers almost 30% of roughly 10,000 drugs in clinic. Though this seems a large library, it pales in comparison with the libraries of novel compounds (100,000 or more) held by pharmaceutical companies. Availability is another issue for drug screening. JHCCL shared the drug collection with over 45 researchers worldwide for drug screening against different diseases. This existing library is publicly and freely available in principle but requires financing of the replacement of drug stocks and shipping costs (Chong and Sullivan, 2007). In general, drug libraries are provided in 384-well plates and can easily be scaled up. However, unlike the other DR methods, activity-based DR requires specialized robotics to screen thousands of wells containing drugs/compounds.
There are several promising hits identified by activity-based DR including cancers, diabetes, and infectious diseases (Chong et al., 2006a,b, 2007; McMahon et al., 2007; Zhang et al., 2008; Kim et al., 2010; Shim et al., 2010; Lin et al., 2011; Platz et al., 2011). Among the hits, anti-fungal agent, itraconazole, and cardiac glycoside, digoxin are promising anti-cancer agents that have been explored through activity-based DR using JHCCL (Chong et al., 2007; Zhang et al., 2008). To date, phase 2 clinical studies evaluating the anti-tumor efficacy of itraconazole were completed for treatment of metastatic non-squamous non-small cell lung cancer (Rudin et al., 2013) and metastatic PCa (Antonarakis et al., 2013). Digoxin was found to have significant effect both at the epidemiological and experimental levels (Platz et al., 2011) and clinically trialed in PCa cases (Lin et al., 2014).
Triclosan [5-chloro-2-(2,4-dichlorophenoxy) phenol] is effective against many different bacteria as well as some fungi and protozoa. It has been widely used as a broad-spectrum anti-bacterial and anti-fungal agent for more than 30 years. It is found in formulations as diverse as cosmetics, fabrics, toothpastes, plastic kitchenware, and toys. In previous studies, triclosan demonstrated blocking effect on lipid synthesis (McMurry et al., 1998; Levy et al., 1999). Deregulated lipid metabolism and FASN inhibition has emerged as a promising therapeutic target in cancer patients. Sadowski et al. (2014) inhibited FASN and compared the cellular and molecular effects of triclosan in both malignant and non-malignant prostate cells compared to the other well-known FASN inhibitors C75 and orlistat. As a result, they proposed triclosan as a promising drug candidate against PCa with a superior cytotoxic profile in comparison with C75 or orlistat.
Clofoctol is an antibiotic used in the therapy of upper respiratory tract infections. It was previously reported to inhibit protein translation in mammalian cells and it is a potential anti-cancer drug (Pelletier et al., 2007). Wang et al. (2014) demonstrated a quite unique mechanism of action of clofoctol that inhibits prostate tumor growth as well as protein translation in PCa cells. It has indirect effect via inducing ER stress and activating unfolded protein response pathways which serve a cellular protective mechanism to cope with an excess of misfolded proteins in the ER.
In Silico Drug Repositioning
In silico drug repositioning is a potentially powerful strategy since data acquired from structural biology and omics technologies have been accumulating in the past few decades. In silico DR provides a faster repurposing process as well as reduced costs through different approaches such as molecular docking, network analysis, data mining, similarity analysis, machine learning, and transcriptional signatures (Figure 1). Different methods covered by in silico DR has been recently reviewed in detail (Vanhaelen et al., 2017).
In recent years, in silico DR methods have been focused on identification of candidate targets and potential drugs considering data from different omics levels as well as the disease-gene-drug triad (Sun et al., 2017). Publicly available clinical data at genomic and/or transcriptomic levels were evaluated within a comprehensive, systematic, and integrative analysis pipelines in several studies to find out new drug candidates for particular medical problem (Sirota et al., 2011; Jin et al., 2012; Turanli et al., 2017). Computational predictions supported by experimental assessments have been effectively used to determine new DR potentials. In contrast, powerful computational resources such as software for retrospective analysis or structural biology and maintenance of web-based databases are decisive for the collection and evaluation of the experimental data (Vanhaelen et al., 2017).
Risperidone, a benzisoxazole derivative, is an anti-psychotic agent which has proven efficacy in the treatment of most common central nervous system disorders such as depression, bipolar disorder, and schizophrenia (Roth et al., 2004). Although the drug mechanism is not clear, it has been reported that it has a high binding affinity for both dopamine D2 and serotonin 5-HT2 receptors (Abou El-Magd et al., 2010). Another molecular target of risperidone, 17-b-hydroxysteroid dehydrogenase 10 (17HSD10), plays a significant role as an intracellular binding partner for amyloid β-peptide which is important in Alzheimer’s disease. Additionally, it is suggested for treatment of PCa to stimulate dihydrotestosterone synthesis in the absence of testosterone and also highly expressed in PCa bone metastases (Ayan et al., 2012). Recently, Dilly et al. (2017) reported risperidone as a DR candidate targeting 17HSD10. They combined both activity-based and in silico DR methods through the discovery process, and then tested other similar drugs in addition to risperidone in silico, in vitro and in vivo through validation process.
Acetic acid derivative, zenarestat is an aldose reductase inhibitor originally used for preventing diabetic cataractogenesis, retinopathy, and neuropathy (Schemmel et al., 2010; Zhu, 2013). Since aldose reductases activate the transcription of NF-κB and AP-1 which transcribe the genes of inflammatory cytokines and oxidative stress-induced inflammation which promotes the formation of cancerous tumors, recent investigations strongly suggest that the use of aldose reductase inhibitors can be novel chemotherapeutic agents against cancer (Tammali et al., 2011). As an in silico DR application, the web-based DR tool, geneXpharma, has taken into consideration comprehensive gene expression profiling and the disease-gene-drug association data and repurposed zenarestat as a candidate drug to be used in PCa treatment at the point of experimental validation (Turanli et al., 2017).
As always, every computational approach has its own advantages and limits based on the problem to be solved and on the type, quality, and quantity of information available about the problem in the literature or in public/commercial databases. As an example, molecular docking requires high resolution structural information of drugs and targets (Shim and Liu, 2014). The integration of different computational methods and information coming from different sources such as biological, structural, and clinical databases could serve valuable opportunities in the manner of purposing new use of an old drug (March-Vila et al., 2017). However, none of these methods are sufficient enough to model the complex interactions among drugs, targets and diseases. Keeping this in mind, in silico drug repositioning could represent a primary method in order to highlight novel small molecules or drug compounds through initiating with a hypothesis or generating new hypotheses about how these compounds work and what their primary targets are and eventually, such hypotheses may drive the systematic design of downstream experiments. Although there are plenty of computational methods, their integration can surpass the methods applied alone.
After brief explanations of each perspective, it sounds that any of them has their own pros and cons as summarized in Table 2. Knowledge-based DR is solely dependent on researches’ knowledge and ability to interpret the scientific observations or just coincidences. It is not only a time- and cost-efficient process, but also easy to validate in pre-clinical and clinical studies if observations were experimentally investigated. On the other hand, it is not the systematic way comparing to the other DR methods, and therefore it may appear risky. In silico systems has reduced time and cost, however, it always hinges on the availability of the experimental data such as structure or gene expression profiles at first. The biological significance of the putative targets predicted by the computationally method must also be experimentally assessed. On the other hand, activity-based DR is time- and labor-consuming; requiring an entire collection of existing drugs, specialized equipment and develop a screening assay, but it can be employed without requiring structural information of target proteins or database. Moreover, activity-based DR is easy to validate since it experiences low false positive rates with regard to in silico repositioning (Shim and Liu, 2014).
Repurposed and Clinically Evaluated Non-Cancer Drugs for PCa Treatment
We briefly summarize the non-cancer approved drugs which are repurposing for PCa treatment via different DR approaches in Table 1. Moreover, chemical structures of all potential non-cancer drugs against PCa were shown in Figure 2. Here, we provide an overview only on existing drugs with anti-PCa activities that are under clinical investigation as reviewed in Table 3.
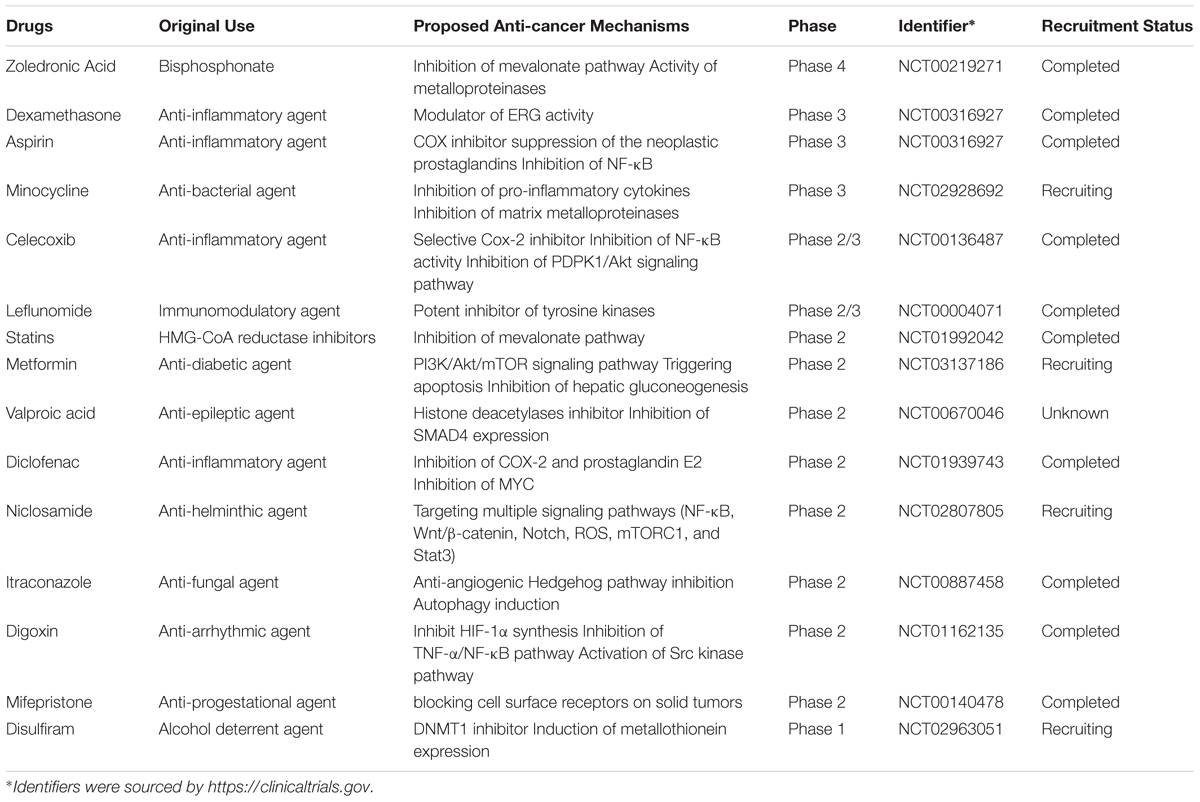
TABLE 3. Non-cancer drug repositioning candidates under clinical investigation for the treatment of prostate cancer.
Dexamethasone
Dexamethasone is one of the glucocorticoids that prevent inflammation and has immunosuppressive activity. It serves potential to treat several different diseases such as allergic disorders, inflammatory disorders (ulcerative colitis, arthritis, lupus, psoriasis), or breathing disorders (Buttgereit et al., 2011). Dexamethasone in combination with other chemotherapeutic drugs is used to counteract certain side effects of chemotherapy such as edema, emesis, etc. For instance, it is used to stabilize the development of edema in brain tumors, which could eventually compress other brain structures or spinal cords (Chu et al., 2014). The antiemetic action of dexamethasone reduces pain and the concomitant use of opioids, which in turn reduces opioid-related nausea and vomiting (Chu et al., 2014). Besides, dexamethasone can be used alone as a direct chemotherapeutic agent in certain hematological malignancies, especially in the treatment of multiple myeloma (Bhattarai et al., 2016). Although combination of dexamethasone with chemotherapeutic agents were already clinically tested in PCa patients (Shamash et al., 2011), oncogenic transcription factors were also targeted via systematic drug-transcription factor. Analysis predicts the candidate small molecules in one recent in silico DR study, and they showed the effect of dexamethasone on prostate tumor by inhibiting ERG Activity (Gayvert et al., 2016).
Aspirin
Acetylsalicylic acid or aspirin is used as an analgesic and non-steroidal anti-inflammatory drug (NSAID) in a variety of conditions ranging from headache, fever associated with the common cold, pain in rheumatoid arthritis and other similar musculoskeletal conditions (Kar, 2007).
It is known as a cyclo-oxygenase enzyme (COX-1 and 2) inhibitor with a great affinity (Yoshimura et al., 2000). To date, the NSAIDs such as aspirin, which inhibits both COX-dependent and/or independent pathways has been speculated to be used against some malignancies including colorectal, esophageal cancer, breast as well as prostate (Downer et al., 2017). Particularly, COX-2 which plays role in carcinogenesis, angiogenesis, poorer prognosis in PCa (Liu et al., 2014), and inhibition of immune surveillance (Kirschenbaum et al., 2001) is highly expressed in malignant prostate tissue compared to benign human prostate tissue. Anti-cancer mechanism of aspirin is the inhibition of COX-2 which leads to suppression of the neoplastic prostaglandins production. In addition to its classical role as COX inhibitor, it suppresses activation of NF-κB induced by TNF, upregulation of tumor suppressor gene, and downregulation of anti-apoptotic gene (Bhattarai et al., 2016).
To date, several epidemiologic studies and meta-analyses of prospective and case-control cohort studies have discussed the use of NSAID as well as aspirin in PCa cases worldwide and reviewed in detail (Fowke et al., 2009; Mahmud et al., 2010; Liu et al., 2014; Vidal et al., 2015; Bilani et al., 2017). However, results were inconsistent since some studies suggested a reduced risk of total PCa and PCa-specific mortality in association with NSAID use, some reported no benefit or elevated risk of PCa (Assayag et al., 2015). Liu et al. (2014) indicated the effect of any NSAID on PCa varied by geographic region. North American studies reported a decreased risk of PCa (Vidal et al., 2015), whereas studies from Europe found elevated risk due to the use of any NSAID. Potent effect of aspirin for PCa detection were also addressed in the context of PCa prevention (Fowke et al., 2009).
Diclofenac
Diclofenac is another anti-inflammatory and analgesic from the NSAID family and it functions as an inhibitor of COX-2 and prostaglandin E2 synthesis like aspirin. Combination or monotherapies of diclofenac have been reported to have positive effects on treating multi cancer types including ovarian cancer, colorectal cancer, neuroblastoma, and melanoma (Pantziarka et al., 2016).
High expression of MYC is characterized in many human malignancies and it leads to the upregulation of glycolytic enzymes such as glucose transporter-1 (GLUT1) and lactate dehydrogenase-A (LDHA) resulting high rates of glucose uptake and glycolysis. Therefore, MYC is a promising target for cancer therapy. COX-independent effect of diclofenac has been proposed that it represses MYC and regulates glucose metabolism via significantly decreased GLUT1 and LDHA gene expression in melanoma, PCa, leukemia cell line proliferation in vitro, and reduced melanoma growth in vivo (Gottfried et al., 2013). As a conventional COX inhibitor, diclofenac augmented the effect of RT on PCa and proposed as a potential radiosensitizer for treatment of PCa according to in vitro and in vivo outcomes (Inoue et al., 2013).
Celecoxib
Celecoxib is another anti-inflammatory drug used for the treatment of adult arthritis and unlike aspirin and diclofenac, it is a selective COX-2 inhibitor (Davies et al., 2000). Since COX-2 is expressed in the malignant epithelial cells as well as the tumor-feeding neovasculature, celecoxib is a promising candidate for treating cancer. Additionally, long-term use of NSAIDs inhibiting both COX-1 and COX-2 have been known to inhibit the production of COX-1-derived prostaglandins required for preservation of gut, kidney, and platelet functions (Leahy et al., 2002). Celecoxib have been reported not only in the treatment of human epithelial tumor types, such as colorectal, breast, and non-small cell lung cancers as well as prostate (Elder et al., 2000; Harris et al., 2000; Hsu et al., 2000), but also in the prevention of colorectal cancer in high-risk patients with familial adenomatous polyposis linked to the APC oncogene mutation (Ponder, 1997).
The main anti-cancer mechanism of celecoxib is to decrease prostaglandin production in the COX-2 expressed tumors, resulting in an inhibition of the proliferation and induction of apoptosis. Moreover, anti-cancer mechanism of celecoxib via COX-2-independent pathway has been reported such as inhibition of PDPK1/Akt signaling pathway, inhibition of NF-κB activity, alteration of APC/β-catenin pathway, and mitochondrial apoptosis pathway (Kulp et al., 2004; Patel et al., 2005; Grösch et al., 2006).
The effect of celecoxib on the expression of anti-apoptotic protein Bcl-2 as well as anti-apoptotic kinase Akt were examined. Results demonstrated inhibition of Akt activation and induced apoptosis independent from Bcl-2 via LNCaP and PC-3 cells in vitro (Hsu et al., 2000). Celecoxib and its analog that does not inhibit COX-2 were investigated in PC-3 and DU-145 in vitro and PC-3 tumor xenograft in vivo resulting inhibition of PDPK1/Akt signaling pathway (Kulp et al., 2004). Findings from in vivo and in vitro studies suggested that celecoxib has significant COX-2-independent anti-tumor properties which makes it active even in tumors that do not express significant amounts of COX-2. PCa cell growth was inhibited by inducing a G1 cell cycle block and reducing DNA synthesis (Patel et al., 2005). Recently, celecoxib and zoledronic acid were considered complementary targeting clinically later-stage disease (Mason et al., 2017).
Minocycline
Minocycline is a semi-synthetic tetracycline derivative that maintains the efficacy against both Gram-positive and Gram-negative bacteria. It has been approved by FDA, more than 30 years ago, for the treatment of acne and some sexually transmitted diseases. Subsequently, experimental models revealed non-antibiotic properties of minocycline and determined minocycline beneficial against various disorders with an inflammatory basis such as dermatitis, periodontitis, atherosclerosis, and autoimmune diseases such as rheumatoid arthritis, inflammatory bowel disease (Garrido-Mesa et al., 2013).
Minocycline inhibits the action of proinflammatory cytokines and matrix metalloproteinases (MMPs) which are extracellular matrix-degrading enzymes implicated in cancer invasion and metastasis. Up until now, the drug presents anti-cancer activities against various cancer cell lines, such as ovarian cancer, glioma, PCa, melanoma, and breast cancers (Lokeshwar, 2011; Ataie-Kachoie et al., 2013; Garrido-Mesa et al., 2013). Moreover, minocycline has been in phase 2 of clinical trials for different carcinomas such as myeloma, esophageal, pancreatic, colorectal, lung, head, and neck cancers (Bhattarai et al., 2016) whereas in phase 3 of clinical trials for PCa.
Lokeshwar (2011) investigated doxycycline, minocycline, and chemically modified tetracycline (CMT) analogs for treatment of PCa. Some of these CMTs, notably, CMT-3 and CMT-308, were found significantly more potent than doxycycline or minocycline in inhibiting tumor cell-derived MMPs and inducing apoptosis in vitro and in vivo. In another study, pharmacokinetic interactions for minocycline with retinoic acid metabolism were investigated in various PCa cell lines (Regen et al., 2014).
Niclosamide
Niclosamide, the salicyclamide derivative, is an oral anti-helminthic drug to treat tapeworm infections. In addition to be used as an anti-infective agent afterward, it has been demonstrated to exhibit anti-cancer activity in several cancers such as colorectal cancer (Sack et al., 2011), ovarian cancer (Yo et al., 2012), acute myeloid leukemia (AML) (Jin et al., 2012), and breast cancer (Wang et al., 2013). Niclosamide targets multiple signaling pathways which are closely involved with oncogenesis and oncoprogression such as NF-κB, Wnt/β-catenin, Notch, and mTORC1 (Pan et al., 2012).
Niclosamide targets the Wnt co-receptor LRP6 on the cell surface and that it is potent molecule as a Wnt/β-catenin signaling and anti-cancer agent for human prostate and breast cancer due to in vitro studies (Lu et al., 2011). Androgen receptor (AR) variants that drives resistance to chemotherapeutics such as enzalutamide are another problem that faced through PCa treatment. Therefore, niclosamide was evaluated as potential inhibitor of variant alternatively spliced AR (AR-V7) and preclinical validation proved that it is promising to treat, either alone or in combination with current anti-androgen therapies in advanced PCa patients (Liu et al., 2014).
Itraconazole
Itraconazole was a common triazole anti-fungal agent and used to inhibit the cytochrome P450-dependent lanosterol 14α demethylase (14DM). The drug causes the destruction of the fungal membrane and reduction of ergosterol synthesis. However, the anti-fungal effect of itraconazole is unlikely to be associated with its potential anti-cancer activities. It can reverse chemoresistance mediated by P-glycoprotein, adjust the signal transduction pathways of Hedgehog and Wnt/β-catenin and inhibit angiogenesis (Kim et al., 2010; Tsubamoto et al., 2017). The benefits of itraconazole monotherapy for PCa and basal cell carcinoma were proposed in clinical trials as well as in vitro studies. Besides, combination of itraconazole and chemotherapy showed the better survival rates in various cancer types such as pancreatic, ovarian, and triple negative breast via reducing endothelial cell proliferation and migration (Chong et al., 2007; Aftab et al., 2011; Tsubamoto et al., 2017). Several possible multi-drug combinations have been outlined and are waiting for clinical trials whereas some of these are on-going. Itracanozole is addressed as promising drug candidate for rapid implementation of clinical trials in rare cancers including medulloblastoma, or certain types of sarcomas (rhabdomyosarcoma, chondrosarcoma, and osteosarcoma) in which the Hedgehog pathway has an important role (Pantziarka et al., 2015).
Xu et al. (2010) suggested that itraconazole inhibits the mTOR and VEGFR2 signaling pathways in endothelial cells by damaging cholesterol trafficking. Afterward, phase 2 study evaluating the anti-tumor efficacy of oral itraconazole in men were completed for treatment of metastatic CRPC. They suggested that high-dose itraconazole (600 mg/day) might have modest anti-cancer activity associated with Hedgehog pathway suppression (Antonarakis et al., 2013).
Digoxin
Digoxin, as a cardiac glycoside, has basically steroid-like structure including an unsaturated lactone ring and sugar moieties. It affects cardiac contractility through highly specific inhibition of the Na+/K+-ATPase pump. Anti-cancer activity of digoxin may include intracellular decreased level of K+ and increased level of Na+ and Ca2+ and also inhibits DNA topoisomerase II, IL-8 production, HIF-1α synthesis, and the TNF-α/NF-κB pathway and activate the Src kinase pathway (Slingerland et al., 2013; Calderón-Montaño et al., 2014). Digoxin and its derivatives have been investigated via preclinical studies in several cancer types comprising lung, pancreatic, melanoma, leukemia, renal carcinoma, and the most revised indications were on breast and PCa (Bhattarai et al., 2016).
After an activity-based DR study in which JHCCL was used, 20 drugs were highlighted whereas 2 of them were known HIF-1 inhibitors (rapamycin and roteone) and 11 of these 20 drugs were cardiac glycosides including digoxin, digitoxin, ouabain, etc. As a finding of this study, digoxin treatment increased latency and decreased tumor growth in mice. Digoxin was first reported as an inhibitor of HIF-1α protein and HIF-1 targets such as VEGF, GLUT1, HK1, and HK2 for PCa (Zhang et al., 2008). On the contrary, Lopez-Lazaro (2009) argued that neither digoxin has any inhibitory effect on HIF-1 at therapeutic concentrations, nor the anti-cancer effects observed in mice are relevant in humans. As a result of in vitro drug screening using the same drug library, digoxin has emerged again with inhibitory effect on PCa. As a following study, evaluation of a large-scale cohort study with male patients who had heart diseases and took digoxin over 5 years were indicated significantly reduced incidence among PCa patients who have used it compared with the control group (Platz et al., 2011).
Valproic Acid
The short chain fatty acid, valproic acid (VPA), is an inhibitor of class I histone deacetylases (HDAC) used to treat epilepsy, bipolar disorders, migraine, and schizophrenia. Approximately 20 years ago, the first clinical trial discussing VPA was initiated and then, several clinical trials for different leukemia, and solid tumor entities were followed. In addition to clinical assessments, VPA is still under experimental investigation regarding the numerous mechanisms of anti-cancer activity such as inhibition of tumor-associated inflammation, cancer cell proliferation, tumor angiogenesis, and cancer cell invasion and migration resulting with metastasis and lastly, inhibition of histone deacetylases which plays a central role. Since DNA-region of key anti-cancer genes are tightly bound via histone deacetylase, VPA, as a HDAC inhibitor, allocates silenced genes to be initiated (Michaelis et al., 2007).
Acute and chronic administration of VPA were investigated for PCa cells irrelevant to their androgen sensitivity and also in vivo tumor xenograft models. Acute treatment increased net histone H3 acetylation and resulted with upregulated expression of p21, AR, and cytosolic prostate-specific antigen (PSA) where the effects on AR and PSA were reversed for chronically administered VPA in which the cancer cell proliferation rate was decreased due to increased caspase-2/caspase-3 activation and also demonstrated statistically significant reduction of tumor xenograft growth in vivo (Xia et al., 2006). In phase 2 trial to treat patients with castration-resistant PCa (CRPC), oral VPA was used with biomarker assessment strategy to study relationship among PSA, serum testosterone, VPA blood levels, and histone acetylation. They exerted toxicities regarding oral use of VPA in the treatment of CRPC patients (Sharma et al., 2008). Lee and Kim (2015) exerted that VPA is more effective on cell viability and invasion in metastatic prostate cell line (PC-3) by upregulating the metastasis suppressor protein NDRG1. VPA is used for the monotherapy of some cancers such as metastatic neuroendocrine carcinomas and myeloid malignancies, however, the most of potent anti-cancer activity of VPA is based on combination with other epigenetic modifiers (azacytidine, hydralazine), combinations with cytotoxic chemotherapy agents (5-FU, epirubicin, cyclophosphamide, doxorubicin, karenitecin), and combinations with immune-modulators (Brodie and Brandes, 2014). In another study, VPA inhibited the expression of SMAD4 which plays an important role in cancer metastasis via EMT along with upregulated the expression of miR-34a in prostate carcinoma (Xia et al., 2016). Very recently, the combination of metformin and VPA were reported to induce synergistic apoptosis in the presence of p53 and androgen signaling; inhibited proliferation of LNCaP and PC-3 cell lines and killed more PCa cells than either drug alone (Tran et al., 2017).
Statins
Statins are lipid-lowering agents that act by inhibiting 3-hydroxy-3-methylglutaryl (HMG-CoA) reductase resulting with inhibition of the mevalonate pathway. Statins reduce cholesterol levels and are mainly used for the treatment of hypercholesterolemia as well as atherosclerotic plaques causing cardiovascular problems, such as myocardial infarction, stroke, and atrial fibrillation (Hindler, 2006). The emerging interests in the use of statins as anti-neoplastic agents are based on both in vitro and in vivo studies demonstrating anti-proliferative, anti-metastatic, RT-sensitizing, and apoptosis inducing properties (Chan et al., 2003). Preclinical evidences show that statins inhibit tumor growth and induce apoptosis in melanoma, glioma, neuroblastoma, and leukemia cell lines whereas there are numerous clinical trials that have considered anti-cancer activity of statins in various cancer types such as liver, gastric, acute myeloid leukemia, and squamous cell carcinoma of the cervix and of the head and neck (Chan et al., 2003; Hindler, 2006).
Statin use for the treatment of PCa has been associated with decreased risk of PCa, better prognosis, reduced volume of PCa and PSA levels, along with its protective effect against PCa regarding a retrospective cohort study of men who underwent prostate biopsy (Tan et al., 2011). Numerous studies were in harmony about the lowering PSA effect of statins. Since increased levels of PSA are a common symptom guiding biopsy, reduced PSA levels of statin users were debated in manner of PCa detection in men. Tan et al. (2011) reported no differences on PSA levels of PCa diagnosed patients regardless of statin use and suggested that statin reduces PSA in benign tissue but not in malignant tissues. Additionally, some studies have demonstrated a beneficial effect of statins in reducing PCa related mortality as well as overall PCa risk (Katz et al., 2010; Marcella et al., 2012) whereas other studies have not revealed a significant effect (Caon et al., 2014). Recently, conflicting data sourced from clinical outcomes obstruct the integration of neither beneficial nor harmful associations between the statin use and PCa (Hutchinson and Marignol, 2017).
Mifepristone
Mifepristone (RU-486), a steroid synthesized in the laboratory, is the first anti-progesterone drug with high affinity for the progesterone receptor (PR) and used for termination of early pregnancies. Since progesterone is crucial for the initiation and maintenance of pregnancy, PR also plays an important role in the human reproductive system (Hazra and Pore, 2001).
Due to knowledge of the overabundance of PR in solid tumors, anti-progesterone drugs have been purposed for tumor growth suppression via blocking cell surface receptors, such as PR as well as glucocorticoid receptors (GR) and ERs (Yu et al., 2015). Cancer cell lines from several tumors such as glioma, breast, prostate, ovary, cervix, and osteoblastoma have been treated with mifepristone and the inhibition of the cancer cell growth regardless of reproductive or non-reproductive origin have been reported (Tieszen et al., 2011).
To the best of our knowledge, one of the earliest studies on anti-progesterone administration to PCa reported in vivo suppression of the growth of the androgen-insensitive prostatic carcinoma within combined estrogen therapy (Mobbs and Johnson, 1991). Later, considerable anti-tumor activity of mifepristone was presented in both androgen-sensitive and androgen-insensitive variants of the LNCaP via mouse models (El Etreby et al., 2000). In addition, mifepristone was also assessed in patients with CRPC through phase 2 study. The combination therapy with promising agents such as corticosteroids, ketoconazole, or 5-reductase inhibitors might be used to block the compensatory rise in adrenal androgens and be effective in patients with CRPC (Taplin et al., 2008).
Disulfiram
Disulfiram is the first FDA approved alcohol aversive drug used for the treatment of alcohol dependence for more than 60 years. It inhibits the aldehyde dehydrogenase (ALDH) leading to accumulation of acetaldehyde in blood (Kalra et al., 2014). As a result of high-throughput cell-based drug screening, disulfiram was found as an anti-cancer drug regarding to reduce tumor growth in vivo and induced metallothionein expression. Additionally, the effect of the drug was enhanced by copper in vitro (Iljin et al., 2009). To date, numerous clinical trial studies on administration of disulfiram were carried out to treat various cancers such as breast, pancreas, prostate, brain, lung, melanoma as well as metastatic types of some of them.
Disulfiram as acting like a DNA methyltransferase (DNMT1) inhibitor provide advantage by restoring tumor suppressor genes and leading DNA demethylation in PCa cells. It has been linked to reductions in 5-methyl cytosine (5meC) content and decreased methylation in APC and RARB gene promoters (Lin et al., 2011). As follows, disulfiram has been assessed as a potential epigenetic therapy, a pilot trial (NCT01118741) was carried out to evaluate the effect of disulfiram on demethylating changes with biochemically recurrent PCa via quantifying changes in 5meC content in peripheral blood mononuclear cells (PBMC) (Schweizer et al., 2013).
Disulfiram treatment alone showed only minimal effects in castration-resistant PCa xenografts, however, drug supplemented with Cu2+ significantly reduced tumor growth (Safi et al., 2014). Very recently, Skrott et al. (2017) reported that the ditiocarb (disulfiram metabolite) and copper complex has anti-cancer effects in vitro and in vivo. Moreover, NPL4 protein has been proposed a molecular target which is involved in protein turnover of tumorigenesis promoting stress-response pathways.
Leflunomide
Leflunomide is a selective inhibitor of the rate limiting step in the de novo synthesis of pyrimidines. Teriflunomide (A77 1726) is the active metabolite of leflunomide which suppress dihydroorotate dehydrogenase (DHODH) activity as well as IL-1 and TNF-α. It is used mainly for rheumatoid arthritis due to its anti-inflammatory effects (Breedveld and Dayer, 2000).
Additionally, leflunomide has been suggested as a potent inhibitor of tyrosine kinases and some growth factor receptors such as EGFR, FGFR, and PDGFR. Since their activation is associated with numerous cancer types, leflunomide has the potential to be used as an anti-cancer agent (Gupta et al., 2013). One of the earliest studies in which suggested anti-tumor activity of leflunomide showed the inhibition of cancer cells including glioma, ovarian, and prostate which express PDGFR in contrast to cancer cell cultures which only express EGFR (Shawver et al., 1997). Similarly, the active metabolite of leflunomide, teriflunomide, was found more effective at inhibiting the tyrosine kinase activity of PDGFR than that of EGFR and had no effect on the FGFR (Xu et al., 1999).
In a phase 2 trial with leflunomide against hormone resistant PCa, PDGFR expression was noticed in more than 80% of both metastases and primary PCas. Monotherapy of leflunomide demonstrated PSA reduction of more than 50% of patients and also stated significant improvement of pain (Ko et al., 2001). In another study, preliminary results represented chemopreventative effects of leflunomide in PCa (Hail et al., 2010). Mono- and combination therapy of leflunomide is in phase 2/3 of clinical stage for PCa and brain and central nervous system.
Metformin
Metformin is an anti-diabetic agent primarily being used in type 2 diabetes and increasingly in polycystic ovary syndrome treatment. The direct effect of metformin at the molecular level is to activate AMPK through a tumor suppressor, LKB-1, resulting in decreased glucose level in blood. Moreover, AMPK inhibits mTOR which is key mediator of frequently deregulated PI3K/Akt signaling pathway in malignant cells and activates tumor suppressor p53 leading to cancer cell apoptosis. Indirect effect of the drug is inhibition of hepatic gluconeogenesis and reduces insulin resistance in peripheral tissue. Since tumor cells express high levels of the insulin receptor, reduced insulin levels associated with metformin use provide better prognosis for a number of cancers, including breast, colon, and PCa (Evans et al., 2005; Whitburn et al., 2017). Metformin were reported as an anti-cancer agent against breast, prostate, lung, cervix, and ovarian cancer cells, up to the date. It is also in phase 1–4 clinical trials for various cancers such as lung, prostate, breast, liver, pancreatic, and brain (Jalving et al., 2010).
First epidemiological studies demonstrated metformin as a potent anti-cancer agent in reducing cancer incidence and mortality considering numerous anti-neoplastic biological effects via a range of molecular mechanisms. Some studies supported the oncogenic benefit of metformin for PCa patients regardless their diabetic status (Noto et al., 2012), some of them emphasized heterogeneous and biased results or no significant effects on incidence of PCa (Margel et al., 2013). Since there are plenty of investigations, the current evidences were recently summarized a role for metformin in PCa therapy (Whitburn et al., 2017). Besides, Electronic Health Records were assessed the use of metformin and suggested an association with decreased mortality after a cancer diagnosis compared with diabetic and non- diabetic cancer patients (Xu et al., 2015).
Every single anti-diabetic drug does not show the similar tendency as an anti-cancer agent. The use of metformin was linked with reduced risk of PCa diagnosis, whereas other oral diabetic medications had no effect (Preston et al., 2014). Combination therapy was also another option to use metformin in cancer treatment. Hirsch et al. (2013) showed that combination of metformin with chemotherapy might obstruct tumor growth and prevent recurrence of PCa cells associated with blocking inflammatory pathways in xenograft models. Recent study showed that metformin and VPA synergistically repressed the proliferation in both LNCaP and PC-3. The combination therapy was also found to cause increased apoptosis in patient-derived prostate tumor explants. Concisely, two drugs have been suggested that working synergistically to destroy more PCa cells than either monotherapies alone (Tran et al., 2017).
Zoledronic Acid
Zoledronic acid (ZA) is an aminobisphosphonate with high affinity for bone mineral and approved for the treatment of bone related diseases such as osteoporosis. When it is administered intravenously, it quickly localizes to bone and inhibits osteoclastic bone resorption by negative regulation of the action of the farnesyl pyrophosphate synthase enzyme in the mevalonate pathway (Lewiecki, 2009). Zoledronic acid is the first bisphosphonate with displayed efficacy in the treatment of bone metastases of patients with a broad range of tumor types including breast, prostate, and lung and in multiple myeloma as well as hypercalcemia of malignancy. It also reduces incidence of skeletal relevant complications and palliate the pain in patients with bone metastasis (Perry and Figgitt, 2004). In addition to other cancer types, there are more than 50 phase 4 clinical trials which are completed for PCa and bone metastasis according to https://clinicaltrials.gov.
The anti-cancer mechanisms of ZA may comprise apoptosis associated with the mevalonate pathway, activation of caspases, effects on invasiveness of the target cells, and arbitrated by changes in expression and activity of metalloproteinases (Corey et al., 2003). Considering PCa, ZA effectively prevents both bone loss in patients with locally advanced disease receiving androgen deprivation therapy and skeletal complications in men with hormone-refractory metastatic disease. Moreover, it assumed gold standard medication for the prevention and treatment of skeletal complications in patients with bone metastases due to all solid tumors with an acceptable safety profile and tolerability (Polascik and Mouraviev, 2008).
The ZA has known anti-PCa effects, demonstrated both preclinically with in vitro and in vivo studies (Corey et al., 2003) and clinically through placebo-controlled trials and long-term efficacy for the prevention of skeletal complications in later-stage disease (Saad et al., 2002, 2004). Combination therapy ZA with docetaxel demonstrated major improvements in survival of PCa patients (James et al., 2016), and recently, COX-2 inhibitors such as celecoxib and ZA therapy considered complementary for the treatment of advanced or metastatic PCa but findings showed no overall evidence of improved survival within the combinatorial therapy (Mason et al., 2017). ZA is generally used every 3 weeks based on initial clinical trials, however, the current evidences suggested similar outcomes with a reasonable biochemical response when ZA is used every 12 weeks in men with bone metastases from CRPC. Less frequent treatments may release the side effects, inconvenience, and cost (Niraula et al., 2018).
Systems Biology Perspective in Drug Discovery for Prostate Cancer
The preliminary drug targeting approaches were correlating diseases with the precise genetic malfunctions and subjected to discover only the drugs that modulate these gene targets. This reductionist point of view has not been totally successful since the biology behind diseases is complex and diseases are rarely up to single protein or gene (Schneider and Klabunde, 2013). In this manner, systems biology as a holistic approach can handle abundancy of high-throughput data from diverse omics techniques and other sources as well as their processes via mathematical modeling, and computational analysis tools (Figure 3A) (Mardinoglu and Nielsen, 2015; Zhang and Hua, 2016; Benfeitas et al., 2017; Mardinoglu et al., 2018a).
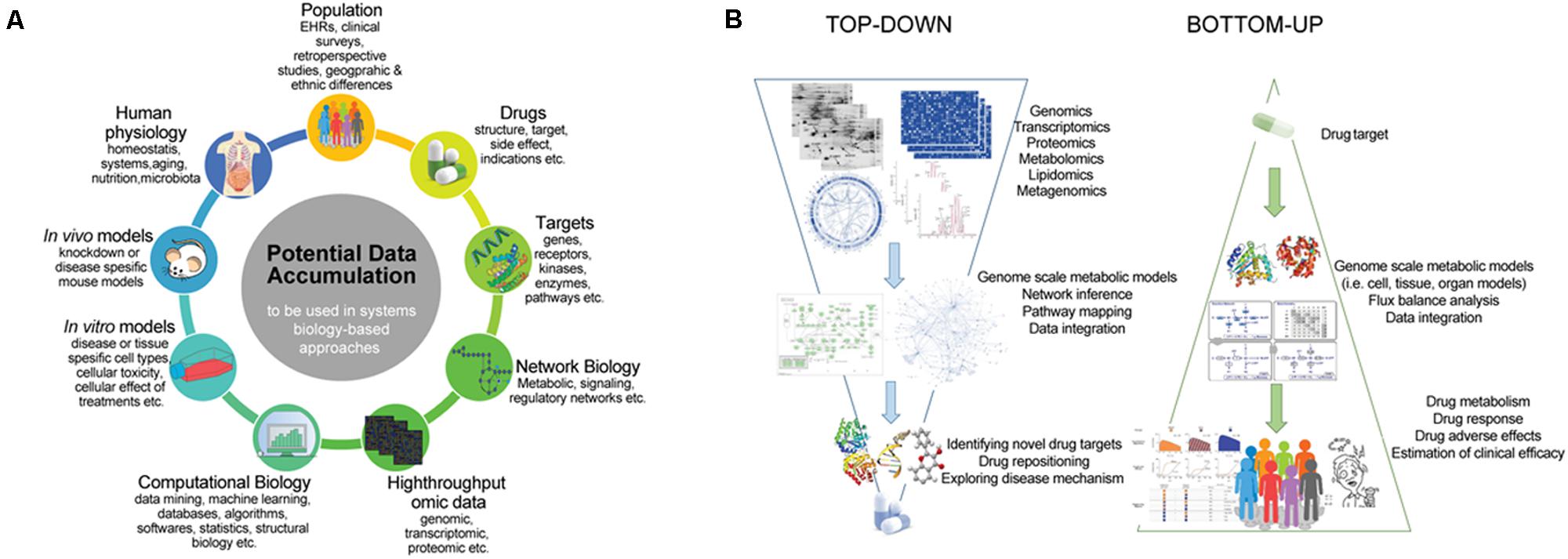
FIGURE 3. The use of system biology in the drug repositioning. (A) Potential data accumulation to be used in systems biology based approaches. (B) The application of two fundamental approaches in systems biology for drug design and discovery.
To date, systems biology approaches have been applied to decipher the disease mechanism and therapy options (Lee et al., 2016, 2017; Mardinoglu et al., 2017, 2018b). As an example, network biology approach called mode-of-action by network identification was used to identify the mediator genes and pathways in both primary and metastatic prostate tumors (Ergün et al., 2007). Besides, regulatory modular networks based on disease-specific miRNA-mRNA correlation were constructed to explore substantial variation across primary and metastatic prostate tumors (Zhang et al., 2012). Systems biology approaches also enable to discover potent biomarkers for predicting PCa outcomes as Ummanni et al. (2011) revealed novel protein biomarkers such as eIF4A3, DDAH1, ARG2, Prdx3, and Prdx4 associated with altered proteomic expression in PCa compared to benign prostate. There are also integrative studies combining multi-platform and multi-dimensional datasets. Comprehensive study utilizing different omics data from miRNA, mRNA, and protein expressions to copy number alterations was used for the effective stratification of patients with primary prostate tumor (Abeshouse et al., 2015). In another study, habitual genomics and transcriptomics data were integrated with phosphoproteomic to identify significant kinase signaling networks in advanced PCa (Tan et al., 2016).
The two fundamental approaches of systems biology, the “top-down” and “bottom-up” methods, are also applicable to the drug design and discovery in addition to other application such as network biology, biomarker discovery, etc. As pointed out in Figure 3B, top-down systems biology approaches aim to analyze high-throughput omics datasets to elucidate networks and logic behind the biological phenomena. From the drug discovery perspective, top-down methods provide detailed understanding of the causes of disease via perturbed networks and identifying reporter signatures as candidate biomarkers or drug targets. As an example of top-down methods, Jin et al. (2012) used transcriptional responses in PC-3 cells as well as MCF7 and HL60 before and after drug treatment. They developed drug off-target-based hybrid method that combines Bayesian factor regression model and network component called cancer-signaling bridges to analyze transcriptome data associated with drug usage. On the other hand, bottom-up methods object to simulate nature by utilizing known parameters and generating models of living cells. Each individual components of a system are gathered into a generated network to chase dynamic properties at the system level. Different computational modeling methods such as ODE-based, logic-based, or stoichiometric modeling can be applicable to investigate drug mechanism, its responses, and side effects. Gallaher et al. (2014) designed a computational model to predict patient outcome due to therapy response. They highlighted that such models and/or genetic algorithms are particularly useful for oncologists to predict the therapy response or drug combination therapies for effective treatment and cure of metastatic castrate resistant PCa patients.
Since the metabolic changes are quite important to detect and treat PCa regardless the disease stage (Eidelman et al., 2017), genome-scale metabolic models (GEMs) might be a very helpful source to create and/or test the hypothesis for drug repositioning in addition to elucidation of physiological mechanisms or side effects (Mardinoglu and Nielsen, 2012; Zhang and Hua, 2016) so GEMs can be used as a tool in both “top-down” and “bottom-up” methods in the context of drug discovery. GEMs have been employed for studying cancer metabolism utilizing either generic/personalized or tumor/cell-specific which may translate into clinically relevant applications. They can also be used to identify drug targets leading inhibition of cancer-related phenotypes or drug resistance in cancer therapy. Furthermore, the fortification of GEMs can be obtained via integration of omics data like genomic, transcriptomic, and proteomic as well as incorporation of regulatory molecules to the metabolism (Lee et al., 2018). GEMs also provide valuable insight on the interaction between cancer cells and supporting cells in their niches as paving the way for whole-cell modeling (Ghaffari et al., 2015; Yizhak et al., 2015). There are valuable GEMs studies for drug-related purposes. Metabolic transformation algorithm (MTA) was purposed to search for drug targets that could restore the metabolism within the cell from the disease state to the healthy state (Yizhak et al., 2013). Agren et al. (2014) revised the INIT algorithm to tINIT and used this for reconstruction of personalized GEMs for HCC patients and 83 healthy cell types via integration of proteomics data and HMR 2.0 (Mardinoglu et al., 2014). Reconstructed personalized GEMs were used to identify selective anti-metabolites for cancer therapy. GEMs were also used for predicting drug side effects and the identification of key metabolic reactions and biomarkers via implementation of model-based phenotype predictor approach that leverages medical informatics resources and GEMs (Shaked et al., 2016). PCa-specific generic GEM (Agren et al., 2012) and personalized GEMs for PCa patients (Uhlen et al., 2017) have been reconstructed for revealing the molecular mechanism of the tumor progression. They developed INIT algorithm and used HMR 2.0 to reconstruct cell type–specific metabolic models with information on protein abundances from the Human Proteome Atlas. Very recently, GEMs for two clonal subpopulations from PC-3 cell line were modeled to explore their metabolic differences. Highly invasive, low metastatic PC-3/S, and highly metastatic, low invasive PC-3/M cells present the opposite phenotype. Addition to unveiling key metabolic nodes related to tumor heterogeneity, results also highlighted potential subpopulation-specific targets with potential therapeutic implications. Such model for PC-3/M cells showed sensitivity to etomoxir, an inhibitor of long-chain fatty acid transport to the mitochondria (Marin de Mas et al., 2018).
Future Directions
Known non-cancer drugs with new therapeutic applications in oncology has been reviewed in detail comprising drug mode of actions, rationale behind the approaches, practical evidences such as preclinical or clinical studies, and opportunities for repurposing drugs as anti-cancer agents (Gupta et al., 2013; Shim and Liu, 2014; Bhattarai et al., 2016; Würth et al., 2016).
Drugs from knowledge- and activity-based methods were quite more applied than in silico methods. Since the available drug libraries were already tested for PCa and there is still the need of expanded new drug libraries, these methods could be in bottleneck, however, in silico methods were not elaborated, yet but showed high potential for validation of drugs as well as discovery process which might be achieved via computational methods. Several studies have been discussing in silico methods and their integrations in detail (Jiao et al., 2015; Hodos et al., 2016; March-Vila et al., 2017; Vanhaelen et al., 2017).
Gut microbiota has a crucial role in drug efficacy both directly and indirectly. Hence, diet has also enormous influence on the gut microbiota, pioneering computational models comprising xenobiotic metabolism and microbial enzymes (Shoaie et al., 2015) should be constructed for integrating diet-microbiota-drug interactions and evaluated in the context of PCa treatment (Thiele et al., 2017).
Current standard treatment strategy for localized PCa consists of prostatectomy, RT supplemented with hormone therapy. However, recurrence after surgery or formation of castration-resistant PCa after hormone therapy is common. Chemotherapy has merely moderate effect for the treatment of metastatic tumor. Most of the drugs (repurposed or already used in clinic) target growth factors and/or their receptors, which are abundant in tumors. Some of them modulate survival, angiogenesis, or downstream signaling pathways. In general, researchers focused on metastatic PCa more than primary tumor because of high mortality and poor survival rates. However, primary prostate tumor with intermediate or high-risk score cause higher mortality regardless of initial therapy (Fu et al., 2012).
The most lethal form of PCa is considered as metastatic castration-resistant PCa whereas it is progressed form of primary tumors. Therefore, effective treatment strategies on prostate primary tumors are as important as on metastatic ones. The use of GEMs to detect metabolic alterations and their drug targets is already discussed in this review. However, there is a need for comprehensive model which presents not only metastatic PCa but also primary PCa. On the other hand, omics data integration is a significant value for a metabolic model in which generally transcriptomics and proteomics have utilized mainly. Whether GEMs are deliberated as generic disease–specific model, it is also important to represent each individual difference inside. Considering key stones, GEMs should be more evaluated for drug repositioning in the future.
Although DR is generally emphasized within advantages, many challenges deserve attention. Not all approved non-cancer drugs should be tested for cancer treatment without valid molecular insights into their potent efficacies. There are also several studies that take into account abandoned drugs for repurposing, however, extra care in selecting abandoned drugs is recommended regarding insufficient pharmacokinetic and pharmacodynamic data. Another point is uncertainties whether drug doses, formulations, and routes of administration for the original indication are similar to the need of the anti-cancer indication. Although approved drug acquired via DR methods do not work as expected, structures and targets of the approved drugs can also help in designing improved drug with new properties such as better water solubility, increased selectivity, long retention time, etc.
Although DR should significantly reduce the time and cost, considering the obstacles associated with phase 2 and 3 clinical trials, failure in these phases cannot be reduced by DR. Much money, larger number of patients and longer time is still needed after phase 2 of clinical trial with existing failure chance, unfortunately. Intellectual property right and regulatory standards comprising the discussions on off-patent drugs and their mechanism of action (MOU) patents are another issue considered for the commercialization of new cancer indication of an already approved drug as previously mentioned (Shim and Liu, 2014; Jiao et al., 2015; Bhattarai et al., 2016).
As we have mentioned in the study, North American studies reported a decreased risk of PCa (Vidal et al., 2015), whereas studies from Europe found elevated risk due to the use of any NSAID. Therefore, population and variations based on geographic region could be another challenge for DR. However, particularly in silico DR methodologies based on signature matching techniques might give expression signatures for each individual and expectedly translate into a personalized DR (Li and Jones, 2012; Hodos et al., 2016).
The DR provide significant opportunities to establish novel collaborations between academia and pharmaceutical industry. Moreover, there are some recent examples as mentioned previously. The National Institutes of Health has collaborated with pharmaceutical companies to investigate almost 60 abandoned drugs for new clinical purposes. Likewise, the United Kingdom Medical Research Council is making million dollars investments to the United Kingdom researchers for investigation of 22 abandoned compounds (Gupta et al., 2013).
Conclusion
In this review, we highlighted repurposed non-cancer drugs and their mechanisms to treat PCa through diverse perspectives. Among these repurposed drugs, 10 drugs were obtained through different drug repositioning approaches as we categorized in 3: knowledge-based, activity-based, and in silico DR methods (Table 1). Most of them have shown anti-cancer activity only in preclinical studies. However, these observations will translate into the clinic remains to be seen. Another 14 drugs and 1 drug family (statins) were discussed in detail with a particular emphasis on non-cancer drugs under clinical investigation for PCa, up to the date (Table 3). Since drugs summarized in Table 3 have already completed several phases of clinical trials, the known safety profiles of these drugs decrease their chances of failure to become an approved drug.
Mono- or combination therapy of these medications have shown anti-cancer and palliative activities in cancer patients. To the best of our knowledge, there are a few drugs such as thalidomide, celecoxib, methotrexate which have been approved for treatment of cancer patients, in addition to their original uses. However, zoledronic acid is the only repurposed and approved non-cancer drug for PCa patients this far. Anti-cancer activities of existing drugs shown in this study cover diverse mechanisms such as inhibition of mTOR and VEGFR2 signaling, PI3K/Akt signaling inhibition, COX and selective COX-2 inhibition, NF-κB inhibition, Wnt/β-Catenin pathway inhibition, DNMT1 inhibition, GSK-3β inhibition, suppression of anti-apoptotic proteins, tyrosine kinases and some growth factor inhibition, HIF-1α suppression, tumor angiogenesis reduction, and matrix metalloproteinases enzyme inhibition to resist invasion and metastasis (Figure 4).
Most of the repurposing studies summarized in this study were targeting metastatic or advanced PCa cases in which mortality rates were high and hormone therapy as far as medications do not help. However, treatment of primary tumors and prevention of the disease should also be considered in the context of drug repositioning. We anticipate that this review article will not only shed a light on current repurposed drugs but also help researchers in repurposing more therapeutics from already approved non-cancer drugs in the future.
Author Contributions
BT wrote the manuscript. AM and KA conceived the study. All authors contributed to the manuscript during the progress of the work.
Funding
This work was supported by TUBITAK, project numbers 2214/A-1059B141600581 and 117S489, and funded by Knut and Alice Wallenberg Foundation.
Conflict of Interest Statement
The authors declare that the research was conducted in the absence of any commercial or financial relationships that could be construed as a potential conflict of interest.
Acknowledgments
Even though we aimed to be comprehensive, we sincerely apologize to our colleagues whose work could not be cited because of space considerations.
Abbreviations
AR, androgen receptor; CMT, chemically modified tetracycline; CRPC, castration-resistant prostate cancer; DR, drug repositioning; EMT, epithelial-to-mesenchymal transition; ER, endoplasmic reticulum; HDAC, histone deacetylases; HMG-CoA, 3-hydroxy-3-methylglutaryl; JHCCL, Johns Hopkins Clinical Compound Library; MMP, matrix metalloproteinases; NCGC, National Health Institute Chemical Genomics Center; NPC, NCGC pharmaceutical collection; NSAID, non-steroidal anti-inflammatory drug; PCa, prostate cancer; PSA, prostate-specific antigen; VPA, valproic acid; ZA, zoledronic acid.
References
Abeshouse, A., Ahn, J., Akbani, R., Ally, A., Amin, S., Andry, C. D., et al. (2015). The molecular taxonomy of primary prostate cancer. Cell 163, 1011–1025. doi: 10.1016/j.cell.2015.10.025
Abou El-Magd, R., Park, H., Kawazoe, T., Iwana, S., Ono, K., Chung, S., et al. (2010). The effect of risperidone on D-amino acid oxidase activity as a hypothesis for a novel mechanism of action in the treatment of schizophrenia. J. Psychopharmacol. 24, 1055–1067. doi: 10.1177/0269881109102644
Aftab, B. T., Dobromilskaya, I., Liu, J. O., and Rudin, C. M. (2011). Itraconazole inhibits angiogenesis and tumor growth in non-small cell lung cancer. Cancer Res. 71, 6764–6772. doi: 10.1158/0008-5472.CAN-11-0691
Agren, R., Bordel, S., Mardinoglu, A., Pornputtapong, N., Nookaew, I., and Nielsen, J. (2012). Reconstruction of genome-scale active metabolic networks for 69 human cell types and 16 cancer types using INIT. PLoS Comput. Biol. 8:e1002518. doi: 10.1371/journal.pcbi.1002518
Agren, R., Mardinoglu, A., Asplund, A., Kampf, C., Uhlen, M., and Nielsen, J. (2014). Identification of anticancer drugs for hepatocellular carcinoma through personalized genome-scale metabolic modeling. Mol. Syst. Biol. 10:721. doi: 10.1002/msb.145122
Antonarakis, E. S., Heath, E. I., Smith, D. C., Rathkopf, D., Blackford, A. L., Danila, D. C., et al. (2013). Repurposing itraconazole as a treatment for advanced prostate cancer: a noncomparative randomized phase II trial in men with metastatic castration-resistant prostate cancer. Oncologist 18, 163–173. doi: 10.1634/theoncologist.2012-314
Assayag, J., Pollak, M. N., and Azoulay, L. (2015). The use of aspirin and the risk of mortality in patients with prostate cancer. J. Urol. 193, 1220–1225. doi: 10.1016/j.juro.2014.11.018
Ataie-Kachoie, P., Morris, D. L., and Pourgholami, M. H. (2013). Minocycline suppresses interleukine-6, its receptor system and signaling pathways and impairs migration, invasion and adhesion capacity of ovarian cancer cells: in vitro and in vivo studies. PLoS One 8:e60817. doi: 10.1371/journal.pone.0060817
Ayan, D., Maltais, R., and Poirier, D. (2012). Identification of a 17β-hydroxysteroid dehydrogenase type10 steroidal inhibitor: a tool to investigate the role of type10 in Alzheimer’s disease and prostate cancer. ChemMedChem 7, 1181–1184. doi: 10.1002/cmdc.201200129
Benfeitas, R., Uhlen, M., Nielsen, J., and Mardinoglu, A. (2017). New challenges to study heterogeneity in cancer redox metabolism. Front. Cell Dev. Biol. 5:65. doi: 10.3389/fcell.2017.00065
Bhattarai, D., Singh, S., Jang, Y., Han, S. H., Lee, K., and Choi, Y. (2016). An insight into drug repositioning for the development of novel anti-cancer drugs. Curr. Top. Med. Chem. 16, 2156–2168. doi: 10.2174/1568026616666160216153618
Bilani, N., Bahmad, H., and Abou-Kheir, W. (2017). Prostate cancer and aspirin use: Synopsis of the proposed molecular mechanisms. Front. Pharmacol. 8:145. doi: 10.3389/fphar.2017.00145
Breedveld, F. C., and Dayer, J.-M. (2000). Leflunomide: mode of action in the treatment of rheumatoid arthritis. Ann. Rheum. Dis. 59, 841–849. doi: 10.1136/ard.59.11.841
Brodie, S. A., and Brandes, J. C. (2014). Could valproic acid be an effective anticancer agent? The evidence so far. Expert Rev. Anticancer Ther. 14, 1097–1100. doi: 10.1586/14737140.2014.940329
Buttgereit, F., Burmester, G. R., Straub, R. H., Seibel, M. J., and Zhou, H. (2011). Exogenous and endogenous glucocorticoids in rheumatic diseases. Arthritis Rheum. 63, 1–9. doi: 10.1002/art.30070
Calderón-Montaño, J., Estefanía, B.-M., Manuel Luis, O., and Maldonado-Navas, D. (2014). Evaluating the cancer therapeutic potential of cardiac glycosides. Biomed Res. Int. 2014:794930. doi: 10.1155/2014/794930
Caon, J., Paquette, M., Hamm, J., and Pickles, T. (2014). Does statin or ASA affect survival when prostate cancer is treated with external beam radiation therapy? Prostate Cancer 2014, 184297. doi: 10.1155/2014/184297
Chan, K. K. W., Oza, A. M., and Siu, L. L. (2003). The statins as anticancer agents. Clin. Cancer Res. 9, 10–19. doi: 10.1158/1078-0432.ccr-13-1524
Chang, W.-L., Hsu, L.-C., Leu, W.-J., Chen, C.-S., and Guh, J.-H. (2015). Repurposing of nitroxoline as a potential anticancer agent against human prostate cancer - a crucial role on AMPK/mTOR signaling pathway and the interplay with Chk2 activation. Oncotarget 6, 39806–39820. doi: 10.18632/oncotarget.5655
Chong, C., and Sullivan, D. J. (2007). New uses for old drugs. Nature 448, 645–646. doi: 10.1038/448645a
Chong, C. R., Chen, X., Shi, L., Liu, J. O., and Sullivan, D. J. (2006a). A clinical drug library screen identifies astemizole as an antimalarial agent. Nat. Chem. Biol. 2, 415–416. doi: 10.1038/nchembio806
Chong, C. R., Qian, D. Z., Pan, F., Wei, Y., Pili, R., Sullivan, D. J., et al. (2006b). Identification of type 1 inosine monophosphate dehydrogenase as an antiangiogenic drug target. J. Med. Chem. 49, 2677–2680. doi: 10.1021/jm051225t
Chong, C. R., Xu, J., Lu, J., Bhat, S., Sullivan, D. J., and Liu, J. O. (2007). Inhibition of angiogenesis by the antifungal drug itraconazole. ACS Chem. Biol. 2, 263–270. doi: 10.1021/cb600362d
Chu, C. C., Hsing, C. H., Shieh, J. P., Chien, C. C., Ho, C. M., and Wang, J. J. (2014). The cellular mechanisms of the antiemetic action of dexamethasone and related glucocorticoids against vomiting. Eur. J. Pharmacol. 722, 48–54. doi: 10.1016/j.ejphar.2013.10.008
Corey, E., Brown, L. G., Quinn, J. E., Poot, M., Roudier, M. P., Higano, C. S., et al. (2003). Zoledronic acid exhibits inhibitory effects on osteoblastic and osteolytic metastases of prostate cancer. Clin. Cancer Res. 9, 295–306.
Davies, N. M., McLachlan, A. J., Day, R. O., and Williams, K. M. (2000). Clinical pharmacokinetics and pharmacodynamics of celecoxib: a selective cyclo-oxygenase-2 inhibitor. Clin. Pharmacokinet. 38, 225–242. doi: 10.2165/00003088-200038030-00003
Deotarse, P. P., Jain, A. S., Baile, M. B., Kolhe, N. S., and Kulkarni, A. A. (2015). Drug repositioning: a review. Int. J. Pharma Res. Rev. 4, 51–58.
Dilly, S. J., Clark, A. J., Marsh, A., Mitchell, D. A., Cain, R., Fishwick, C. W. G., et al. (2017). A chemical genomics approach to drug reprofiling in oncology: antipsychotic drug risperidone as a potential adenocarcinoma treatment. Cancer Lett. 393, 16–21. doi: 10.1016/j.canlet.2017.01.042
Downer, M. K., Allard, C. B., Preston, M. A., Gaziano, J. M., Stampfer, M. J., Mucci, L. A., et al. (2017). Regular aspirin use and the risk of lethal prostate cancer in the physicians’ Health Study. Eur. Urol. 72, 821–827. doi: 10.1016/j.eururo.2017.01.044
Eidelman, E., Twum-Ampofo, J., Ansari, J., and Siddiqui, M. M. (2017). The metabolic phenotype of prostate cancer. Front. Oncol. 7:131. doi: 10.3389/fonc.2017.00131
El Etreby, M. F., Liang, Y., and Lewis, R. W. (2000). Induction of apoptosis by mifepristone and tamoxifen in human LNCaP prostate cancer cells in culture. Prostate 43, 31–42. doi: 10.1002/(SICI)1097-0045(20000401)43:1<31::AID-PROS5>3.0.CO;2-
Elder, D. J. E., Halton, D. E., Crew, T. E., and Paraskeva, C. (2000). Apoptosis induction and cyclooxygenase-2 regulation in human colorectal adenoma and carcinoma cell lines by the cyclooxygenase-2-selective non-steroidal anti-inflammatory drug Ns-398. Int. J. Cancer 86, 553–560. doi: 10.1002/(SICI)1097-0215(20000515)86:4<553::AID-IJC18>3.0.CO;2-9
Ergün, A., Lawrence, C. A., Kohanski, M. A., Brennan, T. A., and Collins, J. J. (2007). A network biology approach to prostate cancer. Mol. Syst. Biol. 3:82. doi: 10.1038/msb4100125
Evans, J. M. M., Donnelly, L. A., Emslie-Smith, A. M., Alessi, D. R., and Morris, A. D. (2005). Metformin and reduced risk of cancer in diabetic patients. Br. Med. J. 330, 1304–1305. doi: 10.1136/bmj.38415.708634.F7
Ferlay, J., Soerjomataram, I., Dikshit, R., Eser, S., Mathers, C., Rebelo, M., et al. (2015). Cancer incidence and mortality worldwide: Sources, methods and major patterns in GLOBOCAN 2012. Int. J. Cancer 136, E359–E386. doi: 10.1002/ijc.29210
Fowke, J. H., Motley, S. S., Smith, J. A., Cookson, M. S., Concepcion, R., Chang, S. S., et al. (2009). Association of nonsteroidal anti-inflammatory drugs, prostate specific antigen and prostate volume. J. Urol. 181, 2064–2070. doi: 10.1016/j.juro.2009.01.031
Fu, W., Madan, E., Yee, M., and Zhang, H. (2012). Progress of molecular targeted therapies for prostate cancers. Biochim. Biophys. Acta 1825, 140–152. doi: 10.1016/j.bbcan.2011.11.003
Gallaher, J., Cook, L. M., Gupta, S., Araujo, A., Dhillon, J., Park, J. Y., et al. (2014). Improving treatment strategies for patients with metastatic castrate resistant prostate cancer through personalized computational modeling. Clin. Exp. Metastasis 31, 991–999. doi: 10.1007/s10585-014-9674-1
Gantt, S., Casper, C., and Ambinder, R. F. (2013). Insights into the broad cellular effects of nelfinavir and the HIV protease inhibitors supporting their role in cancer treatment and prevention. Curr. Opin. Oncol. 25, 495–502. doi: 10.1097/CCO.0b013e328363dfee
Garrido-Mesa, N., Zarzuelo, A., and Gálvez, J. (2013). Minocycline: Far beyond an antibiotic. Br. J. Pharmacol. 169, 337–352. doi: 10.1111/bph.12139
Gayvert, K. M., Dardenne, E., Cheung, C., Boland, M. R., Lorberbaum, T., Wanjala, J., et al. (2016). A computational drug repositioning approach for targeting oncogenic transcription factors. Cell Rep. 15, 2348–2356. doi: 10.1016/j.celrep.2016.05.037
Ghaffari, P., Mardinoglu, A., Asplund, A., Shoaie, S., Kampf, C., Uhlen, M., et al. (2015). Identifying anti-growth factors for human cancer cell lines through genome-scale metabolic modeling. Sci. Rep. 5:8183. doi: 10.1038/srep08183
Gonnissen, A., Isebaert, S., McKee, C. M., Dok, R., Haustermans, K., and Muschel, R. J. (2016). The hedgehog inhibitor GANT61 sensitizes prostate cancer cells to ionizing radiation both in vitro and in vivo. Oncotarget 7, 84286–84298. doi: 10.18632/oncotarget.12483
Gottfried, E., Lang, S. A., Renner, K., Bosserhoff, A., Gronwald, W., Rehli, M., et al. (2013). New aspects of an old drug - diclofenac targets MYC and glucose metabolism in tumor cells. PLoS One 8:e66987. doi: 10.1371/journal.pone.0066987
Grösch, S., Maier, T. J., Schiffmann, S., and Geisslinger, G. (2006). Cyclooxygenase-2 (COX-2)-independent anticarcinogenic effects of selective COX-2 inhibitors. J. Natl. Cancer Inst. 98, 736–747. doi: 10.1093/jnci/djj206
Guan, M., Su, L., Yuan, Y. C., Li, H., and Chow, W. A. (2015). Nelfinavir and nelfinavir analogs block site-2 protease cleavage to inhibit castration-resistant prostate cancer. Sci. Rep. 5:9698. doi: 10.1038/srep09698
Gupta, S. C., Sung, B., Prasad, S., Webb, L. J., and Aggarwal, B. B. (2013). Cancer drug discovery by repurposing: Teaching new tricks to old dogs. Trends Pharmacol. Sci. 34, 508–517. doi: 10.1016/j.tips.2013.06.005
Hafeez, B. B., Ganju, A., Sikander, M., Kashyap, V. K., Hafeez, Z., Bin, et al. (2017). Ormeloxifene suppresses prostate tumor growth and metastatic phenotypes via inhibition of oncogenic β-catenin signaling and EMT progression. Mol. Cancer Ther. 16, 2267–2280. doi: 10.1158/1535-7163.MCT-17-0157
Hail, N., Chen, P., and Bushman, L. R. (2010). Teriflunomide (Leflunomide) promotes cytostatic, antioxidant, and apoptotic effects in transformed prostate epithelial cells: evidence supporting a role for teriflunomide in prostate cancer chemoprevention. Neoplasia 12, 464–475. doi: 10.1593/neo.10168
Harris, R. E., Alshafie, G. A., Abou-Issa, H., and Seibert, K. (2000). Chemoprevention of breast cancer in rats by celecoxib, a cyclooxygenase 2 inhibitor. Cancer Res. 60, 2101–2103.
Hazra, B. G., and Pore, V. S. (2001). Mifepristone (RU-486), the recently developed antiprogesterone drug and its analogues. J. Indian Inst. Sci. 81, 287–298.
Hindler, K. (2006). The role of statins in cancer therapy. Oncologist 11, 306–315. doi: 10.1634/theoncologist.11-3-306
Hirsch, H. A., Iliopoulos, D., and Struhl, K. (2013). Metformin inhibits the inflammatory response associated with cellular transformation and cancer stem cell growth. Proc. Natl. Acad. Sci. U.S.A. 110, 972–977. doi: 10.1073/pnas.1221055110
Hodos, R. A., Kidd, B. A., Khader, S., Readhead, B. P., and Dudley, J. T. (2016). In silico methods for drug repurposing and pharmacology. WIREs Syst. Biol. Med. 8, 186–210. doi: 10.1002/wsbm.1337
Hsu, A. L., Ching, T. T., Wang, D. S., Song, X., Rangnekar, V. M., and Chen, C. S. (2000). The cyclooxygenase-2 inhibitor celecoxib induces apoptosis by blocking Akt activation in human prostate cancer cells independently of Bcl-2. J. Biol. Chem. 275, 11397–11403. doi: 10.1074/jbc.275.15.11397
Huang, R., Southall, N., Wang, Y., Yasgar, A., Shinn, P., Jadhav, A., et al. (2011). The NCGC pharmaceutical collection: a comprehensive resource of clinically approved drugs enabling repurposing and chemical genomics. Sci. Transl. Med. 3:80ps16. doi: 10.1126/scitranslmed.3001862
Hutchinson, J., and Marignol, L. (2017). Clinical potential of statins in prostate cancer radiation therapy. Anticancer Res. 37, 5363–5372. doi: 10.21873/anticanres.11962
Iljin, K., Ketola, K., Vainio, P., Halonen, P., Kohonen, P., Fey, V., et al. (2009). High-throughput cell-based screening of 4910 known drugs and drug-like small molecules identifies disulfiram as an inhibitor of prostate cancer cell growth. Clin. Cancer Res. 15, 6070–6078. doi: 10.1158/1078-0432.CCR-09-1035
Inoue, T., Anai, S., Onishi, S., Miyake, M., Tanaka, N., Hirayama, A., et al. (2013). Inhibition of COX-2 expression by topical diclofenac enhanced radiation sensitivity via enhancement of TRAIL in human prostate adenocarcinoma xenograft model. BMC Urol. 13:1. doi: 10.1186/1471-2490-13-1
Iwamoto, Y., Ishii, K., Kanda, H., Kato, M., Miki, M., Kajiwara, S., et al. (2017). Combination treatment with naftopidil increases the efficacy of radiotherapy in PC-3 human prostate cancer cells. J. Cancer Res. Clin. Oncol. 143, 933–939. doi: 10.1007/s00432-017-2367-9
Jaggi, M., Johansson, S. L., Baker, J. J., Smith, L. M., Galich, A., and Balaji, K. C. (2005). Aberrant expression of E-cadherin and beta-catenin in human prostate cancer. Urol. Oncol. Semin. Orig. Investig. 23, 402–406. doi: 10.1016/j.urolonc.2005.03.024
Jaggi, M., Nazemi, T., Abrahams, N. A., Baker, J. J., Galich, A., Smith, L. M., et al. (2006). N-cadherin switching occurs in high Gleason grade prostate cancer. Prostate 66, 193–199. doi: 10.1002/pros.20334
Jalving, M., Gietema, J. A., Lefrandt, J. D., de Jong, S., Reyners, A. K. L., Gans, R. O. B., et al. (2010). Metformin: Taking away the candy for cancer? Eur. J. Cancer 46, 2369–2380. doi: 10.1016/j.ejca.2010.06.012
James, N. D., Sydes, M. R., Clarke, N. W., Mason, M. D., Dearnaley, D. P., Spears, M. R., et al. (2016). Addition of docetaxel, zoledronic acid, or both to first-line long-term hormone therapy in prostate cancer (STAMPEDE): survival results from an adaptive, multiarm, multistage, platform randomised controlled trial. Lancet 387, 1163–1177. doi: 10.1016/S0140-6736(15)01037-5
Jiang, H., Taggart, J. E., Zhang, X., Benbrook, D. M., Lind, S. E., and Ding, W.-Q. (2012). Nitroxoline (5-amino-8-hydroxyquinoline) is more a potent anticancer agent than clioquinol (5-chloro-7-iodo-8-quinoline). Cancer Lett. 312, 11–17. doi: 10.1016/j.canlet.2011.06.032.Nitroxoline
Jiao, M., Liu, G., Xue, Y., and Ding, C. (2015). Computational drug repositioning for cancer therapeutics. Curr. Top. Med. Chem 15, 767–775. doi: 10.2174/1568026615666150302105831
Jin, G., Fu, C., Zhao, H., Cui, K., Chang, J., and Wong, S. T. C. (2012). A novel method of transcriptional response analysis to facilitate drug repositioning for cancer therapy. Cancer Res. 72, 33–44. doi: 10.1158/0008-5472.CAN-11-2333
Kalra, G., de Sousa, A., and Shrivastava, A. (2014). Disulfiram in the management of alcohol dependence: a comprehensive clinical review. Open J. Psychiatry 4, 43–52. doi: 10.1111/nyas.12538
Katz, M. S., Carroll, P. R., Cowan, J. E., Chan, J. M., and D’Amico, A. V. (2010). Association of statin and nonsteroidal anti-inflammatory drug use with prostate cancer outcomes: results from CaPSURE. BJU Int. 106, 627–632. doi: 10.1111/j.1464-410X.2010.09232.x
Kim, J., Tang, J. Y., Gong, R., Kim, J., Lee, J. J., Clemons, K. V., et al. (2010). Itraconazole, a commonly used antifungal that inhibits hedgehog pathway activity and cancer growth. Cancer Cell 17, 388–399. doi: 10.1016/j.ccr.2010.02.027
Kirschenbaum, A., Liu, X. H., Yao, S., and Levine, A. C. (2001). The role of cyclooxygenase-2 in prostate cancer. Urology 58(2 Suppl. 1), 127–131. doi: 10.1016/S0090-4295(01)01255-9
Ko, Y. J., Small, E. J., Kabbinavar, F., Chachoua, A., Taneja, S., Reese, D., et al. (2001). A multi-institutional phase ii study of SU101, a platelet-derived growth factor receptor inhibitor, for patients with hormone-refractory prostate cancer. Clin. Cancer Res. 7, 800–805.
Koltai, T. (2015). Fenofibrate in cancer: mechanisms involved in anticancer activity. F1000Research 4:55. doi: 10.12688/f1000research.6153.2
Kondratskyi, A., Kondratska, K., Vanden Abeele, F., Gordienko, D., Dubois, C., Toillon, R. A., et al. (2017). Ferroquine, the next generation antimalarial drug, has antitumor activity. Sci. Rep. 7:15896. doi: 10.1038/s41598-017-16154-2
Kulp, S. K., Yang, Y., Hung, C., Chen, K., Lai, J., Tseng, P., et al. (2004). 3-Phosphoinositide-dependent protein kinase-1 / Akt signaling represents a major cyclooxygenase-2-independent target for celecoxib in prostate cancer cells 3-phosphoinositide-dependent protein kinase-1 / Akt signaling represents a major cyclooxygenase-2-I. Cancer Res. 64, 1444–1451. doi: 10.1158/0008-5472.CAN-03-2396
Leahy, K. M., Ornberg, R. L., Wang, Y., Zweifel, B. S., Koki, A. T., and Masferrer, J. L. (2002). Cyclooxygenase-2 inhibition by celecoxib reduces proliferation and induces apoptosis in angiogenic endothelial cells in vivo. Cancer Res. 62, 625–631.
Lee, J. E., and Kim, J. H. (2015). Valproic acid inhibits the invasion of PC3 prostate cancer cells by upregulating the metastasis suppressor protein NDRG1. Genet. Mol. Biol. 38, 527–533. doi: 10.1590/S1415-475738420150028
Lee, S., Zhang, C., Arif, M., Liu, Z., Benfeitas, R., Bidkhori, G., et al. (2018). TCSBN: A database of tissue and cancer specific biological networks. Nucleic Acids Res. 46, D595–D600. doi: 10.1093/nar/gkx994
Lee, S., Zhang, C., Kilicarslan, M., Piening, B. D., Bjornson, E., Hallström, B. M., et al. (2016). Integrated network analysis reveals an association between plasma mannose levels and insulin resistance. Cell Metab. 24, 172–184. doi: 10.1016/j.cmet.2016.05.026
Lee, S., Zhang, C., Liu, Z., Klevstig, M., Mukhopadhyay, B., Bergentall, M., et al. (2017). Network analyses identify liver-specific targets for treating liver diseases. Mol. Syst. Biol. 13:938. doi: 10.15252/msb.20177703
Levy, C. W., Roujeinikova, A., Sedelnikova, S., Baker, P. J., Stuitje, A. R., Slabas, A. R., et al. (1999). Molecular basis of triclosan activity. Nature 398, 383–384. doi: 10.1038/18803
Lewiecki, E. M. (2009). Intravenous zoledronic acid for the treatment of osteoporosis: the evidence of its therapeutic effect. Core Evid. 4, 13–23. doi: 10.2147/CE.S6011
Li, Y. Y., and Jones, S. J. (2012). Drug repositioning for personalized medicine. Genome Med. 4:27. doi: 10.1186/gm326
Lin, J., Haffner, M. C., Zhang, Y., Lee, B. H., Brennen, W. N., Britton, J., et al. (2011). Disulfiram is a DNA demethylating agent and inhibits prostate cancer cell growth. Prostate 71, 333–343. doi: 10.1002/pros.21247
Lin, J., Zhan, T., Duffy, D., Hoffman-Censits, J., Kilpatrick, D., Trabulsi, E. J., et al. (2014). A pilot phase II study of digoxin in patients with recurrent prostate cancer as evident by a rising PSA. Am. J. cancer Ther. Pharmacol. 2, 21–32.
Liu, Y., Chen, J. Q., Xie, L., Wang, J., Li, T., He, Y., et al. (2014). Effect of aspirin and other non-steroidal anti-inflammatory drugs on prostate cancer incidence and mortality: a systematic review and meta-analysis. BMC Med. 12:55. doi: 10.1186/1741-7015-12-55
Lokeshwar, B. L. (2011). Chemically modified non-antimicrobial tetracyclines are multifunctional drugs against advanced cancers. Pharmacol. Res. 63, 146–150. doi: 10.1016/j.phrs.2010.11.003
Lopez-Lazaro, M. (2009). Digoxin, HIF-1, and cancer. Proc. Natl. Acad. Sci. U.S.A. 106:E26. doi: 10.1073/pnas.0813047106
Lu, W., Lin, C., Roberts, M. J., Waud, W. R., Piazza, G. A., and Li, Y. (2011). Niclosamide suppresses cancer cell growth by inducing Wnt co-receptor LRP6 degradation and inhibiting the Wnt/β-catenin pathway. PLoS One 6:e29290. doi: 10.1371/journal.pone.0029290
Mahmud, S. M., Franco, E. L., and Aprikian, A. G. (2010). Use of nonsteroidal anti-inflammatory drugs and prostate cancer risk: a meta-analysis. Int. J. Cancer 127, 1680–1691. doi: 10.1002/ijc.25186
Marcella, S. W., David, A., Ohman-Strickland, P. A., Carson, J., and Rhoads, G. G. (2012). Statin use and fatal prostate cancer: a matched case-control study. Cancer 118, 4046–4052. doi: 10.1002/cncr.26720
March-Vila, E., Pinzi, L., Sturm, N., Tinivella, A., Engkvist, O., Chen, H., et al. (2017). On the integration of in silico drug design methods for drug repurposing. Front. Pharmacol. 8:298. doi: 10.3389/fphar.2017.00298
Mardinoglu, A., Agren, R., Kampf, C., Asplund, A., Uhlen, M., and Nielsen, J. (2014). Genome-scale metabolic modelling of hepatocytes reveals serine deficiency in patients with non-alcoholic fatty liver disease. Nat. Commun. 5:3083. doi: 10.1038/ncomms4083
Mardinoglu, A., Bjornson, E., Zhang, C., Klevstig, M., Söderlund, S., Ståhlman, M., et al. (2017). Personal model-assisted identification of NAD + and glutathione metabolism as intervention target in NAFLD. Mol. Syst. Biol. 13:916. doi: 10.15252/msb.20167422
Mardinoglu, A., Boren, J., Smith, U., Uhlen, M., and Nielsen, J. (2018a). Systems biology in hepatology: approaches and applications. Nat. Rev. Gastroenterol. Hepatol. (in press). doi: 10.1038/s41575-018-0007-8
Mardinoglu, A., and Nielsen, J. (2012). Systems medicine and metabolic modelling. J. Int. Med. 271, 142–154. doi: 10.1111/j.1365-2796.2011.02493.x
Mardinoglu, A., and Nielsen, J. (2015). New paradigms for metabolic modeling of human cells. Curr. Opin. Biotechnol. 34, 91–97. doi: 10.1016/j.copbio.2014.12.013
Mardinoglu, A., Wu, H., Bjornson, E., Zhang, C., Hakkarainen, A., Räsänen, S. M., et al. (2018b). An integrated understanding of the rapid metabolic benefits of a carbohydrate-restricted diet on hepatic steatosis in humans. Cell Metab. 27, 559.e5–571.e5. doi: 10.1016/j.cmet.2018.01.005
Margel, D., Urbach, D. R., Lipscombe, L. L., Bell, C. M., Kulkarni, G., Austin, P. C., et al. (2013). Metformin use and all-cause and prostate cancer-specific mortality among men with diabetes. J. Clin. Oncol. 31, 3069–3075. doi: 10.1200/JCO.2012.46.7043
Marin de Mas, I., Aguilar, E., Zodda, E., Balcells, C., Marin, S., Dallmann, G., et al. (2018). Model-driven discovery of long-chain fatty acid metabolic reprogramming in heterogeneous prostate cancer cells. PLoS Comput. Biol. 14:e1005914. doi: 10.1371/journal.pcbi.1005914
Mason, M. D., Clarke, N. W., James, N. D., Dearnaley, D. P., Spears, M. R., Ritchie, A. W. S., et al. (2017). Adding celecoxib with or without zoledronic acid for hormone-naïve prostate cancer: long-term survival results from an adaptive, multiarm, multistage, platform, randomized controlled trial. J. Clin. Oncol. 35, 1530–1541. doi: 10.1200/JCO.2016.69.0677
Masumori, N. (2011). Naftopidil for the treatment of urinary symptoms in patients with benign prostatic hyperplasia. Ther. Clin. Risk Manage. 7, 227–238. doi: 10.2147/TCRM.S13883
McMahon, M. A., Jilek, B. L., Brennan, T. P., Shen, L., Zhou, Y., Wind-Rotolo, M., et al. (2007). The HBV drug entecavir — Effects on HIV-1 replication and resistance. N. Engl. J. Med. 356, 2614–2621. doi: 10.1056/NEJMoa067710
McMurry, L. M., Oethinger, M., and Levy, S. B. (1998). Triclosan targets lipid synthesis. Nature 394, 531–532. doi: 10.1038/28970
Michaelis, M., Doerr, H. W., and Cinatl, J. (2007). Valproic acid as anti-cancer drug. Curr. Pharm. Des. 13, 3378–3393. doi: 10.2174/138161207782360519
Mobbs, B. G., and Johnson, I. E. (1991). Suppression of the growth of the androgen-insensitive R3327 hi rat prostatic carcinoma by combined estrogen and antiprogestin treatment. J. Steroid Biochem. Mol. Biol. 39, 713–722. doi: 10.1016/0960-0760(91)90371-B
Niraula, S., Templeton, A. J., Vera-Badillo, F., Dodd, A., Nugent, Z., Joshua, A. M., et al. (2018). Duration of suppression of bone turnover following treatment with zoledronic acid in men with metastatic castration-resistant prostate cancer. Futur. Sci. OA 4:FSO253. doi: 10.4155/fsoa-2017
Noto, H., Goto, A., Tsujimoto, T., and Noda, M. (2012). Cancer risk in diabetic patients treated with metformin: a systematic review and meta-analysis. PLoS One 7:e33411. doi: 10.1371/journal.pone.0033411
Padhy, B. M., and Gupta, Y. K. (2011). Drug repositioning: re-investigating existing drugs for new therapeutic indications. J. Postgrad. Med. 57, 153–160. doi: 10.4103/0022-3859.81870
Pan, J. X., Ding, K., and Wang, C. Y. (2012). Niclosamide, an old antihelminthic agent, demonstrates antitumor activity by blocking multiple signaling pathways of cancer stem cells. Chin. J. Cancer 31, 178–184. doi: 10.5732/cjc.011.10290
Pantziarka, P., Bouche, G., Meheus, L., Sukhatme, V., and Sukhatme, V. P. (2014). The repurposing drugs in oncology (ReDO) project. ecancer 8:442. doi: 10.3332/ecancer.2014.442
Pantziarka, P., Sukhatme, V., Bouche, G., Meheus, L., and Sukhatme, V. P. (2015). Repurposing drugs in oncology (ReDO)—itraconazole as an anti-cancer agent. Ecancermedicalscience 9:521. doi: 10.3332/ecancer.2015.521
Pantziarka, P., Sukhatme, V. V. P., Bouche, G., Melhuis, L., and Sukhatme, V. V. P. (2016). Repurposing drugs in oncology (ReDO)—diclofenac as an anti-cancer agent. Ecancermedicalscience 10:610. doi: 10.3332/ecancer.2016.610
Patel, M. I., Subbaramaiah, K., Du, B., Patel, M. I., Subbaramaiah, K., Du, B., et al. (2005). Celecoxib inhibits prostate cancer growth: evidence of a cyclooxygenase-2-independent mechanism. Clin. Cancer. Res. 11, 1999–2007. doi: 10.1158/1078-0432.CCR-04-1877
Pelletier, J., Bordeleau, M., Lindqvist, L., Francis, R., Sukarieh, R., and Tanaka, J. (2007). Chemotherapeutic agents for inhibition of protein translation Vol. WO2007/009264 A1, A61K 31/58 Edn.
Perry, C. M., and Figgitt, D. P. (2004). Zoledronic acid: a review of its use in patients with advanced cancer. Drugs 64, 1197–1211. doi: 10.2165/00003495-200464110-00004
Piao, S., and Amaravadi, R. K. (2016). Targeting the lysosome in cancer. Ann. N. Y. Acad. Sci. 1371, 45–54. doi: 10.1111/nyas.12953
Platz, E. A., Yegnasubramanian, S., Liu, J. O., Chong, C. R., Shim, J. S., Kenfield, S. A., et al. (2011). A novel two-stage, transdisciplinary study identifies digoxin as a possible drug for prostate cancer treatment. Cancer Discov. 1, 68–77. doi: 10.1158/2159-8274.CD-100020
Polascik, T. J., and Mouraviev, V. (2008). Zoledronic acid in the management of metastatic bone disease. Ther. Clin. Risk Manage. 4, 261–268. doi: 10.2147/TCRM.S2707
Ponder, B. (1997). Genetic testing for cancer risk. Science 278, 1050–1054. doi: 10.1126/science.278.5340.1050
Preston, M. A., Riis, A. H., Ehrenstein, V., Breau, R. H., Batista, J. L., Olumi, A. F., et al. (2014). Metformin use and prostate cancer risk. Eur. Urol. 66, 1012–1020. doi: 10.1016/j.eururo.2014.04.027
Qi, C., Li, B., Yang, Y. Y., Yang, Y. Y., Li, J. J., Zhou, Q., et al. (2016). Glipizide suppresses prostate cancer progression in the TRAMP model by inhibiting angiogenesis. Sci. Rep. 6:27819. doi: 10.1038/srep27819
Rebecca, V. W., and Amaravadi, R. K. (2016). Emerging strategies to effectively target autophagy in cancer. Oncogene 35, 1–11. doi: 10.1038/onc.2015.99
Regen, F., Heuser, I., Herzog, I., and Hellmann-Regen, J. (2014). Striking growth-inhibitory effects of minocycline on human prostate cancer cell lines. Urology 83, 509.e1–506.e1. doi: 10.1016/j.urology.2013.10.029
Roth, B. L., Sheffler, D. J., and Kroeze, W. K. (2004). Magic shotguns versus magic bullets: selectively non-selective drugs for mood disorders and schizophrenia. Nat. Rev. Drug Discov. 3, 353–359. doi: 10.1038/nrd1346
Rudin, C. M., Brahmer, J. R., Juergens, R. A., Hann, C. L., Ettinger, D. S., Sebree, R., et al. (2013). Phase 2 study of pemetrexed and itraconazole as second-line therapy for metastatic nonsquamous non-small-cell lung cancer. J. Thorac. Oncol. 8, 619–623. doi: 10.1097/JTO.0b013e31828c3950
Saad, F., Gleason, D. M., Murray, R., Tchekmedyian, S., Venner, P., Lacombe, L., et al. (2002). A randomized, placebo-controlled trial of zoledronic acid in patients with hormone-refractory metastatic prostate carcinoma. J. Natl. Cancer Inst. 94, 1458–1468. doi: 10.1093/jnci/94.19.1458
Saad, F., Gleason, D. M., Murray, R., Tchekmedyian, S., Venner, P., Lacombe, L., et al. (2004). Long-term efficacy of zoledronic acid for the prevention of skeletal complications in patients with metastatic hormone-refractory prostate cancer. J. Natl. Cancer Inst. 96, 879–882. doi: 10.1093/jnci/djh141
Sack, U., Walther, W., Scudiero, D., Selby, M., Kobelt, D., Lemm, M., et al. (2011). Novel effect of antihelminthic niclosamide on s100a4-mediated metastatic progression in colon cancer. J. Natl. Cancer Inst. 103, 1018–1036. doi: 10.1093/jnci/djr190
Sadowski, M. C., Pouwer, R. H., Gunter, J. H., Lubik, A. A., Quinn, R. J., and Nelson, C. C. (2014). The fatty acid synthase inhibitor triclosan: repurposing an anti- microbial agent for targeting prostate cancer. Oncotarget 5, 9362–9381. doi: 10.18632/oncotarget.2433
Safi, R., Nelson, E. R., Chitneni, S. K., Franz, K. J., George, D. J., Zalutsky, M. R., et al. (2014). Copper signaling axis as a target for prostate cancer therapeutics. Cancer Res. 74, 5819–5831. doi: 10.1158/0008-5472.CAN-13-3527
Schemmel, K. E., Padiyara, R. S., and D’Souza, J. J. (2010). Aldose reductase inhibitors in the treatment of diabetic peripheral neuropathy: a review. J. Diabetes Complications 24, 354–360. doi: 10.1016/j.jdiacomp.2009.07.005
Schneider, H. C., and Klabunde, T. (2013). Understanding drugs and diseases by systems biology? Bioorganic Med. Chem. Lett. 23, 1168–1176. doi: 10.1016/j.bmcl.2012.12.031
Schweizer, M. T., Lin, J., Blackford, A., Bardia, A., King, S., Armstrong, A. J., et al. (2013). Pharmacodynamic study of disulfiram in men with non-metastatic recurrent prostate cancer. Prostate Cancer Prostatic Dis. 16, 357–361. doi: 10.1038/pcan.2013.28
Shaked, I., Oberhardt, M. A., Atias, N., Sharan, R., and Ruppin, E. (2016). Metabolic network prediction of drug side effects. Cell Syst. 2, 209–213. doi: 10.1016/j.cels.2016.03.001
Shamash, J., Powles, T., Sarker, S. J., Protheroe, A., Mithal, N., Mills, R., et al. (2011). A multi-centre randomised phase III trial of Dexamethasone vs Dexamethasone and diethylstilbestrol in castration-resistant prostate cancer: immediate vs deferred Diethylstilbestrol. Br. J. Cancer 104, 620–628. doi: 10.1038/bjc.2011.7
Sharma, S., Symanowski, J., Wong, B., Dino, P., Manno, P., and Vogelzang, N. (2008). A phase II clinical trial of oral valproic acid in patients with castration-resistant prostate cancers using an intensive biomarker sampling strategy. Transl. Oncol. 1, 141–147. doi: 10.1593/tlo.08136
Shawver, K., Schwartz, P., Mann, E., Chen, H., Tsai, J., Chu, L., et al. (1997). Inhibition of platelet-derived growth factor-mediated signal transduction and tumor growth by N-[4-(trifluoromethyl)-phenyl]5-methylisoxazole-4-carboxamide. Clin. Cancer Res. 3, 1167–1177
Shim, J. S., and Liu, J. O. (2014). Recent advances in drug repositioning for the discovery of new anticancer drugs. Int. J. Biol. Sci. 10, 654–663. doi: 10.7150/ijbs.9224
Shim, J. S., Matsui, Y., Bhat, S., Nacev, B. A., Xu, J., Bhang, H. E. C., et al. (2010). Effect of nitroxoline on angiogenesis and growth of human bladder cancer. J. Natl. Cancer Inst. 102, 1855–1873. doi: 10.1093/jnci/djq457
Shoaie, S., Ghaffari, P., Kovatcheva-Datchary, P., Mardinoglu, A., Sen, P., Pujos-Guillot, E., et al. (2015). Quantifying diet-induced metabolic changes of the human gut microbiome. Cell Metab. 22, 320–331. doi: 10.1016/j.cmet.2015.07.001
Siegel, R. L., Miller, K. D., and Jemal, A. (2018). Cancer statistics, 2018. CA Cancer J. Clin. 68, 7–30. doi: 10.3322/caac.21442
Sirota, M., Dudley, J. T., Kim, J., Chiang, A. P., Morgan, A. A., Sweet-Cordero, A., et al. (2011). Discovery and preclinical validation of drug indications using compendia of public gene expression data. Sci. Transl. Med. 77:96ra77. doi: 10.1126/scitranslmed.3001318
Skrott, Z., Mistrik, M., Andersen, K. K., Friis, S., Majera, D., Gursky, J., et al. (2017). Alcohol-abuse drug disulfiram targets cancer via p97 segregase adaptor NPL4. Nature 552, 194–199. doi: 10.1038/nature25016
Slingerland, M., Cerella, C., Guchelaar, H. J., Diederich, M., and Gelderblom, H. (2013). Cardiac glycosides in cancer therapy: From preclinical investigations towards clinical trials. Invest. New Drugs 31, 1087–1094. doi: 10.1007/s10637-013-9984-1
Sosiè, I., Mirkoviæ, B., Arenz, K., Štefane, B., Kos, J., and Gobec, S. (2013). Development of new cathepsin b inhibitors: combining bioisosteric replacements and structure-based design to explore the structure-activity relationships of nitroxoline derivatives. J. Med. Chem. 56, 521–533. doi: 10.1021/jm301544x
Sun, P., Guo, J., Winnenburg, R., and Baumbach, J. (2017). Drug repurposing by integrated literature mining and drug–gene–disease triangulation. Drug Discov. Today 22, 615–619. doi: 10.1016/j.drudis.2016.10.008
Sussman, G. L., Mason, J., Compton, D., Stewart, J., and Ricard, N. (1999). The efficacy and safety of fexofenadine HCl and pseudoephedrine, alone and in combination, in seasonal allergic rhinitis. J. Allergy Clin. Immunol. 104, 100–106. doi: 10.1016/S0091-6749(99)70120-X
Tammali, R., Srivastava, S. K., and Ramana, K. V. (2011). Targeting aldose reductase for the treatment of cancer. Curr. Cancer Drug Targets 11, 560–571. doi: 10.2174/156800911795655958
Tan, N., Klein, E. A., Li, J., Moussa, A. S., and Jones, J. S. (2011). Statin use and risk of prostate cancer in a population of men who underwent biopsy. J. Urol. 186, 86–90. doi: 10.1016/j.juro.2011.03.004
Tan, X., Liu, P., Huang, Y., Zhou, L., Yang, Y., Wang, H., et al. (2016). Phosphoproteome analysis of invasion and metastasis-related factors in pancreatic cancer cells. PLoS One 11:e0152280. doi: 10.1371/journal.pone.0152280
Taplin, M. E., Manola, J., Oh, W. K., Kantoff, P. W., Bubley, G. J., Smith, M., et al. (2008). A phase II study of mifepristone (RU-486) in castration-resistant prostate cancer, with a correlative assessment of androgen-related hormones. BJU Int. 101, 1084–1089. doi: 10.1111/j.1464-410X.2008.07509.x
Thiele, I., Clancy, C. M., Heinken, A., and Fleming, R. M. T. (2017). Quantitative systems pharmacology and the personalized drug–microbiota–diet axis. Curr. Opin. Syst. Biol. 4, 43–52. doi: 10.1016/j.coisb.2017.06.001
Tieszen, C. R., Goyeneche, A. A., Brandhagen, B. N., Ortbahn, C. T., and Telleria, C. M. (2011). Antiprogestin mifepristone inhibits the growth of cancer cells of reproductive and non-reproductive origin regardless of progesterone receptor expression. BMC Cancer 11:207. doi: 10.1186/1471-2407-11-207
Tran, L. N. K., Kichenadasse, G., Butler, L. M., Centenera, M. M., Morel, K. L., Ormsby, R. J., et al. (2017). The combination of metformin and valproic acid induces synergistic apoptosis in the presence of p53 and androgen signaling in prostate cancer. Mol. Cancer Ther. 16, 2689–2700. doi: 10.1158/1535-7163.MCT-17-0074
Tsubamoto, H., Ueda, T., Inoue, K., Sakata, K., Shibahara, H., and Sonoda, T. (2017). Repurposing itraconazole as an anticancer agent (Review). Oncol. Lett. 14, 1240–1246. doi: 10.3892/ol.2017.6325
Turanli, B., Gulfidan, G., and Arga, K. Y. (2017). Transcriptomic-guided drug repositioning supported by a new bioinformatics search tool: geneXpharma. OMICS 21, 584–591. doi: 10.1089/omi.2017.0127
Uhlen, M., Zhang, C., Lee, S., Sjöstedt, E., Fagerberg, L., Bidkhori, G., et al. (2017). A pathology atlas of the human cancer transcriptome. Science 357:eaan2507. doi: 10.1126/science.aan2507
Ummanni, R., Mundt, F., Pospisil, H., Venz, S., Scharf, C., Barett, C., et al. (2011). Identification of clinically relevant protein targets in prostate cancer with 2D-DIGE coupled mass spectrometry and systems biology network platform. PLoS One 6:e16833. doi: 10.1371/journal.pone.0016833
Vanhaelen, Q., Mamoshina, P., Aliper, A. M., Artemov, A., Lezhnina, K., Ozerov, I., et al. (2017). Design of efficient computational workflows for in silico drug repurposing. Drug Discov. Today 22, 210–222. doi: 10.1016/j.drudis.2016.09.019
Vidal, A. C., Howard, L. E., Moreira, D. M., Castro-Santamaria, R., Andriole, G. L., and Freedland, S. J. (2015). Aspirin, NSAIDs, and risk of prostate cancer: results from the REDUCE study. Clin. Cancer Res. 21, 756–762. doi: 10.1158/1078-0432.CCR-14-2235
Wang, M., Shim, J. S., Li, R. J., Dang, Y., He, Q., Das, M., et al. (2014). Identification of an old antibiotic clofoctol as a novel activator of unfolded protein response pathways and an inhibitor of prostate cancer. Br. J. Pharmacol. 171, 4478–4489. doi: 10.1111/bph.12800
Wang, Y.-C., Chao, T.-K., Chang, C.-C., Yo, Y.-T., Yu, M.-H., and Lai, H.-C. (2013). Drug screening identifies niclosamide as an inhibitor of breast cancer stem-like cells. PLoS One 8:e74538. doi: 10.1371/journal.pone.0074538
Whitburn, J., Edwards, C. M., and Sooriakumaran, P. (2017). Metformin and prostate cancer: a new role for an old drug. Curr. Urol. Rep. 18:46. doi: 10.1007/s11934-017-0693-8
Würth, R., Thellung, S., Bajetto, A., Mazzanti, M., Florio, T., and Barbieri, F. (2016). Drug-repositioning opportunities for cancer therapy: novel molecular targets for known compounds. Drug Discov. Today 21, 190–199. doi: 10.1016/j.drudis.2015.09.017
Xia, Q., Sung, J., Chowdhury, W., Chen, C.-L., Höti, N., Shabbeer, S., et al. (2006). Chronic administration of valproic acid inhibits prostate cancer cell growth in vitro and in vivo. Cancer Res. 66, 7237–7244. doi: 10.1158/0008-5472.CAN-05-0487
Xia, W., Lan, X., Lv, J., Ma, J., Chen, W., Gao, L., et al. (2016). Valproic acid (VPA) suppresses the expression of SMAD4 in prostate carcinoma by up-regulating miR-34a. Int. J. Clin. Exp. Med. 9, 20466–20473.
Xu, T., Brandmaier, S., Messias, A. C., Herder, C., Draisma, H. H. M., Demirkan, A., et al. (2015). Effects of metformin on metabolite profiles and LDL cholesterol in patients with type 2 diabetes. Diabetes Care 38, 1858–1867. doi: 10.2337/dc15-0658
Xu, X., Shen, J., Mall, J. W., Myers, J. A., Huang, W., Blinder, L., et al. (1999). In vitro and in vivo antitumor activity of a novel immunomodulatory drug. Leflunomide 58, 1405–1413. doi: 10.1016/S0006-2952(99)00228-2
Xu, Y., Fang, F., Sun, Y., St Clair, D. K., and St Clair, W. H. (2010). RelB-dependent differential radiosensitization effect of STI571 on prostate cancer cells. Mol. Cancer Ther. 9, 803–812. doi: 10.1158/1535-7163.MCT-09-1001
Yizhak, K., Chaneton, B., Gottlieb, E., and Ruppin, E. (2015). Modeling cancer metabolism on a genome scale. Mol. Syst. Biol. 11, 817–817. doi: 10.15252/msb.20145307
Yizhak, K., Gabay, O., Cohen, H., and Ruppin, E. (2013). Model-based identification of drug targets that revert disrupted metabolism and its application to ageing. Nat. Commun. 4:2632. doi: 10.1038/ncomms3632
Yo, Y.-T., Lin, Y.-W., Wang, Y.-C., Balch, C., Huang, R.-L., Chan, M. W. Y., et al. (2012). Growth inhibition of ovarian tumor-initiating cells by Niclosamide. Mol. Cancer Ther. 11, 1703–1712. doi: 10.1158/1535-7163.MCT-12-0002
Yoshimura, R., Sano, H., Masuda, C., Kawamura, M., Tsubouchi, Y., Chargui, J., et al. (2000). Expression of cyclooxygenase-2 in prostate carcinoma. Cancer 89, 589–596. doi: 10.1002/1097-0142(20000801)89:3<589::AID-CNCR14>3.0.CO;2-C
Yu, S., Yang, X., Zhu, Y., Xie, F., Lu, Y., Yu, T., et al. (2015). Systems pharmacology of mifepristone (RU486) reveals its 47 hub targets and network: comprehensive analysis and pharmacological focus on FAK-Src-Paxillin complex. Sci. Rep. 5:7830. doi: 10.1038/srep07830
Zhang, C., and Hua, Q. (2016). Applications of genome-scale metabolic models in biotechnology and systems medicine. Front. Physiol. 6:413. doi: 10.3389/fphys.2015.00413
Zhang, H., Qian, D. Z., Tan, Y. S., Lee, K., Gao, P., Ren, Y. R., et al. (2008). Digoxin and other cardiac glycosides inhibit HIF-1alpha synthesis and block tumor growth. Proc. Natl. Acad. Sci. U.S.A. 105, 19579–19586. doi: 10.1073/pnas.0809763105
Zhang, Q., Wang, S., Yang, D., Pan, K., Li, L., and Yuan, S. (2016). Preclinical pharmacodynamic evaluation of antibiotic nitroxoline for anticancer drug repurposing. Oncol. Lett. 11, 3265–3272. doi: 10.3892/ol.2016.4380
Zhang, W., Edwards, A., Fan, W., Flemington, E. K., and Zhang, K. (2012). MiRNA-mRNA correlation-network modules in human prostate cancer and the differences between primary and metastatic tumor subtypes. PLoS One 7:e40130. doi: 10.1371/journal.pone.0040130
Keywords: prostate cancer, drug repositioning, non-cancer therapeutics, repurposing, approved drugs
Citation: Turanli B, Grøtli M, Boren J, Nielsen J, Uhlen M, Arga KY and Mardinoglu A (2018) Drug Repositioning for Effective Prostate Cancer Treatment. Front. Physiol. 9:500. doi: 10.3389/fphys.2018.00500
Received: 24 February 2018; Accepted: 18 April 2018;
Published: 15 May 2018.
Edited by:
Xiaogang Wu, Institute for Systems Biology, United StatesReviewed by:
Hyun Uk Kim, Korea Advanced Institute of Science & Technology (KAIST), South KoreaAo Zhou, Personalis, Inc., United States
Copyright © 2018 Turanli, Grøtli, Boren, Nielsen, Uhlen, Arga and Mardinoglu. This is an open-access article distributed under the terms of the Creative Commons Attribution License (CC BY). The use, distribution or reproduction in other forums is permitted, provided the original author(s) and the copyright owner are credited and that the original publication in this journal is cited, in accordance with accepted academic practice. No use, distribution or reproduction is permitted which does not comply with these terms.
*Correspondence: Adil Mardinoglu, YWRpbG1Ac2NpbGlmZWxhYi5zZQ==